Longitudinal Study Design
Julia Simkus
Editor at Simply Psychology
BA (Hons) Psychology, Princeton University
Julia Simkus is a graduate of Princeton University with a Bachelor of Arts in Psychology. She is currently studying for a Master's Degree in Counseling for Mental Health and Wellness in September 2023. Julia's research has been published in peer reviewed journals.
Learn about our Editorial Process
Saul McLeod, PhD
Editor-in-Chief for Simply Psychology
BSc (Hons) Psychology, MRes, PhD, University of Manchester
Saul McLeod, PhD., is a qualified psychology teacher with over 18 years of experience in further and higher education. He has been published in peer-reviewed journals, including the Journal of Clinical Psychology.
Olivia Guy-Evans, MSc
Associate Editor for Simply Psychology
BSc (Hons) Psychology, MSc Psychology of Education
Olivia Guy-Evans is a writer and associate editor for Simply Psychology. She has previously worked in healthcare and educational sectors.
A longitudinal study is a type of observational and correlational study that involves monitoring a population over an extended period of time. It allows researchers to track changes and developments in the subjects over time.

What is a Longitudinal Study?
In longitudinal studies, researchers do not manipulate any variables or interfere with the environment. Instead, they simply conduct observations on the same group of subjects over a period of time.
These research studies can last as short as a week or as long as multiple years or even decades. Unlike cross-sectional studies that measure a moment in time, longitudinal studies last beyond a single moment, enabling researchers to discover cause-and-effect relationships between variables.
They are beneficial for recognizing any changes, developments, or patterns in the characteristics of a target population. Longitudinal studies are often used in clinical and developmental psychology to study shifts in behaviors, thoughts, emotions, and trends throughout a lifetime.
For example, a longitudinal study could be used to examine the progress and well-being of children at critical age periods from birth to adulthood.
The Harvard Study of Adult Development is one of the longest longitudinal studies to date. Researchers in this study have followed the same men group for over 80 years, observing psychosocial variables and biological processes for healthy aging and well-being in late life (see Harvard Second Generation Study).
When designing longitudinal studies, researchers must consider issues like sample selection and generalizability, attrition and selectivity bias, effects of repeated exposure to measures, selection of appropriate statistical models, and coverage of the necessary timespan to capture the phenomena of interest.
Panel Study
- A panel study is a type of longitudinal study design in which the same set of participants are measured repeatedly over time.
- Data is gathered on the same variables of interest at each time point using consistent methods. This allows studying continuity and changes within individuals over time on the key measured constructs.
- Prominent examples include national panel surveys on topics like health, aging, employment, and economics. Panel studies are a type of prospective study .
Cohort Study
- A cohort study is a type of longitudinal study that samples a group of people sharing a common experience or demographic trait within a defined period, such as year of birth.
- Researchers observe a population based on the shared experience of a specific event, such as birth, geographic location, or historical experience. These studies are typically used among medical researchers.
- Cohorts are identified and selected at a starting point (e.g. birth, starting school, entering a job field) and followed forward in time.
- As they age, data is collected on cohort subgroups to determine their differing trajectories. For example, investigating how health outcomes diverge for groups born in 1950s, 1960s, and 1970s.
- Cohort studies do not require the same individuals to be assessed over time; they just require representation from the cohort.
Retrospective Study
- In a retrospective study , researchers either collect data on events that have already occurred or use existing data that already exists in databases, medical records, or interviews to gain insights about a population.
- Appropriate when prospectively following participants from the past starting point is infeasible or unethical. For example, studying early origins of diseases emerging later in life.
- Retrospective studies efficiently provide a “snapshot summary” of the past in relation to present status. However, quality concerns with retrospective data make careful interpretation necessary when inferring causality. Memory biases and selective retention influence quality of retrospective data.
Allows researchers to look at changes over time
Because longitudinal studies observe variables over extended periods of time, researchers can use their data to study developmental shifts and understand how certain things change as we age.
High validation
Since objectives and rules for long-term studies are established before data collection, these studies are authentic and have high levels of validity.
Eliminates recall bias
Recall bias occurs when participants do not remember past events accurately or omit details from previous experiences.
Flexibility
The variables in longitudinal studies can change throughout the study. Even if the study was created to study a specific pattern or characteristic, the data collection could show new data points or relationships that are unique and worth investigating further.
Limitations
Costly and time-consuming.
Longitudinal studies can take months or years to complete, rendering them expensive and time-consuming. Because of this, researchers tend to have difficulty recruiting participants, leading to smaller sample sizes.
Large sample size needed
Longitudinal studies tend to be challenging to conduct because large samples are needed for any relationships or patterns to be meaningful. Researchers are unable to generate results if there is not enough data.
Participants tend to drop out
Not only is it a struggle to recruit participants, but subjects also tend to leave or drop out of the study due to various reasons such as illness, relocation, or a lack of motivation to complete the full study.
This tendency is known as selective attrition and can threaten the validity of an experiment. For this reason, researchers using this approach typically recruit many participants, expecting a substantial number to drop out before the end.
Report bias is possible
Longitudinal studies will sometimes rely on surveys and questionnaires, which could result in inaccurate reporting as there is no way to verify the information presented.
- Data were collected for each child at three-time points: at 11 months after adoption, at 4.5 years of age and at 10.5 years of age. The first two sets of results showed that the adoptees were behind the non-institutionalised group however by 10.5 years old there was no difference between the two groups. The Romanian orphans had caught up with the children raised in normal Canadian families.
- The role of positive psychology constructs in predicting mental health and academic achievement in children and adolescents (Marques Pais-Ribeiro, & Lopez, 2011)
- The correlation between dieting behavior and the development of bulimia nervosa (Stice et al., 1998)
- The stress of educational bottlenecks negatively impacting students’ wellbeing (Cruwys, Greenaway, & Haslam, 2015)
- The effects of job insecurity on psychological health and withdrawal (Sidney & Schaufeli, 1995)
- The relationship between loneliness, health, and mortality in adults aged 50 years and over (Luo et al., 2012)
- The influence of parental attachment and parental control on early onset of alcohol consumption in adolescence (Van der Vorst et al., 2006)
- The relationship between religion and health outcomes in medical rehabilitation patients (Fitchett et al., 1999)
Goals of Longitudinal Data and Longitudinal Research
The objectives of longitudinal data collection and research as outlined by Baltes and Nesselroade (1979):
- Identify intraindividual change : Examine changes at the individual level over time, including long-term trends or short-term fluctuations. Requires multiple measurements and individual-level analysis.
- Identify interindividual differences in intraindividual change : Evaluate whether changes vary across individuals and relate that to other variables. Requires repeated measures for multiple individuals plus relevant covariates.
- Analyze interrelationships in change : Study how two or more processes unfold and influence each other over time. Requires longitudinal data on multiple variables and appropriate statistical models.
- Analyze causes of intraindividual change: This objective refers to identifying factors or mechanisms that explain changes within individuals over time. For example, a researcher might want to understand what drives a person’s mood fluctuations over days or weeks. Or what leads to systematic gains or losses in one’s cognitive abilities across the lifespan.
- Analyze causes of interindividual differences in intraindividual change : Identify mechanisms that explain within-person changes and differences in changes across people. Requires repeated data on outcomes and covariates for multiple individuals plus dynamic statistical models.
How to Perform a Longitudinal Study
When beginning to develop your longitudinal study, you must first decide if you want to collect your own data or use data that has already been gathered.
Using already collected data will save you time, but it will be more restricted and limited than collecting it yourself. When collecting your own data, you can choose to conduct either a retrospective or prospective study .
In a retrospective study, you are collecting data on events that have already occurred. You can examine historical information, such as medical records, in order to understand the past. In a prospective study, on the other hand, you are collecting data in real-time. Prospective studies are more common for psychology research.
Once you determine the type of longitudinal study you will conduct, you then must determine how, when, where, and on whom the data will be collected.
A standardized study design is vital for efficiently measuring a population. Once a study design is created, researchers must maintain the same study procedures over time to uphold the validity of the observation.
A schedule should be maintained, complete results should be recorded with each observation, and observer variability should be minimized.
Researchers must observe each subject under the same conditions to compare them. In this type of study design, each subject is the control.
Methodological Considerations
Important methodological considerations include testing measurement invariance of constructs across time, appropriately handling missing data, and using accelerated longitudinal designs that sample different age cohorts over overlapping time periods.
Testing measurement invariance
Testing measurement invariance involves evaluating whether the same construct is being measured in a consistent, comparable way across multiple time points in longitudinal research.
This includes assessing configural, metric, and scalar invariance through confirmatory factor analytic approaches. Ensuring invariance gives more confidence when drawing inferences about change over time.
Missing data
Missing data can occur during initial sampling if certain groups are underrepresented or fail to respond.
Attrition over time is the main source – participants dropping out for various reasons. The consequences of missing data are reduced statistical power and potential bias if dropout is nonrandom.
Handling missing data appropriately in longitudinal studies is critical to reducing bias and maintaining power.
It is important to minimize attrition by tracking participants, keeping contact info up to date, engaging them, and providing incentives over time.
Techniques like maximum likelihood estimation and multiple imputation are better alternatives to older methods like listwise deletion. Assumptions about missing data mechanisms (e.g., missing at random) shape the analytic approaches taken.
Accelerated longitudinal designs
Accelerated longitudinal designs purposefully create missing data across age groups.
Accelerated longitudinal designs strategically sample different age cohorts at overlapping periods. For example, assessing 6th, 7th, and 8th graders at yearly intervals would cover 6-8th grade development over a 3-year study rather than following a single cohort over that timespan.
This increases the speed and cost-efficiency of longitudinal data collection and enables the examination of age/cohort effects. Appropriate multilevel statistical models are required to analyze the resulting complex data structure.
In addition to those considerations, optimizing the time lags between measurements, maximizing participant retention, and thoughtfully selecting analysis models that align with the research questions and hypotheses are also vital in ensuring robust longitudinal research.
So, careful methodology is key throughout the design and analysis process when working with repeated-measures data.
Cohort effects
A cohort refers to a group born in the same year or time period. Cohort effects occur when different cohorts show differing trajectories over time.
Cohort effects can bias results if not accounted for, especially in accelerated longitudinal designs which assume cohort equivalence.
Detecting cohort effects is important but can be challenging as they are confounded with age and time of measurement effects.
Cohort effects can also interfere with estimating other effects like retest effects. This happens because comparing groups to estimate retest effects relies on cohort equivalence.
Overall, researchers need to test for and control cohort effects which could otherwise lead to invalid conclusions. Careful study design and analysis is required.
Retest effects
Retest effects refer to gains in performance that occur when the same or similar test is administered on multiple occasions.
For example, familiarity with test items and procedures may allow participants to improve their scores over repeated testing above and beyond any true change.
Specific examples include:
- Memory tests – Learning which items tend to be tested can artificially boost performance over time
- Cognitive tests – Becoming familiar with the testing format and particular test demands can inflate scores
- Survey measures – Remembering previous responses can bias future responses over multiple administrations
- Interviews – Comfort with the interviewer and process can lead to increased openness or recall
To estimate retest effects, performance of retested groups is compared to groups taking the test for the first time. Any divergence suggests inflated scores due to retesting rather than true change.
If unchecked in analysis, retest gains can be confused with genuine intraindividual change or interindividual differences.
This undermines the validity of longitudinal findings. Thus, testing and controlling for retest effects are important considerations in longitudinal research.
Data Analysis
Longitudinal data involves repeated assessments of variables over time, allowing researchers to study stability and change. A variety of statistical models can be used to analyze longitudinal data, including latent growth curve models, multilevel models, latent state-trait models, and more.
Latent growth curve models allow researchers to model intraindividual change over time. For example, one could estimate parameters related to individuals’ baseline levels on some measure, linear or nonlinear trajectory of change over time, and variability around those growth parameters. These models require multiple waves of longitudinal data to estimate.
Multilevel models are useful for hierarchically structured longitudinal data, with lower-level observations (e.g., repeated measures) nested within higher-level units (e.g., individuals). They can model variability both within and between individuals over time.
Latent state-trait models decompose the covariance between longitudinal measurements into time-invariant trait factors, time-specific state residuals, and error variance. This allows separating stable between-person differences from within-person fluctuations.
There are many other techniques like latent transition analysis, event history analysis, and time series models that have specialized uses for particular research questions with longitudinal data. The choice of model depends on the hypotheses, timescale of measurements, age range covered, and other factors.
In general, these various statistical models allow investigation of important questions about developmental processes, change and stability over time, causal sequencing, and both between- and within-person sources of variability. However, researchers must carefully consider the assumptions behind the models they choose.
Longitudinal vs. Cross-Sectional Studies
Longitudinal studies and cross-sectional studies are two different observational study designs where researchers analyze a target population without manipulating or altering the natural environment in which the participants exist.
Yet, there are apparent differences between these two forms of study. One key difference is that longitudinal studies follow the same sample of people over an extended period of time, while cross-sectional studies look at the characteristics of different populations at a given moment in time.
Longitudinal studies tend to require more time and resources, but they can be used to detect cause-and-effect relationships and establish patterns among subjects.
On the other hand, cross-sectional studies tend to be cheaper and quicker but can only provide a snapshot of a point in time and thus cannot identify cause-and-effect relationships.
Both studies are valuable for psychologists to observe a given group of subjects. Still, cross-sectional studies are more beneficial for establishing associations between variables, while longitudinal studies are necessary for examining a sequence of events.
1. Are longitudinal studies qualitative or quantitative?
Longitudinal studies are typically quantitative. They collect numerical data from the same subjects to track changes and identify trends or patterns.
However, they can also include qualitative elements, such as interviews or observations, to provide a more in-depth understanding of the studied phenomena.
2. What’s the difference between a longitudinal and case-control study?
Case-control studies compare groups retrospectively and cannot be used to calculate relative risk. Longitudinal studies, though, can compare groups either retrospectively or prospectively.
In case-control studies, researchers study one group of people who have developed a particular condition and compare them to a sample without the disease.
Case-control studies look at a single subject or a single case, whereas longitudinal studies are conducted on a large group of subjects.
3. Does a longitudinal study have a control group?
Yes, a longitudinal study can have a control group . In such a design, one group (the experimental group) would receive treatment or intervention, while the other group (the control group) would not.
Both groups would then be observed over time to see if there are differences in outcomes, which could suggest an effect of the treatment or intervention.
However, not all longitudinal studies have a control group, especially observational ones and not testing a specific intervention.
Baltes, P. B., & Nesselroade, J. R. (1979). History and rationale of longitudinal research. In J. R. Nesselroade & P. B. Baltes (Eds.), (pp. 1–39). Academic Press.
Cook, N. R., & Ware, J. H. (1983). Design and analysis methods for longitudinal research. Annual review of public health , 4, 1–23.
Fitchett, G., Rybarczyk, B., Demarco, G., & Nicholas, J.J. (1999). The role of religion in medical rehabilitation outcomes: A longitudinal study. Rehabilitation Psychology, 44, 333-353.
Harvard Second Generation Study. (n.d.). Harvard Second Generation Grant and Glueck Study. Harvard Study of Adult Development. Retrieved from https://www.adultdevelopmentstudy.org.
Le Mare, L., & Audet, K. (2006). A longitudinal study of the physical growth and health of postinstitutionalized Romanian adoptees. Pediatrics & child health, 11 (2), 85-91.
Luo, Y., Hawkley, L. C., Waite, L. J., & Cacioppo, J. T. (2012). Loneliness, health, and mortality in old age: a national longitudinal study. Social science & medicine (1982), 74 (6), 907–914.
Marques, S. C., Pais-Ribeiro, J. L., & Lopez, S. J. (2011). The role of positive psychology constructs in predicting mental health and academic achievement in children and adolescents: A two-year longitudinal study. Journal of Happiness Studies: An Interdisciplinary Forum on Subjective Well-Being, 12( 6), 1049–1062.
Sidney W.A. Dekker & Wilmar B. Schaufeli (1995) The effects of job insecurity on psychological health and withdrawal: A longitudinal study, Australian Psychologist, 30: 1,57-63.
Stice, E., Mazotti, L., Krebs, M., & Martin, S. (1998). Predictors of adolescent dieting behaviors: A longitudinal study. Psychology of Addictive Behaviors, 12 (3), 195–205.
Tegan Cruwys, Katharine H Greenaway & S Alexander Haslam (2015) The Stress of Passing Through an Educational Bottleneck: A Longitudinal Study of Psychology Honours Students, Australian Psychologist, 50:5, 372-381.
Thomas, L. (2020). What is a longitudinal study? Scribbr. Retrieved from https://www.scribbr.com/methodology/longitudinal-study/
Van der Vorst, H., Engels, R. C. M. E., Meeus, W., & Deković, M. (2006). Parental attachment, parental control, and early development of alcohol use: A longitudinal study. Psychology of Addictive Behaviors, 20 (2), 107–116.
Further Information
- Schaie, K. W. (2005). What can we learn from longitudinal studies of adult development?. Research in human development, 2 (3), 133-158.
- Caruana, E. J., Roman, M., Hernández-Sánchez, J., & Solli, P. (2015). Longitudinal studies. Journal of thoracic disease, 7 (11), E537.

- Bipolar Disorder
- Therapy Center
- When To See a Therapist
- Types of Therapy
- Best Online Therapy
- Best Couples Therapy
- Best Family Therapy
- Managing Stress
- Sleep and Dreaming
- Understanding Emotions
- Self-Improvement
- Healthy Relationships
- Student Resources
- Personality Types
- Sweepstakes
- Guided Meditations
- Verywell Mind Insights
- 2024 Verywell Mind 25
- Mental Health in the Classroom
- Editorial Process
- Meet Our Review Board
- Crisis Support
What Is a Longitudinal Study?
Tracking Variables Over Time
Steve McAlister / The Image Bank / Getty Images
The Typical Longitudinal Study
Potential pitfalls, frequently asked questions.
A longitudinal study follows what happens to selected variables over an extended time. Psychologists use the longitudinal study design to explore possible relationships among variables in the same group of individuals over an extended period.
Once researchers have determined the study's scope, participants, and procedures, most longitudinal studies begin with baseline data collection. In the days, months, years, or even decades that follow, they continually gather more information so they can observe how variables change over time relative to the baseline.
For example, imagine that researchers are interested in the mental health benefits of exercise in middle age and how exercise affects cognitive health as people age. The researchers hypothesize that people who are more physically fit in their 40s and 50s will be less likely to experience cognitive declines in their 70s and 80s.
Longitudinal vs. Cross-Sectional Studies
Longitudinal studies, a type of correlational research , are usually observational, in contrast with cross-sectional research . Longitudinal research involves collecting data over an extended time, whereas cross-sectional research involves collecting data at a single point.
To test this hypothesis, the researchers recruit participants who are in their mid-40s to early 50s. They collect data related to current physical fitness, exercise habits, and performance on cognitive function tests. The researchers continue to track activity levels and test results for a certain number of years, look for trends in and relationships among the studied variables, and test the data against their hypothesis to form a conclusion.
Examples of Early Longitudinal Study Design
Examples of longitudinal studies extend back to the 17th century, when King Louis XIV periodically gathered information from his Canadian subjects, including their ages, marital statuses, occupations, and assets such as livestock and land. He used the data to spot trends over the years and understand his colonies' health and economic viability.
In the 18th century, Count Philibert Gueneau de Montbeillard conducted the first recorded longitudinal study when he measured his son every six months and published the information in "Histoire Naturelle."
The Genetic Studies of Genius (also known as the Terman Study of the Gifted), which began in 1921, is one of the first studies to follow participants from childhood into adulthood. Psychologist Lewis Terman's goal was to examine the similarities among gifted children and disprove the common assumption at the time that gifted children were "socially inept."
Types of Longitudinal Studies
Longitudinal studies fall into three main categories.
- Panel study : Sampling of a cross-section of individuals
- Cohort study : Sampling of a group based on a specific event, such as birth, geographic location, or experience
- Retrospective study : Review of historical information such as medical records
Benefits of Longitudinal Research
A longitudinal study can provide valuable insight that other studies can't. They're particularly useful when studying developmental and lifespan issues because they allow glimpses into changes and possible reasons for them.
For example, some longitudinal studies have explored differences and similarities among identical twins, some reared together and some apart. In these types of studies, researchers tracked participants from childhood into adulthood to see how environment influences personality , achievement, and other areas.
Because the participants share the same genetics , researchers chalked up any differences to environmental factors . Researchers can then look at what the participants have in common and where they differ to see which characteristics are more strongly influenced by either genetics or experience. Note that adoption agencies no longer separate twins, so such studies are unlikely today. Longitudinal studies on twins have shifted to those within the same household.
As with other types of psychology research, researchers must take into account some common challenges when considering, designing, and performing a longitudinal study.
Longitudinal studies require time and are often quite expensive. Because of this, these studies often have only a small group of subjects, which makes it difficult to apply the results to a larger population.
Selective Attrition
Participants sometimes drop out of a study for any number of reasons, like moving away from the area, illness, or simply losing motivation . This tendency, known as selective attrition , shrinks the sample size and decreases the amount of data collected.
If the final group no longer reflects the original representative sample , attrition can threaten the validity of the experiment. Validity refers to whether or not a test or experiment accurately measures what it claims to measure. If the final group of participants doesn't represent the larger group accurately, generalizing the study's conclusions is difficult.
The World’s Longest-Running Longitudinal Study
Lewis Terman aimed to investigate how highly intelligent children develop into adulthood with his "Genetic Studies of Genius." Results from this study were still being compiled into the 2000s. However, Terman was a proponent of eugenics and has been accused of letting his own sexism , racism , and economic prejudice influence his study and of drawing major conclusions from weak evidence. However, Terman's study remains influential in longitudinal studies. For example, a recent study found new information on the original Terman sample, which indicated that men who skipped a grade as children went on to have higher incomes than those who didn't.
A Word From Verywell
Longitudinal studies can provide a wealth of valuable information that would be difficult to gather any other way. Despite the typical expense and time involved, longitudinal studies from the past continue to influence and inspire researchers and students today.
A longitudinal study follows up with the same sample (i.e., group of people) over time, whereas a cross-sectional study examines one sample at a single point in time, like a snapshot.
A longitudinal study can occur over any length of time, from a few weeks to a few decades or even longer.
That depends on what researchers are investigating. A researcher can measure data on just one participant or thousands over time. The larger the sample size, of course, the more likely the study is to yield results that can be extrapolated.
Piccinin AM, Knight JE. History of longitudinal studies of psychological aging . Encyclopedia of Geropsychology. 2017:1103-1109. doi:10.1007/978-981-287-082-7_103
Terman L. Study of the gifted . In: The SAGE Encyclopedia of Educational Research, Measurement, and Evaluation. 2018. doi:10.4135/9781506326139.n691
Sahu M, Prasuna JG. Twin studies: A unique epidemiological tool . Indian J Community Med . 2016;41(3):177-182. doi:10.4103/0970-0218.183593
Almqvist C, Lichtenstein P. Pediatric twin studies . In: Twin Research for Everyone . Elsevier; 2022:431-438.
Warne RT. An evaluation (and vindication?) of Lewis Terman: What the father of gifted education can teach the 21st century . Gifted Child Q. 2018;63(1):3-21. doi:10.1177/0016986218799433
Warne RT, Liu JK. Income differences among grade skippers and non-grade skippers across genders in the Terman sample, 1936–1976 . Learning and Instruction. 2017;47:1-12. doi:10.1016/j.learninstruc.2016.10.004
Wang X, Cheng Z. Cross-sectional studies: Strengths, weaknesses, and recommendations . Chest . 2020;158(1S):S65-S71. doi:10.1016/j.chest.2020.03.012
Caruana EJ, Roman M, Hernández-Sánchez J, Solli P. Longitudinal studies . J Thorac Dis . 2015;7(11):E537-E540. doi:10.3978/j.issn.2072-1439.2015.10.63
By Kendra Cherry, MSEd Kendra Cherry, MS, is a psychosocial rehabilitation specialist, psychology educator, and author of the "Everything Psychology Book."
- Skip to secondary menu
- Skip to main content
- Skip to primary sidebar
Statistics By Jim
Making statistics intuitive
Longitudinal Study: Overview, Examples & Benefits
By Jim Frost Leave a Comment
What is a Longitudinal Study?
A longitudinal study is an experimental design that takes repeated measurements of the same subjects over time. These studies can span years or even decades. Unlike cross-sectional studies , which analyze data at a single point, longitudinal studies track changes and developments, producing a more dynamic assessment.
A cohort study is a specific type of longitudinal study focusing on a group of people sharing a common characteristic or experience within a defined period.
Imagine tracking a group of individuals over time. Researchers collect data regularly, analyzing how specific factors evolve or influence outcomes. This method offers a dynamic view of trends and changes.

