Dollars and sense: The case for teaching personal finance
Americans aren’t good at managing their money — and there are signs that the problem is getting worse.
Already saddled with record levels of student debt, young adults today, for example, are even more unlikely to monitor their credit card debt and bank balances. Some people trick themselves into thinking that store refunds or anything less than $5 amount to free money . And too many people pay for online subscriptions they don’t use.
Thanks to the pioneering work of Stanford economist Annamaria Lusardi , numerous studies show how little people know about money. For two decades, Lusardi has been tracking financial literacy rates using three basic questions that she helped design and are now used as a standard measure around the world.
Her latest analysis of how Americans responded to those three questions in 2021 underscores their lack of financial know-how.
Only 53.1 percent of respondents demonstrated an understanding of how inflation works as prices on everything from cereal and cars were spiking. About two-thirds (69.4 percent) knew how to do a simple interest-rate calculation, but only 41.5 percent understood how, when it comes to investment risks, mutual funds are generally safer investments than a single company’s stock.
In all, just 28.5 percent of survey participants answered all three questions correctly, while the rest either got them wrong, or indicated they didn’t know.
The results are especially troubling as methods of managing money have evolved, says Lusardi, a globally recognized expert on personal finance who joined Stanford in September as a senior fellow at the Stanford Institute for Economic Policy Research (SIEPR) and director of the new Initiative for Financial Decision-Making. Workers now shoulder more of their retirement planning; consumers quickly and easily move money using their mobile phones; and investors make increasingly complex decisions.
“The world is changing really fast and we just expect people to have the skills to make financial decisions that have critical lifelong impacts,” says Lusardi, who is also a professor of finance (by courtesy) at the Graduate School of Business (GSB).
High rates of financial illiteracy are also problematic, she says, given today’s heightened economic uncertainty and growing wealth inequality. Respondents who were young, less educated, female, or not employed scored the lowest. Black Americans and Hispanics were also among the least financially literate.

A global pattern of illiteracy
Financial illiteracy, it turns out, is pervasive around the world, according to a newly published global analysis in the Journal of Financial Literacy and Wellbeing . Whether they are in a Nordic country with strong education systems, like Finland, or in a Latin American country, like Peru, which experienced inflation in 1990 upwards of 10,000 percent, most people don’t understand how money works, says Lusardi. And just like in the United States, the least knowledgeable tend to be women, racial minorities, the least-educated, and the unemployed.
Lusardi’s latest U.S. analysis — co-authored with Jialu Streeter , the executive director and a senior research scholar at SIEPR — is part of a special edition of the journal that includes analyses of 16 countries. Each study in the issue is based on the results of the “Big Three” questions that Lusardi and her longtime collaborator, economist Olivia Mitchell of The Wharton School at the University of Pennsylvania, crafted 20 years ago.
In 2011, Lusardi oversaw and contributed to a similar series of country comparisons — which yielded similar results and appeared in the Journal of Pension Economics & Finance .
“Financial illiteracy has been and continues to be a global phenomenon,” says Lusardi, who is one of the founders and inaugural editors of the Journal of Financial Literacy and Wellbeing , published by Cambridge University Press .
Why the ABCs of money matters
Beyond measuring and analyzing financial literacy rates, Lusardi’s extensive research has found how people who understand basic financial concepts are better at managing money. They save more for retirement, make smarter investment decisions, and manage their debts more effectively. Lusardi’s latest study shows that people who are financially literate are more likely to have money on hand to weather at least the early stages of an economic shock like a pandemic.
Lusardi has also shown that people think they know more about personal finance than they actually do, which she says makes them even more vulnerable to poor decision-making.
Stanford’s commitment to improving financial literacy is a key reason Lusardi says she joined The Farm. In addition to the Initiative for Financial Decision-Making — a collaboration between SIEPR, the GSB, and the Department of Economics in the School of Humanities and Sciences — Lusardi continues to serve as academic director of the Global Financial Literacy Excellence Center , which she founded in 2011. Prior to Stanford, Lusardi was the University Professor of Economics and Accountancy at The George Washington University.
The Big Three as global standard
In Lusardi, Stanford gains a leader in establishing financial literacy as a specialty within the field of economics.
Lusardi’s contributions to the field began in 2004, when The University of Michigan’s closely watched Health and Retirement Study added the so-called Big Three to a module dedicated to financial literacy and retirement planning. Then, in 2009, the financial education arm of the Financial Industry Regulatory Authority, which helps provide oversight of registered securities brokers and brokerage firms, began incorporating the same measures in its triennial survey of roughly 25,000 Americans.
Since then, other organizations, including central banks around the world, have integrated the Big Three into their respective assessments of household finances.
The underlying datasets in these surveys differ, but the results have uniformly shown that most people don’t understand how money, or financial systems broadly, functions, Lusardi says. In the U.S., this remained the case even after the Great Recession of 2008 and 2009 — the most severe economic downturn since the Great Depression — buffeted household finances.
“The continuous surprise is just how low financial literacy is in the United States and around the world,” says Lusardi, whose policy work includes advising the U.S. Treasury, the Organisation for Economic Co-operation and Development, and chairing the Italian Financial Education Committee in charge of designing a national strategy for financial literacy.
Solutions in education
To Lusardi, the answer to financial illiteracy lies in providing people with a basic education on the ABCs of personal finance.
“Developing personal finance skills is as important as learning how to read and write,” says Lusardi, who has been teaching financial literacy to undergraduate and graduate students for more than a decade. In fact, her move to Stanford is rooted in her experience working with SIEPR’s Michael Boskin and John Shoven to organize the first annual Teaching Personal Finance Conference in 2022.
“I’m not talking about expecting people to become Warren Buffet,” she says. “I’m talking about teaching people, especially the young, how to make savvy financial decisions. For first-generation or low-income students, it often means talking about topics they seldom discuss with their parents.”
Even as personal finance education has become somewhat of a cottage industry, results are mixed at best. Instructors, Lusardi says, often lack training and students tend to forget what they learn. In a 2014 journal publication , Lusardi and Mitchell noted that lack of sufficient funding or teacher training in financial education are still an issue; in a follow-up paper published this past fall, however, they said there’s reason for optimism.
More than half of U.S. states, for example, have added personal finance instruction as a high school graduation requirement. Universities, including Stanford, are now offering personal finance courses. Employers, too, are recognizing that financial anxiety hurts employee productivity and are sponsoring personal finance lessons in the workplace.
“Financial literacy education is really accelerating,” Lusardi says. “We’re finally seeing things turn around and, to me, that’s a very positive result.”
This story was updated on Feb. 15, 2024 with the new official name of Stanford's Initiative for Financial Decision-Making.
More News Topics
A plan for election-year rate cuts without the political chaos.
- Media Mention
- Politics and Media
- Money and Finance
Hospitals that pursue patients for unpaid bills will have to tell L.A. County
The world’s most valuable unused resource.

- Centers for Economic Education
- Certified Educator
- Partners and Donors
- Staff & Board of Directors
- Privacy Policy
- Free Workshops
- Professional Development & Certification
- Economic Decision-Making
- Mini-Economy Classroom Start-up Experience
- Performance Assessments
- Reading Makes Cents
- State Standards & Resources
- Stock Market Game™
- Virginia Economic Educator Awards
- Remote Learning Lessons
- Virginia Reads One Book
- Virginia Personal Finance Teacher Fellowship Program
- Life After High School School Resources
- Economics & Personal Finance Course
- Free Personal Finance & Economic Ed Teacher Resources
- Governor’s Challenge
- Register For SMG
- Classroom Resources
- Scholarships
- Stock Market Game Winners
- Frequently Asked Questions
Personal Finance Case Study
Regardless of whether you are able to participate in the Governor’s Challenge, we hope you will review the issues raised in the case study and consider using them in the classroom as group presentations and breakouts. Doing so will provide a good opportunity to apply many of the personal finance concepts and knowledge for your students. Below are examples of Case Studies. They also provide a good opportunity to exercise financial knowledge.
Click to download our Case Study Teacher Guidelines
Click to download the 2021 Governor’s Challenge Case Study
Click here to download the 2021 Governor’s Challenge Rubric
Click to download the 2021 Governor’s Challenge Financial Statements
Example 1 Personal Finance Case Study Example 1 Case Study Financial Statements Example 1 Rubric for Judging
Example 2 Personal Finance Case Study Example 2 Case Study Financial Statements Example 2 Rubric for Judging
2017 Governor’s Challenge Personal Finance Case Study
2016 Governor’s Challenge Personal Finance Case Study
2015 Governor’s Challenge Personal Finance Case Study
Yes, I'd like to help!
Sign up for educator emails.
Get resources for your K-12 classroom from the Virginia Council on Economic Education.
- Name * First Last
- School Name
- School Division School Division Not Teaching Independent Administrator Homeschool Private School Other Accomack County Albemarle County Alexandria Alleghany County Amelia County Amherst County Appomattox County Arlington County Augusta County Bath County Bedford County Bland County Botetourt County Bristol Brunswick County Buchanan County Buckingham County Buena Vista Campbell County Caroline County Carroll County Charles City County Charlotte County Charlottesville Chesapeake Chesterfield County Clarke County Colonial Beach Colonial Heights Covington Craig County Culpeper County Cumberland County Danville Dickenson County Dinwiddie County Essex County Fairfax County Falls Church Fauquier County Floyd County Fluvanna County Franklin City Franklin County Frederick County Fredericksburg Galax Giles County Gloucester County Goochland County Grayson County Greene County Greensville County Halifax County Hampton Hanover County Harrisonburg Henrico County Henry County Highland County Hopewell Isle of Wight County King and Queen County King George County King William County Lancaster County Lee County Lexington Loudoun County Louisa County Lunenburg County Lynchburg Madison County Manassas Manassas Park Martinsville Mathews County Mecklenburg County Middlesex County Montgomery County Nelson County New Kent County Newport News Norfolk Northampton County Northumberland County Norton Nottoway County Orange County Page County Patrick County Petersburg Pittsylvania County Poquoson Portsmouth Powhatan County Prince Edward County Prince George County Prince William County Pulaski County Radford Rappahannock County Richmond City Richmond County Roanoke City Roanoke County Rockbridge County Rockingham County Russell County Salem Scott County Shenandoah County Smyth County Southampton County Spotsylvania County Stafford County Staunton Suffolk Surry County Sussex County Tazewell County Virginia Beach Warren County Washington County Waynesboro West Point Westmoreland County Williamsburg-James City County Winchester Wise County Wythe County York County
- Middle School
- High School
- Comments This field is for validation purposes and should be left unchanged.
Information
- Author Services
Initiatives
You are accessing a machine-readable page. In order to be human-readable, please install an RSS reader.
All articles published by MDPI are made immediately available worldwide under an open access license. No special permission is required to reuse all or part of the article published by MDPI, including figures and tables. For articles published under an open access Creative Common CC BY license, any part of the article may be reused without permission provided that the original article is clearly cited. For more information, please refer to https://www.mdpi.com/openaccess .
Feature papers represent the most advanced research with significant potential for high impact in the field. A Feature Paper should be a substantial original Article that involves several techniques or approaches, provides an outlook for future research directions and describes possible research applications.
Feature papers are submitted upon individual invitation or recommendation by the scientific editors and must receive positive feedback from the reviewers.
Editor’s Choice articles are based on recommendations by the scientific editors of MDPI journals from around the world. Editors select a small number of articles recently published in the journal that they believe will be particularly interesting to readers, or important in the respective research area. The aim is to provide a snapshot of some of the most exciting work published in the various research areas of the journal.
Original Submission Date Received: .
- Active Journals
- Find a Journal
- Proceedings Series
- For Authors
- For Reviewers
- For Editors
- For Librarians
- For Publishers
- For Societies
- For Conference Organizers
- Open Access Policy
- Institutional Open Access Program
- Special Issues Guidelines
- Editorial Process
- Research and Publication Ethics
- Article Processing Charges
- Testimonials
- Preprints.org
- SciProfiles
- Encyclopedia
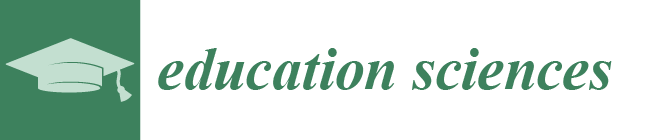
Article Menu
- Subscribe SciFeed
- Recommended Articles
- Google Scholar
- on Google Scholar
- Table of Contents
Find support for a specific problem in the support section of our website.
Please let us know what you think of our products and services.
Visit our dedicated information section to learn more about MDPI.
JSmol Viewer
Managing personal finance literacy in the united states: a case study.
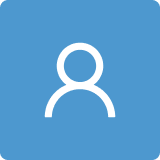
1. Introduction
2. review of related literature, 2.1. student debts, 2.2. financial literacy, 2.3. underqualified teachers, 2.4. pedagogy, 2.5. starting personal finances with youth, 3. methodology, 4. participants, 5.1. foresight, 5.2. co-curricular implementation, 5.3. math infusion, 5.4. esteemed personal finance, 5.5. education, 5.7. generational findings, 6. discussion, 7. conclusions, 8. limitations and future recommendations, author contributions, conflicts of interest.
- Dyer, S.P.; Lambeth, D.T.; Martin, E.P. Effects of multimodal instruction on personal finance skills for high school students. J. Sch. Educ. Technol. 2016 , 11 , 1–17. [ Google Scholar ]
- Hite, N.G.; Slocombe, T.E.; Railsback, B.; Miller, D. Personal finance education in recessionary time. J. Educ. Bus. 2011 , 86 , 253–257. [ Google Scholar ] [ CrossRef ]
- Franklin, D. Teacher Involvement in Implementing State Personal Finance Mandates. Ph.D. Thesis, Indiana State University, Terre Haute, Indiana, 2015. [ Google Scholar ]
- Danes, S.M.; Huddleston-Casas, C.; Boyce, L. Financial planning curriculum for teens: Impact evaluation. J. Financ. Couns. Plan. 1999 , 10 , 26–39. [ Google Scholar ]
- Harter, C.L.; Harter, J.F.R. Assessing the effectiveness of financial fitness for life in Eastern Kentucky. J. Appl. Econ. Policy 2009 , 28 , 20–33. [ Google Scholar ]
- Hilgert, M.A.; Hogarth, J.M.; Beverly, S. Household financial management: The connection between knowledge and behavior. Fed. Reserve Bull. 2003 , 89 , 309–322. [ Google Scholar ]
- Campbell, J.L. The U.S. financial crisis: Lessons for theories of institutional complementarity. Socio-Econ. Rev. 2011 , 9 , 211–234. [ Google Scholar ] [ CrossRef ]
- Financial Crisis Inquiry Commission & United States. The Financial Crisis Inquiry Report, Authorized Edition: Final Report of the National Commission on the Causes of the Financial and Economic Crisis in the United States ; Public Affairs: New York, NY, USA, 2011; pp. 1–623. [ Google Scholar ]
- Acharya, V.V.; Richardson, M. Restoring Financial Stability: How to Repair a Failed System ; John Wiley & Sons: Hoboken, NJ, USA, 2009; pp. 1–381. [ Google Scholar ]
- Claessens, S.; Kodres, L.E. The Regulatory Responses to the Global Financial Crisis: Some Uncomfortable Questions ; International Monetary Fund: Washington, DC, USA, 2014; pp. 1–29. [ Google Scholar ]
- Dinwoodie, J. Ignorance is not a bliss: Financial illiteracy, the mortgage market collapse, and the global economic crisis. Univ. Miami Bus. Law Rev. 2010 , 18 , 181–206. [ Google Scholar ] [ CrossRef ]
- Frank, H. The Financial crisis of 2008: A clarion call to include economic policy and financial illiteracy on public administration’s intellectual radar screen. Adm. Theory Prax. 2009 , 31 , 409–416. [ Google Scholar ] [ CrossRef ]
- Taylor, M.W.; Arner, D.W. Global regulation for global markets? In Lessons from the Financial Crisis: Causes, Consequences, and Our Economic Future ; Kolb, R., Ed.; Wiley: Hoboken, NJ, USA, 2010; pp. 383–391. [ Google Scholar ]
- Lusardi, A.; Tufano, P. Debt literacy, financial experiences, and overindebtedness. J. Pension Econ. Financ. 2015 , 14 , 332–368. [ Google Scholar ] [ CrossRef ] [ Green Version ]
- Boatman, A.; Evans, B.J. How financial literacy, federal aid knowledge, and credit market experience predict loan aversion for education. Am. Acad. Political Soc. Sci. 2017 , 671 , 49–68. [ Google Scholar ] [ CrossRef ]
- Baum, S.; Schwartz, S. Student Aid, Student Behavior, and Educational Attainment ; George Washington University: Washington, DC, USA, 2013; pp. 1–26. [ Google Scholar ]
- Dynarski, S. How to and how not to manage student debt. The Milken Institute Review. Available online: https://www.milkenreview.org/articles/how-to-and-how-not-to-manage-student-debt (accessed on 1 March 2019).
- Federal Reserve Bank of New York. Quarterly Report on Household Debt and Credit ; Federal Reserve Bank: New York, NY, USA, 2016; pp. 1–33. [ Google Scholar ]
- Poll, H. The 2017 Consumer Financial Literacy Survey, National Foundation for Credit Counseling. Available online: https://nfcc.org/wp-content/uploads/2017/03/NFCC_BECU_2017-FLS_datasheet-with-key-findings.pdf (accessed on 1 March 2019).
- Clark, R.L.; Morrill, M.S.; Allen, S.G. The role of financial literacy in determining retirement plans. Econ. Inq. 2012 , 50 , 851–866. [ Google Scholar ] [ CrossRef ]
- Lusardi, A.; Mitchell, O.S. Financial literacy and retirement preparedness: Evidence and implications for financial education. Bus. Econ. 2007 , 42 , 35–44. [ Google Scholar ] [ CrossRef ]
- Carpenter, M. School programs aim to teach kids about money because parents don’t. Pittsburgh Post-Gazette. Available online: https://archives.post-gazette.com/image/96551396 (accessed on 1 March 2019).
- Green, A. Push for financial literacy spreads to schools. Christ. Sci. Monit. 2009 , 101 , 13. [ Google Scholar ]
- Lusardi, A.; Mitchell, O.S. Financial literacy and retirement planning in the United States. J. Pension Econ. Financ. 2011 , 10 , 509–525. [ Google Scholar ] [ CrossRef ] [ Green Version ]
- Lusardi, A.; Scheresberg, C. Financial literacy and high-cost borrowing in the United States ; NBER Working Papers; National Bureau of Economic Research: Cambridge, MA, USA, 2013; Volume 2, pp. 1–41. [ Google Scholar ]
- Mandell, L. The Financial Literacy of Young American Adults: Results of 2008 National Jump $ Start Coalition Survey of High School Seniors and College Students ; Jumpstart Colation: Washington, DC, USA, 2008; pp. 1–243. [ Google Scholar ]
- Kadlec, D. Why We Want but Can’t Have Personal Finance in Schools. TIME . Available online: http://business.time.com/2013/10/10/why-we-want-but-cant-have-personal-finance-in-schools/ (accessed on 1 March 2019).
- Walstad, W. Economics education in U.S. high schools. J. Econ. Perspect. 2001 , 15 , 195–210. [ Google Scholar ] [ CrossRef ]
- Finkelstein, N.; Hanson, T.; Huang, C.; Hirschman, B.; Huang, M. Effects of Problem Based Economics on High School Economics Instruction ; US Department of Education: Washington, DC, USA, 2011; pp. 1–102.
- Atchley, R.C. Educating the public about personal finance: A call for action. J. Financ. Serv. Prof. 1998 , 52 , 28–32. [ Google Scholar ]
- Blue, L.; Grootenboer, P.; Brimble, M. Financial literacy education in the curriculum: Making the grade or missing the mark? Int. Rev. Econ. Educ. 2014 , 16 , 51–62. [ Google Scholar ] [ CrossRef ] [ Green Version ]
- Way, W.L.; Holden, K.C. Teachers’ background and capacity to teach personal finance: Results of a national study. J. Financ. Couns. Plan. 2009 , 20 , 64–78. [ Google Scholar ]
- Gundmunson, C.G.; Zuiker, V.S.; Katras, M.J.; Sabri, M.F. Enhancing personal and family finance courses using case studies. Coll. Stud. J. 2015 , 49 , 321–330. [ Google Scholar ]
- Haynes, D.; Chinadle, N. Private sector/educator collaboration: Project improves financial, economic literacy of America’s youth. J. Fam. Consum. Sci. 2007 , 99 , 8–10. [ Google Scholar ]
- Mandell, L.; Klein, L. The impact of financial literacy education on subsequent financial behavior. J. Financ. Couns. Plan. 2009 , 20 , 15–24. [ Google Scholar ]
- Walstad, W.B.; Salemi, M.K. Results from a faculty development program in teaching economics. J. Econ. Educ. 2011 , 42 , 283–293. [ Google Scholar ] [ CrossRef ]
- Totenhagen, C.; Casper, D.; Faber, K.; Bosch, L.; Wiggs, C.; Borden, L. Youth financial literacy: A review of key considerations and promising delivery methods. J. Fam. Econ. Issues 2015 , 36 , 167. [ Google Scholar ] [ CrossRef ]
- Miller, D.; Hite, N.G.; Slocombe, T.; Railsback, B. Student Perspectives toward key personal finance variables. Delta Pi Epsil. J. 2010 , 52 , 168–181. [ Google Scholar ]
- Mandell, L.; Klein, L.S. Motivation and financial literacy. Financ. Serv. Rev. 2007 , 16 , 105–116. [ Google Scholar ]
- The 2016 National State of Financial & Economic Education. Available online: http://www.surveyofthestates.com/#situation-1 (accessed on 3 March 2019).
- McCormick, M. The effectiveness of youth financial education: A review of the literature. J. Financ. Couns. Plan. 2009 , 20 , 70–83. [ Google Scholar ]
- Starting Younger: Evidence Supporting the Effectiveness of Personal Finance Education for Pre-High School Students. Available online: http://www.nationaltheatre.com/ntccom/pdfs/financialliteracy.pdf (accessed on 3 March 2019).
- Godfrey, N.S. Making our students smart about money. Educ. Dig. 2006 , 71 , 21–26. [ Google Scholar ]
- Chen, W.; Heath, J.A. The efficacy of financial education in the early grades: Results from a statewide program. In Reframing Financial Literacy: Exploring the Value of Social Currency ; Lucey, T., Lucey, J., Eds.; Information Age Publishing, Inc.: Charlotte, NC, USA, 2012; pp. 189–208. [ Google Scholar ]
- Amagir, A.; Groot, W.; Maassen van den Brink, H.; Wilschut, A. A review of financial-literacy education programs for children and adolescents. Citizsh. Soc. Econ. Educ. 2018 , 17 , 56–80. [ Google Scholar ] [ CrossRef ]
- Morton, J. The interdependence of economic and personal finance education. Soc. Educ. 2005 , 69 , 66–69. [ Google Scholar ]
- Crowe, S.; Creswell, K.; Robertson, A.; Huby, G.; Avery, A.; Sheikh, A. The case study approach. BMC Med Res. Methodol. 2011 , 11 , 100–108. [ Google Scholar ] [ CrossRef ]
- Yin, R.K. The case study crisis: Some answers. Adm. Sci. Q. 1981 , 26 , 58–65. [ Google Scholar ] [ CrossRef ]
- Creswell, J. Qualitative Inquiry & Research Design: Choosing among Five Approaches , 3rd ed.; Sage: Thousand Oaks, CA, USA, 2013; pp. 1–442. [ Google Scholar ]
- Charmaz, K. Constructing Grounded Theory , 2nd ed.; Sage: Thousand Oaks, CA, USA, 2014; pp. 1–379. [ Google Scholar ]
- Strauss, A.L. Qualitative Analysis for Social Scientists ; Cambridge University Press: New York, NY, USA, 1987; pp. 1–317. [ Google Scholar ]
- Saldana, J. The Coding Manual for Qualitative Researchers , 3rd ed.; Sage: Thousand Oaks, CA, USA, 2016; pp. 1–333. [ Google Scholar ]
- Smola, K.; Sutton, C. Generational differences: Revisiting generational work values for the new millennium. J. Organ. Behav. 2002 , 23 , 363–382. [ Google Scholar ] [ CrossRef ]
- Taylor, P. The Next America: Boomers, Millennials, and the Looming Generational Showdown ; Public Affairs: New York, NY, USA, 2014; pp. 1–345. [ Google Scholar ]
- Walstad, W.; Urban, C.; Asarta, C.; Breitbach, E.; Bosshard, W.; Heath, J.; O’Neill, B.; Wagner, J.; Xio, J. Perspectives on evaluation in financial education: Landscape, issues, and studies. J. Econ. Educ. 2017 , 48 , 93–112. [ Google Scholar ] [ CrossRef ]
- Walstad, W.; Rebeck, K. The test of financial literacy: Development and measurement characteristics. J. Econ. Educ. 2017 , 48 , 113–122. [ Google Scholar ] [ CrossRef ]
- Financial Fitness for Life. Available online: http://fffl.councilforeconed.org/ (accessed on 4 March 2019).
- Batty, M.; Collins, J.M.; Odders-White, E. Experimental evidence on the effects of financial education on elementary school students’ knowledge, behavior, and attitudes. J. Consum. Aff. 2015 , 49 , 69–96. [ Google Scholar ] [ CrossRef ]
- National Endowment for Financial Education. Available online: https://www.nefe.org/What-We-Do (accessed on 5 March 2019).
- Suiter, M.C.; Wolla, S.A. Considering the times: Resources for teaching economic and financial literacy in light of the great recession. Soc. Educ. 2015 , 79 , 74–77. [ Google Scholar ]
- Jacob, K. Evaluating Your Financial Literacy Program: A Practical Guide ; Woodstock Institute: Chicago, IL, USA, 2002; pp. 1–19. [ Google Scholar ]
Participant | Generation | Gender |
---|---|---|
P1 | Gen X | M |
P2 | Gen X | F |
P3 | Gen Z | M |
P4 | Gen Z | M |
P5 | Gen X | F |
P6 | Gen Z | M |
P7 | Gen Z | F |
P8 | Gen X | F |
P9 | Gen X | F |
P10 | Gen X | F |
P11 | Gen Z | F |
P12 | Gen Z | F |
P13 | Millennial | M |
P14 | Millennial | M |
P15 | Millennial | F |
Share and Cite
Beck, J.J.; Garris, R.O., III. Managing Personal Finance Literacy in the United States: A Case Study. Educ. Sci. 2019 , 9 , 129. https://doi.org/10.3390/educsci9020129
Beck JJ, Garris RO III. Managing Personal Finance Literacy in the United States: A Case Study. Education Sciences . 2019; 9(2):129. https://doi.org/10.3390/educsci9020129
Beck, Joshua J., and Richard O. Garris, III. 2019. "Managing Personal Finance Literacy in the United States: A Case Study" Education Sciences 9, no. 2: 129. https://doi.org/10.3390/educsci9020129
Building Case Studies
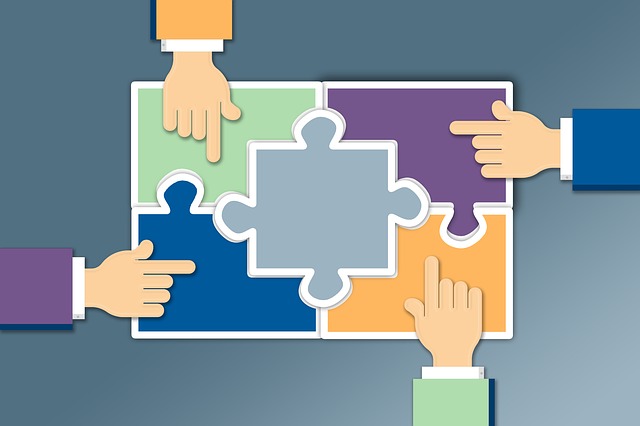
At some point in your business career you will likely be asked to build a case study. Whether it’s for school or for work, building a case study is a very methodical task. While case studies will differ across companies and sectors, your process should remain the same. When conducting a case study, you should try to include these core pieces: a summary of the company’s background, analysis of their background, the company’s internal strengths and weaknesses, their opportunities and threats, the external environment that they compete in, an evaluation of your SWOT analysis, and some recommendations to remedy potential issues you find. After figuring out which company you’re looking to examine, the first step will be to write a summary of that company’s background.
Summarizing a Company’s Background
A summary of a company’s background is just as it sounds — a brief synopsis of who the company is and what they do. Here is a summary of Ford Motor Co. found on CNNMoney’s website:
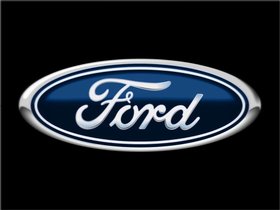
The summary is short and succinct. It explains who Ford is, which segments they operate in, and a brief explanation of what they do in each of their segments. When making a summary of your own, you’ll want to keep it short and sweet; the summary is only meant to introduce the company and what they do, it’s not meant to be comprehensive. Once you’ve summarized your company’s background your next step will be to start analyzing their history, development, and growth.
Analyzing a Company’s Background
Now that you’ve summarized the company, you’ll want to more thoroughly understand their background to get an idea of how they got to where they are today. Primarily, you’ll want to analyze their history, development, and growth.
When mulling through a company’s history you’ll start at their inception. You will be looking backwards to see how they got to where they are. The good, the bad, the ugly, the fantastic; you’ll want to see how your company dealt with both their successes and their failures. It can show you how they handle certain situations. When faced with competition, do they try to be more creative to get a leg up, or do they budget more effectively and out finance their opposition? Finding the answers to these questions can explain how the company will likely handle similar situations in the future.
Development and growth shows how the company developed and grew from the beginning until now. Did they bring a revolutionary idea to the market and change an industry like Ford, or did they take something old and put a new spin on it? Did they burst onto the scene and never look back or did they slowly build into a profitable company? When analyzing your company’s growth and development you’ll want to see how successful they were in growing their business. With exponential increases in available capital, a successful business will find ways to continue growing at a healthy rate — until a certain point. Eventually every company will reach their growth potential and will look to extract as much value from their business as possible, such as General Electric. Observing how well your company uses their cash flows to grow and develop their business will give you an insight into how they plan to develop in the future and how likely they are to succeed.
SWOT Analysis
After you’ve finished analyzing your company’s background, you will begin conducting a Strengths, Weaknesses, Opportunities, and Threats (SWOT) analysis. The first part of your SWOT analysis will be identifying the company’s internal strengths and weaknesses.
Internal Strengths and Weaknesses
When analyzing a company’s internal strengths and weaknesses, you should be looking only at things within the company’s control. This will typically include the company’s culture, expertise, and resources.
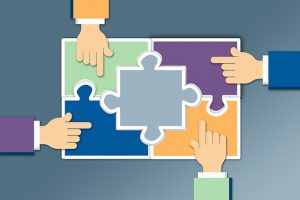
Management Structure
Part of looking at a company’s culture will involve understanding their management structure and control systems. The management structure shows who is in authority and how work is divided. There are three main types of management structures: horizontal, vertical, and matrixed.
Horizontal management is one where there is no middle management between staff members and executives. In this style of management, each supervisor has a larger number of people to manage. This means that a manager in a horizontal structure will have more responsibility than one in a vertical structure. The benefit to this is that information moves quicker, since there is no middle management to pass through. It also benefits workers, as it allows them to be more autonomous and directly involved in the decision-making process. Horizontal management is more common in companies that are growing and trying to harbor innovation. Since innovation requires lots of free-flowing information, it is better when there are fewer people it must pass through to reach the top executives.
Vertical management is one where information is passed from the top to the bottom — from supervisors to staff members. You can think of vertical management as a pyramid — where you have a CEO at the top then three vice presidents then five senior managers and so on. Unlike horizontal management, vertical management is very rigid and bureaucratic. Information flows in a slow, steady manner, passing from supervisor to subordinate until it reaches the very bottom. This style of management is more common in larger conglomerates like General Electric, who would prefer more executives since they operate in many different sectors. These companies don’t require information to move as quickly, so they’d rather it be refined as it moves through the chain of command.
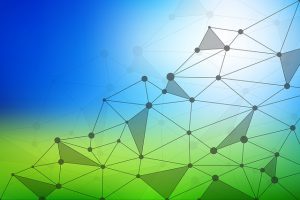
A company’s expertise involves how knowledgeable they are in their given field. Expertise is measured by comparing how vital a company’s skills are relative to the market they compete in. If your company has skilled employees in computer software, but there are other companies with similar skills, their expertise may not be considered a strength. On the other hand, if your company is significantly more knowledgeable than their competitors, it could be considered a strength. While expertise involves an external factor by comparing your company’s knowledge to their competitors, it’s something that is internally controlled. A company can control their expertise by hiring and investing in highly skilled employees; they cannot control who their competitors hire and how they invest in their skills.
A company’s resources include their finances, assets, and infrastructure. Analyzing a company’s resources involves observing how they manage their resources compared to their competitors.
Corporate Level Strategy
Using a company’s resources, you can begin to examine their corporate level strategy. Corporate level strategy is concerned with the company’s business decisions and how they affect the entire organization. Financials, mergers and acquisition, and the overall management of resources are all things involved with corporate level strategy.
Usually, companies within the same industry will have similar corporate level strategies. For example, companies in the telecom industry have higher levels of debt than companies in other industries. This is because they must make larger initial investments in infrastructure before they can start to deliver their products. However, that doesn’t mean companies who break from the industry tradition are always weaker, in fact, it could be a strength.
Understanding your company’s corporate level strategy will help explain some of the decisions they’ve made, as well as allow you to formulate recommendations that help them enhance their strategy.
Intellectual Property
A company’s resources can also include their intellectual property. Intellectual property is a creative invention that a company has legal access to use commercially. This includes trademarks, patents, and copyrights. Intellectual property can be a big internal strength for a company, especially if it restricts competitors from being covered under fair use, such as Apples pinch-to-zoom feature.
Now that you’ve covered the Strengths and Weaknesses portion of your SWOT analysis, you’ll need to find identify the company’s Opportunities and Threats. This is done by looking at the external environment of your company.
External Environment
The external environment is composed of all the outside factors that impact your company’s operations. These are the factors that your company doesn’t have control over. It can include their competitors, their customers, the government, and the economic climate. From the external environment, you will be able to observe potential threats to your company as well as potential opportunities they may have.
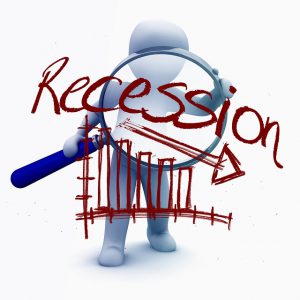
Opportunities
Opportunities in your SWOT analysis will consist of ways that your company can potentially improve their business operations. These will include both internal and external strengths. Building on employee knowledge, launching of a new product, or a positive economic forecast are all potential opportunities for a company. The primary goal of an opportunity is to positively affect a company’s business operations.
Once you’ve completed your SWOT analysis, you will then want to evaluate what you’ve found. What you find will likely be part of the recommendations that you formulate to your company.
Evaluating your SWOT analysis
By conducting your Strengths, Weaknesses, Opportunities, and Threats analysis, you will have identified what your company does well, what they need to work on, what opportunities are present for them, and what threats they may face. The information you’ve discovered will then be used to help formulate recommendations to the company.
Formulating Recommendations
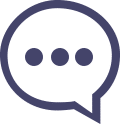
Get PersonalFinanceLab
This lesson is part of the PersonalFinanceLab curriculum library. Schools with a PersonalFinanceLab.com site license can get this lesson, plus our full library of 300 others, along with our budgeting game, stock game, and automatically-graded assessments for their classroom - complete with LMS integration and rostering support!
- Search Search
From Our Blog
- Feature Highlight – Updated Lesson Plans
- Feature Highlight – Stocks by Sector
- Planning For Unexpected Expenses
- Feature Highlight – Student Stock Comparison Tool
- Feature Highlight – Weekend Choices
Personal Finance Lab
Finder’s case study database: Share your story
Share your personal finance experiences and you could get £60 if we use your story..
Finder’s research team sometimes needs case studies who have had positive or negative experiences with their personal finances to accompany our research in order to help bring it to life. We are therefore building a database of case studies who would be willing to share their personal finance stories with us.
What’s in it for me?
If we use your case study in research that we send to journalists, we will pay you £60 for your contribution. This may also be featured on our website, like the example below. We also need case studies solely for our website from time to time and will pay £20 for people we select for this (if you haven’t already given your testimony as a press case study). Last but not least, you get to share your story and help other people with their finances!
Case study: Bank branch closure has left Samantha stressed
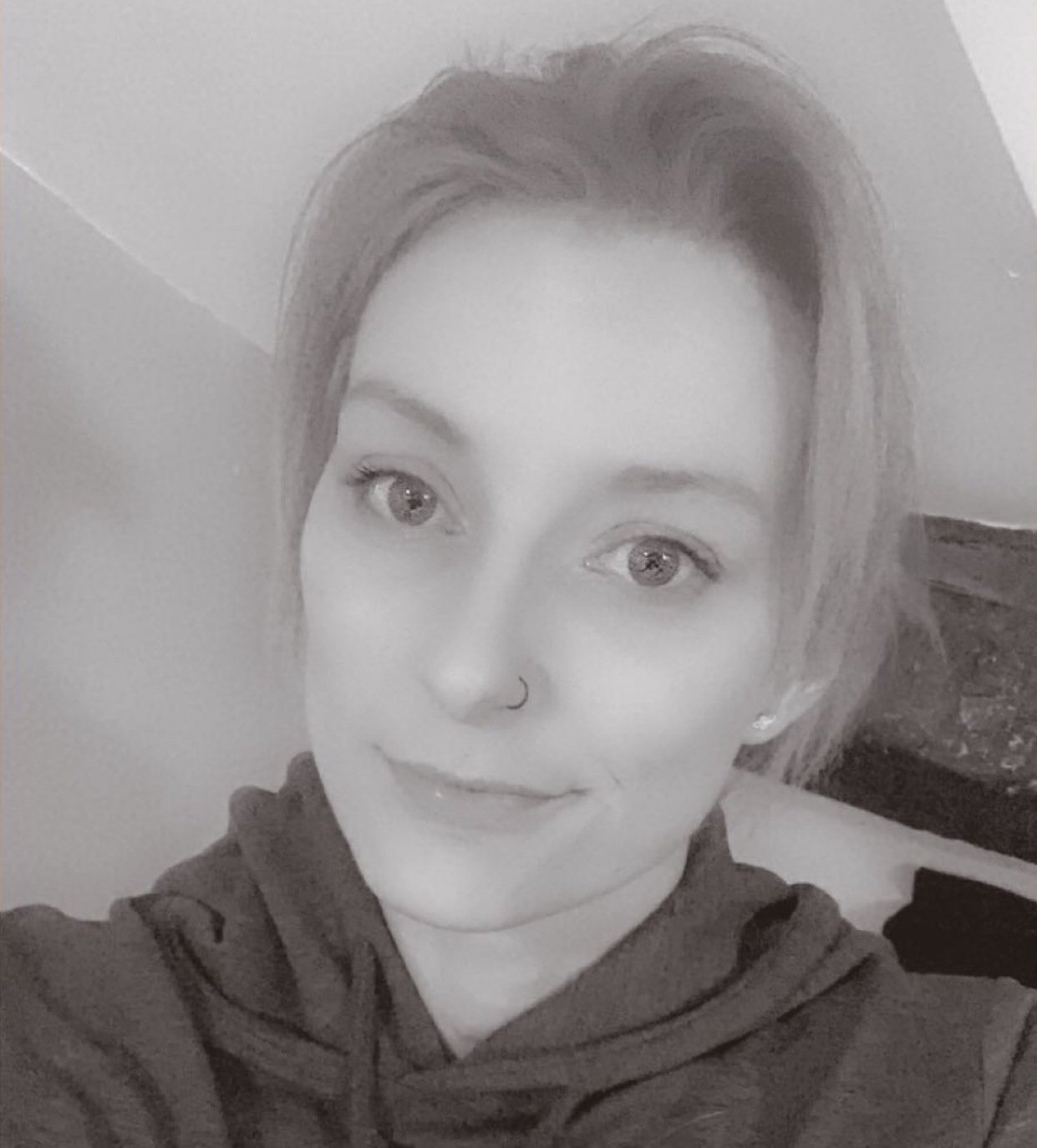
Samantha Evans
It has made sure it's harder for me to access actual face-to-face support. It's meant that if I have a problem, I have to call or use my mobile banking app to access support.
There was no support offered or in place. To be honest, there wasn't very much warning either, and it was only by chance that I discovered it was closing.
What do I have to do?
To submit your story and be in with a chance of earning £60 for being a case study, you need to fill in this form.
The more detail you include in your response, the better, as this will increase the likelihood that we choose you to be part of our research! Experiences can be positive (e.g. a provider you have been impressed by or success you’ve had with a side hustle) or negative (you’ve been dealing with issues in a particular area or a company has treated you badly).
To give some further examples, this could be you talking about how you have a digital-only bank account (like Starling and Monzo) or about the steps you’ve taken to deal with the cost of living crisis or your mortgage.
Please leave your email below and if you prefer to be contacted via Twitter, you can also follow our head of research, Matt, on twitter and he might contact you on there as opposed to email. His handle is @mckmatt10 (https://twitter.com/MckMatt10).
If we decide to use your case study in our research, your story and a picture could appear in national news publications.
If you’re not comfortable with having your name and picture in the press, please don’t fill out this form.
Submit your case study
What might it look like if my case study is used.
The research, including your story and a picture, could appear in national news sites. In the unlikely event a TV news show wanted to cover the research, you may also be asked to be filmed for this.
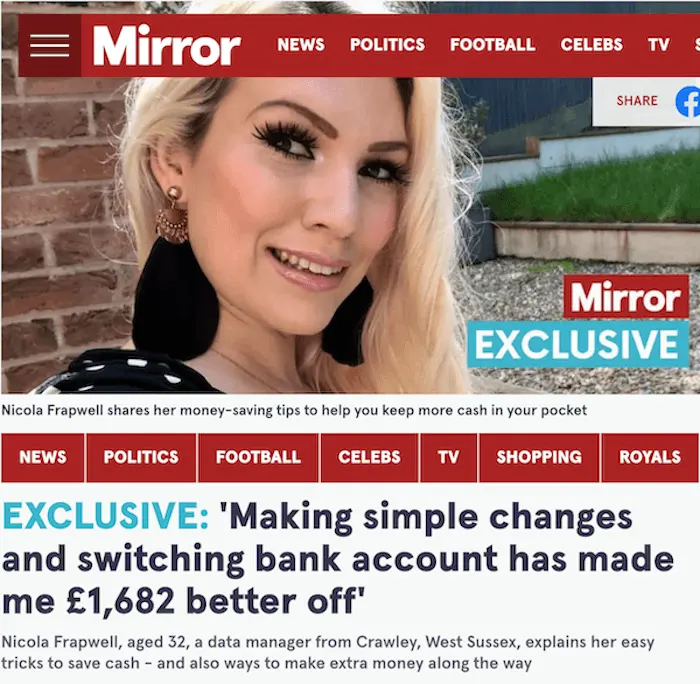
Why do we use case studies?
Case studies bring our research to life and help people understand the real life stories behind personal finance issues, whether it’s bank accounts, investing, credit cards or the general cost of living (or anything else relating to finance!)
What happens if I no longer want to be part of the case study database?
Please direct any questions, concerns or requests to be taken off the database via email to: [email protected] and you can see our terms and policy here: https://www.finder.com/uk/privacy-policy
Click here for more research. For all media enquiries, please contact –
Matt Mckenna UK Head of Communications T: +44 20 8191 8806
More guides on Finder
Finder Studios creates multimedia sponsored content with partners. Engage a new audience with finance content like they’ve never seen before.
Our Editorial Review Board and expert contributors are a key part of Finder’s content. Here are the opportunities for experts who work with us.
Contact details for organisations offering free money guidance, debt support, help with benefits and support with complaints about a provider.
A note from Frank, Fred and J.
If you’d like to help, you can donate through one of these registered charities which are supporting Ukrainians.
How to share your product experiences with Finder and our readers.
Please rate your experience of finder.com!
We love hearing from our users, so pick up the phone, send us an email, tweet us, post to our wall or pop by the office. We’re more than happy to answer your questions and help you out any way we can.
Say ‘Hi’ to the personal finance pros producing the content at Finder UK!
About our partnerships.
How likely would you be to recommend Finder to a friend or colleague?
Our goal is to create the best possible product, and your thoughts, ideas and suggestions play a major role in helping us identify opportunities to improve.
Advertiser Disclosure
finder.com is an independent comparison platform and information service that aims to provide you with the tools you need to make better decisions. While we are independent, the offers that appear on this site are from companies from which finder.com receives compensation. We may receive compensation from our partners for placement of their products or services. We may also receive compensation if you click on certain links posted on our site. While compensation arrangements may affect the order, position or placement of product information, it doesn't influence our assessment of those products. Please don't interpret the order in which products appear on our Site as any endorsement or recommendation from us. finder.com compares a wide range of products, providers and services but we don't provide information on all available products, providers or services. Please appreciate that there may be other options available to you than the products, providers or services covered by our service.
We update our data regularly, but information can change between updates. Confirm details with the provider you're interested in before making a decision.
Learn how we maintain accuracy on our site.
Newly Launched - AI Presentation Maker

Researched by Consultants from Top-Tier Management Companies

AI PPT Maker
Powerpoint Templates
Icon Bundle
Kpi Dashboard
Professional
Business Plans
Swot Analysis
Gantt Chart
Business Proposal
Marketing Plan
Project Management
Business Case
Business Model
Cyber Security
Business PPT
Digital Marketing
Digital Transformation
Human Resources
Product Management
Artificial Intelligence
Company Profile
Acknowledgement PPT
PPT Presentation
Reports Brochures
One Page Pitch
Interview PPT
All Categories
Must-Have Financial Case Study Examples with Samples and Templates
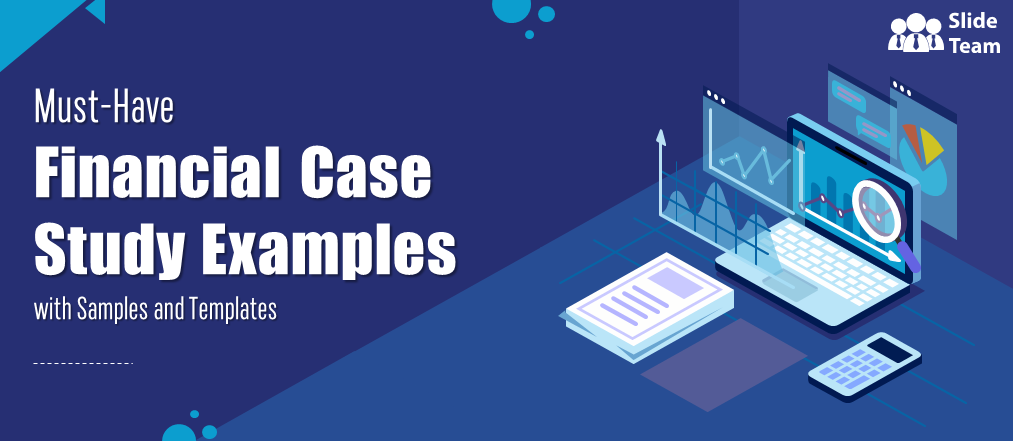
Mayuri Gangwal

Case studies are valuable tools for understanding the real-world applications of financial concepts and strategies. They provide insights into practical scenarios, showcasing the decision-making processes and outcomes in various financial situations. Whether you are a student, professional, entrepreneur, having access to well-crafted financial case study templates can be immensely beneficial in developing a deeper understanding of financial principles and honing your analytical skills.
SlideTeam’s premium PPT templates help you grasp complex financial concepts like investment analysis, financial planning, risk management, etc. Each case study offers a unique scenario, presenting a problem or challenge that requires thoughtful analysis and strategic decision-making.
By using these content-ready slides, you can enhance your problem-solving abilities, learn from real-world success stories and mistakes, and gain valuable insights into the intricacies of financial decision-making. The included samples and templates are practical tools for structuring your case studies, enabling you to apply your knowledge and skills to different financial scenarios.
Whether preparing for exams, a professional seeking to broaden your financial expertise, or an entrepreneur looking to make informed business decisions, these financial case study examples, samples, and templates are indispensable resources to elevate your financial understanding and make well-informed decisions in your personal or professional life.
Financial Case Study Templates
Template 1: financial case study environment business solution problems.
Introducing our ready to use template designed to elevate your content and make you look like a presentation pro. With a wide range of PPT slides covering various topics, this deck encompasses all the core areas of your business needs.
The deck focuses on Financial Case Study Environment Business Solution Problems, offering professionally designed templates that combine suitable graphics and relevant content. With eight slides, thoughtfully crafted to enhance your message and captivate your audience.
Don't miss out on this opportunity to impress your audience with visually stunning slides and compelling content. Click the download button and access our pre-designed PPT presentation and take your presentations to the next level. We also have templates to propose a business case if you aim for a higher company turnover.