Consider a study tracking 100 high school students’ academic performances annually for ten years. Researchers observe how various factors like teaching methods, family background, and personal habits impact their academic growth over time.
Researchers frequently use longitudinal studies in the following fields:
- Psychology: Understanding behavioral changes.
- Sociology: Observing societal trends.
- Medicine: Tracking disease progression.
- Education: Assessing long-term educational outcomes.
Learn more about Experimental Designs: Definition and Types .
Duration of Longitudinal Studies
Typically, the objectives dictate how long researchers run a longitudinal study. Studies focusing on rapid developmental phases, like early childhood, might last a few years. On the other hand, exploring long-term trends, like aging, can span decades. The key is to align the duration with the research goals.
Implementing a Longitudinal Study: Your Options
When planning a longitudinal study, you face a crucial decision: gather new data or use existing datasets.
Option 1: Utilizing Existing Data
Governments and research centers often share data from their longitudinal studies. For instance, the U.S. National Longitudinal Surveys (NLS) has been tracking thousands of Americans since 1979, offering a wealth of data accessible through the Bureau of Labor Statistics .
This type of data is usually reliable, offering insights over extended periods. However, it’s less flexible than the data that the researchers can collect themselves. Often, details are aggregated to protect privacy, limiting analysis to broader regions. Additionally, the original study’s variables restrict you, and you can’t tailor data collection to meet your study’s needs.
If you opt for existing data, scrutinize the dataset’s origin and the available information.
Option 2: Collecting Data Yourself
If you decide to gather your own data, your approach depends on the study type: retrospective or prospective.
A retrospective longitudinal study focuses on past events. This type is generally quicker and less costly but more prone to errors.
The prospective form of this study tracks a subject group over time, collecting data as events unfold. This approach allows the researchers to choose the variables they’ll measure and how they’ll measure them. Usually, these studies produce the best data but are more expensive.
While retrospective studies save time and money, prospective studies, though more resource-intensive, offer greater accuracy.
Learn more about Retrospective and Prospective Studies .
Advantages of a Longitudinal Study
Longitudinal studies can provide insight into developmental phases and long-term changes, which cross-sectional studies might miss.
These studies can help you determine the sequence of events. By taking multiple observations of the same individuals over time, you can attribute changes to the other variables rather than differences between subjects. This benefit of having the subjects be their own controls is one that applies to all within-subjects studies, also known as repeated measures design. Learn more about Repeated Measures Designs .
Consider a longitudinal study examining the influence of a consistent reading program on children’s literacy development. In a longitudinal framework, factors like innate linguistic ability, which typically don’t fluctuate significantly, are inherently accounted for by using the same group of students over time. This approach allows for a more precise assessment of the reading program’s direct impact over the study’s duration.
Collectively, these benefits help you establish causal relationships. Consequently, longitudinal studies excel in revealing how variables change over time and identifying potential causal relationships .
Disadvantages of a Longitudinal Study
A longitudinal study can be time-consuming and expensive, given its extended duration.
For example, a 30-year study on the aging process may require substantial funding for decades and a long-term commitment from researchers and staff.
Over time, participants may selectively drop out, potentially skewing results and reducing the study’s effectiveness.
For instance, in a study examining the long-term effects of a new fitness regimen, more physically fit participants might be less likely to drop out than those finding the regimen challenging. This scenario potentially skews the results to exaggerate the program’s effectiveness.
Maintaining consistent data collection methods and standards over a long period can be challenging.
For example, a longitudinal study that began using face-to-face interviews might face consistency issues if it later shifts to online surveys, potentially affecting the quality and comparability of the responses.
In conclusion, longitudinal studies are powerful tools for understanding changes over time. While they come with challenges, their ability to uncover trends and causal relationships makes them invaluable in many fields. As with any research method, understanding their strengths and limitations is critical to effectively utilizing their potential.
Newman AB. An overview of the design, implementation, and analyses of longitudinal studies on aging . J Am Geriatr Soc. 2010 Oct;58 Suppl 2:S287-91. doi: 10.1111/j.1532-5415.2010.02916.x. PMID: 21029055; PMCID: PMC3008590.
Share this:

Reader Interactions
Comments and questions cancel reply.

Longitudinal Studies: Methods, Benefits and Challenges

Introduction
What is a longitudinal study, what are examples of longitudinal studies, longitudinal studies vs. cross-sectional studies, benefits of longitudinal studies, types of longitudinal studies, how do you conduct a longitudinal study, challenges of longitudinal research.
Longitudinal research refers to any study that collects the same sample of data from the same group of people at different points in time. While time-consuming and potentially costly in terms of resources and effort, a longitudinal study has enormous utility in understanding complex phenomena that might change as time passes.
In this article, we will explore the nature and importance of longitudinal studies to allow you to decide whether your research inquiry warrants a longitudinal inquiry or if a cross-sectional study is more appropriate.
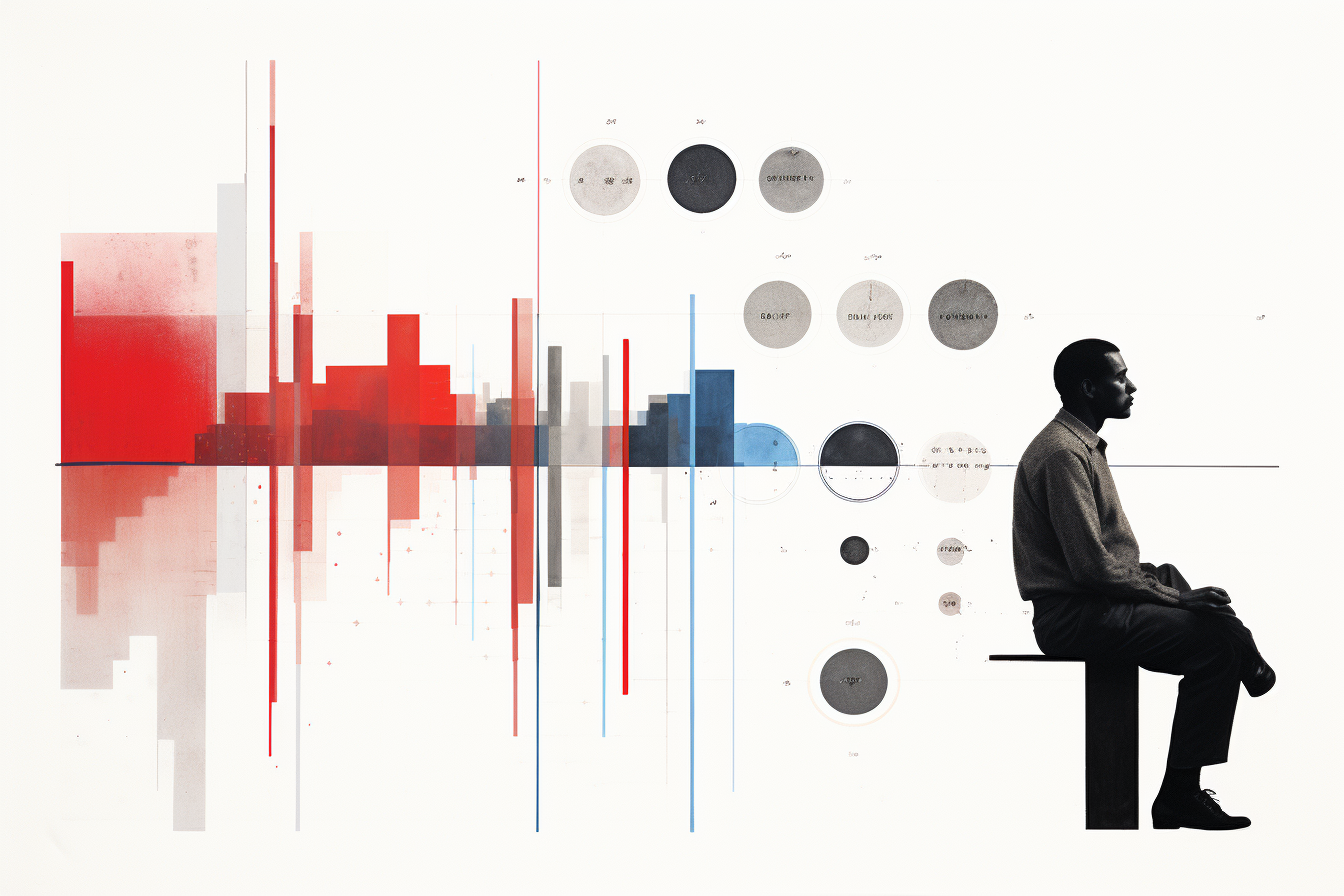
To understand a longitudinal study, let's start with a simple survey as an example. Determining the popularity of a particular product or service at a specific point in time can simply be a matter of collecting and analyzing survey responses from a certain number of people within a population. The qualitative and quantitative data collected from these surveys can tell you what people think at the moment those surveys were conducted. This is what is known as a cross-sectional study .
Now imagine the product that you're trying to assess is seasonal like a brand of ice cream or hot chocolate. What's popular in summer may not be popular in winter, and trends come and go as competing products enter the market. In this context, the one survey that was conducted is merely a snapshot of a moving phenomenon at a single point in time.
In a longitudinal study design, that same survey will be distributed to the same group of people at different time intervals (e.g., twice a year or once a month) to allow researchers to see if there are any changes. Perhaps there is an ice cream that is as popular in the winter as it is in the summer, which may be worth identifying to expand profitability. A longitudinal study would thus be useful to explore this question.
Longitudinal research isn't conducted simply for the sake of being able to say research was conducted over a extended period of time. A longitudinal analysis collects data at different points in time to observe changes in the characteristics of the object of inquiry. Ultimately, collecting data for a longitudinal study can help identify cause-and-effect relationships that cannot otherwise be perceived in discrete or cross-sectional studies.

Longitudinal studies are found in many research fields where time is an important factor. Let's look at examples in three different research areas.
Classroom research is often longitudinal because of the acknowledgment that successful learning takes place over time and not merely in a single class session. Such studies take place over several classes, perhaps over a semester or an entire academic year. A researcher might observe the same group of students as they progress academically or, conversely, identify any significant decline in learning outcomes to determine how changes in teaching and learning over time might affect student development.

Health sciences
Medical research often relies on longitudinal studies to determine the effectiveness and risk factors involved with drugs, treatments, or other medical remedies. Consider a dietary supplement that is purported to help people lose weight. Perhaps, in the beginning, people who take this supplement actually do lose weight. But what happens later on? Do they keep the weight off, gain it back or, even worse, gain even more weight in the long term? A longitudinal study can help researchers determine if that supplement produces sustainable results or is merely a quick fix that has negative side effects later on.

Product life cycles and market trends can take extended periods of time to manifest. In the meantime, competing products might enter the market and consequently affect customer loyalty and product image. If a cross-sectional study captures a snapshot of opinions in the marketplace, then think of a longitudinal study as several snapshots spread out over time to allow researchers to observe changes in market behavior and their underlying causes as time passes.

Cross-sectional studies are discrete studies that capture data within a particular context at a particular point in time. These kinds of studies are more appropriate for research inquiries that don't examine some form of development or evolution, such as concepts or phenomena that are generally static or unchanging over extended periods of time.
To determine which type of study would be more appropriate for your research inquiry, it's important to identify the object of inquiry that is being studied. Ask yourself the following questions when planning your study:
- Do you need an extended period of time to sufficiently capture the phenomenon?
- Is the sample of data collected likely to change over time?
- Is it feasible to commit time and resources to an extended study?
If you said yes to all of these questions, a longitudinal study would be suited to addressing your research questions . Otherwise, cross-sectional studies may be more appropriate for your research.

Intuitive tools, powerful analysis with ATLAS.ti
Make the most of your research with our easy-to-use data analysis platform. Download a free trial today.
A longitudinal study can provide many benefits potentially relevant to the research question you are looking to address. Here are three different advantages you might consider.
Abundance of data
In many cases, research rigor is served by collecting abundant data . Research approaches like thematic analysis and content analysis benefit from a large set of data that helps you identify the most frequently occurring phenomena within a research context. Large data sets collected through longitudinal studies can be useful for separating abundance from anecdotes.
Identification of patterns
Analyzing patterns often implies exploring how things interact sequentially or over time, which is best captured with longitudinal data. Think about, for example, how sports competitions and political elections take place over a year or even multiple years. Construction of ships and buildings can be a long and protracted process. Doctoral students can spend four or more years before earning their degree. A simple cross-sectional study in such contexts may not gather sufficient data captured over a period of time long enough to observe sequences of related events.
Observation of relationships
Certain relationships between different phenomena can only be observed longitudinally. The famous marshmallow test that asserted connections between behaviors in childhood and later life outcomes spawned decades of longitudinal study. Even if your research is much simpler, your research question might involve the observation of distant but related phenomena that only a longitudinal study can capture.
There are two types of longitudinal studies to choose from, primarily depending on what you are looking to examine. Keep in mind that longitudinal study design, no matter what type of study you might pursue, is a matter of sustaining a research inquiry over time to capture the necessary data. It's important that your decision-making process is both transparent and intentional for the sake of research rigor.
Cohort studies
A cohort study examines a group of people that share a common trait. This trait could be a similar age group, a common level of education, or a shared experience.
An example of a cohort study is one that looks to identify factors related to successful aging found in lifestyles among people of middle age. Such a study could observe a group of people, all of whom are similar in age, to identify a common range of lifestyles and activities that are applicable for others of the same age group.

Panel studies
The difference between a cohort study and a panel study is that panel studies collect data from within a general population, rather than a specific set of particular individuals with a common characteristic. The goal of a panel study is to examine a representative sample of a larger population rather than a specific subset of people.
A longitudinal survey that adopts a panel study model, for example, would randomly sample a population and send out questionnaires to the same sample of people over time. Such a survey could look at changes in everyday habits regarding spending or work-life balance and how they might be influenced by environmental or economic shifts from one period of time to the next.
Planning a prospective or future research study that is longitudinal requires careful attention to detail prior to conducting the study. By itself, a longitudinal study can be considered a repeated sequence of the same discrete study across different periods of time.
However, ensuring that multiple iterations of the same study are conducted repeatedly and rigorously is the challenge in longitudinal studies. With that in mind, let's look at some of the different research methods that might be employed in longitudinal research.
Observational research
Action research and ethnographies rely on longitudinal observations to provide sufficient depth to the cultural practices and interactions that are under study. In anthropological and sociological research, some phenomena are so complex or dynamic that they can only be observed longitudinally.
Organizational research, for example, employs longitudinal research to identify how people in the workplace or other similar settings interact with each other. This kind of research is useful for understanding how rapport is established and whether productivity increases as a result.
A longitudinal survey can address research questions that deal with opinions and perspectives that may change over time. Unlike a cross-sectional survey from a particular point in time, longitudinal surveys are administered repeatedly to the same group of people to collect data on changes or developments.
A personal wellness study, for example, might examine how healthy habits (or the lack thereof) affect health by asking respondents questions about their daily routine. By comparing their routines over time with information such as blood pressure, weight, and waist size, survey data on lifestyle routines can allow researchers to identify what habits can cause the greatest improvement in individual health.
Experiments
Various experimental studies, especially in medical research, can be longitudinal in nature. A longitudinal experiment usually collects data from a control group and an experimental group to observe the effects of a certain treatment on the same participants over a period of time.
This type of research is commonly employed to examine the effects of medical treatments on outcomes such as cardiovascular disease or diabetes. The requirements for governmental approval are incredibly stringent and call for rigorous data collection that establishes causality.
Needless to say, longitudinal studies tend to be time-consuming. The most obvious drawback of longitudinal studies is that they take up a significant portion of researchers' time and effort.
However, there are other disadvantages of longitudinal studies, particularly the likelihood of participant attrition. In other words, the more lengthy the study, the more likely it is that participants may drop out of the study. This is especially true when working with vulnerable or marginalized populations such as migrant workers or homeless people, populations that may not always be easy to contact for collecting data.
Over the course of time, the research context that a researcher studies may change with the appearance of new technologies, trends, or other developments that may not have been anticipated. While confounding influences are possible in any study, they are likely to be more abundant in studies on a longitudinal scale. As a result, it's important for the researcher to try to account for these influences when analyzing the data . It could even be worthwhile to examine how the appearance of that phenomenon or concept impacted a relevant outcome of interest in your area.
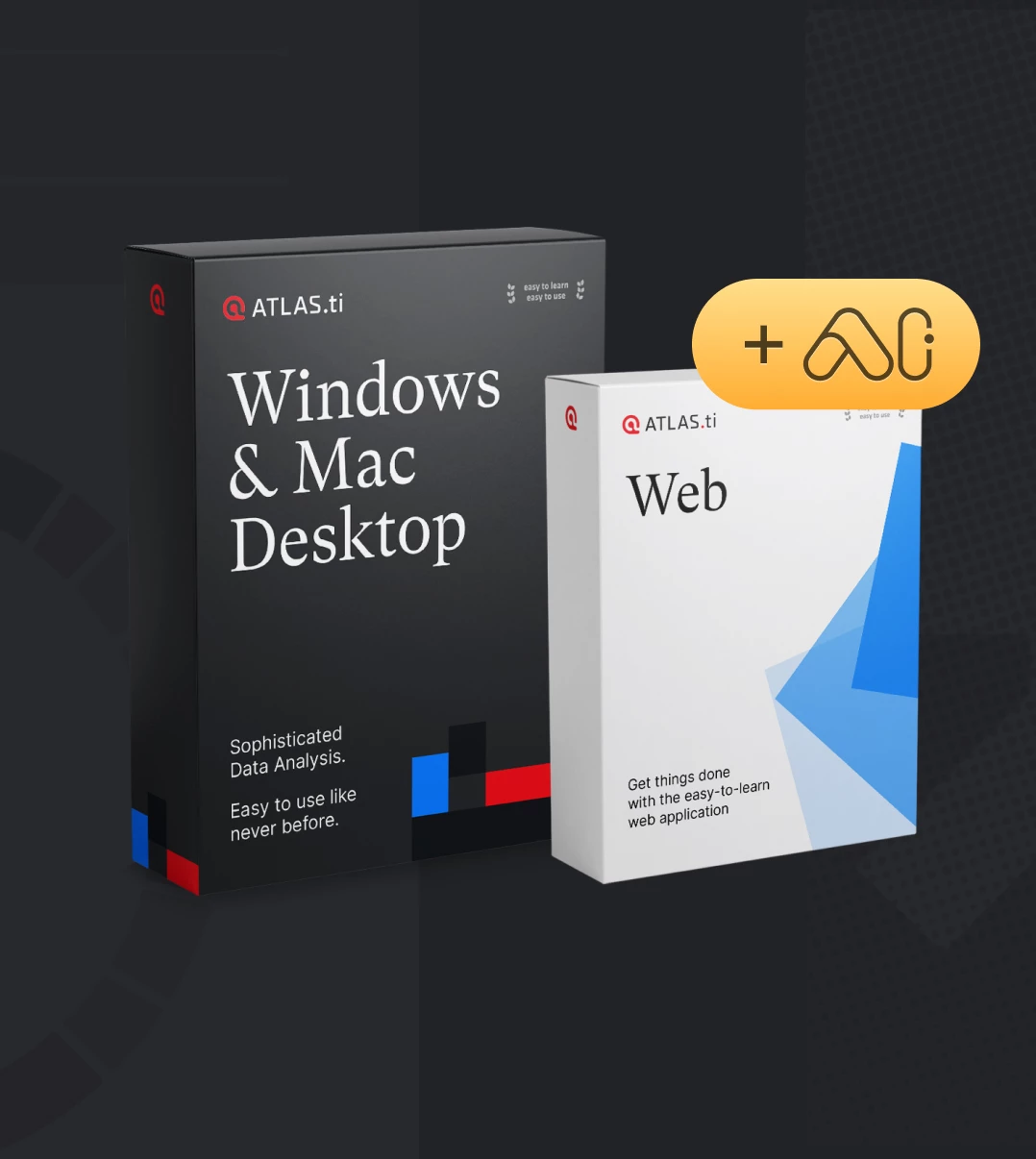
Turn your research into critical insights with ATLAS.ti
Powerful tools to make the most of your data are just a click away. Download a free trial of ATLAS.ti.