Download Now
Template 2: Case Study for Financial Management PowerPoint Template
Introducing our captivating case study template designed to provide an environment conducive to productive discussions and effective decision-making. This template is perfect for showcasing real-life examples and analyzing financial management scenarios visually engagingly.
With its three-stage process, this template simplifies complex concepts and guides your audience through the essential components of a comprehensive business case study. It enables you to present your findings, solutions, and recommendations.
Whether you are analyzing past financial performances, identifying challenges , or proposing solutions, this template provides a flexible framework for organizing and presenting your ideas. You can also elevate your financial management presentations with our marketing Case Study for Financial Management PowerPoint Template . Download it now and unlock a wealth of possibilities to engage your audience, foster integration, and showcase your expertise in financial management.
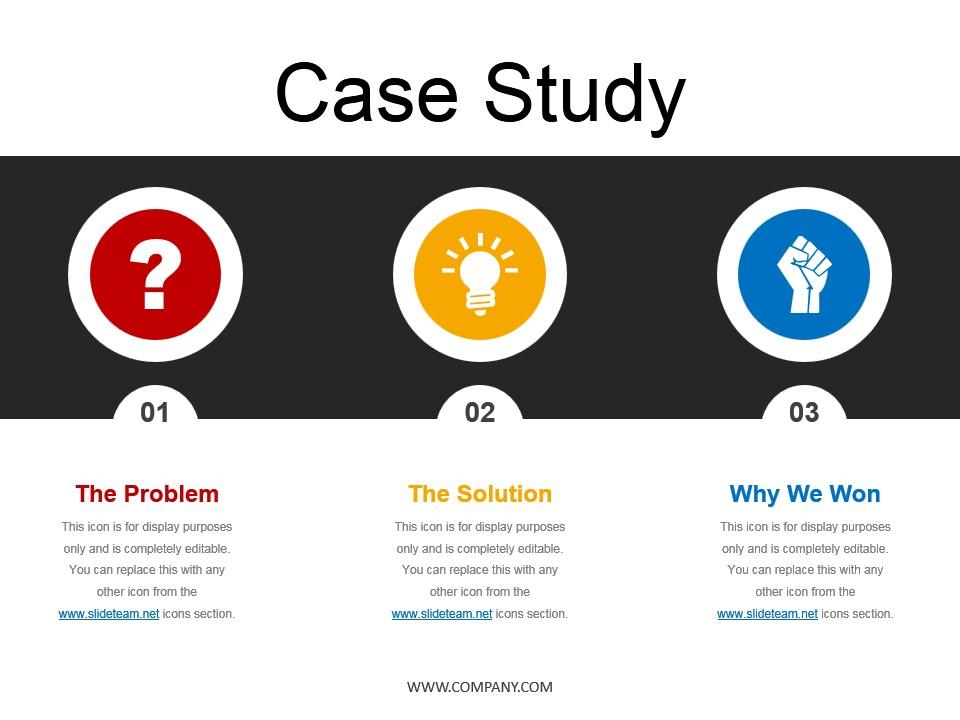
Download Now
Conclusion
Financial case studies are invaluable tools for understanding real-world financial scenarios and developing practical solutions. By examining concrete examples, individuals and organizations can gain insights into financial challenges, apply analytical techniques, and make informed decisions.
This article has highlighted the importance of collecting financial case study examples and accompanying samples and templates as valuable resources for learning and applying financial principles in various contexts. These resources can serve as guides for conducting comprehensive analyses, formulating recommendations, and ultimately achieving financial success.
FAQs on Financial Case Study
What is a case study in finance.
A case study in finance is an in-depth analysis of a specific financial situation, company, investment, or financial strategy. It involves examining real-world scenarios, often based on actual events, to understand and evaluate the financial implications, decision-making processes, and outcomes.
In finance, case studies are commonly used as a teaching and learning tool to assess and explore complex financial issues in academic and professional settings. They provide a practical approach to understanding financial theories, concepts, and practices by applying them to real-life situations.
A finance case study typically involves the following elements:
- Background: The case study begins by presenting relevant information about the company, industry, or financial situation under examination. This includes details about the organization's financial statements, market conditions, competitive landscape, and other pertinent background information.
- Problem or Challenge: The case study outlines the specific financial problem or challenge that needs to be addressed. This could be related to financial analysis, investment decisions, capital budgeting, risk management, financial restructuring, or any other financial aspect of the organization.
- Data Analysis: The case study analyzes financial data, such as income statements, balance sheets, cash flow statements, and key financial ratios. Various financial analysis tools and techniques, such as ratio analysis, discounted cash flow analysis, or valuation models, may be used to evaluate the situation.
- Alternatives and Solutions: Based on the analysis, different alternatives or solutions are identified to address the financial problem or challenge. These could include recommendations for financial strategies, investment decisions, capital allocation, cost reduction measures, or other relevant actions.
- Decision-Making and Implementation: The case study explores the decision-making process, considering risk, return, financial feasibility, and strategic considerations. It also discusses the potential implementation of the recommended solution and the expected outcomes.
- Lessons Learned: The case study concludes by discussing the lessons learned from the financial situation or decision-making process. This may involve reflections on successful strategies, potential pitfalls, and broader implications for financial management and decision-making in similar contexts.
How do you write a financial case study?
Writing a financial case study involves analyzing a real or hypothetical financial situation or problem and presenting a detailed examination of the facts, analysis, and potential solutions. Here is a step-by-step guide on how to write a financial case study:
- Identify the purpose and scope: Clearly define the purpose of the case study and the specific financial issue you want to address. Determine the scope of the study, including the period, entities involved, and relevant financial data.
- Gather information: Collect all relevant financial data and supporting documents related to the case. This may include financial statements, transaction records, market data, industry reports, and any other information necessary for the analysis.
- Describe the background: Provide an overview of the company or individual involved in the case study. Include relevant details such as the company's history, industry , size, key stakeholders, and any recent events or developments that may have a financial impact.
- State the problem or objective: Clearly define the financial problem or objective that needs to be addressed. Identify the key challenges or issues the company or individual faces and explain why they are essential.
- Conduct financial analysis: Analyze the financial data and apply appropriate financial analysis techniques to evaluate the situation. This may involve calculating financial ratios, conducting trend analysis, performing a discounted cash flow analysis, or any other relevant method to gain insights into the financial performance and position of the entity.
- Present findings: Summarize the results of the financial analysis clearly and concisely. Highlight key findings, trends, and any significant financial situation factors. Use graphs, charts, or tables to present data effectively.
- Discuss alternative solutions: Propose different options or strategies to address the financial problem or achieve the objective. Determine the advantages and drawbacks of each solution and provide supporting evidence or calculations to justify your recommendations.
- Make recommendations: Make clear and actionable recommendations based on analyzing and evaluating the alternative solutions. Support your recommendations with logical reasoning and explain how they can improve the financial situation or achieve the desired outcome.
- Provide a conclusion: Summarize the main points of the case study and restate the recommendations. Highlight any potential risks or challenges associated with implementing the proposed solutions.
- Include references and citations: If you have used external sources or references, provide proper citations to give credit to the authors and avoid duplicity or redundancy.
- Edit and proofread: Review the case study for clarity, coherence, and accuracy. Check for any grammatical or spelling errors. Ensure that the document is well-structured and easy to understand.
What is finance study?
Finance study refers to the field of knowledge and an academic discipline that focuses on managing, creating, and allocating financial resources. It involves studying various aspects of financial systems, instruments, markets, and institutions. Finance encompasses the theory and practice of managing money, investments, and financial decision-making.
The study of finance covers a wide range of topics, including:
- Corporate Finance: This area focuses on financial decisions and strategies within corporations. It includes capital budgeting, investment analysis, financial planning, risk management, and corporate valuation.
- Investments: This field examines allocating money to different financial assets including, stocks, mutual funds, real estate, and other derivatives. It involves analyzing risk and return, portfolio management, asset pricing models, and investment strategies.
- Financial Institutions and Markets: This area explores the functioning of financial institutions (such as banks, insurance companies, and investment firms) and financial markets (such as stock markets, bond markets, and foreign exchange markets). It involves studying the role of these institutions and markets in facilitating the flow of funds, managing risks, and pricing financial assets.
- International Finance: This branch focuses on financial transactions and relationships between countries and across borders. It covers foreign exchange rates, international investment, multinational corporations, and global financial markets.
- Personal Finance: This area focuses on individual or household financial management. It involves budgeting, saving, investing, retirement planning, taxation, and managing personal debt.
Related posts:
- How Financial Management Templates Can Make a Money Master Out of You
- Top 5 Capital Budgeting Templates with Samples and Examples
- The Ultimate Spokes Diagram Templates to Enhance Business Presentation
- Top 5 One-page Quarterly Report Templates with Examples and Samples
Liked this blog? Please recommend us

Top 5 Daily Appointment Templates with Samples and Examples
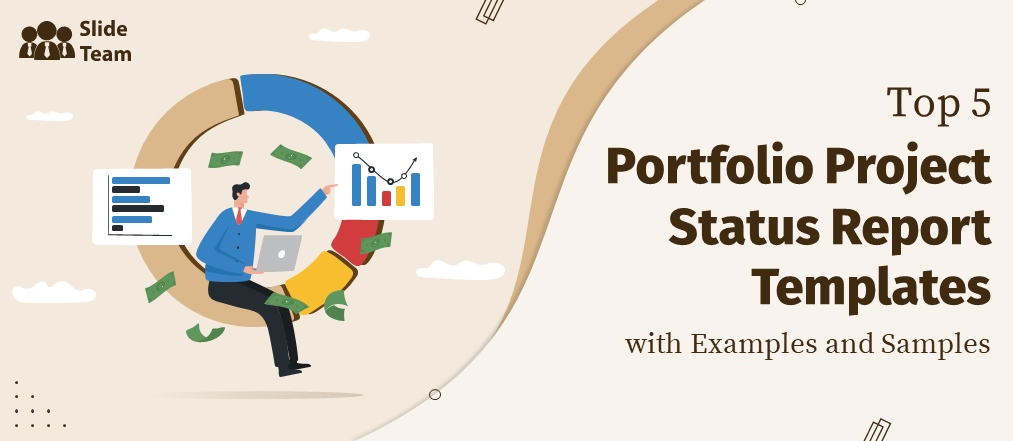
Top 5 Portfolio Project Status Report Templates with Examples and Samples
2 thoughts on “must-have financial case study examples with samples and templates”.
This form is protected by reCAPTCHA - the Google Privacy Policy and Terms of Service apply.
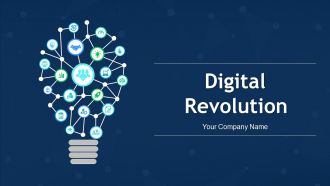
--> Digital revolution powerpoint presentation slides
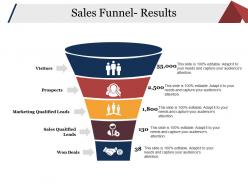

--> Sales funnel results presentation layouts
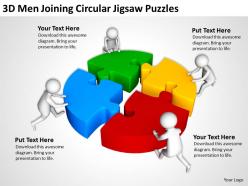
--> 3d men joinning circular jigsaw puzzles ppt graphics icons
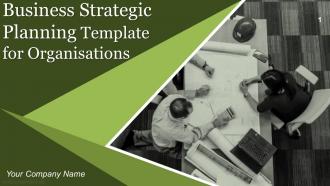
--> Business Strategic Planning Template For Organizations Powerpoint Presentation Slides
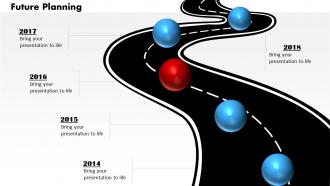
--> Future plan powerpoint template slide
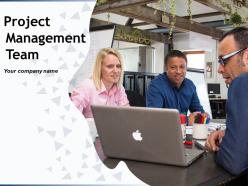
--> Project Management Team Powerpoint Presentation Slides
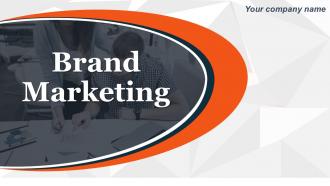
--> Brand marketing powerpoint presentation slides
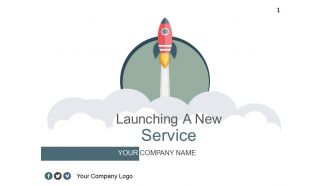
--> Launching a new service powerpoint presentation with slides go to market
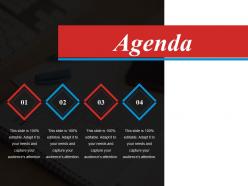
--> Agenda powerpoint slide show
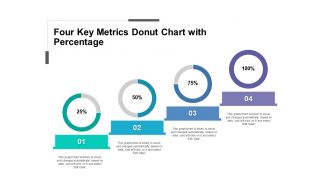
--> Four key metrics donut chart with percentage
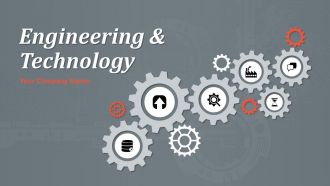
--> Engineering and technology ppt inspiration example introduction continuous process improvement
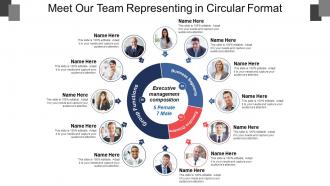
--> Meet our team representing in circular format

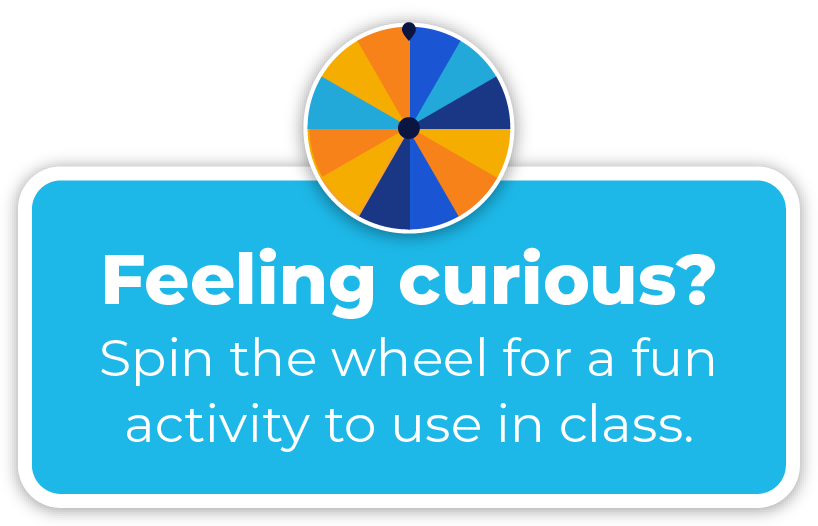
Budgeting Case Studies
New to ngpf.
Save time, increase student engagement, and help your students build life-changing financial skills with NGPF's free curriculum and PD.
Start with a FREE Teacher Account to unlock NGPF's teachers-only materials!
Become an ngpf pro in 4 easy steps:.
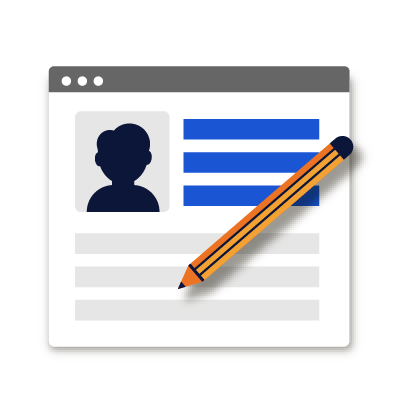
1. Sign up for your Teacher Account
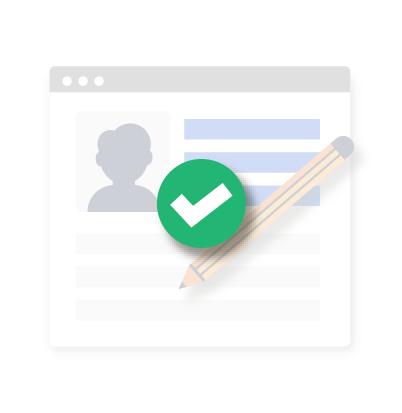
2. Explore a unit page
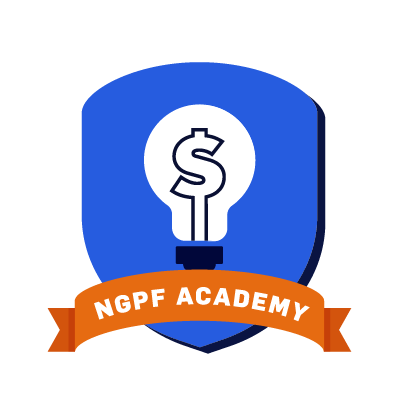
3. Join NGPF Academy
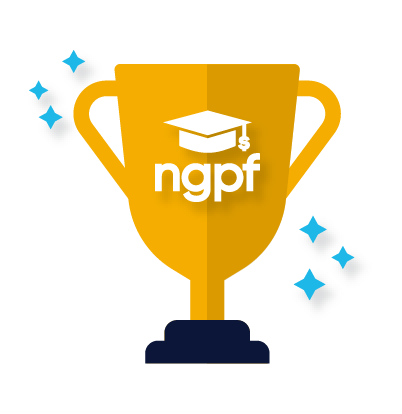
4. Become an NGPF Pro!
Want to see some of our best stuff?
Spin the wheel and discover an engaging activity for your class, your result:.
PROJECT: Who Aced the Interview Challenge?
Sending form...
One more thing.
Before your subscription to our newsletter is active, you need to confirm your email address by clicking the link in the email we just sent you. It may take a couple minutes to arrive, and we suggest checking your spam folders just in case!
Great! Success message here
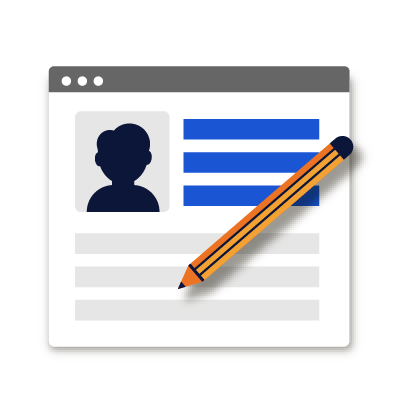
Teacher Account Log In
Not a member? Sign Up
Forgot Password?
Thank you for registering for an NGPF Teacher Account!
Your new account will provide you with access to NGPF Assessments and Answer Keys. It may take up to 1 business day for your Teacher Account to be activated; we will notify you once the process is complete.
Thanks for joining our community!
The NGPF Team
Want a daily question of the day?
Subscribe to our blog and have one delivered to your inbox each morning, create a free teacher account.
Complete the form below to access exclusive resources for teachers. Our team will review your account and send you a follow up email within 24 hours.
Your Information
School lookup, add your school information.
To speed up your verification process, please submit proof of status to gain access to answer keys & assessments.
Acceptable information includes:
- a picture of you (think selfie!) holding your teacher/employee badge
- screenshots of your online learning portal or grade book
- screenshots to a staff directory page that lists your e-mail address
- any other means that can prove you are not a student attempting to gain access to the answer keys and assessments.
Acceptable file types: .png, .jpg, .pdf.
Create a Username & Password
Once you submit this form, our team will review your account and send you a follow up email within 24 hours. We may need additional information to verify your teacher status before you have full access to NGPF.
Already a member? Log In
Welcome to NGPF!
Take the quiz to quickly find the best resources for you!
ANSWER KEY ACCESS
Thank you for visiting nature.com. You are using a browser version with limited support for CSS. To obtain the best experience, we recommend you use a more up to date browser (or turn off compatibility mode in Internet Explorer). In the meantime, to ensure continued support, we are displaying the site without styles and JavaScript.
- View all journals
- Explore content
- About the journal
- Publish with us
- Sign up for alerts
- Open access
- Published: 15 August 2024
Financial network communities and methodological insights: a case study for Borsa Istanbul Sustainability Index
- Larissa M. Batrancea 1 ,
- Ömer Akgüller 2 ,
- Mehmet Ali Balcı ORCID: orcid.org/0000-0003-1465-7153 2 &
- Anca Nichita 3
Humanities and Social Sciences Communications volume 11 , Article number: 1046 ( 2024 ) Cite this article
Metrics details
- Complex networks
- Operational research
This study investigates the influence of Environmental, Social, and Governance (ESG) scores on the clustering and community formation of companies within various network models. Using daily closing prices of 78 companies operating in the Borsa Istanbul Sustainability Index, we constructed correlation, mutual information (both continuous and discrete), and causality (both linear and nonlinear) networks to analyse intercompany relationships. We performed community detection using the Leading Eigenvector and Girvan–Newman methods, which revealed that companies within the same sector, particularly in the financial and manufacturing sectors, tend to form tight-knit communities. These intra-sectoral clusters reflect strong market behaviour correlations driven by sector-specific factors. Additionally, mixed-sector communities highlighted the presence of significant inter-sector dependencies. To assess the impact of ESG scores on these communities, nonparametric tests such as the Kruskal–Wallis, Conover, and log-rank were applied. Results showed that specific ESG factors, including emission, CSR strategy, innovation, and human rights, significantly influenced community formation. For instance, companies with strong performance in emission reduction and CSR strategies were found to form more cohesive communities, emphasizing the role of sustainability in shaping financial networks. Study findings underscore the critical role of ESG factors in financial market dynamics, promoting sustainable investment practices by highlighting the importance of integrating ESG considerations into investment decisions. These results suggest that sustainability metrics not only affect individual company performance but also contribute to the formation of interconnected communities with shared sustainability practices.
Similar content being viewed by others
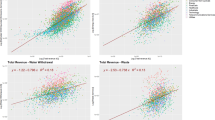
Assessments of the environmental performance of global companies need to account for company size
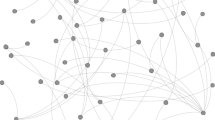
A multilevel analysis of financial institutions’ systemic exposure from local and system-wide information
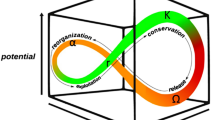
Measuring system resilience through a comparison of information- and flow-based network analyses
Introduction.
Understanding market dynamics requires a deep knowledge of financial networks, which offer a comprehensive and detailed view of the relationships and interactions between various market entities such as stocks, industries, and organizations. We construct these networks by discovering and evaluating the connections between different financial instruments and entities using measures such as price correlations, trade volumes, and other relevant financial data. By undertaking this action, they reveal the market structure and provide significant insight into the interplay between different factors (Chi et al., 2010 , Guo et al., 2018 , Karpman et al., 2023 , Shirokikh et al., 2022 ).
Through the analysis of financial networks, researchers and analysts can identify patterns of connectivity that are not immediately apparent from raw data alone. For example, they can detect clusters of highly interconnected stocks that tend to move together, known as communities or modules, which often correspond to specific sectors or industries. These clusters can highlight sectoral interdependencies and information flow within and between different parts of the market (Raddant and Kenett, 2021 , Pazitka et al., 2021 , Upadhyay et al., 2020 ). Additionally, financial networks can reveal key nodes or hubs, which are entities with a high degree of connectivity that play a significant role in the overall stability and functionality of the market. Identifying these influential nodes is crucial for understanding systemic risk and potential points of vulnerability within the financial system.
The analysis of financial networks also enables the study of information flow and influence among market participants. By examining how information or shocks propagate through the network, analysts can gain insights into the mechanisms of market reactions and the spread of financial contagion. This understanding is critical for predicting market behaviours, as it allows for the anticipation of how different parts of the market may respond to various stimuli, such as economic news, policy changes, and geopolitical events.
In recent years, Environmental, Social, and Governance (ESG) factors have emerged as vital considerations in financial decision-making. ESG factors encompass a wide range of criteria that assess a company’s performance beyond traditional financial metrics. Environmental factors include a company’s impact on the environment, such as carbon emissions and resource usage. Social factors evaluate how a company manages relationships with employees, suppliers, customers, and the communities in which it operates. Governance factors examine a company’s leadership, executive pay, audits, internal controls, and shareholder rights.
The growing prominence of ESG factors in financial analysis reflects a broader shift towards sustainable and responsible investing. Investors are increasingly aware that companies with strong ESG performance are better positioned to manage risks, capitalize on opportunities, and achieve long-term financial success. Studies have shown that integrating ESG metrics into financial analysis can lead to more resilient investment portfolios (Ma 2023 , Naffa and Fain 2020 ). Companies with high ESG ratings tend to have lower volatility, higher profitability, and better long-term returns compared to their peers with poor ESG performance. Moreover, the integration of ESG metrics is recognized for promoting sustainable investment practices. By considering ESG factors, investors can identify companies that are committed to sustainability and ethical practices, which can lead to positive social and environmental outcomes. This approach not only aligns with the growing demand for corporate responsibility but also enhances the resilience of financial markets. Sustainable investment practices can help mitigate risks associated with environmental degradation, social unrest, and poor governance, thereby contributing to the stability and health of the financial system.
Despite the extensive research on financial networks, there remains a significant gap in understanding how ESG factors influence the formation and dynamics of networks. Traditional financial network studies primarily focus on metrics such as price correlations, trading volumes, and return dependencies to analyse market structures and predict behaviours. While these studies provide valuable insights into the interconnectedness and risk propagation within financial markets, they often overlook the integration of ESG metrics, which can offer a more comprehensive understanding of market dynamics.
Existing studies frequently miss the critical role that ESG factors play in shaping the relationships between market entities. ESG metrics encompass a range of non-financial factors that reflect a company’s long-term sustainability and ethical practices, such as carbon footprint, labour practices, board diversity, and transparency. By excluding these factors, current research may fail to capture the full spectrum of influences that drive market behaviours and interdependencies. For instance, companies with strong environmental practices might be more interconnected due to shared sustainability goals, leading to distinct community structures that are not apparent when only financial data are considered.
The integration of ESG metrics into financial network analysis can provide insights into how sustainability practices affect market interconnectivity and community structures. Companies that prioritize ESG criteria may exhibit different connectivity patterns, forming clusters based on shared environmental initiatives, social responsibilities, or governance standards. This can reveal hidden dependencies and relationships that traditional financial metrics might miss, such as the tendency for companies with robust ESG practices to be more resilient during economic downturns or crises due to better risk management and ethical governance. Furthermore, understanding the impact of ESG factors on financial networks can enhance the predictive power of these models. Incorporating ESG metrics can help identify systemic risks and opportunities that are not evident through financial data alone. For example, a network analysis that includes ESG factors might reveal that companies with poor environmental practices are more susceptible to regulatory changes or public backlash, leading to greater market volatility and risk of contagion. Additionally, the integration of ESG metrics into financial network analysis can inform more sustainable investment strategies. Investors increasingly seek to align their portfolios with ethical and sustainable practices, and understanding the role of ESG factors in financial networks can guide them in identifying companies and sectors that are not only financially robust but also committed to long-term sustainability. This can lead to more informed investment decisions that support both financial returns and positive societal impacts.
This study aims to bridge the gap in existing research by investigating the impact of ESG scores on clustering and community formation within various financial network models. The primary objective is to integrate sustainability metrics into the analysis of financial networks to provide a more comprehensive understanding of market dynamics. By incorporating ESG factors, the study seeks to uncover how environmental, social, and governance considerations influence intercompany relationships and the formation of distinct communities within these networks.
To achieve this, the study employs several sophisticated network models, each offering a unique perspective on intercompany relationships. First, correlation network models will be utilized to identify how company stock prices move in relation to one another. In these models, edges represent the degree of correlation between the daily closing prices of different companies, with higher correlations indicating stronger connectivity. This approach will help detect sectoral clusters where companies within the same sector, such as finance or manufacturing, exhibit similar stock price movements due to shared economic factors.
Subsequently, the study will utilize mutual information network models to collect and analyse both linear and nonlinear connections among stock values of different companies. By employing both continuous and discrete mutual information, these networks will surpass basic linear correlations to uncover intricate interdependencies and information exchanges among organizations. This approach seeks to reveal insights into the impact of information flow and common market behaviours on community structures, potentially showing important inter-sectoral linkages. Furthermore, causality network models will be utilized to comprehend the directional effects among stock values of different organizations. These models will illustrate how fluctuations in the stock price of one firm can forecast those of another firm, thus capturing the cause-and-effect interactions within the market. We will conduct both linear and nonlinear causality analyses to obtain a full understanding of these interdependencies.
To assess the influence of ESG metrics on community structures, the study will employ nonparametric tests, including the Kruskal–Wallis, Conover, and log-rank tests. These tests are particularly suitable for this analysis as they do not assume a specific distribution for the data, making them robust tools for evaluating relationships between ESG scores and community formation.
Through this multifaceted approach, the study aims to provide valuable insights into the role of ESG factors in shaping financial networks. By understanding how sustainability metrics influence market connectivity and community structures, the findings of this study can inform more sustainable investment practices and contribute to the development of resilient and responsible financial markets.
Study findings reveal distinct patterns of interconnectivity among companies across different network models. Correlation networks highlight strong sectoral linkages, particularly within the finance and manufacturing sectors. Mutual information networks provide insights into both intra- and inter-sector dependencies, while causality networks demonstrate predictive relationships in stock price movements. The nonparametric test results indicate that ESG factors such as Emission, CSR Strategy, Innovation, and Human Rights significantly influence community formation, suggesting that companies with strong performance in these areas tend to form more cohesive communities.
The remainder of this paper unfolds as follows: section “Literature review” embarks on a comprehensive literature review, laying the groundwork for our study. Section “Methodology” delves into the methodology, detailing the construction of network models and the application of community detection methods. In the section “Results”, we introduce the dataset and provide an in-depth analysis of the influence of ESG sub-scores on overall ESG performance. This section then transitions into a detailed presentation and discussion of network and community results. Section “Discussions” explores the nonparametric tests employed to evaluate the impact of ESG scores, offering a detailed analysis of findings. Finally, the section “Conclusions” ends the study with a summary of key insights and thoughtful suggestions for future research directions.
By integrating ESG factors into financial network analysis, this study not only enhances understanding of market dynamics but also promotes sustainable investment practices. The insights gained from this research can guide investors and policymakers in fostering more resilient and socially responsible financial markets.
Literature review
Understanding the role of Environmental, Social, and Governance (ESG) factors in financial networks is essential for grasping the complexities of market dynamics and sustainable investment practices. This literature review synthesizes insights from recent studies on the integration and impact of ESG metrics within financial networks.
Kim and Li ( 2021 ) explored the relationship between ESG factors and corporate financial performance, showing that higher ESG scores were linked to increased profitability and better credit ratings, particularly in large firms and those with strong governance practices. Similarly, Sinha et al. ( 2019 ) conducted a meta-analysis of 100 studies, confirming that ESG integration generally led to improved financial performance across different geographic domains. Aybars et al. ( 2019 ) demonstrated a significant positive relationship between ESG scores and return on assets for S&P 500 firms, highlighting the importance of ESG factors in enhancing operational performance. Additionally, Caporale et al. ( 2022 ) analysed the impact of ESG factors on financial performance and found that ESG scores significantly enhanced financial stability and resilience against market shocks. Luo et al. ( 2024 ) examined the integration of ESG metrics into portfolio management and found that ESG-oriented portfolios outperformed traditional ones, particularly in volatile markets.
Batrancea et al. ( 2022c ) highlighted the importance of governance quality and social policies in fostering economic growth, with a limited focus on environmental sustainability. Financial performance was measured through GDP growth, investment rates, and industrial production, showing the positive impact of good governance and social policies. Batrancea et al. ( 2022a ) integrated ESG factors by examining environmental policies, social welfare, and governance quality, showing their positive influence on economic growth. Financial performance indicators such as GDP growth, productivity, and investment demonstrated that robust ESG frameworks supported sustainable economic growth. Similarly, the study by Batrancea et al. ( 2022b ) examined the role of SME financing, indirectly addressing environmental and social impacts, with a strong focus on governance and regulatory environments. It concluded that well-financed SMEs significantly contributed to economic growth and stability, as measured by GDP and productivity metrics. The analysis from Batrancea et al. ( 2021 ) emphasized the importance of governance quality and social infrastructure in driving economic growth, with some consideration of environmental sustainability. Financial performance was evaluated through GDP growth and investment flows, showing that better governance and social infrastructure were crucial for sustainable growth. Additionally, Batrancea et al. ( 2023b ) discussed the impact of environmental regulations, social factors, and governance quality on economic growth over the past fifty years. It found that countries with strong ESG frameworks experienced more robust economic growth, measured by GDP, investment, productivity. Batrancea ( 2022 ) focused on the role of SMEs in economic growth, considering environmental sustainability, social equity, and supportive governance. Financial performance was assessed through SME growth rates and GDP, concluding that supportive policies were essential for SME-driven economic sustainability. Finally, Batrancea et al. ( 2023a ) highlighted the positive impact of investments in wellbeing-related infrastructure, such as healthcare and education, on regional economic growth. Financial performance was measured through regional GDP growth and productivity, showing that better social infrastructure determined higher economic growth.
ESG integration in investment strategies involves incorporating environmental, social, and governance factors into investment decisions to enhance long-term financial returns and sustainability. Chan et al. ( 2020 ) showed that incorporating ESG signals into factor-based investing strategies could yield similar historical performance to traditional factor portfolios, suggesting that ESG metrics might enhance financial value and quality factors. Zaccone and Pedrini ( 2020 ) found that private equity firms were increasingly integrating ESG factors into their investment strategies, primarily for risk management and value creation, though challenges in measuring ESG issues persisted. Ma ( 2023 ) discussed international trends in ESG integration, emphasizing the link between ESG ratings and company performance, and suggesting that ESG considerations provided a more comprehensive dimension for investment decision-making. Park and Lee ( 2023 ) highlighted the growing importance of ESG factors in investment strategies, showing that ESG integration generated improved risk-adjusted returns. Park and Oh ( 2022 ) explored how ESG factors influenced investment decisions and found that ESG considerations significantly enhanced portfolio resilience.
ESG factors play a critical role in promoting financial stability by mitigating environmental, social, and governance risks that can impact economic systems. Incorporating ESG considerations into financial decision-making can enhance the resilience and sustainability of financial markets, leading to more stable and predictable economic outcomes. Stolbov and Shchepeleva ( 2022 ) reviewed empirical studies on the impact of ESG factors on financial stability, finding that higher ESG rankings enhanced stability by mitigating individual and systemic risks. Lupu et al. ( 2022 ) examined the impact of ESG scores on European financial stability, using advanced methods to uncover dependencies not evident in average distribution levels. Ling et al. ( 2023 ) found that higher ESG scores determined increased financial stability and reduced systemic risk, particularly in the banking sector.
Integrating ESG factors into business practices can significantly enhance a company’s market value by attracting socially responsible investors and improving long-term financial performance. Companies with strong ESG performance often enjoy higher valuations and better access to capital, as they are perceived to be more sustainable and less risky. Ionescu et al. ( 2019 ) investigated the relationship between ESG factors and market value in the travel and tourism industry, finding that good governance scores significantly influenced market value regardless of geographic region. Lee and Isa ( 2023 ) explored the impact of ESG factors on financial performance in Malaysia, showing that environmental factors positively influenced ROE, while social and governance factors impacted long-term market performance. Yoon et al. ( 2024 ) found that companies with high ESG ratings had higher market values and better financial performance. Furthermore, Junius et al. ( 2020 ) reported no significant direct impact of ESG scores on firm performance and market value in ASEAN countries, suggesting that ESG factors were not fully integrated into performance metrics in these regions.
In recent years, the intersection of financial performance and sustainability practices has garnered significant attention, particularly within the context of emerging markets such as Turkey. Current studies have explored various dimensions of this relationship, providing a nuanced understanding of how ESG factors impact financial markets and corporate behaviour in Borsa, Istanbul. For instance, the relationship between monetary policy and financial asset returns in Turkey has been investigated using time, frequency, and quantile-based effects, highlighting the intricate dynamics between macroeconomic policies and market performance by Kartal et al. ( 2024b ). Additionally, research by Kartal et al. ( 2024a ) modelled the link between environmental, social, and governance disclosures and scores, focusing on publicly traded companies in the Borsa Istanbul Sustainability Index. This study underscores the importance of transparent ESG disclosures in shaping investor perceptions and corporate valuations. Furthermore, the influence of climate risk, carbon allowances, and technological factors on the ESG market has been assessed within the European Union context, providing comparative insights that are relevant for understanding similar dynamics in Turkey by Pata et al. ( 2024 ). These studies collectively emphasize the critical role of ESG factors in financial analysis and emphasize the need for integrating sustainability metrics to foster more responsible financial markets.
ESG metrics provide valuable data for predictive analysis, allowing investors and analysts to forecast financial performance and risks more accurately. By integrating ESG metrics into predictive models, businesses can identify potential opportunities and threats, leading to more informed and strategic decision-making. Ang et al. ( 2023 ) utilized dynamic intercompany relationships to predict ESG ratings, demonstrating a significant improvement in prediction performance when incorporating network information. Giese et al. ( 2019 ) linked ESG information to company valuation and performance through cash flow and risk channels, suggesting that ESG characteristics could be useful financial indicators. Lee et al. ( 2024 ) analysed how ESG factors predicted stock market movements. Gong et al. ( 2024 ) reported that ESG factors significantly enhanced the predictive power of financial models for investment decisions.
Methodological approaches in ESG research integrate quantitative and qualitative metrics, with future directions focused on developing sophisticated predictive models. Meiden and Silaban ( 2023 ) emphasized the need for comprehensive and standardized metrics to effectively capture and compare environmental performance within the broader ESG framework, recognizing the interdependencies between these dimensions. Similarly, Escrig-Olmedo et al. ( 2017 ) proposed a fuzzy multicriteria decision-making approach to integrate the preferences of diverse ESG investors, highlighting the importance of sector-specific metrics. Empirical research by Efimova et al. ( 2021 ) demonstrated a positive relationship between ESG commitment and market returns, suggesting that ESG factors could be significant drivers of financial performance. Furthermore, Raza et al. ( 2022 ) developed machine learning algorithms to predict ESG scores based on financial data, advocating for the use of artificial intelligence to enhance the accuracy and relevance of ESG evaluations. Zhang and Zhang ( 2023 ) also reviewed the application of machine learning in ESG research, noting its potential to address challenges in ESG disclosure and improve the reliability of ESG ratings. These studies collectively emphasize the growing trend toward integrating sophisticated predictive models and machine learning techniques in ESG research to enhance the precision and applicability of ESG assessments.
The integration of ESG factors into financial network analysis provides valuable insights into market dynamics and promotes sustainable investment practices. By incorporating ESG metrics, researchers can enhance the predictive power of financial models, identify systemic risks, and support the development of resilient financial systems.
Methodology
Utilizing mathematical principles, particularly those derived from graph theory, enhances our understanding of complex interrelated systems. In these networks, nodes represent discrete items (such as stocks or bonds), while edges indicate the connections or affiliations between them. Mathematically, a graph is a tuple \(G=(V,E)\) , where V represents the set of nodes (or vertices) and \(E\subseteq V\times V\) represents the set of edges. Weight functions \(\omega :E\to {{\mathbb{R}}}^{+}\) are often used to assign weights to edges in real-world scenarios, such as the financial network of the Borsa Istanbul Sustainability Index. This generates the formation of a weighted graph, represented as \(G=(V,E,\omega )\) .
This study examines five distinct approaches to network creation, each establishing the relationship between nodes that represent companies through different methods for generating edges. Weighted financial networks are constructed by assigning weights to the edges based on the selected approach. The primary objective of the study is to examine the clusters in financial networks derived from various methodologies, specifically focusing on graph communities.
Correlation networks
Within the complex realm of global economics, the interconnection of financial networks plays a crucial role in shaping market directions and impacting economic stability. Financial networks, composed of intricate systems of institutions, markets, and tools, are essential in facilitating the movement and distribution of capital globally. The interconnectedness of these networks fosters a dynamic ecosystem where economic activity, investments, and regulations are tightly connected. Understanding the interconnections within financial networks is vital for effectively navigating the complexities of contemporary finance, as it impacts not only individual institutions but also has extensive ramifications for entire economies and societies.
In financial correlation networks, each node represents a financial asset, and the edge weights measure the correlation between the returns of connected assets. To analyse the logarithmic return of the daily closing price of stocks, we use the following formula:
This equation calculates the difference in the daily closing price of stock i at time t .
To compute the Pearson correlation coefficient between stocks i and j , we use the following formula:
where \(\langle \cdot \rangle\) represents the temporal average. The Pearson correlation coefficient is a commonly used statistical measure in finance that quantifies the extent of the linear relationship between two financial time series. A coefficient close to 1 indicates a strong positive correlation, suggesting that the two time series consistently move together. Conversely, a coefficient close to −1 indicates a significant negative correlation, where the variables move in opposite directions. A correlation value close to zero indicates a minor or insignificant correlation, meaning there is no noticeable linear relationship between time series.
The weight function derived from the correlation coefficient is given by
By starting with a comprehensive initial network setup, where each node is intricately connected and assigned weights based on correlation distance, we uncover detailed patterns of relationship dynamics. Notably, as correlations strengthen, either positively or negatively, the corresponding edge weights tend to decrease towards zero, indicating stronger linkages. Conversely, weaker correlations result in weights approaching \(\sqrt{2}\) . In this framework, weights serve as quantifiable measures of relationship intensity, with smaller values indicating stronger links.
Since this approach creates a connection, albeit weak, between every node, the resulting network is mathematically equivalent to a complete graph. To mitigate the impact of insignificant connections on the analysis, filtering techniques are employed to enhance the realism of models by removing weak edges. This study utilizes the triangulated maximally filtered graph (TMFG) filtering approach, known for its effectiveness in financial networks (Akgüller, 2019 ; Balcı et al., 2022 ; Millington, 2022 ; Turiel et al., 2022 ).
TMFGs provide a robust analytical framework for financial networks, methodically extracting important information from complex interconnected systems. In financial networks, TMFGs often begin with a comprehensive network structure where nodes represent different financial firms and edges indicate connections between them. Edges are weighted according to correlation distances, reflecting the intensity of relationships. The triangulation process involves identifying cycles and closing triangles within the network, thereby improving the representation of interdependencies among financial institutions. The TMFG method imposes a structural constraint that limits the inclusion of zero-order correlations to a maximum of 3| V |−6, where | V | represents the number of nodes. The TMFG process starts by selecting four nodes with the highest cumulative correlations to all other nodes. It then systematically adds each node with the highest total of three correlations to the existing network until all nodes are included. For more details, readers are referred to Massara et al. ( 2016 ).
The topologically triangulated structure used throughout this paper is denoted as \({G}_{F}=(V,{E}_{F},\omega )\) , where \({E}_{F}\subset E\) represents the filtered edge set. Implementing this triangulated framework allows for a more detailed understanding of systemic risk and interconnections. By using weighted edges derived from correlation distances, filtering techniques are applied to enhance the network, emphasizing important interactions and reducing the impact of noise.
Mutual information networks
Within the domain of financial systems, the concept of mutual information has become a vital framework for understanding the complex connections and interrelationships within networks. Mutual information in financial networks quantifies the degree to which the actions or performances of one entity provide insights into those of another, revealing the shared information and interconnections among various financial elements. Studying mutual information is crucial for understanding the dynamics that drive market movements, risk transmission, and systemic stability in today’s interconnected and complex financial environments. This quantitative metric provides valuable insights into the flow and sharing of information among different entities in the financial ecosystem, helping one to grasp the relationships that shape the modern financial landscape.
This study constructs a weighted network by using mutual information associations among time series derived from logarithmic returns of closing prices given in Eq. ( 1 ). The use of continuous and discrete bivariate mutual information methodologies allows for the capturing of distinct dynamics.
Calculating continuous mutual information is essential for uncovering the connections between logarithmic returns of closing prices in financial networks. This process involves accurately calculating the continuous mutual information between pairs of organizations, offering a quantitative assessment of how interconnected their return behaviours are. To initiate this calculation, logarithmic returns are treated as continuous stochastic variables, and their joint probability density function is computed using kernel density estimation techniques. The integral of the joint probability density function
quantifies the information that is mutually communicated between the logarithmic returns of two organizations, considering both linear and nonlinear connections. In Eq. ( 4 ), \(p(C{l}_{i},C{l}_{j})\) is the joint probability density function of the logarithmic returns, and \(p(C{l}_{i})\) and \(p(C{l}_{j})\) are the marginal probability density functions. This computation determines how much the returns of one company convey information about the returns of another by comparing the joint probability distribution to marginal distributions. The continuous mutual information values obtained provide a detailed understanding of the intensity and nature of the connections, informing further network analysis and offering valuable insights into the complex dynamics of financial systems. This method is particularly useful for capturing intricate interdependencies that may not be fully represented by linear correlation measures, providing a more comprehensive view of the underlying linkages within financial data (Khoojine et al., 2023 , Nicola et al., 2020 , Zhao et al., 2023 ).
Discrete bivariate mutual information is a crucial step in exploring financial networks, using a discrete representation of logarithmic returns to quantify the informational content between pairs of companies. Initially, the continuous logarithmic returns are discretized into a finite set of bins, allowing for the application of discrete mutual information theory. Using the formula
the computation involves summing over all possible combinations of discretized values, considering their joint and marginal probability mass functions. This method effectively captures the statistical dependencies between discrete logarithmic returns, accounting for both linear and nonlinear associations. The resulting bivariate mutual information values represent the amount of shared information between pairs of companies, revealing the strength and nature of their connections in the context of financial movements. This approach is instrumental in overcoming challenges associated with continuous data, allowing for a more manageable and interpretable analysis (Viegas et al., 2020 , Yadav et al., 2020 ).
The weight assignment schemes used in this study are \(\frac{1}{{I}_{{{c}}}\left(C{l}_{i}{;C}{l}_{j}\right)}\) and \(\frac{1}{{I}_{{{d}}}\left(C{l}_{i}{;C}{l}_{j}\right)}\) for the respective networks. Incorporating the inverses of continuous mutual information and discrete bivariate mutual information as edge weights represents an intriguing approach to constructing financial networks. When considering continuous mutual information, applying edge weights as the reciprocal of estimated mutual information values results in a proportional decrease in the strengths of linkages. Higher mutual information values, indicating stronger connections between financial institutions based on logarithmic returns, lead to lower reciprocal edge weights. This method amplifies weaker connections by assigning them higher weights, resulting in a network representation that emphasizes links that are less connected or less dependent. In the context of discrete bivariate mutual information, the dynamic weighting technique assigns higher weights to edges with lower mutual information values, indicating a focus on relationships with lower information content. This reciprocal weighting approach offers a distinct perspective by prioritizing edges representing less statistically significant relationships within discretized financial data. The TMFG approach is used as a filtering technique to acquire the sets of E F for both networks generated using continuous and discrete bivariate mutual information. It is important to note that in both scenarios, the reciprocals of information values are used to determine the appropriate edge weights for TMFG filtering.
Causality networks
The study of financial networks has progressed beyond traditional linear methods to include causality analysis, revealing complex connections among time series data. Researchers explore the dynamic relationships between the logarithmic returns of closing prices among different companies by analysing both linear and nonlinear causalities (Stavroglou et al., 2017 , 2020 ; Zuhud et al., 2022 ). Statistical approaches, such as the Granger causality test, help identify predictive connections between the historical returns of one company and another, indicating linear causality. Simultaneously, advanced approaches in nonlinear causality analysis provide a more detailed understanding of the complex relationships that may occur, surpassing the limitations of linear models. The resulting financial networks encompass not only direct effects but also intricate, nonlinear interdependencies, offering a comprehensive view of how the financial performances of organizations influence one another. This extensive investigation into linear and nonlinear causality in financial time series data forms a basis for better understanding the underlying dynamics.
Consider two time series \(C{l}_{i}\left(t\right)\) and \(C{l}_{j}\left(t\right)\) , representing the logarithmic returns of daily closing prices for two distinct companies in the stock market. The linear causality between these time series can be formally assessed through the Granger causality test. Let \(C{l}_{i}\left(t\right)\) and \(C{l}_{j}\left(t\right)\) be jointly modelled in a lagged vector autoregressive framework as follows:
Here, p represents the lag order, \({\alpha }_{1s}\) and \({\alpha }_{2s}\) are coefficients, and \({\epsilon }_{i}\) and \({\epsilon }_{j}\) are white noise error terms. The Granger causality test assesses whether past values of \(C{l}_{i}\left(t\right)\) provide significant information in predicting \(C{l}_{j}\left(t\right)\) beyond the information contained in the past values of \(C{l}_{j}\left(t\right)\) itself. Formally, the null hypothesis \({H}_{0}\) is that \(C{l}_{i}\left(t\right)\) does not Granger-cause \(C{l}_{j}\left(t\right)\) . The test involves comparing the F-statistic from the restricted model (excluding lagged values of \(C{l}_{i}\left(t\right)\) ) with the F-statistic from the unrestricted model (including lagged values of \(C{l}_{i}\left(t\right)\) ). Statistical significance in rejecting \({H}_{0}\) indicates the presence of linear causality from \(C{l}_{i}\left(t\right)\) to \(C{l}_{j}\left(t\right)\) , thereby providing insights into the directional influence of one company’s logarithmic returns on another in the stock market.