An official website of the United States government
The .gov means it’s official. Federal government websites often end in .gov or .mil. Before sharing sensitive information, make sure you’re on a federal government site.
The site is secure. The https:// ensures that you are connecting to the official website and that any information you provide is encrypted and transmitted securely.
- Publications
- Account settings
Preview improvements coming to the PMC website in October 2024. Learn More or Try it out now .
- Advanced Search
- Journal List
- HHS Author Manuscripts

An Overview of the Design, Implementation, and Analyses of Longitudinal Studies on Aging
Anne b. newman.
Center for Aging and Population Health, Department of Epidemiology, Graduate School of Public Health, University of Pittsburgh, Pittsburgh, Pennsylvania
Associated Data
Longitudinal studies have contributed substantially to understanding of aging and geriatric syndromes. These efforts have provided a base of knowledge of the critical factors to consider in designing and implementing new longitudinal studies in older adults. This review highlights some of the major considerations in planning and implementing this type of study. Longitudinal studies can assess change over time and specific disease endpoints. Such projects require multidisciplinary teams with expertise in the many health and contextual factors that must be considered. Recent advances in study design include the use of imaging and biomarkers to assess mechanisms and approaches that raise the ceiling on measurement and integrate assessment of exposures over time. Study implementation requires careful planning and monitoring to maintain fidelity to the scientific goals. Analysis of longitudinal data requires approaches that account for inevitable missing data. New studies should take advantage of the experience obtained from longitudinal studies on aging already conducted.
Longitudinal observational studies have played a major role in geriatric research and in defining the scope of many health concerns in older adults, their risk factors, and their natural history. Because all older adults have significant risk for death and disability, studies that include large samples of community-dwelling older adults have provided an important perspective on the scope of the problems facing an aging population. For example, early studies of disability from the Established Populations for the Epidemiologic Study of the Elderly 1 and the National Long Term Care Survey 2 have demonstrated the large burden of difficulty with functioning in daily life after age 65. More-focused efforts on identifying risk factors have shown that disability is multifactorial, and the more-common conditions that should be targeted to prevent disability have been identified. Furthermore, studies that assess risk broadly have illustrated the commonality of risk factors across geriatric syndromes. 3 Focused studies on specific age-related health conditions such as osteoporosis, 4 cardiovascular disease, 5 , 6 stroke, 7 and dementia 8 have not only been able to assess specific biological pathways that lead to adverse outcomes, but have also assessed the role of other conditions in exacerbating these common problems. Other important contributions have focused on social, behavioral, 9 and economic outcomes. 10 Current and future studies on older populations will be designed to better address this complexity by developing life-course approaches that address early changes, precipitants, and earlier stages of disability. 11 , 12
LONGITUDINAL STUDY DESIGN
The design of longitudinal studies on aging should focus on a set of primary questions and hypotheses while taking into account the important contributions of function, comorbid health conditions, and behavioral and environmental factors. By focusing on primary questions and hypotheses, other methodological concerns can be put into perspective, because it is far too costly and burdensome to measure all aspects of health to the same degree as is necessary to address the primary hypotheses. Design concerns can be classified into those addressing the target population, the exposures, the outcomes, and potential confounders. Cost and practicality may limit the degree of precision in measurement, driving questions back to the priorities determined by the primary questions. Thus, remaining focused on the primary study goals is critical for setting priorities.
To ensure the best design and ultimate productivity, the study’s scientific and administrative leader should assemble a team of investigators and staff who have the skills to contribute to successful design and implementation. This includes content experts in relevant diseases, disability, and aging processes important to the scientific questions, as well as methodological experts in sampling, measurement, and biostatistics. The administrative team must have expertise in budget, environment, and human resource issues. Staff need not have prior medical training but should be detail oriented and dedicated to maintaining fidelity to protocol.
The design of a longitudinal study will vary depending on whether the primary goal is to study changes over time or discrete outcomes. Changes over time generally require frequent contacts. Some outcomes such as stroke or cancer can be assessed using record review, whereas dementia requires in-person examinations. Generalizability needs to be weighed against maintaining follow-up, and these are often competing goals. The requirement for an extensive evaluation and years of follow-up can reduce participation rates. Tiered designs can be used to collect screening data to assess representativeness, with more-intensive data collection from a smaller sample. The internal validity of within-person analysis strengthens longitudinal designs. The sample size needed should be based on power calculations for the primary outcomes of interest and thus vary with the outcome rate. Many health outcomes occur at rate of a few percentage points per year in this age group. Thus, sample sizes of several thousand are often needed to have enough events to study within a reasonable time frame.
The target population will vary with the questions being asked. A fundamental question is what age is most appropriate? Today, 65-year-old people are generally healthier than ever and have low rates of disability and most major health events. Several studies have moved to age 70 to better target the problems of aging. 13 , 14 Conversely, interest in the origins of aging requires targeting earlier ages. For example, the Study of Women’s Health Across the Nation targeted women in the perimenopausal period to understand the role of hormonal change in early age-related processes. 15 , 16 Inclusion and exclusion criteria depend on the outcomes and the outcomes measurement criteria. For example, a study of mobility disability 17 excluded individuals using a cane to walk 400 m at baseline, because inability to walk independently was to be the primary study outcome. Recruitment of individuals within the full spectrum of health, including the frailest, 18 will increase generalizability, although having a large number of participants who have already experienced the outcome, reducing power for incidence studies, may offset this strategy. Careful consideration of the level of cognitive function required for participation can dictate the exclusion criteria for dementia. Regardless of the current level of cognitive function, all longitudinal studies of older adults should identify a potential proxy respondent in case of future compromised cognition of the participant. 19 Studies in the United States need to consider the diversity of the target population and whether to overrecruit subgroups to have adequate power within the groups. In most cases, additional resources are needed to reach minority populations. 20
Ethical concerns in longitudinal studies of older adults warrant special attention. Methods must be in place to establish competency for informed consent 21 and procedures put into place if proxy consent is needed at baseline (for studies of cognitively impaired individuals) or is anticipated for the future, which is the case for most long-term follow-up studies. Results from laboratory testing should be reviewed and reported to subjects if clinically significant findings are identified. Procedures should be designed for, and staff should be trained to attend to, patient safety in the use of any diagnostic or other testing equipment being employed in the study.
Longitudinal observational studies are often designed to assess multiple outcomes. Although it is important and efficient to assess more than a primary outcome, the choice of outcomes should be based on the primary hypothesis, and the study should be powered to address the most important questions. This will, in turn, dictate the sample size to be recruited, which is the major driving factor of the study’s cost and feasibility. Nevertheless, there is a huge scientific advantage to assessing other outcomes, in that risk–benefit ratios can be evaluated. The relationship between multiple health events can be assessed together to determine relative importance and contributions to individual-and societal-level outcomes, such as disability and healthcare utilization. For example the Women’s Health Initiative, 22 by including the observational component, was able to assess breast cancer, fracture, cardiovascular events, and cognitive decline because of the sample size recruited. 23 Together, the findings provide a rich picture of the role that these major conditions play in the functional health of older women. 23 - 26 Linkage of cohort data to the National Death Index, Medicare Beneficiary files, the Minimal Data Set, and other public use files can greatly expand opportunities for outcomes assessment. 27 - 32
Disability is an important outcome that has been assessed using a variety of methods, including self-report, professional assessments, and performance-based measures, such as gait speed and timed tests of specific tasks. Important observations regarding the natural history of exacerbations and remission in these outcomes has led to refinements in defining disability outcomes, such as requiring persistence over time, 14 task modification, 33 , 34 and direct assessment 35 with performance measures. These methods continue to evolve, and there is no consensus on a single approach to define disability outcomes. Recent studies on healthy aging outcomes have shown that there is tremendous variation in functioning that is well above the level designated as disabled. To capture the full spectrum of function and to detect early decline, the study designers should consider using instruments designed to capture a full range of function, including normal, high, and exceptional levels. 36
Exposures of interest should be considered together with the design of the outcomes. Major risk factors are usually identified from the literature or hypothesized from new information on etiology. Behavioral and biological factors should be considered. For example, studies of outcomes of vitamin D exposure should assess diet, sun exposure and season, and blood levels of vitamin D and diseases that can affect its metabolism. 37 Medications can be part of the exposure assessment, in that many medications can alter the primary risk factor being assessed. Examples include vitamin supplements and vitamin levels, lipid levels in the era of statin use, and blood pressure in light of antihypertensive use. Although “baseline” exposure assessments are usually conducted, increasing attention is being paid to including more of a life-course perspective and incorporating historical exposure information from self-report 38 or from other sources, such as geocoding. 39 , 40 Efforts to continue long-term follow-up of younger populations will provide the best estimates of life-course exposures in old age. 41 - 43
Potential confounders that should be considered are so numerous that they can greatly expand the cost and burden of studies in older adults. It is important first to rank all measures according to their role as primary outcomes or exposures so that potential confounders do not overtake resources. Most studies of older adults include measures of common psychosocial factors that can influence function, such as depression, social support, and cognition. Education and smoking history are risk factors for almost every adverse health outcome and should always be included. Age itself is usually assessed according to self-report, but studies of longevity show the importance of more-careful assessment and validation of even this apparently simple confounder. 44 Finally, medication, even if not related directly to the exposure or outcome, can be important to assess as a potential confounder, but collection of information on medications requires special expertise to code them in a way that is useful and accessible for analysis. 45 - 47
Blood laboratory testing is often a major component of a longitudinal study. Most large cohort studies have invested in setting up banks of stored serum, plasma, cells, and deoxyribonucleic acid. Blood tests can be used to define clinical health status, as in determining fasting blood sugar to classify diabetes mellitus, but have been most valuable for allowing for later evaluation of important biomarkers and for genetic testing. As novel markers emerge, stored specimens can be analyzed in a cost-effective case–cohort design.
STUDY IMPLEMENTATION
Once a study is designed, numerous procedures must be put into place to ensure that the data are collected with fidelity to the scientific goals. These steps can take weeks to months or even longer. In multicenter observational cohort studies, it is typical to spend a year or more developing and beginning to implement the study design before actually launching the study. This planning phase should include finalizing the protocol and writing a manual of operations and procedures. All data collection forms should be pretested before the data entry systems are designed, and the system for entry should be in place before the study begins. Time is also needed to hire and train staff, to lay the groundwork for recruitment, and to be sure that the institutional review board has addressed and approved all human subject concerns.
Fielding a study that is to be conducted over the long term requires special attention to measurement. The scientific rationale of each measure, including its role as an outcome, mediator, or potential confounder, should be spelled out in the operations manual. Reproducibility, even if documented in the literature, should be tested in the specific cohort and setting, especially for longitudinal studies that include measurement of change over time. Measurement error can bias associations with change over time, and this analytical concern can be mitigated with adjustment for measurement error per se. 48 All measures should be pretested individually and as a package to work out the study flow. Regular tracking of major measurements through study logs, with commentary and review of all procedures at regular staff meetings, is critical for the identification of potential problems and solutions. Manuals should be revised as needed and staff retrained and certified at least annually.
Data entry can go smoothly when forms are well designed, when staff members complete them without error, and when reports are set up and reviewed regularly for quality control. Keeping up with data entry and running quality checks daily will avoid future recalls and reduce edits. Backlogs of data entry make it more difficult to identify and correct errors in form completion. Real-time data entry and edits are more feasible with software programs that build in range checks and logic.
A successful longitudinal study is proactive in retaining participants. Numerous aspects of study operation lead to successful retention. Suggested methods include keeping to the requested study visit date and duration as agreed to during enrollment and respecting participants’ time. An exit interview should be conducted with every participant to explain follow-up plans and expectations. Regular contacts for follow-up, newsletters, and birthday and holiday cards maintain the relationship between participants and staff. Finally, it is critical that alternative methods be provided to obtain follow-up, including telephone methods, 49 home and nursing home visits, and proxy interviews. 50 As older adults become more impaired, there is inevitable dropout from full participation. Alternative methods that include home visits, telephone interviews, and proxy interviews can lead to high levels of retention for major morbidity and mortality. 50
DATA ANALYSIS
Once the data are in hand, numerous analytical concerns will arise. Missing data are “a given” in longitudinal studies of older adults because of unanticipated illness and death. Methods should be in place to ensure that this is kept to a minimum. 51 Other analytical concerns in longitudinal studies include measurement error; protocol drift over time; migration in equipment specifications and software that affect estimates of change over time; determining changes that are nonlinear, with curvilinear or threshold effects; and substantial biological variability over time. These should be considered in the study design. Analysis of an outcome might be enhanced if time-dependent covariates can be considered. This level of detail of data also needs to be in place in the study design. The analysis should take into account the previously discussed matters of variability and fluctuations over time.
Given the many challenges of conducting longitudinal studies in older adults, it may seem impossible to do it well; such studies are challenging. Successful studies require leadership, teamwork, and excellent communication. Ultimately, prioritizing the primary focus of each study and applying the best science will optimize success. Lessons learned from previous and ongoing longitudinal studies outlined in this review should be helpful in the design of future longitudinal studies.
Supplementary Material
Supplementary 1, acknowledgments.
Sponsor’s Role: None.
Author Contributions: Dr. Newman was the sole author of the manuscript.
Conflict of Interest: Dr. Newman is supported by Grant AG-023629 from the National Institute on Aging (NIA).
What is a longitudinal study?
Last updated
20 February 2023
Reviewed by
Longitudinal studies are common in epidemiology, economics, and medicine. People also use them in other medical and social sciences, such as to study customer trends. Researchers periodically observe and collect data from the variables without manipulating the study environment.
A company may conduct a tracking study, surveying a target audience to measure changes in attitudes and behaviors over time. The collected data doesn't change, and the time interval remains consistent. This longitudinal study can measure brand awareness, customer satisfaction , and consumer opinions and analyze the impact of an advertising campaign.
Analyze longitudinal studies
Dovetail streamlines longitudinal study data to help you uncover and share actionable insights
- Types of longitudinal studies
There are two types of longitudinal studies: Cohort and panel studies.
Panel study
A panel study is a type of longitudinal study that involves collecting data from a fixed number of variables at regular but distant intervals. Researchers follow a group or groups of people over time. Panel studies are designed for quantitative analysis but are also usable for qualitative analysis .
A panel study may research the causes of age-related changes and their effects. Researchers may measure the health markers of a group over time, such as their blood pressure, blood cholesterol, and mental acuity. Then, they can compare the scores to understand how age positively or negatively correlates with these measures.
Cohort study
A cohort longitudinal study involves gathering information from a group of people with something in common, such as a specific trait or experience of the same event. The researchers observe behaviors and other details of the group over time. Unlike panel studies, you can pick a different group to test in cohort studies.
An example of a cohort study could be a drug manufacturer studying the effects on a group of users taking a new drug over a period. A drinks company may want to research consumers with common characteristics, like regular purchasers of sugar-free sodas. This will help the company understand trends within its target market.
- Benefits of longitudinal research
If you want to study the relationship between variables and causal factors responsible for certain outcomes, you should adopt a longitudinal approach to your investigation.
The benefits of longitudinal research over other research methods include the following:
Insights over time
It gives insights into how and why certain things change over time.
Better information
Researchers can better establish sequences of events and identify trends.
No recall bias
The participants won't have recall bias if you use a prospective longitudinal study. Recall bias is an error that occurs in a study if respondents don't wholly or accurately recall the details of their actions, attitudes, or behaviors.
Because variables can change during the study, researchers can discover new relationships or data points worth further investigation.
Small groups
Longitudinal studies don't need a large group of participants.
- Potential pitfalls
The challenges and potential pitfalls of longitudinal studies include the following:
A longitudinal survey takes a long time, involves multiple data collections , and requires complex processes, making it more expensive than other research methods.
Unpredictability
Because they take a long time, longitudinal studies are unpredictable. Unexpected events can cause changes in the variables, making earlier data potentially less valuable.
Slow insights
Researchers can take a long time to uncover insights from the study as it involves multiple observations.
Participants can drop out of the study, limiting the data set and making it harder to draw valid conclusions from the results.
Overly specific data
If you study a smaller group to reduce research costs, results will be less generalizable to larger populations versus a study with a larger group.
Despite these potential pitfalls, you can still derive significant value from a well-designed longitudinal study by uncovering long-term patterns and relationships.
- Longitudinal study designs
Longitudinal studies can take three forms: Repeated cross-sectional, prospective, and retrospective.
Repeated cross-sectional studies
Repeated cross-sectional studies are a type of longitudinal study where participants change across sampling periods. For example, as part of a brand awareness survey , you ask different people from the same customer population about their brand preferences.
Prospective studies
A prospective study is a longitudinal study that involves real-time data collection, and you follow the same participants over a period. Prospective longitudinal studies can be cohort, where participants have similar characteristics or experiences. They can also be panel studies, where you choose the population sample randomly.
Retrospective studies
Retrospective studies are longitudinal studies that involve collecting data on events that some participants have already experienced. Researchers examine historical information to identify patterns that led to an outcome they established at the start of the study. Retrospective studies are the most time and cost-efficient of the three.
- How to perform a longitudinal study
When developing a longitudinal study plan, you must decide whether to collect your data or use data from other sources. Each choice has its benefits and drawbacks.
Using data from other sources
You can freely access data from many previous longitudinal studies, especially studies conducted by governments and research institutes. For example, anyone can access data from the 1970 British Cohort Study on the UK Data Service website .
Using data from other sources saves the time and money you would have spent gathering data. However, the data is more restrictive than the data you collect yourself. You are limited to the variables the original researcher was investigating, and they may have aggregated the data, obscuring some details.
If you can't find data or longitudinal research that applies to your study, the only option is to collect it yourself.
Collecting your own data
Collecting data enhances its relevance, integrity, reliability, and verifiability. Your data collection methods depend on the type of longitudinal study you want to perform. For example, a retrospective longitudinal study collects historical data, while a prospective longitudinal study collects real-time data.
The only way to ensure relevant and reliable data is to use an effective and versatile data collection tool. It can improve the speed and accuracy of the information you collect.
What is a longitudinal study in research?
A longitudinal study is a research design that involves studying the same variables over time by gathering data continuously or repeatedly at consistent intervals.
What is an example of a longitudinal study?
An excellent example of a longitudinal study is market research to identify market trends. The organization's researchers collect data on customers' likes and dislikes to assess market trends and conditions. An organization can also conduct longitudinal studies after launching a new product to understand customers' perceptions and how it is doing in the market.
Why is it called a longitudinal study?
It’s a longitudinal study because you collect data over an extended period. Longitudinal data tracks the same type of information on the same variables at multiple points in time. You collect the data over repeated observations.
What is a longitudinal study vs. a cross-sectional study?
A longitudinal study follows the same people over an extended period, while a cross-sectional study looks at the characteristics of different people or groups at a given time. Longitudinal studies provide insights over an extended period and can establish patterns among variables.
Cross-sectional studies provide insights about a point in time, so they cannot identify cause-and-effect relationships.
Should you be using a customer insights hub?
Do you want to discover previous research faster?
Do you share your research findings with others?
Do you analyze research data?
Start for free today, add your research, and get to key insights faster
Editor’s picks
Last updated: 18 April 2023
Last updated: 27 February 2023
Last updated: 6 February 2023
Last updated: 5 February 2023
Last updated: 16 April 2023
Last updated: 9 March 2023
Last updated: 30 April 2024
Last updated: 12 December 2023
Last updated: 11 March 2024
Last updated: 4 July 2024
Last updated: 6 March 2024
Last updated: 5 March 2024
Last updated: 13 May 2024
Latest articles
Related topics, .css-je19u9{-webkit-align-items:flex-end;-webkit-box-align:flex-end;-ms-flex-align:flex-end;align-items:flex-end;display:-webkit-box;display:-webkit-flex;display:-ms-flexbox;display:flex;-webkit-flex-direction:row;-ms-flex-direction:row;flex-direction:row;-webkit-box-flex-wrap:wrap;-webkit-flex-wrap:wrap;-ms-flex-wrap:wrap;flex-wrap:wrap;-webkit-box-pack:center;-ms-flex-pack:center;-webkit-justify-content:center;justify-content:center;row-gap:0;text-align:center;max-width:671px;}@media (max-width: 1079px){.css-je19u9{max-width:400px;}.css-je19u9>span{white-space:pre;}}@media (max-width: 799px){.css-je19u9{max-width:400px;}.css-je19u9>span{white-space:pre;}} decide what to .css-1kiodld{max-height:56px;display:-webkit-box;display:-webkit-flex;display:-ms-flexbox;display:flex;-webkit-align-items:center;-webkit-box-align:center;-ms-flex-align:center;align-items:center;}@media (max-width: 1079px){.css-1kiodld{display:none;}} build next, decide what to build next, log in or sign up.
Get started for free

What (Exactly) Is A Longitudinal Study?
A plain-language explanation & definition (with examples).
By: Derek Jansen (MBA) | June 2020
If you’re new to the world of research, or it’s your first time writing a dissertation or thesis, you’re probably feeling a bit overwhelmed by all the technical lingo that’s hitting you. If you’ve landed here, chances are one of these terms is “longitudinal study”, “longitudinal survey” or “longitudinal research”.
Worry not – in this post, we’ll explain exactly:
- What a longitudinal study is (and what the alternative is)
- What the main advantages of a longitudinal study are
- What the main disadvantages of a longitudinal study are
- Whether to use a longitudinal or cross-sectional study for your research
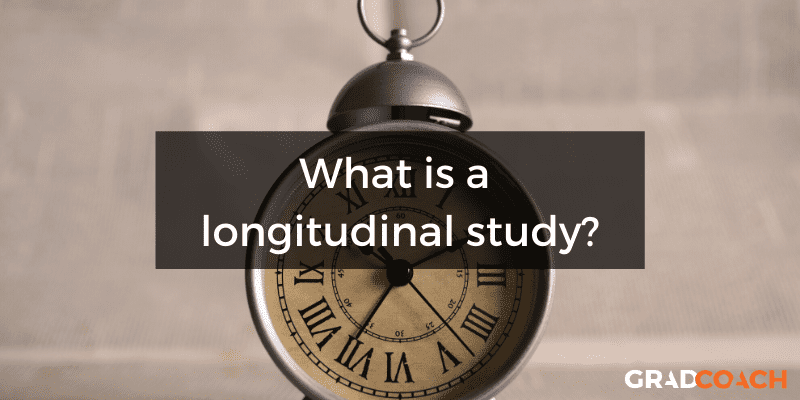
What is a longitudinal study?
A longitudinal study or a longitudinal survey (both of which make up longitudinal research) is a study where the same data are collected more than once, at different points in time . The purpose of a longitudinal study is to assess not just what the data reveal at a fixed point in time, but to understand how (and why) things change over time.
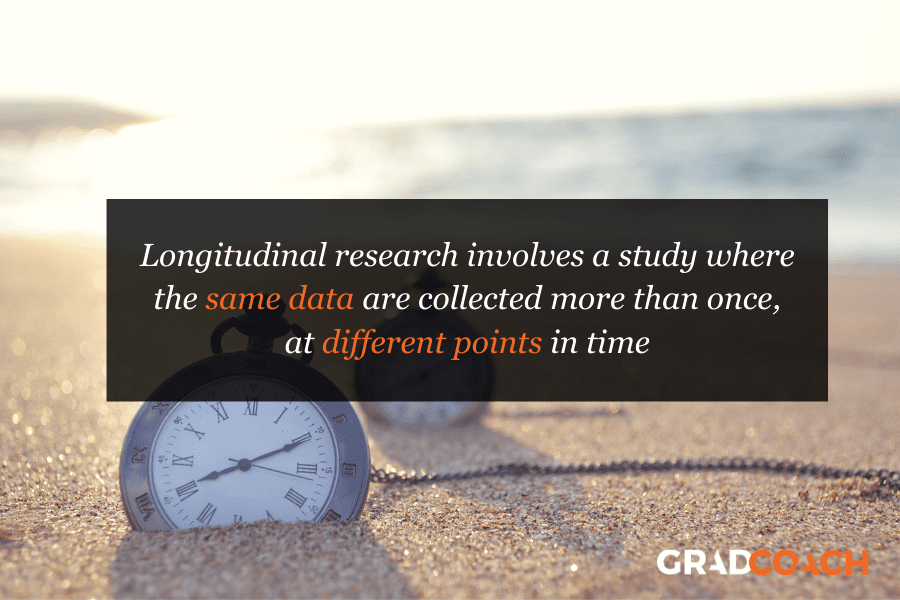
Example: Longitudinal vs Cross-Sectional
Here are two examples – one of a longitudinal study and one of a cross-sectional study – to give you an idea of what these two approaches look like in the real world:
Longitudinal study: a study which assesses how a group of 13-year old children’s attitudes and perspectives towards income inequality evolve over a period of 5 years, with the same group of children surveyed each year, from 2020 (when they are all 13) until 2025 (when they are all 18).
Cross-sectional study: a study which assesses a group of teenagers’ attitudes and perspectives towards income equality at a single point in time. The teenagers are aged 13-18 years and the survey is undertaken in January 2020.
From this example, you can probably see that the topic of both studies is still broadly the same (teenagers’ views on income inequality), but the data produced could potentially be very different . This is because the longitudinal group’s views will be shaped by the events of the next five years, whereas the cross-sectional group all have a “2020 perspective”.
Additionally, in the cross-sectional group, each age group (i.e. 13, 14, 15, 16, 17 and 18) are all different people (obviously!) with different life experiences – whereas, in the longitudinal group, each the data at each age point is generated by the same group of people (for example, John Doe will complete a survey at age 13, 14, 15, and so on).
There are, of course, many other factors at play here and many other ways in which these two approaches differ – but we won’t go down that rabbit hole in this post.
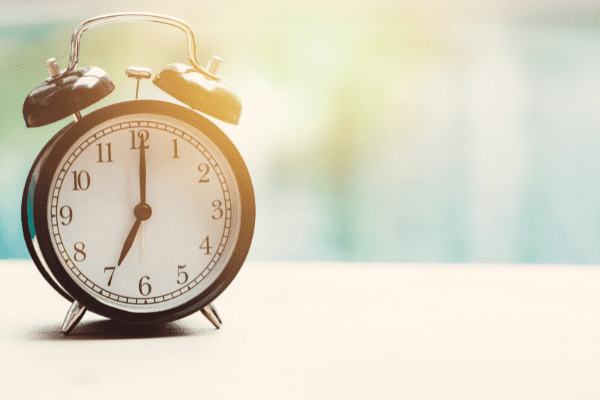
What are the advantages of a longitudinal study?
Longitudinal studies and longitudinal surveys offer some major benefits over cross-sectional studies. Some of the main advantages are:
Patterns – because longitudinal studies involve collecting data at multiple points in time from the same respondents, they allow you to identify emergent patterns across time that you’d never see if you used a cross-sectional approach.
Order – longitudinal studies reveal the order in which things happened, which helps a lot when you’re trying to understand causation. For example, if you’re trying to understand whether X causes Y or Y causes X, it’s essential to understand which one comes first (which a cross-sectional study cannot tell you).
Bias – because longitudinal studies capture current data at multiple points in time, they are at lower risk of recall bias . In other words, there’s a lower chance that people will forget an event, or forget certain details about it, as they are only being asked to discuss current matters.
Need a helping hand?
What are the disadvantages of a longitudinal study?
As you’ve seen, longitudinal studies have some major strengths over cross-sectional studies. So why don’t we just use longitudinal studies for everything? Well, there are (naturally) some disadvantages to longitudinal studies as well.
Cost – compared to cross-sectional studies, longitudinal studies are typically substantially more expensive to execute, as they require maintained effort over a long period of time.
Slow – given the nature of a longitudinal study, it takes a lot longer to pull off than a cross-sectional study. This can be months, years or even decades. This makes them impractical for many types of research, especially dissertations and theses at Honours and Masters levels (where students have a predetermined timeline for their research)
Drop out – because longitudinal studies often take place over many years, there is a very real risk that respondents drop out over the length of the study. This can happen for any number of reasons (for examples, people relocating, starting a family, a new job, etc) and can have a very detrimental effect on the study.
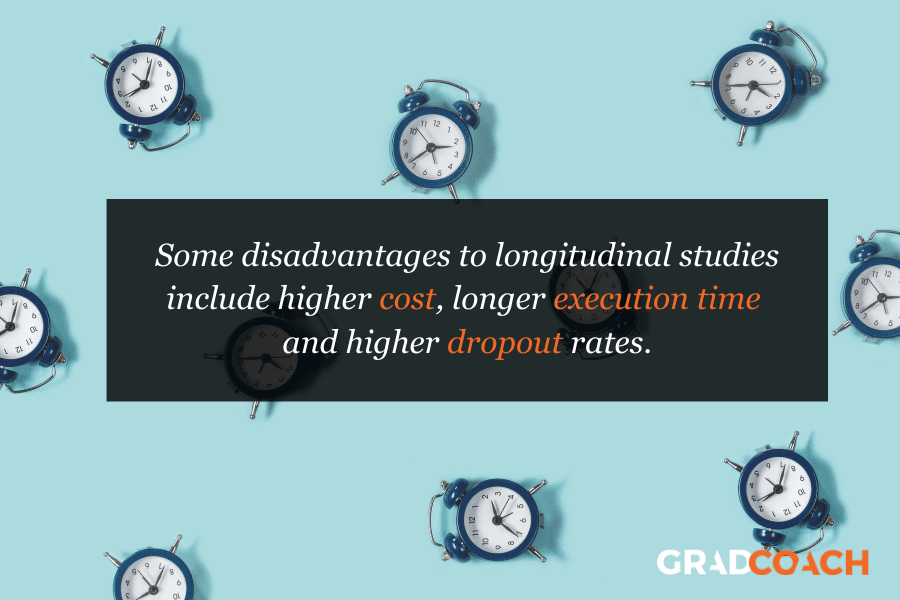
Which one should you use?
Choosing whether to use a longitudinal or cross-sectional study for your dissertation, thesis or research project requires a few considerations. Ultimately, your decision needs to be informed by your overall research aims, objectives and research questions (in other words, the nature of the research determines which approach you should use). But you also need to consider the practicalities. You should ask yourself the following:
- Do you really need a view of how data changes over time, or is a snapshot sufficient?
- Is your university flexible in terms of the timeline for your research?
- Do you have the budget and resources to undertake multiple surveys over time?
- Are you certain you’ll be able to secure respondents over a long period of time?
If your answer to any of these is no, you need to think carefully about the viability of a longitudinal study in your situation. Depending on your research objectives, a cross-sectional design might do the trick. If you’re unsure, speak to your research supervisor or connect with one of our friendly Grad Coaches .
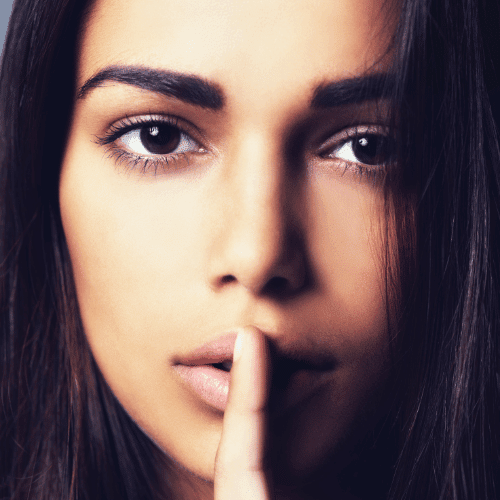