Advanced techniques, such as Transfer Entropy, are used to investigate the nonlinear causality between time series. Let us denote the entropies of \(C{l}_{i}\left(t\right)\) and \(C{l}_{j}(t)\) as \(H\left(C{l}_{i}\left(t\right)\right)\) and \(H\left(C{l}_{j}\left(t\right)\right)\) , respectively. The conditional entropy of \(C{l}_{i}\left(t\right)\) given \(C{l}_{j}\left(t\right)\) is represented as \(H(C{l}_{i}|C{l}_{j})\) . Transfer Entropy, represented as \({TE}(C{l}_{i}\to C{l}_{j})\) , measures the decrease in uncertainty regarding the future values of \(C{l}_{i}\left(t\right)\) when the previous values of \(C{l}_{j}\left(t\right)\) are considered, surpassing the predictive capability of \(C{l}_{i}\left(t\right)\) based solely on its own previous values. In a formal sense, the expression
represents the Transfer Entropy from \(C{l}_{i}\) to \(C{l}_{j}\) . Nonlinear causality is identified when the value of \({TE}(C{l}_{i}\to C{l}_{j})\) is significantly different from zero. This method identifies the specific direction in which information flows between the logarithmic returns of the two companies, offering valuable insights into nonlinear connections that extend beyond linear links identified by conventional Granger causality analysis. Understanding these nonlinear causal connections enhances the description of dynamic interactions in the stock market.
Assigning weights to edges in networks generated by linear and nonlinear causality analyses using p -values provides a statistical method to determine the importance and reliability of detected causal connections among time series. For linear causality networks, edges can be assigned weights based on the p -values derived from Granger causality tests. Smaller p -values indicate greater statistical significance, so edges associated with more significant causal relationships are assigned lower weights. This methodology ensures that the network represents only statistically robust and reliable causal relationships. Similarly, for networks resulting from nonlinear causality analysis, p -values obtained from tests such as those evaluating the significance of Transfer Entropy can be used to assign weights to edges. Smaller p-values in the context of nonlinear causality provide stronger evidence for the existence of meaningful causal links. By integrating p-values into the weighting of edges, these networks not only visually depict causal structure but also allow researchers to focus on the most statistically plausible connections, enhancing the reliability of insights derived from network analysis.
Incorporating p-values in the context of TMFG filtering is highly relevant and enhances the robustness and reliability of network representation. The TMFG filtration technique aims to identify the most crucial connections within a network by selecting edges based on their robustness and significance. p-values provide an inherent criterion for this objective, offering a statistical assessment of the reliability of identified causal connections. By assigning lower weights to edges associated with lower p-values, the TMFG filtration can be tailored to emphasize connections that are statistically significant. This approach ensures that the final graph captures the most significant information flow and highlights edges with a high level of confidence in their causal influence.
Network Communities
Graph communities, mathematically referred to as subsets of nodes within a graph that exhibit higher connectivity among themselves than with nodes outside the subset, are essential structures in network analysis. Let \(G=\left(V,\,{E}_{F},\,\omega \right)\) represent a weighted filtered graph. A weighted community is then a subset \(C\subseteq V\) where the total edge weight within the community is significantly higher than the expected total edge weight in a null model. Mathematically, for a given partition of nodes into weighted communities \({\mathcal{C}}=\left\{{C}_{1},{C}_{2},\ldots ,{C}_{k}\right\}\) , the modularity \({Q}_{w}\) for weighted graphs is often employed as an optimization metric. The weighted modularity is an extension of the modularity concept for unweighted graphs, considering the sum of edge weights within communities. Formally,
where \(w({C}_{i})\) is the total weight of edges within community \({C}_{i}\) , \({w}_{j}\) is the weight of edge j , and W is the total weight of all edges in the graph. Weighted graph communities offer a more nuanced understanding of network structures by capturing varying strengths of connections between nodes, which is particularly relevant in applications where the intensity or significance of relationships plays a crucial role.
Graph communities are crucial for understanding intricate dynamics in financial networks and provide deep insights into the underlying structure of the market (Ferretti 2023 , Torri & Giacometti 2023 ). The process of identifying and analysing graph communities offers a perspective to comprehend the interrelationships and interdependencies among publicly listed organizations. These communities consist of organizations that have comparable financial features, market behaviours, or sustainability practices. As a result, they exhibit patterns that contribute to the overall resilience of the market.
When evaluating various network formation principles, including correlation, mutual information, and causation in financial networks, graph communities are particularly useful in capturing intricate dynamics. Correlation-based networks can reveal the interdependence between enterprises in a certain economic domain by identifying communities that showcase sectors or industries with strongly associated stock price movements. Conversely, networks built on mutual information expose communities that exhibit substantial information exchange, suggesting clusters of enterprises that react similarly to market occurrences. Introducing causality in network creation adds complexity by revealing how one company’s financial performance causally affects another, providing better understanding of cause-and-effect interactions within the financial ecosystem.
Within the framework of the Borsa Istanbul Sustainability Index, graph communities play a crucial role in identifying groups of enterprises that not only share financial connections but also engage in similar sustainability practices. Companies in a certain community may have comparable environmental, social, and governance (ESG) values, which collectively contribute to the overall sustainability goals of the index. Examining these communities enables stakeholders to identify areas of proficiency, possible weaknesses, and prospects for enhancement in relation to sustainability performance.
Two distinct community detection algorithms are employed in this study, each based on the modularity metric provided in Eq. 9 .
The identification of graph communities based on the leading eigenvector of the modularity matrix involves a more detailed understanding of the spectral clustering process (Newman 2006 ). The modularity matrix B is defined as
where A is the adjacency matrix, \({d}_{i}\) and \({d}_{j}\) are the weighted degrees of nodes i and j , and m is the total edge weight in the graph. Normalizing B by dividing each entry by 2 m yields a symmetric matrix.
The spectral clustering procedure commences by calculating the eigenvectors and eigenvalues of the normalized modularity matrix. The dominant eigenvector, associated with the largest eigenvalue, is obtained. The components of this eigenvector are subsequently employed as coordinates for the nodes, thus mapping them onto a multi-dimensional space. Subsequently, a conventional clustering technique is employed to process these coordinates. Common options include k-means clustering or hierarchical clustering. The partition of nodes that emerges from this process creates separate communities within the graph. Nodes that are assigned to the same cluster demonstrate comparable patterns in their connection strengths. Mathematically, let \({{\boldsymbol{v}}}_{1}\) be the leading eigenvector corresponding to the largest eigenvalue \({\lambda }_{1}\) of the normalized modularity matrix. Form a matrix \({{\boldsymbol{V}}}_{1}\) by stacking the entries of \({{\boldsymbol{v}}}_{1}\) , and apply a clustering algorithm to the rows of \({{\boldsymbol{V}}}_{1}\) This clustering process reveals graph communities based on the spectral properties of the modularity matrix.
The second approach we employ is founded on edge betweenness and is often known as the Girvan–Newman algorithm (Girvan & Newman 2002 ). Mathematically, the weighted betweenness centrality ( \(B(e)\) ) of an edge e in a weighted graph is computed as:
where \({g}_{{st}}(e)\) represents the number of shortest paths from node s to node t that traverse edge e , and \({g}_{{st}}\) is the total number of shortest paths from s to t . The weight of an edge is considered in these calculations.
The Girvan–Newman algorithm then proceeds by iteratively calculating the weighted betweenness centrality for all edges, identifying and removing the edge with the highest weighted betweenness. The process continues until the desired number of communities is reached or the graph becomes fully disconnected. Mathematically, let \({E}_{k}\) represent the set of edges removed during k iterations, and \({G}_{k}=\left(V,{E}_{k},{W}_{k}\right)\) be the graph after k iterations, where \({W}_{k}\) is the updated weight matrix. The iterative removal of edges partitions the graph into disjoint components, each representing a community. The modularity is used as an optimization criterion to assess the quality of the resulting communities. The Girvan–Newman algorithm for weighted graphs provides a powerful means of community detection, considering both the presence and strength of connections. This adaptation enhances its applicability to diverse systems where edge weights convey additional information about the underlying network structure.
Comparative Analyses
This study introduces five methodologies for comparing network communities. The initial approach involves comparing two distinct community detection methodologies implemented on the same network. The comparison includes the consideration of normalized mutual information, variation of information, split join distance, Rand index, and adjusted Rand index values.
When comparing two weighted network communities obtained by different community detection approaches, it is essential to employ appropriate evaluation metrics to assess the quality of identified communities. One commonly used metric is Normalized Mutual Information (NMI). NMI quantifies the mutual dependence between two sets of communities while considering variations in community sizes. The formula for NMI is given by
where \(I\left({{\mathcal{C}}}_{1};{{\mathcal{C}}}_{2}\right)\) represents the mutual information between the communities \({{\mathcal{C}}}_{1}\) and \({{\mathcal{C}}}_{2}\) , and \(H\left({{\mathcal{C}}}_{1}\right)\) and \(H\left({{\mathcal{C}}}_{2}\right)\) are the entropies of \({{\mathcal{C}}}_{1}\) and \({{\mathcal{C}}}_{2}\) , respectively. NMI ranges from 0 to 1, with higher values indicating greater similarity between community structures.
Variation of Information (VOI) is another crucial metric for community comparison. It captures both the uncertainty within individual communities and the uncertainty about the relationship between them. The formula for VOI is
where \(H\left({{\mathcal{C}}}_{1}\right)\) and \(H\left({{\mathcal{C}}}_{2}\right)\) are the entropies of \({{\mathcal{C}}}_{1}\) and \({{\mathcal{C}}}_{2}\) , respectively. Lower values of VOI correspond to better community detection results.
Split Join Distance (SJD) is a metric that measures the dissimilarity between two community structures by considering how communities split or merge. This metric is particularly suitable for detecting changes in community memberships. The formula for SJD is
where \(s\left({{\mathcal{C}}}_{1},{{\mathcal{C}}}_{2}\right)\) and \(s\left({{\mathcal{C}}}_{2},{{\mathcal{C}}}_{1}\right)\) represent the fraction of nodes split from \({{\mathcal{C}}}_{1}\) to \({{\mathcal{C}}}_{2}\) and from \({{\mathcal{C}}}_{2}\) to \({{\mathcal{C}}}_{1}\) .
Rand Index (RI) and Adjusted Rand Index (ARI) are widely used metrics for assessing the similarity between two sets of partitions. Rand Index quantifies the proportion of pairs of nodes that are correctly classified as either belonging or not belonging to the same community. The Adjusted Rand Index adjusts for chance agreement. The formulas for Rand Index and Adjusted Rand Index are
where \({c}_{1}\) is the number of pairs of nodes in the same community in both \({{\mathcal{C}}}_{1}\) and \({{\mathcal{C}}}_{2}\) , \({c}_{2}\) is the number of pairs of nodes in different communities in both \({{\mathcal{C}}}_{1}\) and \({{\mathcal{C}}}_{2}\) , and \({\mathbb{E}}\left[{RI}\left({{\mathcal{C}}}_{1},{{\mathcal{C}}}_{2}\right)\right]\) is the expected Rand Index.
This paper introduces a novel comparison methodology based on random walk that intends to evaluate networks obtained through various methods, in addition to the five existing methodologies for comparing network communities. In a random walk on a weighted network where lower edge weights signify stronger relationships, we can represent the process mathematically using equations that reflect this inverse relationship.
Let us consider a network represented by \(G=(V,{E}_{F},\omega )\) . Each edge \({e}_{{ij}}\in {E}_{F}\) connecting nodes i and j has an associated weight \({w}_{{ij}}\) . In this network, a lower weight implies a stronger relationship, so the transition probability from node i to node j is inversely proportional to the weight \({w}_{{ij}}\) . The transition probability \({P}_{{ij}}\) for a walker to move from node i to node j is given by:
where \(N(i)\) denotes the set of neighbours of node i , and \({w}_{{ik}}\) are the weights of the edges connecting node i to its neighbours. Here, \(1/{w}_{{ij}}\) represents the ‘strength’ of the connection, and the denominator is the normalization factor ensuring that the sum of probabilities from node i to all its neighbours equals 1.
Over time, the random walk can be analysed in terms of a Markov Chain, whose transition matrix M is defined by the probabilities \({P}_{{ij}}\) . The state of the walker after n steps is given by the distribution vector \({{\boldsymbol{v}}}^{\left(n\right)}\) , where
and \({{\boldsymbol{v}}}^{\left(0\right)}\) is the initial state of the walker.
In the long run, the system may reach a steady state described by a stationary distribution Π , where \({\boldsymbol{\Pi }}{\boldsymbol{M}}={\boldsymbol{\Pi }}\) . This stationary distribution depends intricately on the network’s topology and the inverse weighting of the edges, providing insights into the most ‘strongly connected’ components of the network. This mathematical framework is valuable for understanding various real-world networks, such as financial networks, where stronger ties have less ‘resistance’ or ‘distance’ and thus are more frequently traversed in the random walk process.
Over a sufficiently extended period, the random walker can visit every node in the network. By defining the transitions of the random walker, some nodes will be visited with varying frequencies. Therefore, over time, each node can be assigned distinct values based on their centrality within the network. Given that all networks in this study have the same set of nodes, we begin the random walker at the first node, which is labelled as 1 in the node list. We then proceed with the random walk procedure. The visited nodes will generate a series of values. The methodology employed in this study relies on the calculation of transfer entropy between the series under investigation. When computing the transfer entropy, we examine a process similar to the one outlined in Eq. 8 .
The Borsa Istanbul Sustainability Index (XUSRD) is an important initiative by Borsa Istanbul aimed at promoting and highlighting sustainable business practices among the companies listed on the exchange.
The XUSRD Index, introduced on November 4, 2014, aims to assist organizations in formulating policies that consider the potential risks associated with ESG (Environment, Social, Governance) matters. Additionally, it seeks to educate investors about the sustainability practices implemented by these companies. To qualify for inclusion in this index, organizations must meet certain requirements, including a cumulative ESG score of 50 or higher, individual scores of 40 or higher for each of the ESG pillars, and at least 8 category scores of 26 or higher. As of 2023, the Borsa Istanbul Sustainability Index includes 80 companies.
This index provides a competitive edge to Turkish organizations that effectively manage their corporate risks and opportunities. It serves as a benchmark for organizations to assess and improve their sustainability performance, thereby increasing their visibility, reputation, and potential to attract global investments and clients. The index is based on evaluations conducted by Refinitiv Information Limited using publicly available data, and costs associated with assessing companies are funded by Borsa Istanbul (Hizarci-Payne & Kirkulak-Uludag, 2018 , Yılmaz et al., 2020 ).
Borsa Istanbul’s adoption of this index reflects its commitment to sustainability and responsible investment, aligning with the growing global focus on environmental, social, and governance matters. The index incentivizes organizations to continually enhance their sustainability performance, serving as a measurable standard for the creation of various financial instruments such as funds, exchange-traded funds, and structured products that adhere to sustainability principles.
We omitted KRDMR due to its missing values within the specified time frame. Furthermore, we omitted ISBTR because the ISCTR code is also a representation of the same corporation. The time series data used in this study originated from the 78 companies traded in XUSRD. Each time series has 249 observations and includes the daily closing prices of companies traded in each index. Descriptive statistics for the dataset are provided in Table A1 .
Choosing the Borsa Istanbul Sustainability Index for network analysis, with an emphasis on sustainability and corporate responsibility, offers several advantages compared to the broader Borsa Istanbul 100 Index. The Borsa Istanbul Sustainability Index is a specialized index comprising companies that demonstrate exceptional sustainability performance. This focus allows for a detailed examination of how sustainability impacts company networks, investor relations, and market behaviours, highlighting the increasing significance of sustainability in investment decisions. Additionally, by evaluating the included companies based on these criteria, the index provides a unique perspective on ESG concerns. This enables an understanding of the interaction between ESG variables and company policies, which is less apparent in more comprehensive indices such as the Borsa Istanbul 100 Index. Examining the Borsa Istanbul Sustainability Index can uncover novel and insightful market patterns due to the dynamic nature of sustainability in stock indices. Moreover, companies that prioritize sustainability often exhibit greater resilience to environmental and social risks, providing valuable insights into risk management strategies that enhance corporate stability.
ESG scores are specifically formulated to assess a company’s proficiency in addressing diverse sustainability and ethical obstacles. The ratings are divided into various essential sections, each emphasizing different aspects of a company’s operations and influence.
The emissions score assesses a company’s greenhouse gas emissions, considering both the overall emissions generated and the intensity of those emissions in relation to company production. It discusses the company’s efforts to control and diminish these emissions through diverse programs and adherence to environmental rules. The resource use score evaluates the effectiveness and long-term viability of a company’s utilization of natural resources. Important indicators include quantifying water and energy consumption, the amount of trash produced and recycled, and the utilization of sustainable resources. In this area, companies that successfully execute efficient resource management techniques achieve better scores. The innovation score evaluates a company’s allocation of resources towards research and development (R&D) and its capacity to generate novel ideas and solutions that promote sustainability. This includes the creation of novel technologies and goods that minimize harm to the environment, as well as the acquisition of patents pertaining to sustainable technologies. The human rights score focuses on analysing a company’s policies and practices related to safeguarding human rights in its operations and supply chain. It covers topics such as workers’ rights, equitable remuneration, discrimination regulations, and initiatives to combat child and coerced labour. The product responsibility score assesses a company’s ability to effectively handle the safety, quality, and environmental consequences of its products. It encompasses factors such as adherence to product safety regulations, assessment of the environmental impact throughout the product lifecycle, and the incorporation of sustainable materials in the product development process.
The workforce score evaluates how a company treats its employees, with a specific focus on labour practices, employee health and safety, training and development opportunities, and activities related to diversity and inclusion. Companies that cultivate a constructive and fair work atmosphere typically achieve higher scores. The community score is used to measure a company’s level of involvement and influence in the communities where it operates. It includes humanitarian endeavours, community development initiatives, and efforts to address local concerns and make a positive impact on society. The management score domain assesses the calibre and efficiency of a company’s governance structures and processes. This includes the board structure and diversity, executive remuneration, risk management, and overall corporate governance regulations. The shareholder score evaluates how a company prioritizes and resolves its shareholders’ interests and concerns. It considers factors such as transparency, communication, and the company responsiveness to shareholder resolutions and suggestions. The corporate social responsibility (CSR) strategy score evaluates a company’s comprehensive approach to integrating social and environmental factors into its commercial strategy. The CSR framework encompasses company’s dedication to CSR values, execution of CSR initiatives, and documentation of CSR actions and results.
Table A2 details the ESG and sub-scores for each company analysed in this study. These scores are based on self-reported data from each company for the fiscal years 2022 or 2023. The ESG scores encompass a range of subcategories, including Emissions, Resource Use, Innovation, Human Rights, Product Responsibility, Workforce, Community, Management, Shareholders, and CSR Strategy. Each sub-score provides insight into specific aspects of a company’s environmental, social, and governance practices, offering a comprehensive view of their sustainability performance.
The goodness-of-fit metrics provided in Table 1 shed light on the effectiveness of the linear regression model in explaining the variability in ESG scores of companies in the Borsa Istanbul Sustainability Index. Adjusted R -squared value of 0.9402 suggests that the model can account for approximately 94% of the variability in ESG scores, considering the number of predictors. The adjusted R -squared is particularly useful because it adjusts for the number of variables in the model, preventing overestimation of the model’s explanatory power. A value close to 1 suggests a very good fit. The Akaike Information Criterion (AIC) is a measure of the relative quality of statistical models for a given dataset. It provides a means for model selection, with lower values indicating a better-fitting model. In this context, an AIC of 377.12 suggests that the model is relatively efficient in balancing goodness-of-fit and complexity. The Bayesian Information Criterion (BIC) is another criterion for model selection, similar to AIC, but with a stricter penalty for models with more parameters. A lower BIC indicates a better model fit. The BIC value of 405.44 suggests that, while the model is complex, it balances this complexity with data fit. The R -squared value of 0.948 indicates that the model explains about 94.8% of the variability in ESG scores. It is a measure of how well the regression predictions approximate the real data points. A value close to 1 indicates the strong explanatory power of the model. In summary, the high R -squared and adjusted R -squared values indicate that the model is highly effective in explaining the variability in ESG scores. Although AIC and BIC values suggest some model complexity, they support the model fit and efficiency.
The linear regression model in Table 2 provides useful insights into the impact of many factors on the ESG ratings of companies in the Borsa Istanbul Sustainability Index. The intercept, with an estimated value of 4.911, is not statistically significant. This means that the basic ESG score, when all other factors are held constant, does not provide meaningful information on its own. The coefficient for Emission is positive (0.0279) but lacks statistical significance, suggesting that emission levels have a negligible and non-significant influence on ESG ratings. On the other hand, there is a strong and positive link between Resource Use and ESG ratings, as indicated by a coefficient of 0.117 and a p -value of \(6.7\times {10}^{-6}\) . This implies that effective allocation of resources is closely linked to improved ESG performance.
The impact of Innovation on ESG scores is extremely significant, as evidenced by a coefficient of 0.054 and a p -value of \(6.6\times {10}^{-6}\) . This shows the crucial role of innovation in attaining higher ESG ratings. The analysis indicates that there is a substantial and positive relationship between human rights practices and ESG scores. The coefficient of 0.05 and the p -value of 0.039 provide statistical evidence for this relationship. It suggests that organizations with stronger human rights practices generally get higher ESG scores. The coefficient of 0.089 and the p -value of 0.00014 indicate that there is a strong link between product accountability and higher ESG ratings. This means that organizations that accept responsibility for their goods are more likely to have better ESG scores. Workforce, with a coefficient of 0.173 and a p -value of \(6.7\times {10}^{-6}\) , exhibits a statistically significant positive relationship with ESG scores. Hence, implementing effective workforce practices is crucial for attaining high ESG ratings. The statistical analysis reveals that community participation has a coefficient of 0.14 and a p -value of \(1.5\times {10}^{-8}\) , indicating a high level of significance. This emphasizes the crucial role of community involvement in improving ESG performance. The analysis reveals a significant and positive relationship between management practices and ESG scores. The coefficient of 0.197 indicates a substantial impact, while the p -value of \(1.3\times {10}^{-21}\) suggests a highly significant relationship. These findings underscore the importance of Management in achieving high ESG scores. The impact of Shareholders on ESG ratings is substantial, as indicated by a coefficient of 0.041 and a p -value of 0.00117. This suggests that active shareholder participation has a favourable effect on ESG scores. Furthermore, the coefficient of CSR Strategy is 0.036 with a p -value of 0.0188, suggesting that a strong CSR strategy is linked to elevated ESG scores.
ANOVA results in Table 3 provide an in-depth analysis of the impact of various factors on the ESG scores of companies included in the index. Each factor is evaluated in terms of its contribution to the overall model and its statistical significance, as indicated by the sum of squares (SS), mean squares (MS), F -statistics, and p -values.
Emission shows an F -statistic of 224.437 with a highly significant p -value of \(4.5\times {10}^{-23}\) , indicating a substantial impact on ESG scores. Resource Use has an F- statistic of 418.749 and a p -value of \(1.5\times {10}^{-30}\) , highlighting its critical importance in the model. Innovation, with an F -statistic of 27.1374 and a p -value of \(1.9\times {10}^{-6}\) , also significantly influences ESG scores. Human Rights is another highly significant factor, reflected by its F -statistic of 123.313 and a p -value of \(7.7\times {10}^{-17}\) . Product Responsibility shows a moderate significance with an F -statistic of 4.46292 and a p -value of 0.038. Workforce management is strongly significant, indicated by an F -statistic of 152.156 and a p -value of \(6.6\times {10}^{-19}\) . Community involvement also plays a significant role, with an F -statistic of 58.7096 and a p -value of \(9.9\times {10}^{-11}\) . Management practices, with an F -statistic of 194.79 and a p -value of \(1.6\times {10}^{-21}\) , underscore the importance of governance. Shareholders’ involvement is significant, as shown by an F -statistic of 11.0519 and a p -value of 0.00144. Finally, the CSR Strategy demonstrates significance with an F -statistic of 5.79067 and a p -value of 0.01887. These results collectively confirm that factors significantly impact ESG scores, reflecting their multifaceted roles in sustainability performance.