Psst... there’s more!
This post was based on one of our popular Research Bootcamps . If you're working on a research project, you'll definitely want to check this out ...
Submit a Comment Cancel reply
Your email address will not be published. Required fields are marked *
Save my name, email, and website in this browser for the next time I comment.
- Print Friendly
Frequently asked questions
What is the difference between a longitudinal study and a cross-sectional study.
Longitudinal studies and cross-sectional studies are two different types of research design . In a cross-sectional study you collect data from a population at a specific point in time; in a longitudinal study you repeatedly collect data from the same sample over an extended period of time.
Longitudinal study | Cross-sectional study |
---|---|
observations | Observations at a in time |
Observes the multiple times | Observes (a “cross-section”) in the population |
Follows in participants over time | Provides of society at a given point |
Frequently asked questions: Methodology
Attrition refers to participants leaving a study. It always happens to some extent—for example, in randomized controlled trials for medical research.
Differential attrition occurs when attrition or dropout rates differ systematically between the intervention and the control group . As a result, the characteristics of the participants who drop out differ from the characteristics of those who stay in the study. Because of this, study results may be biased .
Action research is conducted in order to solve a particular issue immediately, while case studies are often conducted over a longer period of time and focus more on observing and analyzing a particular ongoing phenomenon.
Action research is focused on solving a problem or informing individual and community-based knowledge in a way that impacts teaching, learning, and other related processes. It is less focused on contributing theoretical input, instead producing actionable input.
Action research is particularly popular with educators as a form of systematic inquiry because it prioritizes reflection and bridges the gap between theory and practice. Educators are able to simultaneously investigate an issue as they solve it, and the method is very iterative and flexible.
A cycle of inquiry is another name for action research . It is usually visualized in a spiral shape following a series of steps, such as “planning → acting → observing → reflecting.”
To make quantitative observations , you need to use instruments that are capable of measuring the quantity you want to observe. For example, you might use a ruler to measure the length of an object or a thermometer to measure its temperature.
Criterion validity and construct validity are both types of measurement validity . In other words, they both show you how accurately a method measures something.
While construct validity is the degree to which a test or other measurement method measures what it claims to measure, criterion validity is the degree to which a test can predictively (in the future) or concurrently (in the present) measure something.
Construct validity is often considered the overarching type of measurement validity . You need to have face validity , content validity , and criterion validity in order to achieve construct validity.
Convergent validity and discriminant validity are both subtypes of construct validity . Together, they help you evaluate whether a test measures the concept it was designed to measure.
- Convergent validity indicates whether a test that is designed to measure a particular construct correlates with other tests that assess the same or similar construct.
- Discriminant validity indicates whether two tests that should not be highly related to each other are indeed not related. This type of validity is also called divergent validity .
You need to assess both in order to demonstrate construct validity. Neither one alone is sufficient for establishing construct validity.
- Discriminant validity indicates whether two tests that should not be highly related to each other are indeed not related
Content validity shows you how accurately a test or other measurement method taps into the various aspects of the specific construct you are researching.
In other words, it helps you answer the question: “does the test measure all aspects of the construct I want to measure?” If it does, then the test has high content validity.
The higher the content validity, the more accurate the measurement of the construct.
If the test fails to include parts of the construct, or irrelevant parts are included, the validity of the instrument is threatened, which brings your results into question.
Face validity and content validity are similar in that they both evaluate how suitable the content of a test is. The difference is that face validity is subjective, and assesses content at surface level.
When a test has strong face validity, anyone would agree that the test’s questions appear to measure what they are intended to measure.
For example, looking at a 4th grade math test consisting of problems in which students have to add and multiply, most people would agree that it has strong face validity (i.e., it looks like a math test).
On the other hand, content validity evaluates how well a test represents all the aspects of a topic. Assessing content validity is more systematic and relies on expert evaluation. of each question, analyzing whether each one covers the aspects that the test was designed to cover.
A 4th grade math test would have high content validity if it covered all the skills taught in that grade. Experts(in this case, math teachers), would have to evaluate the content validity by comparing the test to the learning objectives.
Snowball sampling is a non-probability sampling method . Unlike probability sampling (which involves some form of random selection ), the initial individuals selected to be studied are the ones who recruit new participants.
Because not every member of the target population has an equal chance of being recruited into the sample, selection in snowball sampling is non-random.
Snowball sampling is a non-probability sampling method , where there is not an equal chance for every member of the population to be included in the sample .
This means that you cannot use inferential statistics and make generalizations —often the goal of quantitative research . As such, a snowball sample is not representative of the target population and is usually a better fit for qualitative research .
Snowball sampling relies on the use of referrals. Here, the researcher recruits one or more initial participants, who then recruit the next ones.
Participants share similar characteristics and/or know each other. Because of this, not every member of the population has an equal chance of being included in the sample, giving rise to sampling bias .
Snowball sampling is best used in the following cases:
- If there is no sampling frame available (e.g., people with a rare disease)
- If the population of interest is hard to access or locate (e.g., people experiencing homelessness)
- If the research focuses on a sensitive topic (e.g., extramarital affairs)
The reproducibility and replicability of a study can be ensured by writing a transparent, detailed method section and using clear, unambiguous language.
Reproducibility and replicability are related terms.
- Reproducing research entails reanalyzing the existing data in the same manner.
- Replicating (or repeating ) the research entails reconducting the entire analysis, including the collection of new data .
- A successful reproduction shows that the data analyses were conducted in a fair and honest manner.
- A successful replication shows that the reliability of the results is high.
Stratified sampling and quota sampling both involve dividing the population into subgroups and selecting units from each subgroup. The purpose in both cases is to select a representative sample and/or to allow comparisons between subgroups.
The main difference is that in stratified sampling, you draw a random sample from each subgroup ( probability sampling ). In quota sampling you select a predetermined number or proportion of units, in a non-random manner ( non-probability sampling ).
Purposive and convenience sampling are both sampling methods that are typically used in qualitative data collection.
A convenience sample is drawn from a source that is conveniently accessible to the researcher. Convenience sampling does not distinguish characteristics among the participants. On the other hand, purposive sampling focuses on selecting participants possessing characteristics associated with the research study.
The findings of studies based on either convenience or purposive sampling can only be generalized to the (sub)population from which the sample is drawn, and not to the entire population.
Random sampling or probability sampling is based on random selection. This means that each unit has an equal chance (i.e., equal probability) of being included in the sample.
On the other hand, convenience sampling involves stopping people at random, which means that not everyone has an equal chance of being selected depending on the place, time, or day you are collecting your data.
Convenience sampling and quota sampling are both non-probability sampling methods. They both use non-random criteria like availability, geographical proximity, or expert knowledge to recruit study participants.
However, in convenience sampling, you continue to sample units or cases until you reach the required sample size.
In quota sampling, you first need to divide your population of interest into subgroups (strata) and estimate their proportions (quota) in the population. Then you can start your data collection, using convenience sampling to recruit participants, until the proportions in each subgroup coincide with the estimated proportions in the population.
A sampling frame is a list of every member in the entire population . It is important that the sampling frame is as complete as possible, so that your sample accurately reflects your population.
Stratified and cluster sampling may look similar, but bear in mind that groups created in cluster sampling are heterogeneous , so the individual characteristics in the cluster vary. In contrast, groups created in stratified sampling are homogeneous , as units share characteristics.
Relatedly, in cluster sampling you randomly select entire groups and include all units of each group in your sample. However, in stratified sampling, you select some units of all groups and include them in your sample. In this way, both methods can ensure that your sample is representative of the target population .
A systematic review is secondary research because it uses existing research. You don’t collect new data yourself.
The key difference between observational studies and experimental designs is that a well-done observational study does not influence the responses of participants, while experiments do have some sort of treatment condition applied to at least some participants by random assignment .
An observational study is a great choice for you if your research question is based purely on observations. If there are ethical, logistical, or practical concerns that prevent you from conducting a traditional experiment , an observational study may be a good choice. In an observational study, there is no interference or manipulation of the research subjects, as well as no control or treatment groups .
It’s often best to ask a variety of people to review your measurements. You can ask experts, such as other researchers, or laypeople, such as potential participants, to judge the face validity of tests.
While experts have a deep understanding of research methods , the people you’re studying can provide you with valuable insights you may have missed otherwise.
Face validity is important because it’s a simple first step to measuring the overall validity of a test or technique. It’s a relatively intuitive, quick, and easy way to start checking whether a new measure seems useful at first glance.
Good face validity means that anyone who reviews your measure says that it seems to be measuring what it’s supposed to. With poor face validity, someone reviewing your measure may be left confused about what you’re measuring and why you’re using this method.
Face validity is about whether a test appears to measure what it’s supposed to measure. This type of validity is concerned with whether a measure seems relevant and appropriate for what it’s assessing only on the surface.
Statistical analyses are often applied to test validity with data from your measures. You test convergent validity and discriminant validity with correlations to see if results from your test are positively or negatively related to those of other established tests.
You can also use regression analyses to assess whether your measure is actually predictive of outcomes that you expect it to predict theoretically. A regression analysis that supports your expectations strengthens your claim of construct validity .
When designing or evaluating a measure, construct validity helps you ensure you’re actually measuring the construct you’re interested in. If you don’t have construct validity, you may inadvertently measure unrelated or distinct constructs and lose precision in your research.
Construct validity is often considered the overarching type of measurement validity , because it covers all of the other types. You need to have face validity , content validity , and criterion validity to achieve construct validity.
Construct validity is about how well a test measures the concept it was designed to evaluate. It’s one of four types of measurement validity , which includes construct validity, face validity , and criterion validity.
There are two subtypes of construct validity.
- Convergent validity : The extent to which your measure corresponds to measures of related constructs
- Discriminant validity : The extent to which your measure is unrelated or negatively related to measures of distinct constructs
Naturalistic observation is a valuable tool because of its flexibility, external validity , and suitability for topics that can’t be studied in a lab setting.
The downsides of naturalistic observation include its lack of scientific control , ethical considerations , and potential for bias from observers and subjects.
Naturalistic observation is a qualitative research method where you record the behaviors of your research subjects in real world settings. You avoid interfering or influencing anything in a naturalistic observation.
You can think of naturalistic observation as “people watching” with a purpose.
A dependent variable is what changes as a result of the independent variable manipulation in experiments . It’s what you’re interested in measuring, and it “depends” on your independent variable.
In statistics, dependent variables are also called:
- Response variables (they respond to a change in another variable)
- Outcome variables (they represent the outcome you want to measure)
- Left-hand-side variables (they appear on the left-hand side of a regression equation)
An independent variable is the variable you manipulate, control, or vary in an experimental study to explore its effects. It’s called “independent” because it’s not influenced by any other variables in the study.
Independent variables are also called:
- Explanatory variables (they explain an event or outcome)
- Predictor variables (they can be used to predict the value of a dependent variable)
- Right-hand-side variables (they appear on the right-hand side of a regression equation).
As a rule of thumb, questions related to thoughts, beliefs, and feelings work well in focus groups. Take your time formulating strong questions, paying special attention to phrasing. Be careful to avoid leading questions , which can bias your responses.
Overall, your focus group questions should be:
- Open-ended and flexible
- Impossible to answer with “yes” or “no” (questions that start with “why” or “how” are often best)
- Unambiguous, getting straight to the point while still stimulating discussion
- Unbiased and neutral
A structured interview is a data collection method that relies on asking questions in a set order to collect data on a topic. They are often quantitative in nature. Structured interviews are best used when:
- You already have a very clear understanding of your topic. Perhaps significant research has already been conducted, or you have done some prior research yourself, but you already possess a baseline for designing strong structured questions.
- You are constrained in terms of time or resources and need to analyze your data quickly and efficiently.
- Your research question depends on strong parity between participants, with environmental conditions held constant.
More flexible interview options include semi-structured interviews , unstructured interviews , and focus groups .
Social desirability bias is the tendency for interview participants to give responses that will be viewed favorably by the interviewer or other participants. It occurs in all types of interviews and surveys , but is most common in semi-structured interviews , unstructured interviews , and focus groups .
Social desirability bias can be mitigated by ensuring participants feel at ease and comfortable sharing their views. Make sure to pay attention to your own body language and any physical or verbal cues, such as nodding or widening your eyes.
This type of bias can also occur in observations if the participants know they’re being observed. They might alter their behavior accordingly.
The interviewer effect is a type of bias that emerges when a characteristic of an interviewer (race, age, gender identity, etc.) influences the responses given by the interviewee.
There is a risk of an interviewer effect in all types of interviews , but it can be mitigated by writing really high-quality interview questions.
A semi-structured interview is a blend of structured and unstructured types of interviews. Semi-structured interviews are best used when:
- You have prior interview experience. Spontaneous questions are deceptively challenging, and it’s easy to accidentally ask a leading question or make a participant uncomfortable.
- Your research question is exploratory in nature. Participant answers can guide future research questions and help you develop a more robust knowledge base for future research.
An unstructured interview is the most flexible type of interview, but it is not always the best fit for your research topic.
Unstructured interviews are best used when:
- You are an experienced interviewer and have a very strong background in your research topic, since it is challenging to ask spontaneous, colloquial questions.
- Your research question is exploratory in nature. While you may have developed hypotheses, you are open to discovering new or shifting viewpoints through the interview process.
- You are seeking descriptive data, and are ready to ask questions that will deepen and contextualize your initial thoughts and hypotheses.
- Your research depends on forming connections with your participants and making them feel comfortable revealing deeper emotions, lived experiences, or thoughts.
The four most common types of interviews are:
- Structured interviews : The questions are predetermined in both topic and order.
- Semi-structured interviews : A few questions are predetermined, but other questions aren’t planned.
- Unstructured interviews : None of the questions are predetermined.
- Focus group interviews : The questions are presented to a group instead of one individual.
Deductive reasoning is commonly used in scientific research, and it’s especially associated with quantitative research .
In research, you might have come across something called the hypothetico-deductive method . It’s the scientific method of testing hypotheses to check whether your predictions are substantiated by real-world data.
Deductive reasoning is a logical approach where you progress from general ideas to specific conclusions. It’s often contrasted with inductive reasoning , where you start with specific observations and form general conclusions.
Deductive reasoning is also called deductive logic.
There are many different types of inductive reasoning that people use formally or informally.
Here are a few common types:
- Inductive generalization : You use observations about a sample to come to a conclusion about the population it came from.
- Statistical generalization: You use specific numbers about samples to make statements about populations.
- Causal reasoning: You make cause-and-effect links between different things.
- Sign reasoning: You make a conclusion about a correlational relationship between different things.
- Analogical reasoning: You make a conclusion about something based on its similarities to something else.
Inductive reasoning is a bottom-up approach, while deductive reasoning is top-down.
Inductive reasoning takes you from the specific to the general, while in deductive reasoning, you make inferences by going from general premises to specific conclusions.
In inductive research , you start by making observations or gathering data. Then, you take a broad scan of your data and search for patterns. Finally, you make general conclusions that you might incorporate into theories.
Inductive reasoning is a method of drawing conclusions by going from the specific to the general. It’s usually contrasted with deductive reasoning, where you proceed from general information to specific conclusions.
Inductive reasoning is also called inductive logic or bottom-up reasoning.
A hypothesis states your predictions about what your research will find. It is a tentative answer to your research question that has not yet been tested. For some research projects, you might have to write several hypotheses that address different aspects of your research question.
A hypothesis is not just a guess — it should be based on existing theories and knowledge. It also has to be testable, which means you can support or refute it through scientific research methods (such as experiments, observations and statistical analysis of data).
Triangulation can help:
- Reduce research bias that comes from using a single method, theory, or investigator
- Enhance validity by approaching the same topic with different tools
- Establish credibility by giving you a complete picture of the research problem
But triangulation can also pose problems:
- It’s time-consuming and labor-intensive, often involving an interdisciplinary team.
- Your results may be inconsistent or even contradictory.
There are four main types of triangulation :
- Data triangulation : Using data from different times, spaces, and people
- Investigator triangulation : Involving multiple researchers in collecting or analyzing data
- Theory triangulation : Using varying theoretical perspectives in your research
- Methodological triangulation : Using different methodologies to approach the same topic
Many academic fields use peer review , largely to determine whether a manuscript is suitable for publication. Peer review enhances the credibility of the published manuscript.
However, peer review is also common in non-academic settings. The United Nations, the European Union, and many individual nations use peer review to evaluate grant applications. It is also widely used in medical and health-related fields as a teaching or quality-of-care measure.
Peer assessment is often used in the classroom as a pedagogical tool. Both receiving feedback and providing it are thought to enhance the learning process, helping students think critically and collaboratively.
Peer review can stop obviously problematic, falsified, or otherwise untrustworthy research from being published. It also represents an excellent opportunity to get feedback from renowned experts in your field. It acts as a first defense, helping you ensure your argument is clear and that there are no gaps, vague terms, or unanswered questions for readers who weren’t involved in the research process.
Peer-reviewed articles are considered a highly credible source due to this stringent process they go through before publication.
In general, the peer review process follows the following steps:
- First, the author submits the manuscript to the editor.
- Reject the manuscript and send it back to author, or
- Send it onward to the selected peer reviewer(s)
- Next, the peer review process occurs. The reviewer provides feedback, addressing any major or minor issues with the manuscript, and gives their advice regarding what edits should be made.
- Lastly, the edited manuscript is sent back to the author. They input the edits, and resubmit it to the editor for publication.
Exploratory research is often used when the issue you’re studying is new or when the data collection process is challenging for some reason.
You can use exploratory research if you have a general idea or a specific question that you want to study but there is no preexisting knowledge or paradigm with which to study it.
Exploratory research is a methodology approach that explores research questions that have not previously been studied in depth. It is often used when the issue you’re studying is new, or the data collection process is challenging in some way.
Explanatory research is used to investigate how or why a phenomenon occurs. Therefore, this type of research is often one of the first stages in the research process , serving as a jumping-off point for future research.
Exploratory research aims to explore the main aspects of an under-researched problem, while explanatory research aims to explain the causes and consequences of a well-defined problem.
Explanatory research is a research method used to investigate how or why something occurs when only a small amount of information is available pertaining to that topic. It can help you increase your understanding of a given topic.
Clean data are valid, accurate, complete, consistent, unique, and uniform. Dirty data include inconsistencies and errors.
Dirty data can come from any part of the research process, including poor research design , inappropriate measurement materials, or flawed data entry.
Data cleaning takes place between data collection and data analyses. But you can use some methods even before collecting data.
For clean data, you should start by designing measures that collect valid data. Data validation at the time of data entry or collection helps you minimize the amount of data cleaning you’ll need to do.
After data collection, you can use data standardization and data transformation to clean your data. You’ll also deal with any missing values, outliers, and duplicate values.
Every dataset requires different techniques to clean dirty data , but you need to address these issues in a systematic way. You focus on finding and resolving data points that don’t agree or fit with the rest of your dataset.
These data might be missing values, outliers, duplicate values, incorrectly formatted, or irrelevant. You’ll start with screening and diagnosing your data. Then, you’ll often standardize and accept or remove data to make your dataset consistent and valid.
Data cleaning is necessary for valid and appropriate analyses. Dirty data contain inconsistencies or errors , but cleaning your data helps you minimize or resolve these.
Without data cleaning, you could end up with a Type I or II error in your conclusion. These types of erroneous conclusions can be practically significant with important consequences, because they lead to misplaced investments or missed opportunities.
Data cleaning involves spotting and resolving potential data inconsistencies or errors to improve your data quality. An error is any value (e.g., recorded weight) that doesn’t reflect the true value (e.g., actual weight) of something that’s being measured.
In this process, you review, analyze, detect, modify, or remove “dirty” data to make your dataset “clean.” Data cleaning is also called data cleansing or data scrubbing.
Research misconduct means making up or falsifying data, manipulating data analyses, or misrepresenting results in research reports. It’s a form of academic fraud.
These actions are committed intentionally and can have serious consequences; research misconduct is not a simple mistake or a point of disagreement but a serious ethical failure.
Anonymity means you don’t know who the participants are, while confidentiality means you know who they are but remove identifying information from your research report. Both are important ethical considerations .
You can only guarantee anonymity by not collecting any personally identifying information—for example, names, phone numbers, email addresses, IP addresses, physical characteristics, photos, or videos.
You can keep data confidential by using aggregate information in your research report, so that you only refer to groups of participants rather than individuals.
Research ethics matter for scientific integrity, human rights and dignity, and collaboration between science and society. These principles make sure that participation in studies is voluntary, informed, and safe.
Ethical considerations in research are a set of principles that guide your research designs and practices. These principles include voluntary participation, informed consent, anonymity, confidentiality, potential for harm, and results communication.
Scientists and researchers must always adhere to a certain code of conduct when collecting data from others .
These considerations protect the rights of research participants, enhance research validity , and maintain scientific integrity.
In multistage sampling , you can use probability or non-probability sampling methods .
For a probability sample, you have to conduct probability sampling at every stage.
You can mix it up by using simple random sampling , systematic sampling , or stratified sampling to select units at different stages, depending on what is applicable and relevant to your study.
Multistage sampling can simplify data collection when you have large, geographically spread samples, and you can obtain a probability sample without a complete sampling frame.
But multistage sampling may not lead to a representative sample, and larger samples are needed for multistage samples to achieve the statistical properties of simple random samples .
These are four of the most common mixed methods designs :
- Convergent parallel: Quantitative and qualitative data are collected at the same time and analyzed separately. After both analyses are complete, compare your results to draw overall conclusions.
- Embedded: Quantitative and qualitative data are collected at the same time, but within a larger quantitative or qualitative design. One type of data is secondary to the other.
- Explanatory sequential: Quantitative data is collected and analyzed first, followed by qualitative data. You can use this design if you think your qualitative data will explain and contextualize your quantitative findings.
- Exploratory sequential: Qualitative data is collected and analyzed first, followed by quantitative data. You can use this design if you think the quantitative data will confirm or validate your qualitative findings.
Triangulation in research means using multiple datasets, methods, theories and/or investigators to address a research question. It’s a research strategy that can help you enhance the validity and credibility of your findings.
Triangulation is mainly used in qualitative research , but it’s also commonly applied in quantitative research . Mixed methods research always uses triangulation.
In multistage sampling , or multistage cluster sampling, you draw a sample from a population using smaller and smaller groups at each stage.
This method is often used to collect data from a large, geographically spread group of people in national surveys, for example. You take advantage of hierarchical groupings (e.g., from state to city to neighborhood) to create a sample that’s less expensive and time-consuming to collect data from.
No, the steepness or slope of the line isn’t related to the correlation coefficient value. The correlation coefficient only tells you how closely your data fit on a line, so two datasets with the same correlation coefficient can have very different slopes.
To find the slope of the line, you’ll need to perform a regression analysis .
Correlation coefficients always range between -1 and 1.
The sign of the coefficient tells you the direction of the relationship: a positive value means the variables change together in the same direction, while a negative value means they change together in opposite directions.
The absolute value of a number is equal to the number without its sign. The absolute value of a correlation coefficient tells you the magnitude of the correlation: the greater the absolute value, the stronger the correlation.
These are the assumptions your data must meet if you want to use Pearson’s r :
- Both variables are on an interval or ratio level of measurement
- Data from both variables follow normal distributions
- Your data have no outliers
- Your data is from a random or representative sample
- You expect a linear relationship between the two variables
Quantitative research designs can be divided into two main categories:
- Correlational and descriptive designs are used to investigate characteristics, averages, trends, and associations between variables.
- Experimental and quasi-experimental designs are used to test causal relationships .
Qualitative research designs tend to be more flexible. Common types of qualitative design include case study , ethnography , and grounded theory designs.
A well-planned research design helps ensure that your methods match your research aims, that you collect high-quality data, and that you use the right kind of analysis to answer your questions, utilizing credible sources . This allows you to draw valid , trustworthy conclusions.
The priorities of a research design can vary depending on the field, but you usually have to specify:
- Your research questions and/or hypotheses
- Your overall approach (e.g., qualitative or quantitative )
- The type of design you’re using (e.g., a survey , experiment , or case study )
- Your sampling methods or criteria for selecting subjects
- Your data collection methods (e.g., questionnaires , observations)
- Your data collection procedures (e.g., operationalization , timing and data management)
- Your data analysis methods (e.g., statistical tests or thematic analysis )
A research design is a strategy for answering your research question . It defines your overall approach and determines how you will collect and analyze data.
Questionnaires can be self-administered or researcher-administered.
Self-administered questionnaires can be delivered online or in paper-and-pen formats, in person or through mail. All questions are standardized so that all respondents receive the same questions with identical wording.
Researcher-administered questionnaires are interviews that take place by phone, in-person, or online between researchers and respondents. You can gain deeper insights by clarifying questions for respondents or asking follow-up questions.
You can organize the questions logically, with a clear progression from simple to complex, or randomly between respondents. A logical flow helps respondents process the questionnaire easier and quicker, but it may lead to bias. Randomization can minimize the bias from order effects.
Closed-ended, or restricted-choice, questions offer respondents a fixed set of choices to select from. These questions are easier to answer quickly.
Open-ended or long-form questions allow respondents to answer in their own words. Because there are no restrictions on their choices, respondents can answer in ways that researchers may not have otherwise considered.
A questionnaire is a data collection tool or instrument, while a survey is an overarching research method that involves collecting and analyzing data from people using questionnaires.
The third variable and directionality problems are two main reasons why correlation isn’t causation .
The third variable problem means that a confounding variable affects both variables to make them seem causally related when they are not.
The directionality problem is when two variables correlate and might actually have a causal relationship, but it’s impossible to conclude which variable causes changes in the other.
Correlation describes an association between variables : when one variable changes, so does the other. A correlation is a statistical indicator of the relationship between variables.
Causation means that changes in one variable brings about changes in the other (i.e., there is a cause-and-effect relationship between variables). The two variables are correlated with each other, and there’s also a causal link between them.
While causation and correlation can exist simultaneously, correlation does not imply causation. In other words, correlation is simply a relationship where A relates to B—but A doesn’t necessarily cause B to happen (or vice versa). Mistaking correlation for causation is a common error and can lead to false cause fallacy .
Controlled experiments establish causality, whereas correlational studies only show associations between variables.
- In an experimental design , you manipulate an independent variable and measure its effect on a dependent variable. Other variables are controlled so they can’t impact the results.
- In a correlational design , you measure variables without manipulating any of them. You can test whether your variables change together, but you can’t be sure that one variable caused a change in another.
In general, correlational research is high in external validity while experimental research is high in internal validity .
A correlation is usually tested for two variables at a time, but you can test correlations between three or more variables.
A correlation coefficient is a single number that describes the strength and direction of the relationship between your variables.
Different types of correlation coefficients might be appropriate for your data based on their levels of measurement and distributions . The Pearson product-moment correlation coefficient (Pearson’s r ) is commonly used to assess a linear relationship between two quantitative variables.
A correlational research design investigates relationships between two variables (or more) without the researcher controlling or manipulating any of them. It’s a non-experimental type of quantitative research .
A correlation reflects the strength and/or direction of the association between two or more variables.
- A positive correlation means that both variables change in the same direction.
- A negative correlation means that the variables change in opposite directions.
- A zero correlation means there’s no relationship between the variables.
Random error is almost always present in scientific studies, even in highly controlled settings. While you can’t eradicate it completely, you can reduce random error by taking repeated measurements, using a large sample, and controlling extraneous variables .
You can avoid systematic error through careful design of your sampling , data collection , and analysis procedures. For example, use triangulation to measure your variables using multiple methods; regularly calibrate instruments or procedures; use random sampling and random assignment ; and apply masking (blinding) where possible.
Systematic error is generally a bigger problem in research.
With random error, multiple measurements will tend to cluster around the true value. When you’re collecting data from a large sample , the errors in different directions will cancel each other out.
Systematic errors are much more problematic because they can skew your data away from the true value. This can lead you to false conclusions ( Type I and II errors ) about the relationship between the variables you’re studying.
Random and systematic error are two types of measurement error.
Random error is a chance difference between the observed and true values of something (e.g., a researcher misreading a weighing scale records an incorrect measurement).
Systematic error is a consistent or proportional difference between the observed and true values of something (e.g., a miscalibrated scale consistently records weights as higher than they actually are).
On graphs, the explanatory variable is conventionally placed on the x-axis, while the response variable is placed on the y-axis.
- If you have quantitative variables , use a scatterplot or a line graph.
- If your response variable is categorical, use a scatterplot or a line graph.
- If your explanatory variable is categorical, use a bar graph.
The term “ explanatory variable ” is sometimes preferred over “ independent variable ” because, in real world contexts, independent variables are often influenced by other variables. This means they aren’t totally independent.
Multiple independent variables may also be correlated with each other, so “explanatory variables” is a more appropriate term.
The difference between explanatory and response variables is simple:
- An explanatory variable is the expected cause, and it explains the results.
- A response variable is the expected effect, and it responds to other variables.
In a controlled experiment , all extraneous variables are held constant so that they can’t influence the results. Controlled experiments require:
- A control group that receives a standard treatment, a fake treatment, or no treatment.
- Random assignment of participants to ensure the groups are equivalent.
Depending on your study topic, there are various other methods of controlling variables .
There are 4 main types of extraneous variables :
- Demand characteristics : environmental cues that encourage participants to conform to researchers’ expectations.
- Experimenter effects : unintentional actions by researchers that influence study outcomes.
- Situational variables : environmental variables that alter participants’ behaviors.
- Participant variables : any characteristic or aspect of a participant’s background that could affect study results.
An extraneous variable is any variable that you’re not investigating that can potentially affect the dependent variable of your research study.
A confounding variable is a type of extraneous variable that not only affects the dependent variable, but is also related to the independent variable.
In a factorial design, multiple independent variables are tested.
If you test two variables, each level of one independent variable is combined with each level of the other independent variable to create different conditions.
Within-subjects designs have many potential threats to internal validity , but they are also very statistically powerful .
Advantages:
- Only requires small samples
- Statistically powerful
- Removes the effects of individual differences on the outcomes
Disadvantages:
- Internal validity threats reduce the likelihood of establishing a direct relationship between variables
- Time-related effects, such as growth, can influence the outcomes
- Carryover effects mean that the specific order of different treatments affect the outcomes
While a between-subjects design has fewer threats to internal validity , it also requires more participants for high statistical power than a within-subjects design .
- Prevents carryover effects of learning and fatigue.
- Shorter study duration.
- Needs larger samples for high power.
- Uses more resources to recruit participants, administer sessions, cover costs, etc.
- Individual differences may be an alternative explanation for results.
Yes. Between-subjects and within-subjects designs can be combined in a single study when you have two or more independent variables (a factorial design). In a mixed factorial design, one variable is altered between subjects and another is altered within subjects.
In a between-subjects design , every participant experiences only one condition, and researchers assess group differences between participants in various conditions.
In a within-subjects design , each participant experiences all conditions, and researchers test the same participants repeatedly for differences between conditions.
The word “between” means that you’re comparing different conditions between groups, while the word “within” means you’re comparing different conditions within the same group.
Random assignment is used in experiments with a between-groups or independent measures design. In this research design, there’s usually a control group and one or more experimental groups. Random assignment helps ensure that the groups are comparable.
In general, you should always use random assignment in this type of experimental design when it is ethically possible and makes sense for your study topic.
To implement random assignment , assign a unique number to every member of your study’s sample .
Then, you can use a random number generator or a lottery method to randomly assign each number to a control or experimental group. You can also do so manually, by flipping a coin or rolling a dice to randomly assign participants to groups.
Random selection, or random sampling , is a way of selecting members of a population for your study’s sample.
In contrast, random assignment is a way of sorting the sample into control and experimental groups.
Random sampling enhances the external validity or generalizability of your results, while random assignment improves the internal validity of your study.
In experimental research, random assignment is a way of placing participants from your sample into different groups using randomization. With this method, every member of the sample has a known or equal chance of being placed in a control group or an experimental group.
“Controlling for a variable” means measuring extraneous variables and accounting for them statistically to remove their effects on other variables.
Researchers often model control variable data along with independent and dependent variable data in regression analyses and ANCOVAs . That way, you can isolate the control variable’s effects from the relationship between the variables of interest.
Control variables help you establish a correlational or causal relationship between variables by enhancing internal validity .
If you don’t control relevant extraneous variables , they may influence the outcomes of your study, and you may not be able to demonstrate that your results are really an effect of your independent variable .
A control variable is any variable that’s held constant in a research study. It’s not a variable of interest in the study, but it’s controlled because it could influence the outcomes.
Including mediators and moderators in your research helps you go beyond studying a simple relationship between two variables for a fuller picture of the real world. They are important to consider when studying complex correlational or causal relationships.
Mediators are part of the causal pathway of an effect, and they tell you how or why an effect takes place. Moderators usually help you judge the external validity of your study by identifying the limitations of when the relationship between variables holds.
If something is a mediating variable :
- It’s caused by the independent variable .
- It influences the dependent variable
- When it’s taken into account, the statistical correlation between the independent and dependent variables is higher than when it isn’t considered.
A confounder is a third variable that affects variables of interest and makes them seem related when they are not. In contrast, a mediator is the mechanism of a relationship between two variables: it explains the process by which they are related.
A mediator variable explains the process through which two variables are related, while a moderator variable affects the strength and direction of that relationship.
There are three key steps in systematic sampling :
- Define and list your population , ensuring that it is not ordered in a cyclical or periodic order.
- Decide on your sample size and calculate your interval, k , by dividing your population by your target sample size.
- Choose every k th member of the population as your sample.
Systematic sampling is a probability sampling method where researchers select members of the population at a regular interval – for example, by selecting every 15th person on a list of the population. If the population is in a random order, this can imitate the benefits of simple random sampling .
Yes, you can create a stratified sample using multiple characteristics, but you must ensure that every participant in your study belongs to one and only one subgroup. In this case, you multiply the numbers of subgroups for each characteristic to get the total number of groups.
For example, if you were stratifying by location with three subgroups (urban, rural, or suburban) and marital status with five subgroups (single, divorced, widowed, married, or partnered), you would have 3 x 5 = 15 subgroups.
You should use stratified sampling when your sample can be divided into mutually exclusive and exhaustive subgroups that you believe will take on different mean values for the variable that you’re studying.
Using stratified sampling will allow you to obtain more precise (with lower variance ) statistical estimates of whatever you are trying to measure.
For example, say you want to investigate how income differs based on educational attainment, but you know that this relationship can vary based on race. Using stratified sampling, you can ensure you obtain a large enough sample from each racial group, allowing you to draw more precise conclusions.
In stratified sampling , researchers divide subjects into subgroups called strata based on characteristics that they share (e.g., race, gender, educational attainment).
Once divided, each subgroup is randomly sampled using another probability sampling method.
Cluster sampling is more time- and cost-efficient than other probability sampling methods , particularly when it comes to large samples spread across a wide geographical area.
However, it provides less statistical certainty than other methods, such as simple random sampling , because it is difficult to ensure that your clusters properly represent the population as a whole.
There are three types of cluster sampling : single-stage, double-stage and multi-stage clustering. In all three types, you first divide the population into clusters, then randomly select clusters for use in your sample.
- In single-stage sampling , you collect data from every unit within the selected clusters.
- In double-stage sampling , you select a random sample of units from within the clusters.
- In multi-stage sampling , you repeat the procedure of randomly sampling elements from within the clusters until you have reached a manageable sample.
Cluster sampling is a probability sampling method in which you divide a population into clusters, such as districts or schools, and then randomly select some of these clusters as your sample.
The clusters should ideally each be mini-representations of the population as a whole.
If properly implemented, simple random sampling is usually the best sampling method for ensuring both internal and external validity . However, it can sometimes be impractical and expensive to implement, depending on the size of the population to be studied,
If you have a list of every member of the population and the ability to reach whichever members are selected, you can use simple random sampling.
The American Community Survey is an example of simple random sampling . In order to collect detailed data on the population of the US, the Census Bureau officials randomly select 3.5 million households per year and use a variety of methods to convince them to fill out the survey.
Simple random sampling is a type of probability sampling in which the researcher randomly selects a subset of participants from a population . Each member of the population has an equal chance of being selected. Data is then collected from as large a percentage as possible of this random subset.
Quasi-experimental design is most useful in situations where it would be unethical or impractical to run a true experiment .
Quasi-experiments have lower internal validity than true experiments, but they often have higher external validity as they can use real-world interventions instead of artificial laboratory settings.
A quasi-experiment is a type of research design that attempts to establish a cause-and-effect relationship. The main difference with a true experiment is that the groups are not randomly assigned.
Blinding is important to reduce research bias (e.g., observer bias , demand characteristics ) and ensure a study’s internal validity .
If participants know whether they are in a control or treatment group , they may adjust their behavior in ways that affect the outcome that researchers are trying to measure. If the people administering the treatment are aware of group assignment, they may treat participants differently and thus directly or indirectly influence the final results.
- In a single-blind study , only the participants are blinded.
- In a double-blind study , both participants and experimenters are blinded.
- In a triple-blind study , the assignment is hidden not only from participants and experimenters, but also from the researchers analyzing the data.
Blinding means hiding who is assigned to the treatment group and who is assigned to the control group in an experiment .
A true experiment (a.k.a. a controlled experiment) always includes at least one control group that doesn’t receive the experimental treatment.
However, some experiments use a within-subjects design to test treatments without a control group. In these designs, you usually compare one group’s outcomes before and after a treatment (instead of comparing outcomes between different groups).
For strong internal validity , it’s usually best to include a control group if possible. Without a control group, it’s harder to be certain that the outcome was caused by the experimental treatment and not by other variables.
An experimental group, also known as a treatment group, receives the treatment whose effect researchers wish to study, whereas a control group does not. They should be identical in all other ways.
Individual Likert-type questions are generally considered ordinal data , because the items have clear rank order, but don’t have an even distribution.
Overall Likert scale scores are sometimes treated as interval data. These scores are considered to have directionality and even spacing between them.
The type of data determines what statistical tests you should use to analyze your data.
A Likert scale is a rating scale that quantitatively assesses opinions, attitudes, or behaviors. It is made up of 4 or more questions that measure a single attitude or trait when response scores are combined.
To use a Likert scale in a survey , you present participants with Likert-type questions or statements, and a continuum of items, usually with 5 or 7 possible responses, to capture their degree of agreement.
In scientific research, concepts are the abstract ideas or phenomena that are being studied (e.g., educational achievement). Variables are properties or characteristics of the concept (e.g., performance at school), while indicators are ways of measuring or quantifying variables (e.g., yearly grade reports).
The process of turning abstract concepts into measurable variables and indicators is called operationalization .
There are various approaches to qualitative data analysis , but they all share five steps in common:
- Prepare and organize your data.
- Review and explore your data.
- Develop a data coding system.
- Assign codes to the data.
- Identify recurring themes.
The specifics of each step depend on the focus of the analysis. Some common approaches include textual analysis , thematic analysis , and discourse analysis .
There are five common approaches to qualitative research :
- Grounded theory involves collecting data in order to develop new theories.
- Ethnography involves immersing yourself in a group or organization to understand its culture.
- Narrative research involves interpreting stories to understand how people make sense of their experiences and perceptions.
- Phenomenological research involves investigating phenomena through people’s lived experiences.
- Action research links theory and practice in several cycles to drive innovative changes.
Hypothesis testing is a formal procedure for investigating our ideas about the world using statistics. It is used by scientists to test specific predictions, called hypotheses , by calculating how likely it is that a pattern or relationship between variables could have arisen by chance.
Operationalization means turning abstract conceptual ideas into measurable observations.
For example, the concept of social anxiety isn’t directly observable, but it can be operationally defined in terms of self-rating scores, behavioral avoidance of crowded places, or physical anxiety symptoms in social situations.
Before collecting data , it’s important to consider how you will operationalize the variables that you want to measure.
When conducting research, collecting original data has significant advantages:
- You can tailor data collection to your specific research aims (e.g. understanding the needs of your consumers or user testing your website)
- You can control and standardize the process for high reliability and validity (e.g. choosing appropriate measurements and sampling methods )
However, there are also some drawbacks: data collection can be time-consuming, labor-intensive and expensive. In some cases, it’s more efficient to use secondary data that has already been collected by someone else, but the data might be less reliable.
Data collection is the systematic process by which observations or measurements are gathered in research. It is used in many different contexts by academics, governments, businesses, and other organizations.
There are several methods you can use to decrease the impact of confounding variables on your research: restriction, matching, statistical control and randomization.
In restriction , you restrict your sample by only including certain subjects that have the same values of potential confounding variables.
In matching , you match each of the subjects in your treatment group with a counterpart in the comparison group. The matched subjects have the same values on any potential confounding variables, and only differ in the independent variable .
In statistical control , you include potential confounders as variables in your regression .
In randomization , you randomly assign the treatment (or independent variable) in your study to a sufficiently large number of subjects, which allows you to control for all potential confounding variables.
A confounding variable is closely related to both the independent and dependent variables in a study. An independent variable represents the supposed cause , while the dependent variable is the supposed effect . A confounding variable is a third variable that influences both the independent and dependent variables.
Failing to account for confounding variables can cause you to wrongly estimate the relationship between your independent and dependent variables.
To ensure the internal validity of your research, you must consider the impact of confounding variables. If you fail to account for them, you might over- or underestimate the causal relationship between your independent and dependent variables , or even find a causal relationship where none exists.
Yes, but including more than one of either type requires multiple research questions .
For example, if you are interested in the effect of a diet on health, you can use multiple measures of health: blood sugar, blood pressure, weight, pulse, and many more. Each of these is its own dependent variable with its own research question.
You could also choose to look at the effect of exercise levels as well as diet, or even the additional effect of the two combined. Each of these is a separate independent variable .
To ensure the internal validity of an experiment , you should only change one independent variable at a time.
No. The value of a dependent variable depends on an independent variable, so a variable cannot be both independent and dependent at the same time. It must be either the cause or the effect, not both!
You want to find out how blood sugar levels are affected by drinking diet soda and regular soda, so you conduct an experiment .
- The type of soda – diet or regular – is the independent variable .
- The level of blood sugar that you measure is the dependent variable – it changes depending on the type of soda.
Determining cause and effect is one of the most important parts of scientific research. It’s essential to know which is the cause – the independent variable – and which is the effect – the dependent variable.
In non-probability sampling , the sample is selected based on non-random criteria, and not every member of the population has a chance of being included.
Common non-probability sampling methods include convenience sampling , voluntary response sampling, purposive sampling , snowball sampling, and quota sampling .
Probability sampling means that every member of the target population has a known chance of being included in the sample.
Probability sampling methods include simple random sampling , systematic sampling , stratified sampling , and cluster sampling .
Using careful research design and sampling procedures can help you avoid sampling bias . Oversampling can be used to correct undercoverage bias .
Some common types of sampling bias include self-selection bias , nonresponse bias , undercoverage bias , survivorship bias , pre-screening or advertising bias, and healthy user bias.
Sampling bias is a threat to external validity – it limits the generalizability of your findings to a broader group of people.
A sampling error is the difference between a population parameter and a sample statistic .
A statistic refers to measures about the sample , while a parameter refers to measures about the population .
Populations are used when a research question requires data from every member of the population. This is usually only feasible when the population is small and easily accessible.
Samples are used to make inferences about populations . Samples are easier to collect data from because they are practical, cost-effective, convenient, and manageable.
There are seven threats to external validity : selection bias , history, experimenter effect, Hawthorne effect , testing effect, aptitude-treatment and situation effect.
The two types of external validity are population validity (whether you can generalize to other groups of people) and ecological validity (whether you can generalize to other situations and settings).
The external validity of a study is the extent to which you can generalize your findings to different groups of people, situations, and measures.
Cross-sectional studies cannot establish a cause-and-effect relationship or analyze behavior over a period of time. To investigate cause and effect, you need to do a longitudinal study or an experimental study .
Cross-sectional studies are less expensive and time-consuming than many other types of study. They can provide useful insights into a population’s characteristics and identify correlations for further research.
Sometimes only cross-sectional data is available for analysis; other times your research question may only require a cross-sectional study to answer it.
Longitudinal studies can last anywhere from weeks to decades, although they tend to be at least a year long.
The 1970 British Cohort Study , which has collected data on the lives of 17,000 Brits since their births in 1970, is one well-known example of a longitudinal study .
Longitudinal studies are better to establish the correct sequence of events, identify changes over time, and provide insight into cause-and-effect relationships, but they also tend to be more expensive and time-consuming than other types of studies.
There are eight threats to internal validity : history, maturation, instrumentation, testing, selection bias , regression to the mean, social interaction and attrition .
Internal validity is the extent to which you can be confident that a cause-and-effect relationship established in a study cannot be explained by other factors.
In mixed methods research , you use both qualitative and quantitative data collection and analysis methods to answer your research question .
The research methods you use depend on the type of data you need to answer your research question .
- If you want to measure something or test a hypothesis , use quantitative methods . If you want to explore ideas, thoughts and meanings, use qualitative methods .
- If you want to analyze a large amount of readily-available data, use secondary data. If you want data specific to your purposes with control over how it is generated, collect primary data.
- If you want to establish cause-and-effect relationships between variables , use experimental methods. If you want to understand the characteristics of a research subject, use descriptive methods.
A confounding variable , also called a confounder or confounding factor, is a third variable in a study examining a potential cause-and-effect relationship.
A confounding variable is related to both the supposed cause and the supposed effect of the study. It can be difficult to separate the true effect of the independent variable from the effect of the confounding variable.
In your research design , it’s important to identify potential confounding variables and plan how you will reduce their impact.
Discrete and continuous variables are two types of quantitative variables :
- Discrete variables represent counts (e.g. the number of objects in a collection).
- Continuous variables represent measurable amounts (e.g. water volume or weight).
Quantitative variables are any variables where the data represent amounts (e.g. height, weight, or age).
Categorical variables are any variables where the data represent groups. This includes rankings (e.g. finishing places in a race), classifications (e.g. brands of cereal), and binary outcomes (e.g. coin flips).
You need to know what type of variables you are working with to choose the right statistical test for your data and interpret your results .
You can think of independent and dependent variables in terms of cause and effect: an independent variable is the variable you think is the cause , while a dependent variable is the effect .
In an experiment, you manipulate the independent variable and measure the outcome in the dependent variable. For example, in an experiment about the effect of nutrients on crop growth:
- The independent variable is the amount of nutrients added to the crop field.
- The dependent variable is the biomass of the crops at harvest time.
Defining your variables, and deciding how you will manipulate and measure them, is an important part of experimental design .
Experimental design means planning a set of procedures to investigate a relationship between variables . To design a controlled experiment, you need:
- A testable hypothesis
- At least one independent variable that can be precisely manipulated
- At least one dependent variable that can be precisely measured
When designing the experiment, you decide:
- How you will manipulate the variable(s)
- How you will control for any potential confounding variables
- How many subjects or samples will be included in the study
- How subjects will be assigned to treatment levels
Experimental design is essential to the internal and external validity of your experiment.
I nternal validity is the degree of confidence that the causal relationship you are testing is not influenced by other factors or variables .
External validity is the extent to which your results can be generalized to other contexts.
The validity of your experiment depends on your experimental design .
Reliability and validity are both about how well a method measures something:
- Reliability refers to the consistency of a measure (whether the results can be reproduced under the same conditions).
- Validity refers to the accuracy of a measure (whether the results really do represent what they are supposed to measure).
If you are doing experimental research, you also have to consider the internal and external validity of your experiment.
A sample is a subset of individuals from a larger population . Sampling means selecting the group that you will actually collect data from in your research. For example, if you are researching the opinions of students in your university, you could survey a sample of 100 students.
In statistics, sampling allows you to test a hypothesis about the characteristics of a population.
Quantitative research deals with numbers and statistics, while qualitative research deals with words and meanings.
Quantitative methods allow you to systematically measure variables and test hypotheses . Qualitative methods allow you to explore concepts and experiences in more detail.
Methodology refers to the overarching strategy and rationale of your research project . It involves studying the methods used in your field and the theories or principles behind them, in order to develop an approach that matches your objectives.
Methods are the specific tools and procedures you use to collect and analyze data (for example, experiments, surveys , and statistical tests ).
In shorter scientific papers, where the aim is to report the findings of a specific study, you might simply describe what you did in a methods section .
In a longer or more complex research project, such as a thesis or dissertation , you will probably include a methodology section , where you explain your approach to answering the research questions and cite relevant sources to support your choice of methods.
Ask our team
Want to contact us directly? No problem. We are always here for you.
- Email [email protected]
- Start live chat
- Call +1 (510) 822-8066
- WhatsApp +31 20 261 6040
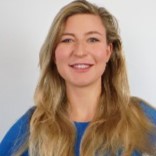
Our team helps students graduate by offering:
- A world-class citation generator
- Plagiarism Checker software powered by Turnitin
- Innovative Citation Checker software
- Professional proofreading services
- Over 300 helpful articles about academic writing, citing sources, plagiarism, and more
Scribbr specializes in editing study-related documents . We proofread:
- PhD dissertations
- Research proposals
- Personal statements
- Admission essays
- Motivation letters
- Reflection papers
- Journal articles
- Capstone projects
Scribbr’s Plagiarism Checker is powered by elements of Turnitin’s Similarity Checker , namely the plagiarism detection software and the Internet Archive and Premium Scholarly Publications content databases .
The add-on AI detector is powered by Scribbr’s proprietary software.
The Scribbr Citation Generator is developed using the open-source Citation Style Language (CSL) project and Frank Bennett’s citeproc-js . It’s the same technology used by dozens of other popular citation tools, including Mendeley and Zotero.
You can find all the citation styles and locales used in the Scribbr Citation Generator in our publicly accessible repository on Github .

10 Famous Examples of Longitudinal Studies
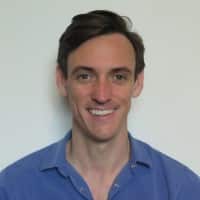
Chris Drew (PhD)
Dr. Chris Drew is the founder of the Helpful Professor. He holds a PhD in education and has published over 20 articles in scholarly journals. He is the former editor of the Journal of Learning Development in Higher Education. [Image Descriptor: Photo of Chris]
Learn about our Editorial Process
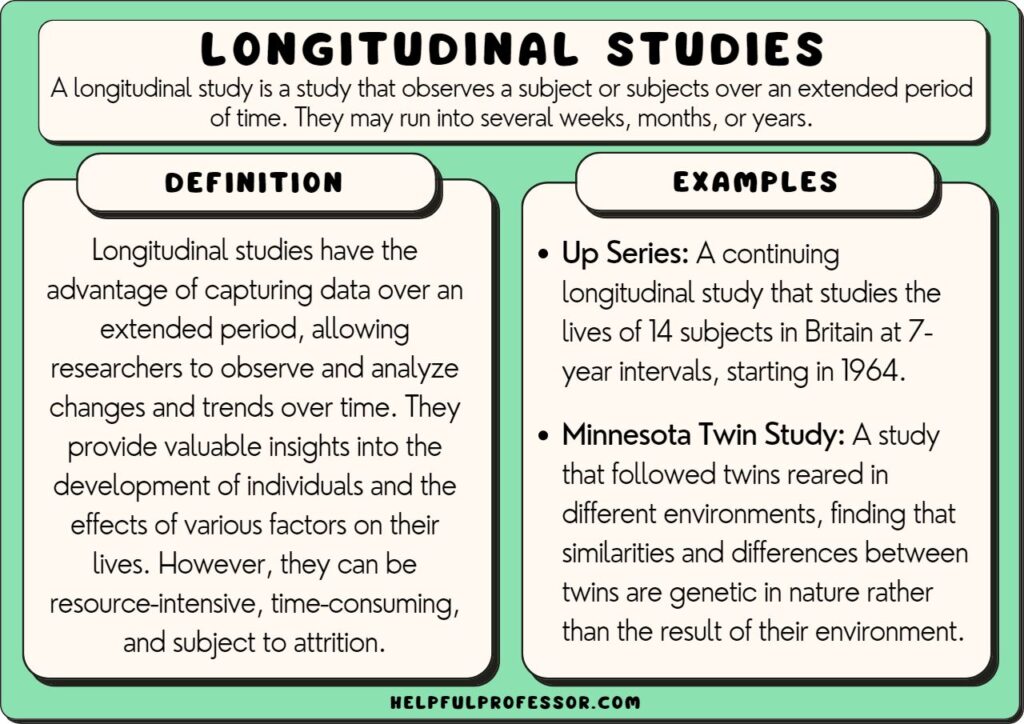
A longitudinal study is a study that observes a subject or subjects over an extended period of time. They may run into several weeks, months, or years. An examples is the Up Series which has been going since 1963.
Longitudinal studies are deployed most commonly in psychology and sociology, where the intention is to observe the changes in the subject over years, across a lifetime, and sometimes, even across generations.
There have been several famous longitudinal studies in history. Some of the most well-known examples are listed below.
Examples of Longitudinal Studies
1. up series.
Duration: 1963 to Now
The Up Series is a continuing longitudinal study that studies the lives of 14 subjects in Britain at 7-year intervals.
The study is conducted in the form of interviews in which the subjects report the changes that have occurred in their lives in the last 7 years since the last interview.
The interviews are filmed and form the subject matter of the critically acclaimed Up series of documentary films directed by Michael Apsted.
When it was first conceived, the aim of the study was to document the life progressions of a cross-section of British children through the second half of the 20th century in light of the rapid social, economic, political, and demographic changes occuring in Britain.
14 children were selected from different socio-economic backgrounds for the first study in 1963 in which all were 7 years old.
The latest installment was filmed in 2019 by which time the participants had reached 63 years of age.
The study noted that life outcomes of subjects were determined to a large extent by their socio-economic and demographic circumstances, and that chances for upward mobility remained limited in late 20th century Britain (Pearson, 2012).
2. Minnesota Twin Study
Duration: 1979 to 1990 (11 years)
Siblings who are twins not only look alike but often display similar behavioral and personality traits.
This raises an oft-asked question: how much of this similarity is genetic and how much of it is the result of the twins growing up together in a similar environment.
The Minnesota twin study was a longitudinal study that set out to find an answer to this question by studying a group of twins from 1979 to 1990 under the supervision of Thomas J Bouchard.
The study found that identical twins who were reared apart in different environments did not display any greater chances of being different from each other than twins that were raised in the same environment.
The study concluded that the similarities and differences between twins are genetic in nature, rather than being the result of their environment (Bouchard et. al., 1990).
3. Grant Study
Duration: 1942 – Present
The Grant Study is one of the most ambitious longitudinal studies. It attempts to answer a philosophical question that has been central to human existence since the beginning of time – what is the secret to living a good life? (Shenk, 2009).
It does so by studying the lives of 268 male Harvard graduates who are interrogated at least every two years with the help of questionnaires, personal interviews, and gleaning information about their physical and mental well-being from their physicians.
Begun in 1942, the study continues to this day.
The study has provided researchers with several interesting insights into what constitutes the human quality of life.
For instance:
- It reveals that the quality of our relationships is more influential than IQ when it comes to our financial success.
- It suggests that our relationships with our parents during childhood have a lasting impact on our mental and physical well-being until late into our lives.
In short, the results gleaned from the study (so far) strongly indicate that the quality of our relationships is one of the biggest factors in determining our quality of life.
4. Terman Life Cycle Study
Duration: 1921 – Present
The Terman Life-Cycle Study, also called the Genetic Studies of Genius, is one of the longest studies ever conducted in the field of psychology.
Commenced in 1921, it continues to this day, over 100 years later!
The objective of the study at its commencement in 1921 was to study the life trajectories of exceptionally gifted children, as measured by standardized intelligence tests.
Lewis Terman, the principal investigator of the study, wanted to dispel the then-prevalent notion that intellectually gifted children tended to be:
- socially inept, and
- physically deficient
To this end, Terman selected 1528 students from public schools in California based on their scores on several standardized intelligence tests such as the Stanford-Binet Intelligence scales, National Intelligence Test, and the Army Alpha Test.
It was discovered that intellectually gifted children had the same social skills and the same level of physical development as other children.
As the study progressed, following the selected children well into adulthood and in their old age, it was further discovered that having higher IQs did not affect outcomes later in life in a significant way (Terman & Oden, 1959).
5. National Food Survey
Duration: 1940 to 2000 (60 years)
The National Food Survey was a British study that ran from 1940 to 2000. It attempted to study food consumption, dietary patterns, and household expenditures on food by British citizens.
Initially commenced to measure the effects of wartime rationing on the health of British citizens in 1940, the survey was extended and expanded after the end of the war to become a comprehensive study of British dietary consumption and expenditure patterns.
After 2000, the survey was replaced by the Expenditure and Food Survey, which lasted till 2008. It was further replaced by the Living Costs and Food Survey post-2008.
6. Millennium Cohort Study
Duration: 2000 to Present
The Millennium Cohort Study (MCS) is a study similar to the Up Series study conducted by the University of London.
Like the Up series, it aims to study the life trajectories of a group of British children relative to the socio-economic and demographic changes occurring in Britain.
However, the subjects of the Millenium Cohort Study are children born in the UK in the year 2000-01.
Also unlike the Up Series, the MCS has a much larger sample size of 18,818 subjects representing a much wider ethnic and socio-economic cross-section of British society.
7. The Study of Mathematically Precocious Youths
Duration: 1971 to Present
The Study of Mathematically Precocious Youths (SMPY) is a longitudinal study initiated in 1971 at the Johns Hopkins University.
At the time of its inception, the study aimed to study children who were exceptionally gifted in mathematics as evidenced from their Scholastic Aptitude Test (SAT) scores.
Later the study shifted to Vanderbilt University and was expanded to include children who scored exceptionally high in the verbal section of the SATs as well.
The study has revealed several interesting insights into the life paths, career trajectories, and lifestyle preferences of academically gifted individuals. For instance, it revealed:
- Children with exceptionally high mathematical scores tended to gravitate towards academic, research, or corporate careers in the STEM fields.
- Children with better verbal abilities went into academic, research, or corporate careers in the social sciences and humanities .
8. Baltimore Longitudinal Study of Aging
Duration: 1958 to Present
The Baltimore Longitudinal Study of Aging (BLSA) was initiated in 1958 to study the effects of aging, making it the longest-running study on human aging in America.
With a sample size of over 3200 volunteer subjects, the study has revealed crucial information about the process of human aging.
For instance, the study has shown that:
- The most common ailments associated with the elderly such as diabetes, hypertension, and dementia are not an inevitable outcome of growing old, but rather result from genetic and lifestyle factors.
- Aging does not proceed uniformly in humans, and all humans age differently.
9. Nurses’ Health Study
Duration: 1976 to Present
The Nurses’ Health Study began in 1976 to study the effects of oral contraceptives on women’s health.
The first commercially available birth control pill was approved by the Food and Drug Administration (FDA) in 1960, and the use of such pills rapidly spread across the US and the UK.
At the same time, a lot of misinformation prevailed about the perceived harmful effects of using oral contraceptives.
The nurses’ health study aimed to study the long-term effects of the use of these pills by researching a sample composed of female nurses.
Nurses were specially chosen for the study because of their medical awareness and hence the ease of data collection that this enabled.
Over time, the study expanded to include not just oral contraceptives but also smoking, exercise, and obesity within the ambit of its research.
As its scope widened, so did the sample size and the resources required for continuing the research.
As a result, the study is now believed to be one of the largest and the most expensive observational health studies in history.
10. The Seattle 500 Study
Duration: 1974 to Present
The Seattle 500 Study is a longitudinal study being conducted by the University of Washington.
It observes a cohort of 500 individuals in the city of Seattle to determine the effects of prenatal habits on human health.
In particular, the study attempts to track patterns of substance abuse and mental health among the subjects and correlate them to the prenatal habits of the parents.
From the examples above, it is clear that longitudinal studies are essential because they provide a unique perspective into certain issues which can not be acquired through any other method .
Especially in research areas that study developmental or life span issues, longitudinal studies become almost inevitable.
A major drawback of longitudinal studies is that because of their extended timespan, the results are likely to be influenced by epochal events.
For instance, in the Genetic Studies of Genius described above, the life prospects of all the subjects would have been impacted by events such as the Great Depression and the Second World War.
The female participants in the study, despite their intellectual precocity, spent their lives as home makers because of the cultural norms of the era. Thus, despite their scale and scope, longitudinal studies do not always succeed in controlling background variables.
Bouchard, T. J. Jr, Lykken, D. T., McGue, M., Segal, N. L., & Tellegen, A. (1990). Sources of human psychological differences: the Minnesota study of twins reared apart. Science , 250 (4978), 223–228. doi: https://doi.org/10.1126/science.2218526
Pearson, A. (2012, May) Seven Up!: A tale of two Englands that, shamefully, still exist The Telegraph https://www.telegraph.co.uk/comment/columnists/allison-pearson/9269805/Seven-Up-A-tale-of-two-Englands-that-shamefully-still-exist.html
Shenk, J.W. (2009, June) What makes us happy? The Atlantic https://www.theatlantic.com/magazine/archive/2009/06/what-makes-us-happy/307439/
Terman, L. M. & Oden, M. (1959). The Gifted group at mid-Life: Thirty-five years’ follow-up of the superior child . Genetic Studies of Genius Volume V . Stanford University Press.
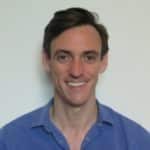
- Chris Drew (PhD) https://helpfulprofessor.com/author/chris-drew-phd-2/ 25 Number Games for Kids (Free and Easy)
- Chris Drew (PhD) https://helpfulprofessor.com/author/chris-drew-phd-2/ 25 Word Games for Kids (Free and Easy)
- Chris Drew (PhD) https://helpfulprofessor.com/author/chris-drew-phd-2/ 25 Outdoor Games for Kids
- Chris Drew (PhD) https://helpfulprofessor.com/author/chris-drew-phd-2/ 50 Incentives to Give to Students
Leave a Comment Cancel Reply
Your email address will not be published. Required fields are marked *
- Chapter 7. Longitudinal studies
Clinical follow up studies
- Chapter 1. What is epidemiology?
- Chapter 2. Quantifying disease in populations
- Chapter 3. Comparing disease rates
- Chapter 4. Measurement error and bias
- Chapter 5. Planning and conducting a survey
- Chapter 6. Ecological studies
- Chapter 8. Case-control and cross sectional studies
- Chapter 9. Experimental studies
- Chapter 10. Screening
- Chapter 11. Outbreaks of disease
- Chapter 12. Reading epidemiological reports
- Chapter 13. Further reading
Follow us on
Content links.
- Collections
- Health in South Asia
- Women’s, children’s & adolescents’ health
- News and views
- BMJ Opinion
- Rapid responses
- Editorial staff
- BMJ in the USA
- BMJ in South Asia
- Submit your paper
- BMA members
- Subscribers
- Advertisers and sponsors
Explore BMJ
- Our company
- BMJ Careers
- BMJ Learning
- BMJ Masterclasses
- BMJ Journals
- BMJ Student
- Academic edition of The BMJ
- BMJ Best Practice
- The BMJ Awards
- Email alerts
- Activate subscription
Information
Longitudinal studies
- December 2015
- Journal of Thoracic Disease 7(11):E537-40
- 7(11):E537-40

- Glenfield Hospital

- Nottingham University Hospitals NHS Trust
- This person is not on ResearchGate, or hasn't claimed this research yet.