Our study uses the ESG scores of companies in the XUSRD dataset, which is highly suitable for examining the impact of various factors on community formation in different network types (correlation networks, mutual information networks, causality networks). The relevance of ESG scores lies in their comprehensive measure of a company’s performance across environmental, social, and governance dimensions, which aligns perfectly with our aim to investigate the factors influencing community formation based on ESG-related criteria. The dataset includes a diverse range of variables, ensuring a comprehensive analysis of these factors. The high adjusted R -squared value demonstrates statistical robustness, indicating that the model explains a substantial portion of the variance in ESG scores. This provides confidence in the ability of data to reveal meaningful patterns and relationships. With data from 78 companies and 249 observations for each time series, the dataset is sufficiently large to allow for robust statistical analyses, making the findings generalizable and not biased by the small sample size.
The subsequent part of this section encompasses networks acquired using various techniques, as well as the graph communities derived from these networks. Such networks are constructed based on distinct methodologies including correlation distance, mutual information (both continuous and discrete), and causality (linear and nonlinear). Each method provides a unique perspective on the interactions and dependencies among companies in the Borsa Istanbul Sustainability Index, highlighting different aspects of their relationships.
The figures used in the following subsections incorporate colour-coded indications to represent sectors to which companies belong, facilitating a visual understanding of sector-specific clustering and community formation. The node colours represent the sectors of each company according to the following rule: red represents commercial, green represents energy, blue represents finance, yellow represents manufacturing, orange represents technology, black represents transportation. This colour-coding scheme allows for an intuitive comparison of sectoral distribution within and across the different network types.
Results on correlation networks
The correlation network depicted on the left side of Fig. 1 illustrates the interconnectedness of companies based on the correlation of their daily closing prices, with nodes colour-coded by sector. The network topology reveals clusters of companies, signifying strong intra-sector connections, especially among manufacturing companies that typically display higher vertex degrees. This suggests that companies within the same sector tend to have more similar stock price movements, reflecting shared market influences and sector-specific factors. The weighted vertex degree distribution on the top right shows that most companies have a moderate number of connections, with some having significantly higher connectivity, reflecting their central role in the network. The edge weight distribution on the bottom right indicates that most connections between companies are relatively strong, as evidenced by the clustering of edge weights around higher values. This suggests robust correlations in daily closing prices between many companies, highlighting the significant influence of market dynamics and sector-specific trends within the network. Overall, the network topology and distributions suggest a well-connected structure, with certain sectors, particularly manufacturing, playing a pivotal role in market dynamics.
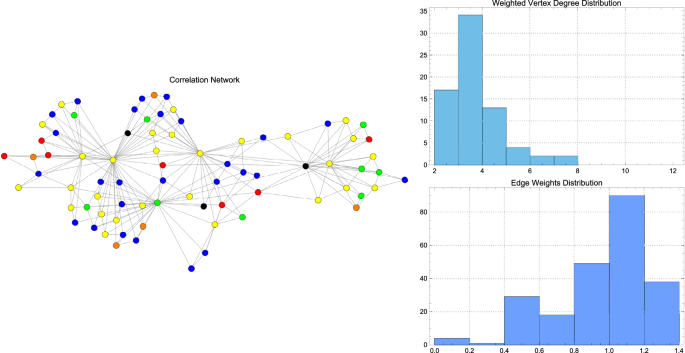
TMFG with correlation distance and weighted degree distribution.
Figure 2 shows the clustering results of the correlation network based on company daily closing prices, with clusters identified using the Leading Eigenvector method on the left and the Girvan–Newman method on the right.
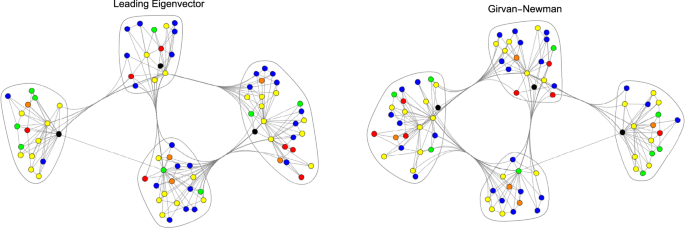
Emerging filtered correlation network communities.
In Fig. 2 both methods reveal distinct communities within the network, with nodes colour-coded by sector, as mentioned before. The Leading Eigenvector method identifies several well-defined clusters, indicating strong intra-sector correlations, particularly within the manufacturing and financial sectors, which appear to form tight-knit groups. This suggests that companies within these sectors exhibit similar stock price movements, likely driven by sector-specific factors and market conditions. Similarly, the Girvan–Newman method also identifies distinct communities, though the clustering patterns show slight variations compared to the Leading Eigenvector method. The financial and manufacturing sectors again show significant clustering, highlighting their strong internal correlations. Both methods reveal that companies within the same sector tend to cluster together, reflecting shared market influences and sectoral trends. Overall, clustering results from both methods show how important sectoral affiliation is in determining how daily closing prices are correlated, with clear community structures showing up in the manufacturing and financial sectors. It indicates that these sectors have more homogenous price movements, likely due to common economic drivers and market conditions.
Table A3 shows the community results that indicate how the Leading Eigenvector and Girvan–Newman algorithms found communities in the correlation network. Each community includes companies from diverse sectors. This diversity within communities indicates that companies from distinct sectors exhibit significant correlations in daily closing prices, likely reflecting interconnected market behaviours and shared external influences. For instance, in Community 1, one sees a mix of financial, manufacturing, and energy companies, suggesting that sectors may be highly correlated in their stock price movements. The presence of companies from multiple sectors within each community is consistent with previous research on significant sectoral interdependencies in the Borsa Istanbul market. Moreover, the weighted vertex degree and eigenvector centrality metrics emphasize the roles and significance of specific companies within their respective communities. In Community 1, for example, companies in the commercial sector like MGROS have a high-weighted vertex degree of 3.67612 and an eigenvector centrality of 0.0601084, which shows that they have a lot of strong connections and influence in the network. Similarly, in the financial sector, ISGYO has a weighted vertex degree of 4.43027 and an eigenvector centrality of 0.0735354, highlighting its critical role in the network structure. In Community 3, TOASO from the manufacturing sector has a weighted vertex degree of 3.41767 and an eigenvector centrality of 0.2251145, making it a central player in maintaining network cohesion.
Results on mutual information networks
This study employs the Kraskov estimator (Kraskov et al., 2004 ) to compute the continuous mutual information values for logarithmic returns of daily closing prices of the companies listed in the XUSRD index.
Figure 3 highlights a network derived from continuous mutual information between company daily closing prices, displayed on the left side.
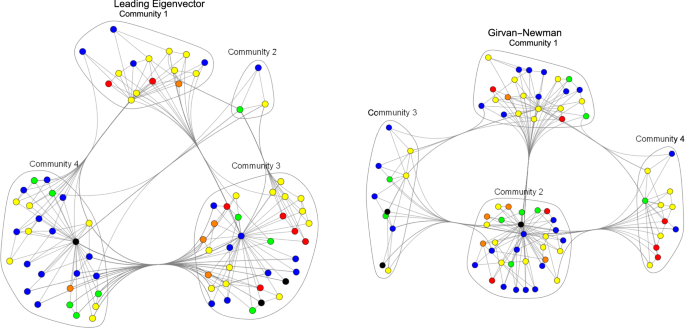
TMFG with continuous mutual information and weighted degree distribution.
The network topology reveals a high degree of interconnectedness, particularly among the financial and manufacturing sectors, which exhibit higher vertex degrees in Fig. 3 . This indicates that sectors share significant informational dependencies, reflecting similar responses to market conditions. The weighted vertex degree distribution, shown on the top right, highlights that most companies have a moderate number of connections, with a few companies having very high degrees, indicating their central role in the network. The centrality of these companies implies their status as key players, closely monitored and highly influential in stock price movements. The edge weights distribution, depicted on the bottom right, shows that most connections have relatively high weights, indicating strong mutual information between closing prices of companies. This suggests that company stock prices contain significant shared information, likely driven by sector-specific dynamics and overall market trends. Network topology and distributions indicate a robust structure, with certain sectors, particularly financials, and manufacturing, playing pivotal roles in the mutual information network based on daily closing prices. This reflects substantial informational linkages within these sectors, emphasizing their importance in the market’s overall behaviour.
Figure 4 displays communities identified in the continuous mutual information network based on company daily closing prices.
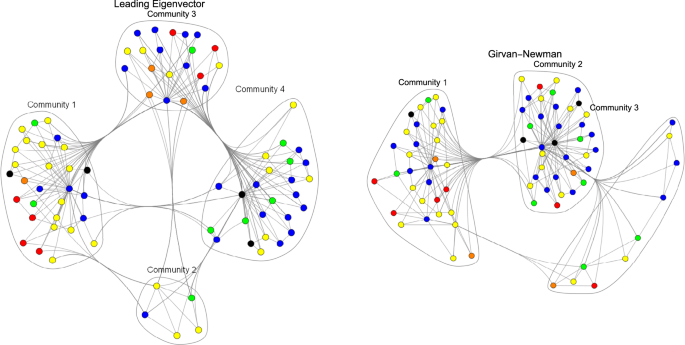
Emerging filtered continuous mutual information network communities.
In Fig. 4 , the left side, derived by using the Leading Eigenvector method, reveals four distinct communities. Community 1 and Community 4 predominantly consist of companies from the financial and manufacturing sectors, with strong intra-sector information sharing. Community 2 and Community 3 include a mix of sectors, highlighting inter-sector dependencies. On the right, the Girvan–Newman method also identifies four communities, but with different compositions. While Community 2 centres around a few key financial and commercial companies, Community 1 is diverse, containing companies from various sectors. Community 3 and Community 4 display a blend of manufacturing and technology sectors, illustrating sectoral and cross-sectoral informational relationships. Both methods highlight that companies within the same sector tend to cluster together due to strong mutual information links, reflecting similar market behaviours and shared influences. The variations in community structure between methods underscore different perspectives on how these informational relationships manifest within the network. Overall, clustering results emphasize the significant role of sectoral affiliations in shaping the network topology based on daily closing prices, with the financial and manufacturing sectors showing particularly strong internal and external connections.
In Table A4 , presenting continuous mutual information network communities, we observe the significance of weighted vertex degree and eigenvector centrality metrics in identifying pivotal companies within their respective communities. For example, in the Leading Eigenvector community, the company DOAS in the commercial sector exhibits a high-weighted vertex degree of 13.4232 and an eigenvector centrality of 0.0527802, indicating its central role in network connectivity. Similarly, in the Girvan–Newman community, the company MAVI, also in the commercial sector, displays a notable weighted vertex degree of 51.0328 and an eigenvector centrality of 0.0634826, highlighting its influence within the network structure. These metrics underline the importance of certain companies in maintaining the overall network integrity and their potential impact on market dynamics. By examining these values, one can infer that companies with higher degrees and centrality are likely to be influential in driving the behaviour and performance of their communities, thereby playing crucial roles in market sustainability and stability. For instance, companies like AGESA in the financial sector and KORDS in the manufacturing sector also show significant metrics, reinforcing their key positions in the network.
Figure 5 presents the network based on discrete bivariate mutual information between company daily closing prices.
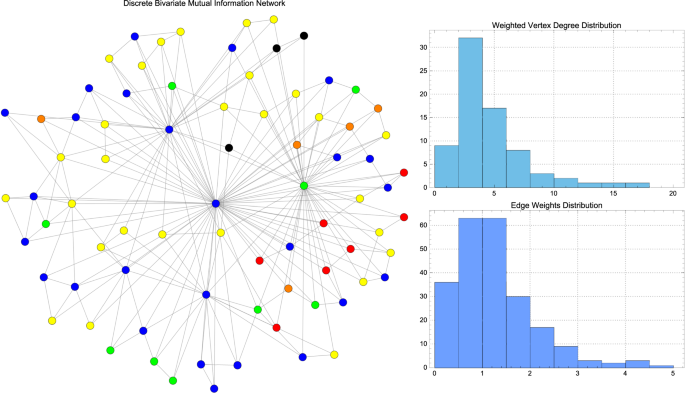
TMFG with discrete bivariate mutual information and weighted degree distribution.
The network topology shows that the manufacturing and financial sectors are closely connected in Fig. 5 , which means that they share a lot of information. This is probably because of factors that are specific to each sector and the way markets are set up. The weighted vertex degree distribution on the top right shows that most companies have a moderate number of connections, with a few companies exhibiting high connectivity, suggesting their central role in the network. Central companies are likely influential in the market, as their price movements provide significant informational content for other companies. The edge weight distribution on the bottom right shows that most connections have high mutual information values, indicating strong relationships between company closing prices. The high mutual information suggests that stock price movements share substantial information, potentially due to shared economic influences or sector-specific dynamics. Overall, the network topology and distributions show that sectors like manufacturing and finance are very important, with a lot of information flowing within and between sectors. This underscores the importance of these sectors in the overall market behaviour, as reflected by the discrete bivariate mutual information derived from daily closing prices.
Figure 6 displays communities identified using discrete bivariate mutual information between daily closing prices, with results from the Leading Eigenvector method on the left and the Girvan–Newman method on the right.
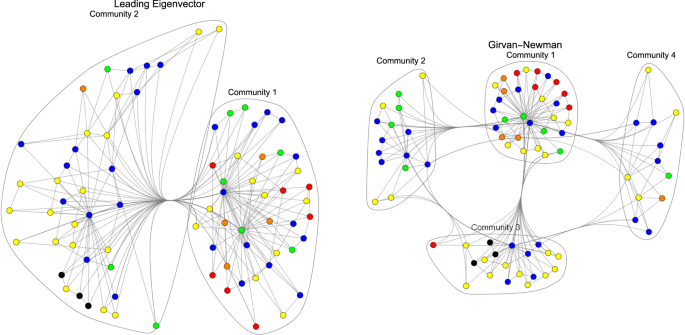
Emerging discrete bivariate mutual information network communities.
The Leading Eigenvector method reveals two major communities in Fig. 6 . Community 1 consists primarily of a mix of financial and manufacturing companies, indicating strong intra-sector mutual information linkages. Community 2 also includes a diverse mix of sectors, highlighting the presence of inter-sector dependencies and shared market behaviours. The Girvan–Newman method identifies four distinct communities. Community 1 is diverse, comprising companies from various sectors, which suggests significant cross-sectoral informational relationships. Community 2 and Community 4 display clusters of manufacturing and financial companies, respectively, reflecting strong sector-specific mutual information linkages. The transportation and energy sectors notably centre in Community 3, indicating specialized inter-sectoral connections. The methods indicate that companies within the same sector tend to form clusters due to high mutual information values, reflecting similar stock price movements driven by common economic factors and sector-specific trends. The differences in community structures between the two methods provide complementary views on how informational relationships manifest within the network, emphasizing both intra-sector and cross-sector dependencies in the financial market. Overall, these clustering results highlight the crucial role of sectoral affiliation in forming a mutual information network based on daily closing prices, particularly emphasizing the financial and manufacturing sectors.
In the discrete bivariate mutual information network, community structures reveal different dynamics, as can be seen in Table A5 . For example, companies like BIMAS in the commercial sector and TUPRS in manufacturing stand out with high-weighted vertex degrees and significant eigenvector centrality. BIMAS, with a weighted vertex degree of 12.8068 and an eigenvector centrality of 0.0327421, is a key player within its community, indicating strong interconnections and influence. This centrality suggests that BIMAS is crucial for the robustness and cohesion of its network cluster. In the manufacturing sector, TUPRS shows a significant presence with a vertex degree of 8.4654, reflecting its substantial role in community connectivity and information dissemination.
Results on causality networks
Figure 7 displays the network, its weighted vertex degree distribution, and edge weights, as well as the network that has been thresholded using the p -values of the linear casualty test. For the linear causality test, we use the order of 1.
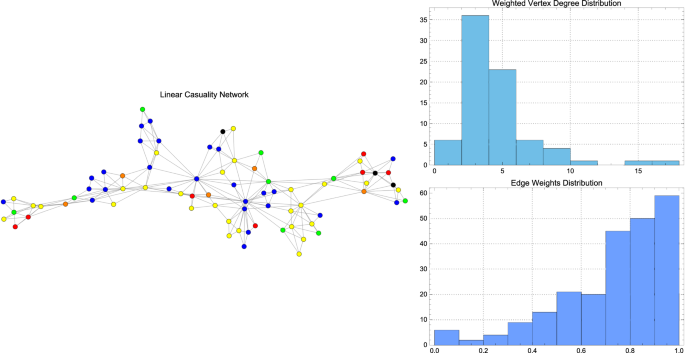
TMFG with linear causality and weighted degree distribution.
The network topology in Fig. 7 shows a well-connected structure with several clusters, particularly within the financial and manufacturing sectors, indicating strong linear causal relationships among companies. This suggests that stock price movements in these sectors can predict each other, reflecting shared market influences and sector-specific dynamics. The weighted vertex degree distribution on the top right reveals that most companies have a moderate number of connections, with a few having very high connectivity, indicating their significant influence within the network. These central companies are likely key players whose price movements are pivotal in driving overall market trends. The edge weight distribution on the bottom right shows that most connections have high weights, indicating strong linear causality between company closing prices. This suggests that the price movements of these companies are highly predictive of each other, further emphasizing the interdependence within sectors. Overall, the network topology and distributions suggest a robust structure, with certain sectors, particularly financial and manufacturing, playing pivotal roles. The presence of strong linear causal relationships highlights the importance of these sectors in the market’s overall behaviour, as reflected by daily closing prices.
Using the Leading Eigenvector and the Girvan–Newman algorithm, the network communities depicted in Fig. 8 were generated.
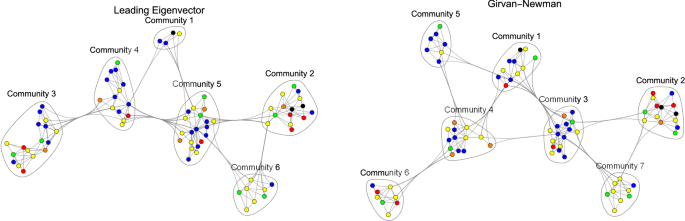
Emerging filtered linear causality network communities.
Figure 8 shows the communities identified in the linear causality network based on company daily closing prices, using the Leading Eigenvector method on the left and the Girvan–Newman method on the right. The Leading Eigenvector method reveals six communities. Financial and manufacturing companies primarily compose Community 1 and Community 2, indicating strong intra-sector linear causal relationships. Communities 3–5 include a mix of sectors, suggesting the presence of significant inter-sector dependencies. Community 6 shows a smaller cluster with notable interactions within the energy sector. The Girvan–Newman method identifies seven communities with a slightly different structure. While Community 3 and Community 4 centre around key financial and commercial companies, reflecting strong internal causal influences, Community 1 and Community 2 also display a mix of sectors. Communities 5–7 demonstrate clusters that include companies from various sectors, underscoring inter-sectoral causal connections. Both methods highlight that companies within the same sector tend to form clusters due to strong linear causal relationships, indicating that their stock price movements are predictive of each other.
Table A6 displays linear causality network communities. It shows that financial companies such as AKBNK and GARAN are at the centre of Community 1, which means they play a big part in how the network changes over time based on linear causal relationships derived from daily closing prices. Similarly, Community 2 also features key financial players such as HALKB and ISCTR, highlighting the strong interconnections within the financial sector. Conversely, Communities 3 and 4 prominently group manufacturing companies such as TOASO, VESTL, and ARCLK. This pattern shows that linear causality is a good way to explain sector-based clustering. It shows how network causal influences line up with the boundaries between sectors. Interestingly, the Leading Eigenvector and Girvan–Newman community detection methods consistently observe these sectoral clusters, demonstrating their robustness in identifying meaningful community structures. Moreover, the weighted vertex degree and eigenvector centrality metrics further emphasize the importance and connectivity of these companies within their respective communities, highlighting their pivotal roles in market dynamics. For example, AKBNK and GARAN exhibit high vertex degrees and eigenvector centrality, indicating their influential positions within the financial sector. Similarly, manufacturing giants like TOASO and VESTL show significant centrality metrics within their clusters, underscoring their critical roles in the manufacturing sector’s network dynamics.
Figure 9 displays the thresholded network, along with its weighted vertex degree and edge weight distributions, as well as the p -value of nonlinear casualty. The following parameters are set for the computation of nonlinear casualty: with a learning rate of 0.01 and a number of iterations set to 50, the hidden layer size for the bivariate model is 4 and that for the univariate model is 2. Additionally, the stochastic gradient descent algorithm is employed.
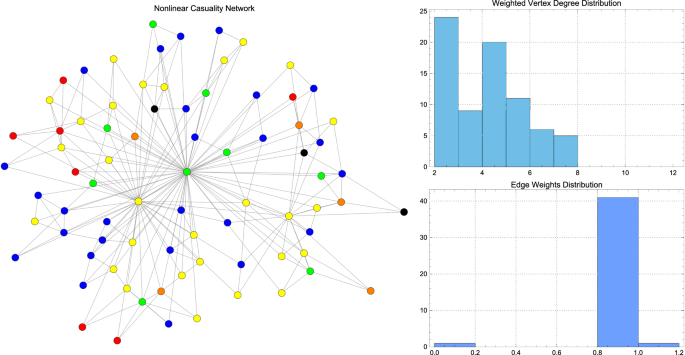
TMFG with nonlinear causality and weighted degree distribution.
The network topology depicted in Fig. 9 exhibits a complex and interdependent structure with multiple core nodes, particularly within the banking and manufacturing sectors. This suggests the presence of robust nonlinear causal linkages among these organizations. The upper right corner displays the vertex degree distribution, which accounts for the weights of the connections. It indicates that most companies have a moderate number of connections, while certain organizations have a significantly high degree, suggesting their central position in the network. These pivotal companies are important actors whose unpredictable price swings have a substantial impact on other enterprises in the network. The distribution of edge weights, shown in the bottom right, reveals that most connections have high weights, showing a significant nonlinear causation between company closing prices. The closely connected price fluctuations of these companies highlight intricate connections among sectors. In general, the network topology and distributions indicate a strong and resilient structure, with the financial and manufacturing sectors playing important roles.
Figure 10 shows the nonlinear network communities that were detected by using the Leading Eigenvector and the Girvan–Newman algorithm.
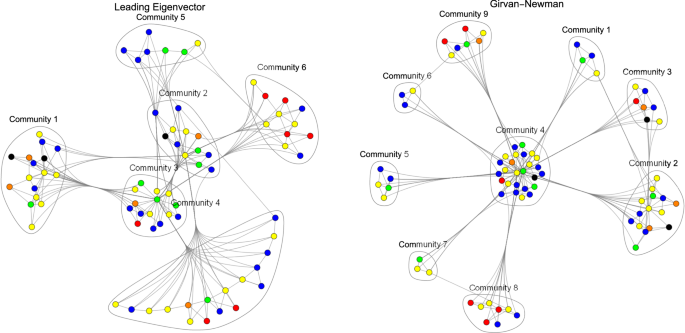
Emerging filtered nonlinear causality network communities.
In Fig. 10 , the Leading Eigenvector method reveals six communities. Manufacturing and financial companies predominantly compose Community 1 and Community 4, indicating strong intra-sector nonlinear causal relationships. Communities 2, 3, and 5 include a diverse mix of sectors, highlighting significant inter-sector dependencies. Community 6 shows a cluster primarily centred around the commercial sector, with sector-specific nonlinear interactions. The Girvan–Newman method identifies nine communities, with Community 4 being the largest and most central, incorporating a mix of sectors that suggests significant cross-sectoral nonlinear causal relationships. Community 1 and Community 2 display a concentration of financial and manufacturing companies, indicating strong sector-specific nonlinear influences. Communities 5 through 9 demonstrate smaller clusters, often involving a combination of sectors, underscoring the presence of inter-sectoral interactions. Both methods stress that companies in the same sector tend to group together because of strong nonlinear causal relationships. This is because similar stock price changes are caused by complex market dynamics and factors that are unique to the sector. Mixed-sector communities are present in both methods, indicating significant cross-sectoral interactions within the market.
Table A7 illustrates the nonlinear causality network communities, showcasing a diverse clustering pattern based on nonlinear relationships between company daily closing prices. Community 1 prominently features energy companies like ZOREN and manufacturing companies like SISE and TOASO, indicating strong nonlinear causal connections within these sectors. Financial firms like GARAN and manufacturing companies like EREGL are central in Community 2, highlighting their significant roles in nonlinear interactions. This table confirms that nonlinear causality induces a unique clustering dynamic that intertwines multiple sectors, differing from linear causality, which predominantly segregates sectors. The presence of companies from different sectors within the same community emphasizes the complex, multifaceted nature of nonlinear relationships in financial markets. This intricacy is evident in companies such as AKBNK and TUPRS, which exhibit high eigenvector centrality and influence, playing pivotal roles across multiple communities. This further underscores the importance of considering nonlinear causality to fully understand the interdependencies and the structural topology of financial networks.