- IEO - Istituto Europeo di Oncologia
Discover the world's research
- 25+ million members
- 160+ million publication pages
- 2.3+ billion citations
- Layanny Silva Soares
- Raphael Crhistian Fernandes Medeiros

- IEEE T MED IMAGING

- Yinlan Wang

- Benjamin Goldschneider
- Samaneh Eftekhari Mahabadi
- Reza Khalifeh

- Nafiseh Hosseini Yekta
- Shaimaa M. Magdy
- Ahmed M. Abdel Halim
- Gayathri De Lanerolle

- Athar Haroon

- NICOTINE TOB RES

- Siddharth Seth
- Daniel Felsky

- Chunyan Liu

- AM J EPIDEMIOL

- Robert S Ware
- Annette Dobson
- Thomas Royle Dawber
- L. D. Fisher
- G. Van Belle
- Michikazu Nakai
- J AM GERIATR SOC
- Anne B Newman
- Thomas R. Dawber
- William B. Kannel
- Lorna P. Lyell
- Recruit researchers
- Join for free
- Login Email Tip: Most researchers use their institutional email address as their ResearchGate login Password Forgot password? Keep me logged in Log in or Continue with Google Welcome back! Please log in. Email · Hint Tip: Most researchers use their institutional email address as their ResearchGate login Password Forgot password? Keep me logged in Log in or Continue with Google No account? Sign up
17 Longitudinal Study Advantages and Disadvantages
Longitudinal studies are a research design which requires repeated observations of the same variables over specific time periods. These may be shorter examinations or designed to collect long-term data. Under most situations, it is treated as a type of observational study, although there are times when researchers can structure them as more of a randomized experiment.
Most longitudinal studies are used in either clinical psychology or social-personality observations. They are useful when observing the rapid fluctuations of emotion, thoughts, or behaviors between two specific baseline points. Some researchers use them to study life events, compare generational behaviors, or review developmental trends across individual lifetimes.
When they are observational, then longitudinal studies are able to observe the world without manipulating it in any way. That means they may have less power to detect casual relationships that may form in their observed subjects. Because there are repeated observations performed at the individual level with this option, there is also more power than other studies to remove time-invariant differences while review the temporal order of events that occur.
The longest-running longitudinal study in the world today was started in 1921 by psychologist Lewis Terman. He wanted to investigate how highly intelligent children would develop as they turned into adults. The original study had over 1,000 participants, but that figure has dropped to under 200. Researchers plan to continue their work until there are no participants left.
These are the crucial longitudinal studies pros and cons to review before setting up this form of a panel study.
List of the Pros of Longitudinal Studies
1. This form of research is designed to be more flexible than other options. There are times when a longitudinal study will look at one specific data point only when researchers begin observing their subjects. You will also find that this option provides enough data when implemented to provide information on unanticipated relationships or patterns that may be meaningful in specific environments. Since most of these studies are not designed to be lengthy, there are more options to pursue tangents here than in other research formats.
Researchers have an opportunity to pursue additional data points which were collected to determine if a shift in focus is necessary to review a complete set of information. If there is something interesting found in the material, then longitudinal studies allow for an option to pursue them.
2. The accuracy rate of the data collected during longitudinal studies is high. When researchers decide to follow longitudinal studies to collect observational data, then the accurate rate of the information they collect is high because everything occurs in a real-time situation. Although mistakes do happen because no one is perfect, the structure and foundation of this option limits the problems that can occur. This information is also useful in the implementation of changes that may be necessary to achieve the best possible outcome during an observational period.’
3. This research method can identify unique developmental trends. When researchers pursue a short-term longitudinal study, then they are looking for answers to very specific questions. If a long-term model is developed, there is an opportunity to identify specific developmental trends that occur in various fields, including sociology, psychology, and general medicine.
Researchers using longitudinal studies have opportunities to track multiple generations in specific family groups while still collecting real-time data on all of the individuals being tracked to see how current decisions can influence future outcomes for some population demographics.
4. It allows for the consistent use of the observational method. It is a simpler process to collect information when using longitudinal studies for research because it almost always uses the observational method. This structure makes it possible to collect consistent data samples at the individual level instead of relying on extrapolation or other methods of personal identification. It is the consistency offered in this approach which provides for exclusion differences for individuals, making it possible to exclude variations that could adversely impact outcomes as it happens with other research options.
5. Longitudinal studies allow for unique a specific data points to be collected. Most research study options provide a structure where data is available over a short time period for collection, offering a small window where cause-and-effect examples can be observed. Longitudinal studies provide an option to increase the amount of time provided for researchers to collect their data, sometimes on a very dramatic scale. There are some studies which are measured in decades or centuries instead of days, weeks, or months. This process makes it possible to examine the macro- and micro-changes that can occur in the various fields of humanity.
6. This process allows for higher levels of research validity. For any research project to be successful, there are laws, regulations, and rules that must be instituted from the very beginning to ensure all researchers follow the same path of data collection. This structure makes it possible of multiple people to collect similar information from unique individuals because everyone is following the same set of processes. It creates a result that offers higher levels of validity because it is a simpler process to verify the data that is being developed from the direct observations of others.
7. There are three different types of longitudinal studies available for use. Researchers have access to three significant types of longitudinal studies to collect the information that they require. Panel studies are the first option, and they involve a sampling of a cross-section of individuals. Cohort studies are the second type, which involves the selection of a group based on specific events, such as their historical experience, household location, or place of birth.
The final option is called a retrospective study. This option looks at the past by reviewing historical information, such as medical records, to determine if there is a pattern in the data that is useful.
List of the Cons of Longitudinal Studies
1. The structure makes it possible for one person to change everything. Longitudinal studies have a robust reliance on the individual interpretations that researchers develop after making their observations. That makes it possible for personal bias, inexperience, or a mistake to inadvertently alter the data being collected in real-time situations. This issue makes it possible for the information to be invalid without researchers realizing that this disadvantage is present in their work. Even if there are numerous people involved with a project, it is possible for a single person to disrupt potentially decades of work because of their incorrect (and possibly inadvertent) approach.
2. It is more expensive to perform longitudinal studies than other research methods. This disadvantage typically applies to the research studies which are designed to take longer periods of time to collect relevant information. Because observations may last for several years (if not decades), the organizations which are behind the effort of information retention can discover that their costs can be up to 50% higher in some situations when they choose this method over the other options that are available. Although the value of the research remains high, some may find the cost to be a significant barrier to cross.
3. The information collected by researchers may have few controls. The real-time observational data that researchers collect during longitudinal studies is both informative and efficient from a cost perspective when looking at short-term situations. One of the problems that this method encounters is that the information being collected comes from a relatively small number of individuals. Unless it is built into the rules for collection, there may be no controls in place for environmental factors or cultural differences between the individuals involved.
4. It can be challenging for longitudinal research to adapt to changes. There is sometimes no follow up to identify changes in thinking or operations that occur when using longitudinal studies as the primary basis of information collection. Researchers sometimes fail to compare attitudes, behaviors, or perceptions from one point of time to another. Most people change as time passes because they have more information available to them upon which they can draw an opinion. Some people can be very different today than they were 10 years ago. Unless the structures are flexible enough to recognize and adapt to this situation, then the data they gather may not be as useful as it should be.
5. Longitudinal studies often require a larger sample size. Researchers use longitudinal studies to develop a recognition for patterns and relationships. That means there is a large amount of data that must be collected from numerous individual sources to draw meaningful connections to the topic under study. If there is not a significant sample size available to researchers for the project, then there may not be enough information available to find specific conclusions.
Even when there is enough data present for researchers to use, the sheer size of what they collect can require data mining efforts that can take time to sort out.
6. Some people do not authentically participate in longitudinal studies. As with any other form of research that is performed today, you will encounter individuals who behave artificially because they know they are part of a longitudinal study program. When this issue occurs, then it becomes challenging for researchers to sort out what the authentic and inauthentic emotions, thoughts, and behaviors are from each other. Some participants may try to behave in the ways that they believe the researchers want to create specific results.
A study by psychologist Robert S. Feldmen and conducted by the University of Massachusetts found that 60% of people lie at least once during a 10-minute conversation. The average person will lie 2-3 times during that discussion. The content of fibs varies between men and women, trying to make themselves look better or to make the person they are talking to feel good respectively. Researchers must recognize this trait early to remove this potential disadvantage.
7. Longitudinal studies rely on the skill set of the researchers. The data that longitudinal studies collects is presented in real-time to researchers, which means it relies on their individual skills to make it useful. Those who are tasked with this job must follow a specific set of steps to ensure that there is authenticity and value to what they observe. Even if you offer step-by-step guidelines on how to perform the work, two different researchers may interpret the instructions differently, which can then lead to an adverse result. The personal views of the information being collected can also impact the results in ways that are not useful.
8. The data that is collected from longitudinal studies may not be reliable. Although the goal of longitudinal studies is to identify patterns, inaccuracies in the information collected can lead to incorrect interpretations of choices, thoughts, and behaviors. All it takes is one piece of data to be inaccurate for the results to be impacted in negative ways. It is possible that the findings of the research could be invalidated by just one incorrect interpretation of a real-time result. That is why any conclusion made using this method is often taken with a “grain of salt” with regard to its viability.
9. There is a time element to consider with longitudinal studies. Researchers may find that it requires several years of direct observation before any meaningful data becomes available through longitudinal studies. Some relationships or observable behaviors may never occur even though it seems like they should, which means this time investment may never offer dividends. These studies must have the means to maintain continuously open lines of communication with all of the involved parties to ensure that the quality of the data remains high throughout the entire effort.
10. Longitudinal studies always offer a factor of unpredictability. Because the structure of longitudinal studies will follow the same individuals over an extended time period, what happens to each person outside of the scope of the research can have a direct impact on the eventual findings that researchers develop. Some people may choose to stop participating in the study altogether, which may reduce the validity of the final result when published. It is possible for some individuals or households to shift their demographic profile so that they are no longer viable candidates for the research. Unless these factors are included in the initial structure of the project, then the findings that are developed from the work could be invalid.
The pros and cons of longitudinal studies provides us with a valuable foundation of data that makes it possible to recognize long-term relationships, determine their value, and where it may be possible to make healthy changes in numerous fields. There are certain risks to consider with this process that may create unpredictable outcomes, but it is also through this research method that we will likely find new ways to transform numerous scientific and medical fields in the future.