Results of network and community comparisons
Figure 11 displays the series that were derived from the edge evaluation using a random walker with a larger transition probability compared to low edge weights. A random walk method with 10,000 steps was chosen.
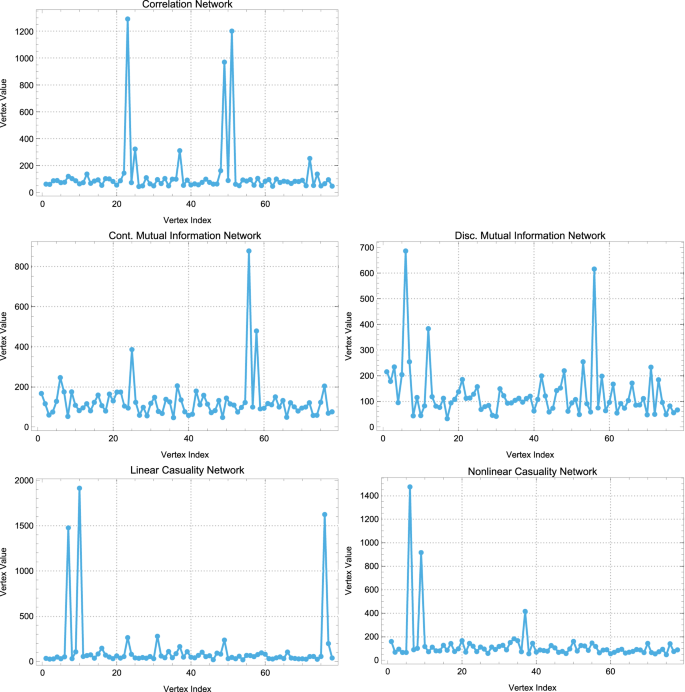
Node visiting values of random walker for different networks.
In Fig. 11 , in the correlation network, specific vertices exhibit very high visit frequencies, indicating their significant centrality and influence within the network. Similar patterns emerge in the continuous and discrete mutual information networks, where certain nodes receive more frequent visits, indicating their crucial roles in information sharing. Linear causality network reveals prominent nodes with high visit frequencies, emphasizing their strong predictive relationships. Nonlinear causality network displays a more distributed pattern, with several vertices showing high centrality, reflecting complex nonlinear dependencies.
To determine the transfer entropy, we adhere to the steps described by Behrendt et al. ( 2019 ). By setting the lags for series to 1, we enable the function to consider the impact of previous values on subsequent ones. We usually set the quantile threshold for calculating transfer entropy at 0.1 to give more weight to extremes of distributions. We use Shannon’s entropy to calculate the transfer entropy. We sort data into quantiles, bins, and limits, then shuffle the data 100 times. We determine the uncertainty of the transfer entropy estimate using 300 bootstrap samples and maintain the stability of the bootstrap estimate by discarding 30 initial samples.
The resulting transfer entropies in the form of \({G}_{F}1\to {G}_{F}2\) are presented in Table 4 .
The values in Table 4 show transfer entropies between various types of networks, including correlation, continuous mutual information, discrete mutual information, linear causality, nonlinear causality. These values come from random walker distribution. Transfer entropy quantifies the amount of directed (asymmetric) information transferred between two processes, in this case, different network structures. Higher values indicate greater predictability or influence from one network to another. Starting with the correlation network, we see relatively high transfer entropy values when compared to other networks, with the highest influence observed towards the nonlinear causality network (0.028385). This suggests that information flows from the correlation network to the nonlinear causality network is significant, indicating that relationships captured by the correlation are relevant to the nonlinear dependencies.
The linear causality network has the highest transfer entropy for the continuous mutual information network (0.0333629). This means that continuous mutual information is most useful for understanding linear causal relationships. This highlights the importance of mutual information in understanding linear dependencies in the network. The discrete mutual information network has moderate transfer entropy values across all other networks, with the highest influence on the linear causality network (0.0280271). Discrete mutual information captures substantial interactions, which linear causality also reflects. The nonlinear causality network receives the highest transfer entropy (0.039678) from the linear causality network, indicating a strong influence of linear causal relationships on nonlinear dependencies. This high value suggests that linear causality encapsulates critical aspects of the dynamics that are further detailed in nonlinear interactions.
Finally, the nonlinear causality network shows significant transfer entropy towards the linear causality network (0.113118), the highest observed in the table. This shows how complicated and two-way this influence is between linear and nonlinear causal networks. It also shows how nonlinear dependencies offer a bigger picture to understand linear causal effects. In summary, transfer entropy values illustrate the interconnectedness and predictive power between different network types. Both linear and nonlinear correlation and causality networks have significant values. This suggests that these networks are capturing important, overlapping parts of financial data.
In addition to looking at the topological structures of networks that were made, it is also important to look at how well communities that were created using two different approaches can cluster. Table 5 displays the outcomes for comparative metrics specified in the preceding sections.
Community comparison metrics in Table 5 provide a comprehensive evaluation of community detection performance across different network types. The correlation network shows a moderate VOI of 1.126, indicating a relatively average level of information loss between true and detected communities. Its NMI of 0.588348 suggests a decent alignment of detected communities with true communities. The SJD of 42, RI of 0.8022, and ARI of 0.4722 further confirm that while communities are reasonably accurate, there is room for improvement in capturing the intricate structures within the network. The continuous mutual information network has the highest VOI of 1.715, indicating significant information loss and less effective community detection. Its NMI is the lowest at 0.3039, reflecting poor correspondence with true communities. The high SJD of 66 also points to considerable discrepancies in community structure, while the RI (0.6567) and ARI (0.1977) indicate relatively weak community detection performance. In contrast, the discrete bivariate mutual information network performs better with a lower VOI of 1.102 and a moderate NMI of 0.4528. The SJD of 37 suggests a more accurate community structure compared to the continuous counterpart. The RI of 0.7126 and ARI of 0.422 indicate an overall moderate performance, balancing between information preservation and accurate community detection. The linear causality network exhibits the best performance, with the lowest VOI of 0.866, indicating minimal information loss. Its NMI of 0.7593 is the highest among all networks, showing strong alignment with true communities. The SJD of 32, along with high RI (0.899) and ARI (0.6356), demonstrates superior community detection accuracy, capturing directed influences effectively.
Finally, the nonlinear causality network has the lowest VOI of 0.573, indicating the least information loss. Its NMI of 0.573 suggests good correspondence with true communities, although not as high as the linear causality network. The SJD of 53 indicates some discrepancies in community structure, but the RI (0.8151) and ARI (0.3471) reflect a strong performance, particularly in capturing nonlinear relationships.
Overall, these results highlight that while continuous mutual information struggles to capture community structures effectively, linear and nonlinear causality networks provide more accurate and robust community detection, reflecting their capability to model complex dependencies in financial networks. The correlation network offers balanced performance, but improvements can be made by leveraging causality-based methods to capture deeper insights into community structures.
Discussions
The next phase of this study involves examining how Environmental, Social, and Governance (ESG) scores impact the clustering of companies within these networks. By performing nonparametric tests on the ESG scores, we aim to determine whether these sustainability metrics significantly affect the formation and structure of communities within the various network models. This analysis will provide insights into the role of ESG factors in shaping financial networks and their potential to drive sustainable investment practices.
We will use nonparametric tests like the Kruskal–Wallis, Conover, and Gehan to evaluate how ESG scores impact clustering patterns in networks. These tests are particularly suitable because they do not assume a specific data distribution, making them robust for evaluating the relationships between ESG scores and community formation. By utilizing these tests, we can rigorously evaluate whether differences in ESG scores across various clusters are statistically significant, providing a deeper understanding of how sustainability practices influence network topology.
In this context, we will analyse several ESG sub-scores, including Emission, Resource Use, Innovation, Human Rights, Product Responsibility, Workforce, Community, Management, Shareholders, and CSR Strategy. Each of these sub-scores represents a critical aspect of a company sustainability performance and can provide nuanced insights into how different elements of ESG influence network dynamics. For instance, higher scores in Innovation or Resource Use might be indicative of companies that are more central in certain network communities due to their advanced practices and efficient operations.
We will treat ESG scores as an independent variable and the community memberships obtained from the various network models as dependent variables. By comparing the distribution of ESG scores across different communities, we can identify whether certain sustainability practices are more prevalent in specific clusters, indicating a correlation between ESG performance and network position. This approach will help in understanding whether companies with superior ESG metrics tend to cluster together, potentially due to similar strategic goals or operational practices that align with sustainability principles.
Furthermore, this analysis will explore whether certain ESG dimensions are more influential in determining community structure within specific types of networks. For example, in correlation networks, we might find that companies with high Human Rights scores cluster together, reflecting their commitment to social governance aspects. In contrast, in mutual information networks, Innovation scores might play a more significant role in community formation, highlighting the importance of technological advancements and innovative practices in these clusters.
Table 6 presents the results of nonparametric tests for ESG scores across correlation network communities, highlighting significant findings for various sustainability metrics.
In Table 6 , the Kruskal–Wallis test results for the Leading Eigenvector method indicate that Product Responsibility ( \(p=0.061\) ) and Human Rights ( \(p=0.092\) ) are marginally significant, suggesting that these factors might influence community formation to some extent. The Conover test, however, shows a more pronounced significance for Resource Use ( \(p=0.017\) ), indicating a strong impact of resource management practices on community clustering. This is further supported by the log-rank test, which highlights Product Responsibility ( \(p=0.0425\) ) and Human Rights ( \(p=0.092\) ) as notable influencing variables.
For the Girvan–Newman method, the Kruskal–Wallis test reveals significant results for Innovation ( \(p=0.0265\) ) and CSR Strategy ( \(p=0.008\) ), suggesting these elements are key drivers in community formation. The Conover test corroborates the significance of Resource Use ( \(p=0.0182\) ) and Innovation ( \(p=0.0748\) ), indicating consistent findings across different test methodologies. The log-rank test underscores the importance of CSR Strategy ( \(p=0.005\) ) and Innovation ( \(p=0.012\) ), further validating the influence of ESG factors on network communities.
When the findings from the correlation network topologies are considered alongside the results of the nonparametric tests for ESG scores, a nuanced picture emerges. The nonparametric tests, particularly the Conover test for the Leading Eigenvector method, indicate that Resource Use significantly affects community formation. This suggests that companies with similar resource use practices are more likely to cluster together within the network, reinforcing the idea that sustainability strategies are a critical factor in driving stock price correlations. The log-rank test further supports this by highlighting the significance of Product Responsibility ( \(p=0.0425\) ) and Human Rights, indicating that these ESG factors also influence clustering.
For the Girvan–Newman method, the nonparametric tests underscore the importance of Innovation and CSR Strategy, suggesting that companies with strong innovation and CSR strategies tend to form distinct communities. This aligns with the observed clustering patterns, where companies with similar ESG practices are more likely to be grouped together. The significance of these ESG factors indicates that sustainable practices are not only important for individual company performance but also play a crucial role in the broader market dynamics and community structures within financial networks.
Overall, the integration of network community analysis and nonparametric test findings highlights the interplay between sectoral affiliation and ESG practices in determining stock price correlations. The distinct communities formed within the manufacturing and financial sectors, driven by strong internal correlations, reflect the shared market influences and sectoral trends.
Table 7 displays the outcomes of nonparametric tests conducted on ESG scores across continuous mutual information network communities.
The nonparametric test results for ESG scores over continuous mutual information network communities, as presented in Table 7 , offer insightful findings. The tests reveal mixed significance levels across different ESG dimensions and community detection methods, shedding light on the nuanced impact of sustainability metrics on network clustering.
For the Leading Eigenvector method, the Kruskal–Wallis test indicates that none of the ESG dimensions, including Emission, Resource Use, Innovation, Human Rights, Product Responsibility, Workforce, Community, Management, Shareholders, and CSR Strategy, show significant differences between communities (all p -values > 0.05). This suggests that ESG scores do not substantially influence the formation of communities when using this method. Similarly, the Conover and log-rank tests do not show any significant results, reinforcing the lack of substantial impact of ESG scores on community structure in this context.
Conversely, the Girvan–Newman method reveals significant results for several ESG dimensions. The Kruskal–Wallis test shows that Emission ( \(p=0.032\) ) and CSR Strategy ( \(p=0.07\) ) have lower p -values, indicating potential significance. The Conover test highlights no significant differences, while the log-rank test identifies Emission ( \(p=0.034\) ), Innovation ( \(p=0.021\) ), and CSR Strategy ( \(p=0.065\) ) as significantly influencing the clustering of companies. These findings suggest that certain ESG scores, particularly Emission, Innovation, and CSR Strategy, play a more pronounced role in defining community structures within the Girvan–Newman-derived networks.
In the continuous mutual information network, the Girvan–Newman algorithm reveals that Community 1 predominantly consists of diverse sector companies, indicating a broad mix influenced by various ESG factors. However, Community 2 includes key financial and commercial companies, which are likely influenced by strong governance and corporate responsibility practices. Community 3 and Community 4, which display a blend of manufacturing and technology sectors, suggest that companies within these sectors share similar innovation and resource use strategies, aligning with their clustering patterns.
Findings highlight how sustainability metrics, such as Emissions and CSR Strategies, play a critical role in shaping network topology, particularly in the continuous mutual information network. Companies with high scores in these ESG areas tend to form distinct clusters, emphasizing the importance of shared sustainability practices. This clustering not only reflects similar market behaviours but also underscores the systemic impact of ESG performance on financial networks.
Table 8 displays the outcomes of nonparametric tests conducted on ESG scores across discrete bivariate mutual information network communities.
The nonparametric test results from Table 8 for ESG scores over discrete bivariate mutual information network communities present several notable insights.
For the Leading Eigenvector method, significant p -values were observed for CSR Strategy in both the Kruskal–Wallis and log-rank tests, indicating a substantial impact of CSR Strategy scores on community formation. This suggests that companies with similar CSR strategies are more likely to cluster together, reflecting shared commitments to corporate social responsibility and potentially similar operational practices. Additionally, Resource Use showed significance in the Conover test, pointing towards its role in influencing how companies group within the network. In the Girvan–Newman method, only community is significant according to the Conover test, reinforcing its importance for community clustering.
Hence, companies with higher resource efficiency and stronger corporate social responsibility practices tend to cluster together, reflecting shared sustainability priorities. For instance, companies like BIZIM and MGROS, which show significant values in these ESG categories, form part of the same community, emphasizing their alignment in sustainability practices.
ESG test results thus complement the community structures observed in the discrete bivariate mutual information network. Companies with similar ESG scores, particularly in Resource Use and CSR Strategy, tend to form distinct clusters, underscoring the influence of sustainability practices on their stock price interrelations. This alignment suggests that shared ESG goals and operational practices drive the formation of these communities, reflecting the interconnectedness of sustainability performance and financial behaviour. The significant ESG factors highlight key areas where company sustainability practices are most impactful, shaping the network topology and providing insights into drivers of sustainable investment.
Table 9 presents the results of nonparametric tests for ESG scores across linear causality network communities, highlighting significant findings for various sustainability metrics.
The nonparametric test results presented in Table 9 for ESG scores over the linear causality network communities reveal some significant findings, particularly for the Leading Eigenvector method. Notably, the Kruskal–Wallis test shows significant results for Human Rights ( \(p=0.012\) ), indicating that there is a meaningful difference in the Human Rights scores across different communities. This is further supported by the Conover test ( \(p=0.003\) ) and the log-rank test ( \(p=0.022\) ), which also show significant differences, suggesting that companies with similar Human Rights scores tend to cluster together more tightly in the network. This finding implies that HR practices are a critical factor influencing the formation of communities within the linear causality network.
Additionally, the log-rank test reveals significant results for Emission ( \(p=0.034\) ) and Innovation ( \(p=0.02\) ) scores in the Girvan–Newman method, indicating that these ESG metrics also play a substantial role in how companies cluster. This suggests that companies with similar Emission and Innovation scores are more likely to form communities, reflecting shared sustainability practices and innovation strategies.
Results highlight that specific ESG scores, particularly Human Rights, Emission, and Innovation, significantly impact the clustering of companies within the linear causality network.
Communities 3–5, which include a mix of sectors, indicate the presence of significant inter-sector dependencies. This is reflected in the significant Kruskal–Wallis test results for Human Rights under the Leading Eigenvector method, suggesting that companies with similar Human Rights scores tend to cluster together. This clustering could be driven by shared practices and policies that transcend sector boundaries, indicating that human rights practices are crucial in forming these inter-sectoral links. Community 6, which shows notable interactions within the energy sector, aligns with the significant results for Emission scores under the Girvan–Newman method. This suggests that companies within the energy sector with similar Emission scores are more likely to form clusters, reflecting common environmental practices and regulatory influences.
The Girvan–Newman method identifies seven communities with a structure that emphasizes both intra- and inter-sector dependencies. The significant results for Innovation scores indicate that companies with similar innovation practices are likely to cluster together, regardless of their sector. This suggests that innovation is a key factor in forming causal connections within the network, with companies sharing similar Innovation scores showing predictive stock price movements. Additionally, the Conover test results for community scores under the Leading Eigenvector method highlight the importance of community engagement and social responsibility in shaping network communities. Companies with strong community engagement practices are likely to be interconnected within the same community, driven by shared social goals and responsibilities.
Table 10 displays the outcomes of nonparametric tests conducted on ESG scores across nonlinear causality network communities.
The nonparametric test results presented in Table 10 for ESG scores over nonlinear causality network communities provide valuable insights into how specific sustainability metrics influence the clustering of companies.
For the Leading Eigenvector method, several ESG scores show significant impacts on community formation. The Kruskal–Wallis test indicates that Innovation ( \(p=0.019\) ), Human Rights ( \(p=0.02\) ), and Emission ( \(p=0.074\) ) scores significantly affect community structure. These results suggest that companies with similar innovation capabilities, human rights practices, and emission policies are more likely to cluster together. The Conover test further supports the influence of Human Rights ( \(p=0.073\) ) and Workforce ( \(p=0.17\) ) on community formation, although it shows slightly different significance levels. The log-rank test reveals significant impacts for Emission ( \(p=0.056\) ), Resource Use ( \(p=0.045\) ), Innovation ( \(p=0.02\) 2), and Human Rights ( \(p=0.038\) ), emphasizing the role of environmental and social governance factors in driving network clustering.
The Girvan–Newman results align with these findings to some extent but also present unique insights. The Kruskal–Wallis test highlights the significant impact of Human Rights ( \(p=0.057\) ) and Shareholders ( \(p=0.081\) ), suggesting that these factors are crucial in determining how companies group within the network. According to the Conover test, Product Responsibility ( \(p=0.013\) ) and Workforce ( \(p=0.079\) ) had a significant impact. The log-rank test underscores the importance of Human Rights ( \(p=0.023\) ) and Shareholders ( \(p=0.059\) ), suggesting that companies with similar practices in these areas tend to form tighter-knit communities.
The nonparametric test results for ESG scores over nonlinear causality network communities reveal critical insights into the role of sustainability metrics in influencing company clustering. Financial companies such as AKBNK and GARAN, which are central in Community 1, highlight the importance of the Emission and Human Rights scores in community formation. The log-rank test shows significant results for Emission and Human Rights within the Leading Eigenvector method. This suggests that companies with lower emissions and strong human rights policies are more likely to cluster together, reflecting shared sustainability practices that influence their stock price movements.
Similarly, Community 2, which features manufacturing giants like TOASO and VESTL, shows the impact of Innovation and Human Rights scores on clustering. The Kruskal–Wallis test for Innovation and Human Rights indicates that companies excelling in these areas form tighter communities, driven by shared innovative practices and robust human rights policies. This emphasizes the role of ESG factors in shaping sector-specific causal relationships.
The diverse sectoral mix in Communities 3–5 suggests significant inter-sector dependencies, which are influenced by ESG scores. The Conover test for Management within the Leading Eigenvector method highlights that strong management practices are critical for inter-sectoral clustering. This shows that companies with effective management strategies, regardless of their sector, tend to form communities with interconnected stock price movements.
The energy sector’s notable interactions in Community 6 align with significant log-rank test results for Resource Use and Innovation. These findings indicate that companies with efficient resource use and innovative practices within the energy sector form strong causal links, reflecting shared operational efficiencies and sustainable practices.
Conclusions
This study aimed to investigate the clustering behaviour of companies within different network models based on daily closing prices and examine the influence of ESG scores on these clusters. By applying various network analysis methods (i.e., correlation networks, continuous and discrete mutual information networks, linear and nonlinear causality networks), we effectively captured the complex interdependencies and interactions among companies. The nonparametric tests performed on ESG scores allowed us to assess the impact of sustainability metrics on community formation, fulfilling the primary objective of understanding how ESG factors shape financial networks.
The comparison of different network models revealed distinct and multifaceted patterns of interconnectivity among companies, offering a comprehensive view of market dynamics. The correlation networks underscored strong sectoral linkages, particularly within the financial and manufacturing sectors. This suggests that companies within these sectors exhibit similar stock price movements, likely driven by sector-specific factors and market conditions. Continuous mutual information networks provided deeper insights into both intra- and inter-sector dependencies, reflecting more nuanced informational relationships that go beyond simple linear correlations. These networks highlighted how certain sectors are interconnected through shared information flows, revealing a complex web of market interactions.
Discrete mutual information networks emphasized the importance of specific informational exchanges between companies. This model illustrated how discrete events or data points can drive connectivity, showcasing the critical role of individual company actions and announcements in shaping market linkages. On the other hand, linear causality networks demonstrated how stock price movements are predictive of each other, capturing the directional influences and temporal dependencies within the market. This approach highlighted the causal pathways through which market shocks or trends propagate across different companies and sectors.
Nonlinear causality networks expanded this perspective by capturing more complex, nonlinear relationships that may not be evident through linear models. This method revealed hidden dependencies and intricate feedback loops within the market, underscoring the rich and dynamic nature of financial interactions. Collectively, diverse network perspectives underscored the varying degrees of connectivity and influence within the financial market, illustrating the complexity and richness of market dynamics. By integrating multiple models, we obtained a holistic understanding of how companies are interlinked, the role of sectoral affiliations, and the intricate web of causal relationships that drive market behaviour.
The community detection results across different network models consistently highlighted the significant role of sectoral affiliation in forming clusters. The Leading Eigenvector and Girvan–Newman methods both revealed that companies within the same sector, such as financial and manufacturing firms, formed tight-knit communities. This indicates strong intra-sector relationships driven by shared market behaviours and sector-specific factors. The robustness of these methods in identifying sectoral clusters demonstrates their effectiveness in capturing the underlying structure of market networks.
In correlation networks, the financial and manufacturing sectors prominently formed distinct communities, reflecting the high degree of correlation within these sectors. This pattern indicates that companies within these sectors exhibit similar stock price movements, likely influenced by common economic drivers and market conditions. Sector-specific clusters provide valuable insights into how market participants within the same industry are interconnected.
The continuous mutual information networks further emphasized the importance of sectoral clustering, while also revealing significant inter-sector dependencies. Mixed-sector communities identified in these networks highlight how different industries are interlinked through shared informational relationships. For instance, financial and commercial companies forming a single community suggest that these sectors may be influenced by common external factors, leading to shared market behaviours.
Discrete mutual information networks showed specific informational exchanges between companies, illustrating how discrete events or data points can drive clustering. This approach highlighted the critical role of individual company actions in shaping network structures, with sectoral affiliations still playing a key role in forming communities.
Linear and nonlinear causality networks captured the directional and complex relationships between companies. Such networks showed that company stock price movements can predict each other, reinforcing the importance of causal influences in market dynamics. Clustering results from these models revealed strong internal causal influences within sectors like financial and manufacturing, while also showing cross-sectoral causal connections.
Overall, the robust clustering patterns observed across different methods underscore their effectiveness in identifying meaningful community structures. These results provide valuable insights into the underlying drivers of market connectivity, highlighting the importance of both intra-sector relationships and inter-sector dependencies in shaping the financial network topology. By understanding community structures, we can better grasp the dynamics of market behaviours and factors driving company interconnectivity.
The nonparametric test results for ESG scores revealed significant insights into the role of sustainability metrics in community formation within financial networks. Notably, ESG factors such as emission, CSR strategy, innovation, and human rights emerged as significant influence variables in different network models. For instance, in the Girvan–Newman method applied to nonlinear causality network, significant results for Emission and CSR Strategy highlighted their impact on clustering. Findings suggest that companies with strong performance in these ESG areas tend to form more cohesive communities, reflecting shared sustainability practices and priorities. Companies with lower emissions or more robust CSR strategies are more likely to be interconnected within the same community, driven by common goals and operational practices related to sustainability. This underscores the critical role of ESG factors in shaping financial networks and promoting sustainable investment practices.
In the continuous mutual information network, ESG factors such as Human Rights and Innovation showed significant p -values, indicating their substantial impact on community structure. Companies excelling in human rights practices or innovation tend to cluster together, suggesting that these aspects of ESG performance foster strong informational linkages and shared market behaviours. Similarly, the linear causality network revealed significant results for ESG scores like Human Rights and Management, highlighting how companies with strong governance and social responsibility practices influence market connectivity.
The discrete bivariate mutual information network also emphasized the importance of specific ESG factors. Significant results for product responsibility and shareholders indicated that these aspects of sustainability play crucial roles in forming community structures. Companies with high scores in product responsibility tend to cluster together, reflecting their commitment to quality and ethical business practices. This clustering based on shared sustainability priorities reinforces the idea that ESG performance drives market behaviours and intercompany relationships.
Overall, findings across different network models and community detection methods demonstrate the multifaceted impact of ESG factors on financial networks. They highlight how sustainability metrics not only influence individual company performance but also shape broader market dynamics by fostering interconnected, cohesive communities of companies with similar ESG practices. This reinforces the importance of integrating ESG considerations into investment decisions to promote sustainable and responsible financial markets.
Despite the comprehensive analysis, this study has several limitations. Firstly, the reliance on daily closing prices may not capture all market dynamics and intra-day variations. Additionally, the ESG scores used were limited to publicly available data, which may not fully represent the companies’ overall sustainability performance. The static nature of network models also does not account for temporal changes in company relationships and market conditions. Furthermore, the study focused on a specific set of companies within the Borsa Istanbul Sustainability Index, which may limit the generalizability of findings to other markets or indices. Finally, the nonparametric tests (while robust) may not fully capture the complex interactions between ESG factors and network communities.
Future research could address these limitations by incorporating intra-day trading data to capture more granular market dynamics and provide a more detailed understanding of company interdependencies. Expanding the dataset to include a broader range of companies and multiple stock indices could enhance the generalizability of findings. Additionally, longitudinal studies that track the evolution of network structures and community formations over time would provide valuable insights into temporal changes and the impact of external events on market connectivity.
Investigating the impact of different types of ESG scores, such as those derived from third-party assessments or alternative data sources like social media sentiment, could offer a more comprehensive view of sustainability practices. Furthermore, integrating machine learning techniques to dynamically adjust network models based on changing market conditions could enhance the robustness and predictive power of the analysis.
Exploring the interactions between ESG scores and other financial metrics, such as volatility or liquidity, could provide a deeper understanding of how sustainability influences broader market behaviours. Additionally, examining the role of regulatory changes and policy interventions on ESG-driven clustering could inform policymakers and investors about the effectiveness of sustainability regulations.
Conducting cross-market comparisons to assess how ESG factors influence network structures in different economic contexts could yield valuable insights into the global applicability of the findings. Finally, developing real-time monitoring tools based on network analysis and ESG metrics could help investors make informed decisions and promote sustainable investment practices.
In conclusion, this study highlights the intricate relationships between ESG factors and financial network structures, demonstrating the significant impact of sustainability metrics on market connectivity. The application of various network models and nonparametric tests provided a comprehensive understanding of how companies cluster based on their financial performance and sustainability practices. These findings underscore the importance of integrating ESG considerations into financial analysis, paving the way for more sustainable and informed investment strategies. As we move forward, embracing advanced analytical techniques and expanding the scope of research will further enhance our understanding of the complex interplay between sustainability and market dynamics, ultimately contributing to the development of more resilient and sustainable financial systems.
Data availability
The datasets generated during and/or analysed during the current study are available from the corresponding author on reasonable request.
Akgüller Ö (2019) A threshold method for financial networks and geometric scattering of agents. Commun Stat Case Stud Data Anal Appl 5(3):230–242
Google Scholar
Ang G, Guo Z, Lim E-P (2023) On predicting ESG ratings using dynamic company networks. ACM Trans Manag Inf Syst 14(3):1–34
Article Google Scholar
Aybars A, Ataünal L, Gürbüz AO (2019) ESG and financial performance: impact of environmental, social, and governance issues on corporate performance. In: Dincer H, Yüksel S (eds.) Handbook of research on managerial thinking in global business economics. IGI Global, Hershey, pp. 520–536
Balcı MA, Batrancea LM, Akgüller Ö, Nichita A (2022) Coarse graining on financial correlation networks. Mathematics 10(12):2118
Batrancea LM (2022) Determinants of economic growth across the European union: a panel data analysis on small and medium enterprises. Sustainability 14(8):4797
Batrancea LM, Balcı MA, Akgüller Ö, Gaban L (2022a) What drives economic growth across European countries? a multimodal approach. Mathematics 10(19):3660
Batrancea LM, Balcı MA, Chermezan L, Akgüller Ö, Masca ES, Gaban L (2022b) Sources of SMEs financing and their impact on economic growth across the European Union: insights from a panel data study spanning sixteen years. Sustainability 14(22):15318
Batrancea LM, Nichita A, Balcı MA, Akgüller Ö (2023a) Empirical investigation on how wellbeing-related infrastructure shapes economic growth: evidence from the European Union regions. PLoS ONE 18(4):e0283277
Batrancea LM, Rathnaswamy MM, Batrancea I (2021) A panel data analysis of economic growth determinants in 34 African countries. J Risk Financ Manag 14(6):260
Batrancea LM, Rathnaswamy MK, Batrancea I (2022c) A panel data analysis on determinants of economic growth in seven non-BCBS countries. J Knowl Econ 13(2):1651–1665
Batrancea LM, Rathnaswamy MM, Rus M-I, Tulai H (2023b) Determinants of economic growth for the last half of century: a panel data analysis on 50 countries. J Knowl Econ 14(3):2578–2602
Behrendt S, Dimpfl T, Peter FJ, Zimmermann DJ (2019) RTransferEntropy-quantifying information flow between different time series using effective transfer entropy. SoftwareX 10:100265
Caporale GM, Gil-Alana L, Plastun A, Makarenko I (2022) Persistence in ESG and conventional stock market indices. J Econ Financ 46(4):678–703
Chan Y, Hogan K, Schwaiger K, Ang A (2020) ESG in factors. J Impact ESG Invest 1(1):26–45
Chi KT, Liu J, Lau FC (2010) A network perspective of the stock market. J Empir Financ 17(4):659–667
Efimova O, Volkov M, Koroleva D (2021) The impact of ESG factors on asset returns: empirical research. Financ Theory Pract 25(4):82–97
Escrig-Olmedo E, Rivera-Lirio JM, Munoz-Torres MJ, Fernandez-Izquierdo MA (2017) Integrating multiple ESG investors preferences into sustainable investment: a fuzzy multicriteria methodological approach. J Clean Prod 162:1334–1345
Ferretti S (2023) On the modeling and simulation of portfolio allocation schemes: an approach based on network community detection. Comput Econ 62(3):969–1005
Giese G, Lee L-E, Melas D, Nagy Z, Nishikawa L (2019) Foundations of ESG investing: how ESG affects equity valuation, risk, and performance. J Portf Manag 45(5):69–83
Girvan M, Newman ME (2002) Community structure in social and biological networks. Proc Natl Acad Sci USA 99(12):7821–7826
Article ADS MathSciNet CAS PubMed PubMed Central Google Scholar
Gong X, Xie F, Zhou Z, Zhang C (2024) The enhanced benefits of ESG in portfolios: a multi-factor model perspective based on LightGBM. Pac Basin Financ J 85:102365
Guo X, Zhang H, Tian T (2018) Development of stock correlation networks using mutual information and financial big data. PLoS ONE 13(4):e0195941
Article PubMed PubMed Central Google Scholar
Hizarci-Payne AK, Kirkulak-Uludag B (2018) Sustainable business practices of Turkish companies listed on the Borsa Istanbul Sustainability Index. In: Moratis L, Melissen F, Idowu S (eds) Sustainable business models. CSR, sustainability, ethics & governance. Springer, Cham, pp. 329–344
Ionescu GH, Firoiu D, Pirvu R, Vilag RD (2019) The impact of ESG factors on market value of companies from travel and tourism industry. Technol Econ Dev Econ 25(5):820–849
Junius D, Adisurjo A, Rijanto YA, Adelina YE (2020) The impact of ESG performance to firm performance and market value. J Aplikasi Akunt 5(1):21–41
Karpman K, Basu S, Easley D, Kim S (2023) Learning financial networks with high-frequency trade data. Data Sci Sci 2(1):2166624
Kartal MT, Kılıç Depren S, Pata UK, Taşkın D, Şavlı T (2024a) Modeling the link between environmental, social, and governance disclosures and scores: the case of publicly traded companies in the Borsa Istanbul Sustainability Index. Financ Innov 10(1):80
Kartal MT, Pata UK, Taşkın D, Ulussever T (2024b) The relationship between monetary policy and financial asset returns in Türkiye: time, frequency, and quantile-based effects. Borsa Istanb Rev 24(3):474–484
Khoojine AS, Feng Z, Shadabfar M, Khoojine NS (2023) Analyzing volatility patterns in the Chinese stock market using partial mutual information-based distances. Eur Phys J B 96(12):165
Article ADS CAS Google Scholar
Kim S, Li Z (2021) Understanding the impact of ESG practices in corporate finance. Sustainability 13(7):3746
Kraskov A, Stögbauer H, Grassberger P (2004) Estimating mutual information. Phys Rev E 69(6):066138
Article ADS MathSciNet Google Scholar
Lee H, Kim JH, Jung HS (2024) Deep-learning-based stock market prediction incorporating ESG sentiment and technical indicators. Sci Rep 14(1):10262
Article ADS CAS PubMed PubMed Central Google Scholar
Lee S-P, Isa M (2023) Environmental, social and governance (ESG) practices and financial performance of shariah-compliant companies in Malaysia. J Islamic Account Bus Res 14(2):295–314
Ling A, Li J, Zhang Y (2023) Can firms with higher ESG ratings bear higher bank systemic tail risk spillover? evidence from Chinese A-share market. Pac Basin Financ J 80:102097
Luo W, Tian Z, Fang X, Deng M (2024) Can good ESG performance reduce stock price crash risk? evidence from Chinese listed companies. Corp Soc Responsib Environ Manag 31(3):1469–1492
Lupu I, Hurduzeu G, Lupu R (2022) How is the ESG reflected in European financial stability? Sustainability 14(16):10287
Ma N (2023) Integration of ESG factors in portfolio management: international trends and practices. Front Bus Econ Manag 12(2):149–152
Article MathSciNet Google Scholar
Massara GP, Di Matteo T, Aste T (2016) Network filtering for big data: triangulated maximally filtered graph. J Complex Netw 5(2):161–178
MathSciNet Google Scholar
Meiden C, Silaban A (2023) Exploring the measurement of environmental performance in alignment with environmental, social, and governance (ESG): a qualitative study. Inf Sci Lett 12(9):2287–2297
Millington T (2022) An investigation into the effects and effectiveness of correlation network filtration methods with financial returns. PLoS ONE 17(9):e0273830
Article CAS PubMed PubMed Central Google Scholar
Naffa H, Fain M (2020) Performance measurement of ESG-themed mega-trend investments in global equity markets using pure factor portfolios methodology. PLoS ONE 15(12):e0244225
Newman ME (2006) Finding community structure in networks using the eigenvectors of matrices. Phys Rev E 74:036104
Article ADS MathSciNet CAS Google Scholar
Nicola G, Cerchiello P, Aste T (2020) Information network modeling for US banking systemic risk. Entropy 22(11):1331
Park SR, Oh K-S (2022) Integration of ESG information into individual investors’ corporate investment decisions: utilizing the UTAUT framework. Front Psychol 13:899480
Park YS, Lee HS (2023) The roles of finance in ESG management. Asia-Pac J Financ Stud 52(3):354–373
Pata UK, Mohammed KS, Serret V, Kartal MT (2024) Assessing the influence of climate risk, carbon allowances, and technological factors on the ESG market in the European Union. Borsa Istanb Rev 24(4):828–837
Pazitka V, Urban M, Wojcik D (2021) Connectivity and growth: financial centres in investment banking networks. Environ Plann A Econ Space 53(7):1789–1809
Raddant M, Kenett DY (2021) Interconnectedness in the global financial market. J Int Money Financ 110:102280
Raza H, Khan MA, Mazliham M, Alam MM, Aman N, Abbas K (2022) Applying artificial intelligence techniques for predicting the environment, social, and governance (ESG) pillar score based on balance sheet and income statement data: a case of non-financial companies of USA, UK, and Germany. Front Environ Sci 10:975487
Shirokikh O, Pastukhov G, Semenov A, Butenko S, Veremyev A, Pasiliao EL, Boginski V (2022) Networks of causal relationships in the US stock market. Depend. Model. 10(1):177–190
Sinha R, Datta M, Ziolo M (2019) Inclusion of ESG factors in investments and value addition: a meta-analysis of the relationship. In: Effective investments on capital markets: 10th Capital Market Effective Investments Conference (CMEI 2018). Springer, pp. 93–109
Stavroglou SK, Pantelous AA, Soramaki K, Zuev K (2017) Causality networks of financial assets. J Netw Theory Financ 3(2):17–67
Stavroglou SK, Pantelous AA, Stanley HE, Zuev KM (2020) Unveiling causal interactions in complex systems. Proc Natl Acad Sci USA 117(14):7599–7605
Stolbov M, Shchepeleva M (2022) The impact of ESG-factors on financial stability. Vopr Econ 11:136–148
Torri G, Giacometti R (2023) Financial contagion in banking networks with community structure. Commun Nonlinear Sci Numer Simul 117:106924
Turiel J, Barucca P, Aste T (2022) Simplicial persistence of financial markets: filtering, generative processes and structural risk. Entropy 24(10):1482
Article ADS MathSciNet PubMed PubMed Central Google Scholar
Upadhyay S, Banerjee A, Panigrahi PK (2020) Causal evolution of global crisis in financial networks. Physica A 554:124690
Viegas E, Goto H, Kobayashi Y, Takayasu M, Takayasu H, Jensen HJ (2020) Allometric scaling of mutual information in complex networks: a conceptual framework and empirical approach. Entropy 22(2):206
Article ADS PubMed PubMed Central Google Scholar
Yadav GS, Guha A, Chakrabarti AS (2020) Measuring complexity in financial data. Front Phys 8:339
Yılmaz MK, Aksoy M, Tatoğlu E (2020) Does the stock market value inclusion in a sustainability index? evidence from Borsa Istanbul. Sustainability 12(2):483
Yoon B, Lee JH, Cho JH (2024) Corporate social responsibility and financial performance: new evidence from the Korean market. SAGE Open 14(2):21582440241255196
Zaccone MC, Pedrini M (2020) ESG factor integration into private equity. Sustainability 12(14):5725
Zhang AY, Zhang JH (2023) Renovation in environmental, social and governance (ESG) research: the application of machine learning. Asian Rev Account. https://doi.org/10.1108/ARA-07-2023-0201
Zhao K, Dong G, Bian D (2023) Detection of illegal transactions of cryptocurrency based on mutual information. Electronics 12(7):1542
Zuhud DAZ, Musa MH, Ismail M, Bahaludin H, Razak FA (2022) The causality and uncertainty of the COVID-19 pandemic to Bursa Malaysia financial services index’s constituents. Entropy 24(8):1100
Download references
Author information
Authors and affiliations.
Department of Business, Babeş-Bolyai University, 400174, Cluj-Napoca, Romania
Larissa M. Batrancea
Department of Mathematics, Muğla Sıtkı Koçman University, 48000, Muğla, Türkiye
Ömer Akgüller & Mehmet Ali Balcı
Faculty of Economics, “1 Decembrie 1918” University of Alba Iulia, 510009, Alba Iulia, Romania
Anca Nichita
You can also search for this author in PubMed Google Scholar
Contributions
All authors contributed to the writing and revision of the paper.
Corresponding author
Correspondence to Larissa M. Batrancea .
Ethics declarations
Competing interests.
The authors declare no competing interests.
Ethical approval
This article does not contain any studies with human participants performed by any of the authors.
Informed consent
As no studies with human participants have been performed for this article, no informed consent was needed.
Additional information
Publisher’s note Springer Nature remains neutral with regard to jurisdictional claims in published maps and institutional affiliations.
Supplementary information
Supplementary material_18.07.24, rights and permissions.
Open Access This article is licensed under a Creative Commons Attribution-NonCommercial-NoDerivatives 4.0 International License, which permits any non-commercial use, sharing, distribution and reproduction in any medium or format, as long as you give appropriate credit to the original author(s) and the source, provide a link to the Creative Commons licence, and indicate if you modified the licensed material. You do not have permission under this licence to share adapted material derived from this article or parts of it. The images or other third party material in this article are included in the article’s Creative Commons licence, unless indicated otherwise in a credit line to the material. If material is not included in the article’s Creative Commons licence and your intended use is not permitted by statutory regulation or exceeds the permitted use, you will need to obtain permission directly from the copyright holder. To view a copy of this licence, visit http://creativecommons.org/licenses/by-nc-nd/4.0/ .
Reprints and permissions
About this article
Cite this article.
Batrancea, L.M., Akgüller, Ö., Balcı, M.A. et al. Financial network communities and methodological insights: a case study for Borsa Istanbul Sustainability Index. Humanit Soc Sci Commun 11 , 1046 (2024). https://doi.org/10.1057/s41599-024-03527-y
Download citation
Received : 24 February 2024
Accepted : 24 July 2024
Published : 15 August 2024
DOI : https://doi.org/10.1057/s41599-024-03527-y
Share this article
Anyone you share the following link with will be able to read this content:
Sorry, a shareable link is not currently available for this article.
Provided by the Springer Nature SharedIt content-sharing initiative
Quick links
- Explore articles by subject
- Guide to authors
- Editorial policies


IMAGES
COMMENTS
Forgiving Medical Debt Won't Make Everyone Happier. by Rachel Layne. Medical debt not only hurts credit access, it can also harm one's mental health. But a study by Raymond Kluender finds that forgiving people's bills—even $170 million of debt—doesn't necessarily reduce stress, financial or otherwise. 18 Jun 2024.
More than half of U.S. states, for example, have added personal finance instruction as a high school graduation requirement. Universities, including Stanford, are now offering personal finance courses. Employers, too, are recognizing that financial anxiety hurts employee productivity and are sponsoring personal finance lessons in the workplace.
250 Case Study 12: Susan Wood Case Study Facts Today is December 31, 2018. Susan Wood has come to you, a CFP® professional, for help in developing a plan to accomplish her financial goals. From your initial meeting together, you have gathered the following information. PERSONAL BACKGROUND AND INFORMATION Susan Wood (Age 50)
NGPF Case Studies. Case Studies present personal finance issues in the context of real-life situations with all their ambiguities. Students will explore decision-making, develop communication skills, and make choices when there is no "right" answer. To help get you started, NGPF has created support guides to walk you through how to complete ...
Personal Finance Case Study. Regardless of whether you are able to participate in the Governor's Challenge, we hope you will review the issues raised in the case study and consider using them in the classroom as group presentations and breakouts. Doing so will provide a good opportunity to apply many of the personal finance concepts and ...
A case study on personal financial planning at Iowa State University, exploring various aspects and providing insights.
We™ll work through a case study of student budgeting to illustrate the -rst couple of rules. We™ll -nish with a review of interest rate math. John Y. Campbell (2019) Introduction to Personal Finance Personal Finance Workshop 3 / 39 ... John Y. Campbell (2019) Introduction to Personal Finance Personal Finance Workshop 11 / 39. Financial ...
Forgiving Medical Debt Won't Make Everyone Happier. by Rachel Layne. Medical debt not only hurts credit access, it can also harm one's mental health. But a study by Raymond Kluender finds that forgiving people's bills—even $170 million of debt—doesn't necessarily reduce stress, financial or otherwise. 16 Jul 2024.
This study investigates the perspectives and impact that personal finance education had on participants in Western Pennsylvania. The researchers begin with a literature review of personal finance courses in the United States (U.S.). The U.S. housing market collapse is also discussed as a key component of the financial crisis that is often overlooked and can be partly attributed to the lack of ...
Jane King, Mary Carey. OUP Oxford, 2014 - Business & Economics- 339 pages. This new title makes the principles of personal financial planning clear and accessible, using engaging case studies and extensive examples to introduce students from a variety of disciplines to an essential set of skills. Encouraging students to take control of their ...
A released Personal Finance Challenge case study is a great culminating project to use in your classroom at the end of the school year. Group students into teams of four, give them Monday-Wednesday to prepare and then have the presentations on Thursday & Friday in your classroom. You can be the judge or invite other teachers, administration, or ...
The core components of a case are a summary of the company's background, analysis of their background, the company's internal strengths and weaknesses, their opportunities and threats, the external environment the they compete in, an evaluation of your SWOT analysis, and some recommendations to remedy potential issues you find.
Finder's research team sometimes needs case studies who have had positive or negative experiences with their personal finances to accompany our research in order to help bring it to life. We are therefore building a database of case studies who would be willing to share their personal finance stories with us.
Behavioral finance replaces the traditional and idealized idea of rational decision makers with real and imperfect people who have social, cognitive, and emotional biases. The resulting inefficiencies in the capital markets can create opportunities for investment managers and firms. Closed for comment; 0 Comments. 1.
A Handbook of Case Studies in Finance 5 research development projects are worth the funding of cash through the firm's capitalization structure (debt, equity or retained earnings). It is the process of allocating resources for major capital, investment or expenditures.
ple Case Study & RubricSample CASE STUDY ANALYSIS Rules:Each team is provided with incomplete financial i. formation about a fictional family's financial situation. You are not given all information necessary and are free to. eate additional information about your family if you wish.Your team is charged to study this family's finances and.
Template 2: Case Study for Financial Management PowerPoint Template. Introducing our captivating case study template designed to provide an environment conducive to productive discussions and effective decision-making. This template is perfect for showcasing real-life examples and analyzing financial management scenarios visually engagingly.
Study with Quizlet and memorize flashcards containing terms like When revising a budget, it is important to make choices that allow you to continue ____________ money., Buying a new car can create a financial challenge because, To revise a monthly budget, changes in which categories might need to be addressed? Check all that apply. and more.
Study with Quizlet and memorize flashcards containing terms like You have a new goal of saving at least $4,500 over the course of the next year. You already have $900 saved. By how much would you need to increase your monthly net savings in order to meet this goal? $100 $150 $200 $250, Open this link to read more about how credit card interest works. Use this information to calculate the cost ...
Case Study: Personal Financial Planning Assignment 100%. 4.9 (78 reviews) Flashcards; Learn; Test; Match; Q-Chat; Get a hint. ... "Personal Financial Planning" YAP Consumer Education Quizzes + Unit 4 Test. Teacher 37 terms. thegravelgobbler. Preview. chapter 6. 19 terms. emstarlyn. Preview. 4-2:Investing basics .
Answer: (a) Personal cash flow statement for Yana for the 2019 financial year: Income: Salary Employer superannuation 5,088 60,088 Expenditure: Taxation Personal superannuation3,850 Health cover 1,650 Union fees 1,100 Household Mortgage payments Credit card payments Entertainment 52,600 Net income (b) Personal balance sheet for Yana at the end ...
the up-front cost. Study with Quizlet and memorize flashcards containing terms like When making a decision about housing, the first step should be, When revising a budget, it is important to make choices that allow you to continue _________ money., When planning a budget, the biggest consideration should be the and more.
Free Budgeting Case Studies from the NGPF Curriculum for your high school classroom. Math; Arcade; Curriculum . Units. Resources & answer keys: All Units; ... MISSION: By 2030, all U.S. high schoolers will take at least one-semester course in Personal Finance before graduation. Promote personal finance courses at your school:
This study investigates the influence of Environmental, Social, and Governance (ESG) scores on the clustering and community formation of companies within various network models. Using daily ...
A drug used in the last global mpox outbreak in 2022-23 is not effective against the more severe virus spreading rapidly in Africa, researchers have found. The antiviral, tecovirimat, did not reduce the duration of lesions among children and adults with clade I mpox in the Democratic Republic of the Congo (DRC), according to the initial results of a placebo-controlled trial run by researchers ...
Study with Quizlet and memorize flashcards containing terms like You have a new goal of saving at least $4,500 over the course of the next year. You already have $900 saved. By how much would you need to increase your monthly net savings in order to meet this goal? A. $100 B. $150 C. $200 D. $250, Open this link to read more about how credit card interest works. Use this information to ...