- Login for Applicant
- Login for ICSSR Officials
ICSSR Call for Collaborative Research Proposals on Longitudinal Studies in Social and Human Sciences
- Google Plus
You are here
The Indian Council of Social Science Research (ICSSR) invites proposals for Longitudinal Studies in Social and Human Sciences. The guidelines entailing details of framework for longitudinal studies, duration of the studies, eligibility criteria, how to apply, budget, remuneration and emoluments of project staff, joining and release of grant, monitoring of research studies and other conditions can be accessed by clicking on the link ICSSR Guidelines for Longitudinal Studies in Social and Human Sciences.
Last date of submission of the application has been extended until 18 August 2024
Important Information:
Guidelines : https://icssr.org/guidelines-longitudinal-studies-in-social-and-human-sc... Link to apply : https://app.icssr.org/ Last date for submission of online form : August 12, 2024 Format for Research Proposal : Download Format for Profile of Project Coordinator : Download Format for Forwarding Letter : Download
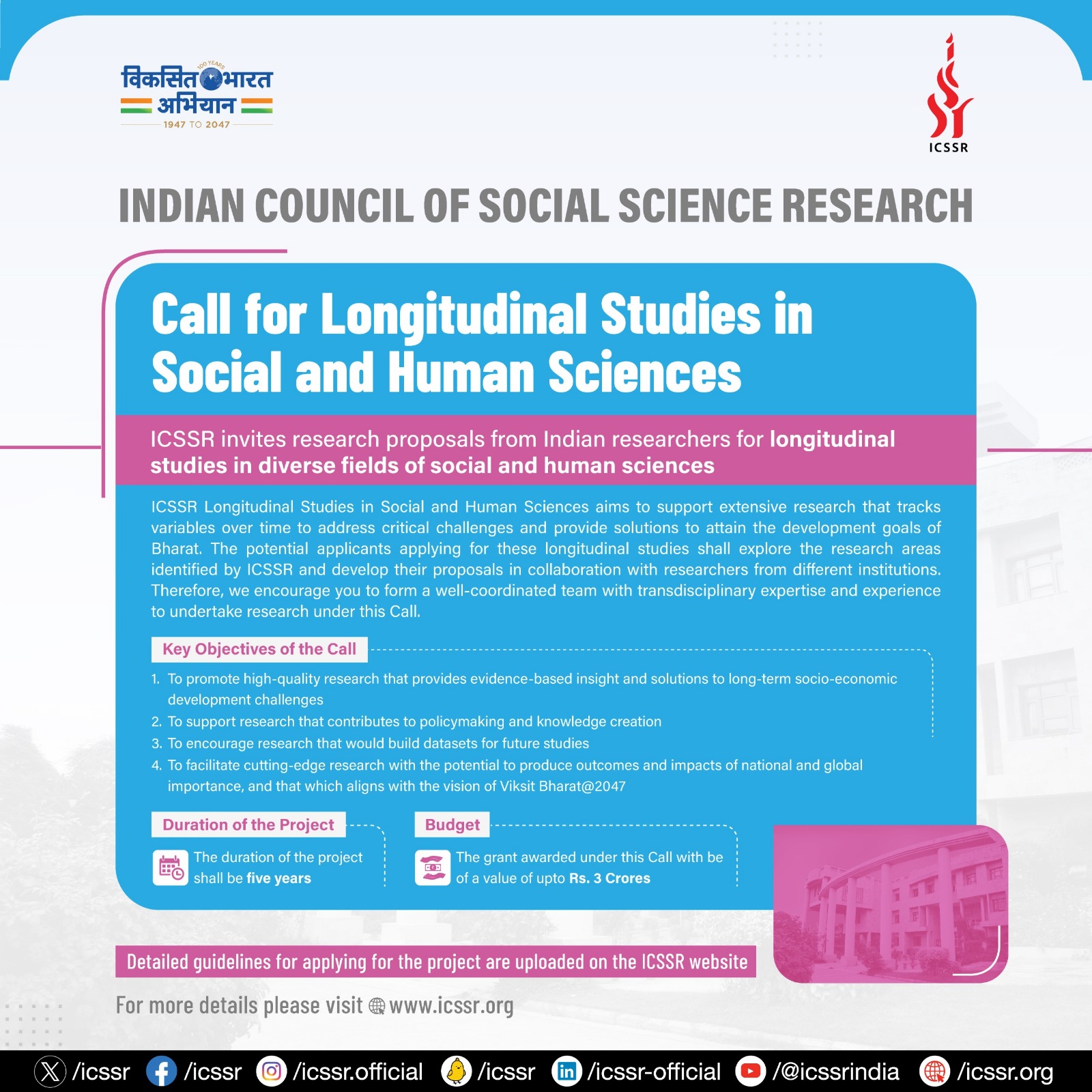
Qualitative longitudinal research in vocational psychology: a methodological approach to enhance the study of contemporary careers
- Open access
- Published: 09 August 2024
Cite this article
You have full access to this open access article
- J. Masdonati ORCID: orcid.org/0000-0002-1897-1425 1 , 2 ,
- C. É. Brazier 1 , 2 ,
- M. Kekki 1 ,
- M. Parmentier 1 , 3 &
- B. Neale 4
69 Accesses
Explore all metrics
Although temporality is pivotal to most career development processes, qualitative longitudinal research (QLR) is still rare in vocational psychology. QLR consists of following individuals over the years and exploring how they develop through time. It implies articulating themes, cases, and processes to reach an understanding of change in the making. Based on two vignettes showing how the entourage influences career change processes, we address the heuristic, praxeological, and transformative potential of using QLR in vocational psychology and, more specifically, to study career transitions. This approach also raises practical and ethical challenges that must be considered.
Bien que la temporalité soit essentielle à la plupart des processus de développement de carrière, la recherche qualitative longitudinale (RQL) est encore rare en psychologie de l'orientation. La RQL consiste à suivre des individus au fil des années et à explorer comment ils se développent dans le temps. Elle implique l'articulation de thèmes, de cas et de processus pour parvenir à une compréhension du changement en cours de réalisation. Basée sur deux vignettes montrant comment l'entourage influence les processus de changement de carrière, nous abordons le potentiel heuristique, praxéologique et transformateur de l'utilisation de la RQL en psychologie de l’orientation et, plus spécifiquement, pour étudier les transitions de carrière. Cette approche soulève également des défis pratiques et éthiques.
Zusammenfassung
Obwohl die Zeitlichkeit für die meisten Karriereentwicklungsprozesse von zentraler Bedeutung ist, ist qualitative Längsschnittforschung (QLR) in der Berufspsychologie noch selten. QLR besteht darin, Individuen über die Jahre zu verfolgen und zu erforschen, wie sie sich im Laufe der Zeit entwickeln. Dies impliziert die Artikulation von Themen, Fällen und Prozessen, um ein Verständnis für Veränderungen im Entstehen zu erreichen. Basierend auf zwei Vignetten, die zeigen, wie das Umfeld Karriereveränderungsprozesse beeinflusst, thematisieren wir das heuristische, praxeologische und transformative Potenzial der Verwendung von QLR in der Berufspsychologie und speziell zur Untersuchung von Karrieretransitionen. Dieser Ansatz wirft auch praktische und ethische Herausforderungen auf, die berücksichtigt werden müssen.
Aunque la temporalidad es fundamental para la mayoría de los procesos de desarrollo de carrera, la investigación longitudinal cualitativa (QLR) aún es rara en la psicología vocacional. QLR consiste en seguir a los individuos a lo largo de los años y explorar cómo se desarrollan con el tiempo. Implica articular temas, casos y procesos para alcanzar una comprensión del cambio en proceso. Basándonos en dos viñetas que muestran cómo el entorno influye en los procesos de cambio de carrera, abordamos el potencial heurístico, praxeológico y transformador de usar QLR en psicología vocacional y, más específicamente, para estudiar las transiciones de carrera. Este enfoque también plantea desafíos prácticos y éticos que deben considerarse.
Similar content being viewed by others
A Philosophical Consideration of Qualitative Career Assessment
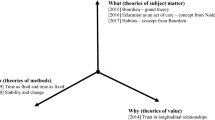
Conducting qualitative research through time: how might theory be useful in longitudinal qualitative research?
Career Adaptability, Employability, and Career Resilience in Managing Transitions
Avoid common mistakes on your manuscript.
Introduction
Sophisticated research methodologies need to be implemented to expand our understanding of contemporary careers, which are increasingly characterized by unpredictability, challenging transitions, and complex career decision-making processes (Chudzikowski, 2012 ; Fouad and Bynner, 2008 ; Lent and Brown, 2020 ; Levin and Lipshits-Braziler, 2022 ; Sullivan and Al Ariss, 2022 ). While a longitudinal approach is progressively considered essential in quantitative careers studies in psychology (Akkermans et al., 2021 ; Rudolph, 2021 ), qualitative longitudinal research (QLR; Neale, 2021b ) is rare, although it has been used to good effect in sociology, for example, in studies of the career trajectories of young people (e.g., Bidart et al., 2013 ), those in mid to later life (e.g., Hermanowicz, 2009 ), or people undergoing welfare to work interventions (e.g., Danneris, 2018 ).
In the present methodological paper, we stress the relevance and challenges of implementing QLR in vocational psychology Footnote 1 to study lifelong career development and, more specifically, career transitions. First, we make the case for QLR in vocational psychology by stressing the temporal nature of contemporary careers, describing how research in vocational psychology traditionally addresses time, describing the main features and procedures of QLR, and highlighting its value for studying career development and transitions. Second, the potential significance of QLR is illustrated through the presentation of vignettes from two participants in a study on relational influences on involuntary career change. Third, we provide an overview of the strengths and challenges of conducting QLR in vocational psychology.
Making the case for qualitative longitudinal research in vocational psychology
The temporal nature of career development.
Temporality “refers to the state of existing in time” (Olry-Louis et al., 2022 , p. 257) and encompasses “everything that relates to time, whether it is the perception of simultaneity, succession or duration, of past/present/future, or how the individual experiences specific moments of time” (p. 258). Temporality is at the core of most career-based behaviors and is inherent in most theories of vocational psychology, such as the life-span, life-space approach to careers (Super et al., 1996 ), career construction theory (Savickas, 2020 ), and systems theory framework of career development (Patton and McMahon, 2015 ). More specifically, it is a key factor in career decision-making, one of the main tasks within career development, which implies a thorough self-awareness and the ability to anticipate the future and to project into possible selves (Gati and Levin, 2015 ; Ibarra and Petriglieri, 2010 ; Lent, 2013 ). Future temporality, as seen in the present situation, is thus of major importance.
Coping with career transitions is another crucial, inherently temporal career-based task (Olry-Louis et al., 2022 ; Sullivan and Al Ariss, 2022 ). A successful transition involves not only being able to tackle the challenges of the ongoing change but also moving on from the past and integrating a new work situation while preserving a minimum of continuity over time (Kulkarni, 2020 ). Thus, the identity work implemented in such a transition consists of maintaining continuity of self despite the career change, which involves articulating the future with the past (Zittoun, 2009 ). Coping with a transition also often involves parallel challenges in other life spheres (e.g., health and family), which have their own rhythms and may be more or less synchronized with the individual’s career (Perkins et al., 2023 ).
Within contemporary careers, temporality is not only central but also underlies challenging processes. Career decision-making has become an incredibly complex task, as the process of anticipating a career is hampered or even rendered irrelevant by a labor market that is constantly, unpredictably, and rapidly changing (Lent, 2013 ; Lent and Brown, 2020 ; Levin and Lipshits-Braziler, 2022 ). A volatile socioeconomic context also makes it difficult to cope with career transitions, which tend to become less predictable and more frequent (Chudzikowski, 2012 ; Sullivan and Al Ariss, 2022 ). For example, unexpected career transitions prevent workers from preserving self-continuity by anticipating possible selves consistent with past and present selves (Brazier et al., 2024 ; Conroy and O’Leary-Kelly, 2014 ). In addition, having to cope with repeated career transitions can jeopardize workers’ health and overall life satisfaction (Udayar et al., 2024 ).
Time-sensitive research in vocational psychology
Given the temporal challenges that characterize contemporary careers, implementing time-sensitive research becomes crucial in vocational psychology (Dlouhy and Biemann, 2017 ). Temporality can be researched retrospectively or prospectively (Audulv et al., 2023 ; Neale, 2021a ; Olry-Louis et al., 2022 ). Retrospective studies aim to understand the present situation in light of past experiences; prospective studies involve tracking the development of participants through “real” time. Both quantitative and qualitative methods can be implemented to address temporal questions, be it retrospectively or prospectively. While quantitative methods are nomothetic and pinpoint generalizable trends and causal patterns, qualitative methods are idiographic and enable researchers to delve deeper into processes, individual experiences, and subjective understandings of causality (Blustein et al., 2005 ; Neale, 2021a ; Ponterotto, 2005 ).
In the field of vocational psychology, quantitative research is dominant, with longitudinal quantitative designs being increasingly prevalent in studying career transitions (Akkermans et al., 2021 ; Akkermans et al., 2024 ). Qualitative research is still marginal in the field, even if it becomes more visible (Heppner et al., 2016 ; Richardson et al., 2022 ; Stead et al., 2012 ). For example, in their meta-study of articles published by the Journal of Career Development between 2000 and 2019, Mehlhouse et al. ( 2023 ) stressed that the ratio of qualitative papers was 20%, one of the lowest among counseling journals. Moreover, existing qualitative research in vocational psychology is merely cross-sectional. For example, all the qualitative papers published in the Journal of Career Development and the International Journal for Educational and Vocational Guidance in 2023 were based on cross-sectional studies. These works provide a fine-grained picture of the vocational processes at play and support the heuristic value of an idiosyncratic approach to career development. At the same time, the sole focus on cross-sectional designs prevents us from understanding career development experiences as they unfold (George et al., 2022 ; Neale, 2021b ).
Recent literature reviews tentatively suggest that more qualitative studies should be conducted to better grasp career decision-making (Richardson et al., 2022 ) and contemporary career transitions (Sullivan and Al Ariss, 2022 ). In particular, QLR is called upon to follow individuals throughout their career transitions (Akkermans et al., 2024 ; Sullivan and Al Ariss, 2022 ). The value of such an approach has been recognized across disciplines, with QLR now being a well-established methodology, for example, in health and nursing research (e.g., Audulv et al., 2023 ; Pinnock et al., 2011 ; SmithBattle et al., 2018 ). Footnote 2 Of particular relevance are a range of studies that have explored career transitions and trajectories in disciplinary contexts other than vocational psychology. In youth research, for example, QLR has been used to trace the career trajectories of young people and their transitions from school to work (e.g., Bidart et al., 2013 ; Hodkinson et al., 1996 ), while the trajectories and changing fortunes of older workers and job seekers have been traced through time in a variety of ways. In sports psychology, Torregrosa et al. ( 2015 ) investigated athletes’ transitions to retirement by interviewing participants before and 10 years after retirement. Hermanowicz ( 2009 ) used a similar follow-up approach, whose longer-term re-study traces the careers of academic scientists over a decade of change. Keskinen et al. ( 2023 ) used a more intensive processual design over a shorter time span to understand the shifting strategies employed by older job seekers to overcome perceived ageism in the labor market. Finally, social policy researchers have used QLR to good effect to trace the changing fortunes of poorly resourced people who are subjected to increasingly punitive welfare-to-work programs (e.g., Danneris, 2018 ; Neary et al., 2021 ; Patrick, 2017 ) or have explored how particular groups (e.g., single mothers or young fathers) cope with the everyday challenges of balancing precarious work and experiences of poverty with their caring responsibilities (Millar, 2007 ; Neale and Davies, 2016 ). Many of these studies explore and illuminate the intersection of career trajectories with other life factors (e.g., socioeconomic, relational, geographical, and age-based) (e.g., Neale and Tarrant, 2024 ).
However, despite these widespread developments across disciplines, QLR is overlooked in vocational psychology. This is surprising given the temporal nature, unpredictability, and changeability of contemporary careers (Chudzikowski, 2012 ; Fouad and Bynner, 2008 ). Yet vocational psychology would benefit from implementing QLR to better understand career issues and processes and the intricate dynamics of career transitions (Akkermans et al., 2021 ; Sullivan and Al Ariss, 2022 ). Indeed, although, similar to any qualitative approach, QLR does not allow for the generalization of results, it provides a distinctive lens through which to explore individuals’ experiences of change and unveil the intrinsically subjective nature of vocational processes.
Characteristics and analytical strategies of qualitative longitudinal research
QLR consists of analyzing qualitative data collected longitudinally. It aims “to look forward, prospectively, and backward, retrospectively, to give a detailed, processual understanding of change in the making” (Neale & Tarrant, 2024 , p. 53). Most QLR involves following individuals or groups over the years and exploring how they develop through time. According to Neale ( 2021b , Neale and Tarrant, 2024 ), QLR is processual in nature and implies moving from “pictures” to “movies,” asking “how” things work or change instead of “what” works and changes. This approach allows researchers to understand how continuities and changes are negotiated and experienced and to study the fluidity of temporal processes at play (Neale, 2021a ). Temporal processes and the dynamics of change can be examined either intensively (through dense data collection over relatively short periods) or extensively (through more distant data collection over more extended periods) (Audulv et al., 2023 ; Neale, 2021b ). Whether intensive or extensive, QLR involves moving beyond comparing two (or more) snapshots in time to examine different outcomes at times A and B. Rather, it aims to understand how and why individuals move from A to B, highlighting the changes in perception and identity that accompany and intersect with concrete changes in circumstances and practices (Neale, 2021b ; Zittoun, 2009 ). This brings new perspectives into the complexities of causal processes, revealing their relational, fluid, and multiple dimensions (Dall and Danneris, 2019 ; Neale, 2021a ).
QLR is considered a general and flexible approach to research that can be operationalized differently depending on each study’s specific paradigm and research question (Audulv et al., 2023 ; Neale, 2021b ). For this reason, QLR can be aligned with other qualitative approaches, such as interpretative phenomenological analysis (Farr and Nizza, 2019 ; McCoy, 2017 ) and thematic analysis (Neale, 2021b ). The analytical logic that shapes QLR—from devising research questions and generating data, to data analysis and the presentation of findings—revolves around an iteration between case, thematic, and processual insights (Audulv et al., 2023 ; Neale, 2021b ). This iteration, which recognizes the fluidity of processes, has been promoted as a central tenet of QLR methodology (Neale, 2021b ). However, the way this iteration occurs is flexible across different studies. As both Neale and Audulv et al. show, how and to what extent these analytical facets are linked together in practice, what priority is accorded to them, and over what time scales vary greatly.
Taking up this theme in their scoping review of circa 300 QLR studies, Audulv et al. ( 2023 ) separate three broad modes of longitudinal analysis. The first concerns studies with a low utilization of longitudinal data. The longitudinal elements of time and change are commonly subordinated to an overly thematic analytical focus and may be lost sight of when data are analyzed and findings presented. Given these limitations, the authors suggest that such studies should be given a separate identity beyond the QLR label. The second mode refers to studies structured according to chronological (linear) time (including recurrent cross-sectional or time series studies) that enable comparison between snapshots taken at different points in time. The third is the most fully developed QLR studies, for they take a processual (through-time) approach to the analysis of QLR data and recognize that beyond a chronological reconstruction, processes are inherently fluid and unpredictable, and causality is necessarily complex (Neale, 2021a ). Within this more rounded processual approach, the study of longitudinal cases, themes, and processes may be separated or combined creatively, not least through a cumulative and incremental process of knowledge building through time itself.
Beyond the prevailing structural principle for addressing time and change, any qualitative longitudinal analysis can be considered to involve four steps—the emphasis being placed on one or other of these steps depending on the type of research question: (1) case description (e.g., pen portraits or case profiles summarizing each case and how it unfolds through time), (2) within case comparison (e.g., grid analyses across time for each case exploring converging or diverging trajectories), (3) within case process tracking (e.g., mapping the processes for each case), and (4) cross-case process analysis (e.g., comparing and grouping similar configurations of processes across cases) (Brazier et al., 2023a ; Neale, 2021b ). Processes addressed in the third and fourth stages can be described and portrayed according to several questions (Saldaña, 2003 ), such as: What is constant, consistent, or recurring over time? What increases, emerges, or is cumulative over time? What diminishes, ceases, or is missing over time? What is idiosyncratic across time? How is the experience of temporality characterized? What are the rhythms and the peaks at play?
Toward qualitative longitudinal research in vocational psychology
As we have seen, studies from disciplines other than vocational psychology confirm that QLR is a powerful way to explore career issues and dynamics. Specifically, QLR seems relevant to study life and career transitions (Treanor et al., 2021 ). QLR entails collecting data with people undergoing a transition to understand their lived experiences and retrospective meaning-making of what contributed to the transition. Follow-up interviews yield insights into the processual development of the transition. In addition to an objective, factual development, QLR addresses “narrative change,” that is, “the unfolding of individual stories across time” (Vogl et al., 2018 , p. 178). Such interviews can also help to explore retrospectively how the transition narrative evolves through time. In this case, the focus is on “participants’ reinterpretation of experiences or feelings that they described earlier” (p. 178). Nevertheless, in vocational psychology, little qualitative research has focused on the development and progress of career transitions nor on how meaning-making evolves through time (Sullivan and Al Ariss, 2022 ).
More specifically, relevant longitudinal research questions around career transitions can be framed in relation to thematic, case, and processual investigation strategies (Audulv et al., 2023 ). For example, a longitudinal themes approach could help understand the evolving experiences of labor market integration processes by comparing young adults’ expectations of entry into the labor market with their concrete integration experience—which can be considered repeatedly during the first months or years of employment. A longitudinal case approach could consist of building a typology of career trajectories following a significant event, such as parenthood, forced inactivity, ill health, or a return to training. A longitudinal process approach could lead to the description of the process and common phases of leaving the world of work and entering retirement or unemployment. While there is no set order for building an analytical strategy, a full QLR leading to a processual analysis might take time to build, starting with a thematic analysis after the first wave, building case and cross-case analyses incrementally after each wave and culminating in a processual analysis as the fieldwork comes to an end. In this way, layers of insight are built up over time.
An illustration: investigating career change processes
To illustrate the relevance of applying QLR in vocational psychology, we draw on an ongoing two-phase, eight-year research program on involuntary career change in Switzerland (Swiss National Science Foundation fundings 100019_192429 and 10001_227634). This program was founded on the insight that little research exists on unexpected and unintentional career transitions (Akkermans et al., 2024 ; Sullivan and Al Ariss, 2021 ) and that the rare studies on involuntary career change addressed a specific population (e.g., injured veterans, Kulkarni, 2020 , or athletes, Chen and Bansal, 2022 ). However, involuntary career change—i.e., an unintentionally triggered move to a new occupational field—needs to be better understood, as it can prove to be a challenging career transition. Indeed, workers forced to change careers face several individual, environmental, and institutional barriers (Fouad and Bynner, 2008 ). Based on these observations, our research aimed to understand how involuntary career change experiences unfolded while also considering the relational influences underlying these experiences. During the first phase of the research program, over a 2-year period, we carried out three waves of interviews with three groups of workers: those who had been forced to change careers because of physical or mental health problems, unemployed people in declining occupational sectors, and migrant job seekers whose qualifications were not recognized in Switzerland.
A cross-sectional analysis of relational influences on involuntary career change
At the end of the first wave of interviews, we conducted a series of cross-sectional analyses focusing on the interpersonal aspects of career transitions (Masdonati et al., 2022 ). Through thematic analysis (Braun and Clarke, 2019 ) of participants’ recollections of their career change process, we showed that relational influences could be divided into three sources (i.e., from personal environments, support structures, or organizations) and take three forms (i.e., positive, negative, or ambivalent). Moreover, participants retrospectively indicated that relational influences operated in distinct ways depending on whether they were leaving their former occupation, shifting from the former to a new occupation, exploring new career options, or implementing a new career plan.
Among the most salient results, we showed that relational influences can involve two forms of ambivalence (Masdonati et al., 2022 ). The first form is situated ambivalence and refers to the fact that the exact source of influence could both support and hinder the career change process. For example, for some career changers, institutional influences were simultaneously a barrier regarding rules rigidity and a resource, thanks to the support of committed career professionals. Conversely, ambivalence can also be temporal, referring to the insight that the exact source of influence could have changing effects over time. For example, some participants reported that their close ones initially hindered the career change process and became more supportive as the process progressed.
Nevertheless, because of the lack of longitudinal data, these retrospective perspectives did not allow us to explore the temporal ambivalence in-depth and at the moment of its operation. Moreover, while collecting data for the second and third waves of interviews, it became clear that the narratives about relational influences fluctuated, sometimes even radically changing. Longitudinal analyses seem, therefore, the best way of gaining a finer understanding of the possible shifting relational influences on the processes of involuntary career change and how they are experienced as they occur. We illustrate the potential of this type of analysis by reporting on the evolution of relational influences from the personal environment of two participants, Jean and Josefa, having both been interviewed three times over two years. The cases were consensually selected by the authors of the present paper, since they vividly illustrated distinct evolutions in relational influences. The first two authors conducted a draft temporal thematic analysis (Neale, 2021b ) to identify the main processual threads of each case. Without intending to provide a comprehensive analysis of study data, these cases are designed to illustrate the relevance of collecting and analyzing longitudinal qualitative data to uncover new insights.
The case of Jean
When we met him for the first interview, Jean was 31 years old and had learned that he could not continue being a truck driver due to an accident that prevented him from working in a seated position. With the support of public invalidity insurance, he was considering a career change toward the security sector. The striking finding regarding Jean’s relational influences is that, at the beginning of the career change process, the persons closest to him, particularly his partner, did not understand him. Jean felt he was being judged and was considered lazy in dealing with his career change. This relational tension led him to fear a break-up with his partner, to isolate himself, and not to share his difficulties with her and his close friends: “I’m not the one who’s going to spontaneously talk about it. If someone asks me, I’m happy to talk about it, but I’m not going to call up my mates and say, ‘I’ve got a training course.’ I don’t really feel like getting excited until it’s concrete.”
When we met him 1 year later for the second interview, Jean had completed a short training and found a fulfilling position as a security manager. He reported that the tensions with his close relationships, particularly his partner, eased as he implemented his career plans, “She admits that she was harsh and not fair, that now when she sees what’s happening now, how happy I am, she totally screwed up on her behavior.” His partner and friends were now emotionally supporting him in his efforts, “Once I started working, quite the opposite, it was total support.” Consequently, Jean no longer felt ashamed to share his situation with others. However, his entourage tended to attribute the success of Jean’s career change to luck and not to his efforts, which irritated him.
At the third interview, Jean’s situation had stabilized; he had obtained a full-time permanent contract in a job that suited his limitations. Generally satisfied, he was still concerned about the after-effects of his accident, which were still present, and the fear of experiencing overwork. In terms of his relationships with his close circle, the patterns identified in the second interview were further consolidated. He saw his family as an important resource and was increasingly able to talk about his career change experience. This resulted in him finding it easier to share his experiences in general and also, advocating for a better social representation of disability insurance and its beneficiaries:
Now that it’s classified, I talk about it because I’m no longer ashamed to say where I am now, I’m working for such and such, and that’s that. And if people ask me questions about what, when, and how, well, I explain that too because, once again, I want to improve the image of disability insurance.
Finally, as in the second wave of interviews, he still sometimes felt a certain judgment on the part of his entourage: “I talk about it a lot more freely; after that, I always have a bit of a twitch in my eye when people say to me: ‘ah but you were lucky then.’”
The case of Josefa
When we interviewed Josefa for the first time, she was 35 years old, married, and mother of one child. She had arrived from Western Europe in Switzerland 7 years before and worked as a cleaner, then as a housekeeper in a private clinic and a hotel. She suffered burnout and had chronic health issues preventing her from continuing to work in her field. Having been made redundant, she was unemployed and aspired to retrain as an administrative assistant. Her husband was supportive and understanding, telling her that work is less important than health. She also used to talk a lot about her situation with her mother. She reported her son wanting to help her, “They completely understand the difficulties I have on a day-to-day basis with my health, and if I find a job that I like and that I can do without any problems, that would be a relief for them too.” Feeling support was important to her since it helped “to cope with the ups and downs of life.”
During the second interview, Josefa reported that she was neither officially unemployed nor on disability insurance and that her doctor recommended that she apply for disability funding. She mentioned her loneliness and her sadness for not being able to have normal family activities, which her son reminded her of repeatedly during the past year. She experienced both being a burden and feeling guilty for not having a salary, “If I earned a little money for the house, I’d feel more useful too, not to overload my husband, for example with, only with his salary.” She no longer spoke to her family, except to her husband, because they were not able to understand her pain and her challenging experience: “Even we don’t understand our bodies sometimes. How are people going to understand? How if they don’t have it, they’ve never experienced it in their lives?” On the other hand, she had recently joined a peer support group, where she found compassion and mutual support.
At time 3, she was still awaiting a disability insurance decision and could not engage in any professional activities for the past year. She found it very hard to cope with being out of work. However, she described being in an emancipatory process in which she could redefine her needs and limits and assert herself. As a consequence, she was less reluctant to talk about health issues and their impacts: “I used to feel a bit ashamed of explaining to people that I had a few limits, or whatever. And now, for example, I was at the wedding and said, ‘I don’t feel well’ if I didn’t feel well.” She also became very involved in the peer support group and hoped to raise awareness of the issues.
Toward a longitudinal understanding of relational influences on career change
These vignettes can result in different observations, depending on whether researchers focus their analytical attention primarily on themes, cases, or processes. A thematic longitudinal focus means pinpointing key themes within both vignettes and their evolution. This would lead to the statement, for example, that the sources and forms of Jean’s and Josefa’s relational influences were diversified. In both cases, the partner seemed to play a pivotal and enduring role, whereas other sources of influence from the personal environment were more volatile. While the partners’ role remained central throughout the process, the form of their influence varied through time: they could be perceived as much support as an obstacle (notably by making judgments or pressuring the career changers). The experience of the change process thus appears to be firmly and constantly dependent on the validation from partners.
A case-centered longitudinal focus would prioritize the comparison of the two vignettes. At Time 1, Josefa was better supported than Jean, who experienced his personal environment as more of a barrier. However, their situation evolved distinctively: Josefa felt her family began to fail to understand her struggle and pain. In contrast, Jean felt people close to him increasingly supported him while having been “suspicious” at time 1. The unfolding of the career change situation and the passing of time seem to have operated differently. Time “worked in Jean’s favor,” allowing those around him to gradually understand the complexity of his career change process. This gradual awareness was doubtless facilitated by the fact that Jean could activate himself and find a satisfying new occupation. On the contrary, time “worked against” Josefa. For example, her family was initially empathetic to her difficulty in coping with multiple health issues and changing careers. Yet, this empathy seems to fade as time goes by and Josefa does not integrate back into the labor market. This progressive incomprehension can be attributed to her stagnant career change process, where nothing changes for 2 years. Thus, in Josefa’s case, relational influences move from an understanding attitude to one of incomprehension, leading her to reduce the circle of people she could open up to and seek authentic support elsewhere (i.e., in a group of peers). Time seems to have had a progressive filtering-displacement effect, while in Jean’s case, time had a settling effect over the three study waves.
Finally, a process-oriented focus would indicate that, beyond these distinct developments, common processes appear to characterize the relational dynamics surrounding the unfolding of Jean and Josefa’s career change. In both cases, it seems that the initial reactions of their entourage did not completely fade with time, as if there were some sort of residual relational influences. In Josefa’s case, this can be seen in her recognition of her husband’s central and constant supportive role; in Jean’s case, there is still a hint of judgment in his perception of how his entourage sees his career instability over the years. Moreover, although each in their way and rhythm, Jean and Josefa have gone from an initial period of suffering, reflected in self-isolation and feelings of shame, to a phase of self-affirmation and empowerment. Indeed, 2 years after initiating the career change process, Jean advocated the importance of disability insurance support, while Josefa took an increasingly leading role in her peer support group.
Overall, this illustration shows that the added value of a qualitative longitudinal analysis lies in its potential for understanding how the impact of others on involuntary career change unfolds and through which underlying processes. These findings, if echoed in the narratives of other participants, could indicate the usefulness of the support of personal entourage in implementing a new career plan as a source of reinforcement for a process already oriented toward resolving the career change. Conversely, the role of this entourage has its limits and seems more fragile when the person is stuck in a liminal state and does not give the impression of advancing in the process. For Jean, this period was short but left behind some frustrations; for Josefa, this period was prolonged, leading her to look elsewhere for the support she lacked from her entourage.
This observation on the impact of others on involuntary career changes would have relevant implications for research in vocational psychology and career guidance and counseling practices. Implications for research would consist, for example, of stressing that the entourage of adults in transition can be affected by their transitional journey and that its influence fluctuates depending on the evolution and length of the career change process. Such findings would provide a more dynamic understanding of the relational and interpersonal elements that impact career transitions. In terms of implications for practice, these results would suggest the importance of providing institutional support when the personal environment may be less helpful, for example, in the complex and prolonged process of grieving the loss of one’s former occupation, finding and implementing a new career plan. Group interventions that include the entourage and aim to consider its point of view and raise awareness of its key role would also be practical implications arising from these results.
Potentials and challenges of implementing qualitative longitudinal research in vocational psychology
In addition to its relevance to address temporal processes in vocational psychology, QLR has several distinctive strengths that make it a methodological approach with significant potential for the study of career transitions. However, the implementation of QLR in vocational psychology is not without its drawbacks and challenges.
Heuristic, practical, and transformative strengths
Implementing QLR would be beneficial for at least three reasons, resulting from three forms of strengths of this methodological approach: heuristic, practical, and transformative. Concerning the heuristic power of QLR, the illustration of the fluctuating influences of the entourage on Jean’s and Josefa’s career transition process is just one example of the spectrum of potential new perspectives on vocational issues that emanate from QLR. Indeed, QLR provides a subtle understanding of the processual and dynamic features of a career transition (Akkermans et al., 2021 ; George et al., 2022 ; Sullivan and Al Ariss, 2022 ), captures the salience of temporality and shows how narratives about the experience of change evolve—or not—over time (Brazier et al., 2023a ; Neale and Davies, 2016 ). As a result, QLR can provide a more accurate picture of what transitions mean and how they unfold. In this sense, it has a double complementarity with prevailing methodologies in the field. First, it adds a longitudinal dimension to cross-sectional qualitative research and complements it by integrating the question of time and change into the analysis of career experiences. The illustration presented above on the unfolding of relational influences on involuntary career change is an example of such a contribution. Second, QLR adds a qualitative dimension to longitudinal quantitative research with significant explanatory power; it enables an idiosyncratic and granular understanding of what may explain general trends within career development (Akkermans et al., 2024 ). For example, the results of person-centered research on career paths (e.g., Udayar et al., 2024 ) could be enhanced with qualitative data within a mixed-method study to understand how people experience different types of career trajectories.
As for the practical power of QLR, a plethora of implications for career guidance and counseling could naturally emerge from qualitative longitudinal studies. Based on the Audulv et al. ( 2023 ) typology, the results of a QLR prioritizing a themes approach may serve to identify the salient areas of a transition experience on which it is most important to concentrate support. Findings from a case approach of QLR would enable support to be tailored to the types of career trajectories counselees undertake. Studies prioritizing a process approach would help identify the most appropriate moments for counseling and yield rich insights into how lived experiences of career transitions mesh with organizational and institutional processes. Overall, the fact that QLR shows that career transitions take time to unravel and underpin demanding experiences over time is a strong argument for advocating long-term career interventions.
Concerning the transformative power of QLR, engaging in such research is already transformative for participants (Thomson and Holland, 2003 ). It is acknowledged that participants in qualitative studies gain from sharing their experience with a nonjudgmental interviewer interested in their subjective journey without any material or social desirability concerns. In this regard, it is worth considering a question raised by Birch and Millar (2000), “Can the invitation to narrate past and present experiences, together with future hopes, avoid offering potential therapeutic opportunities?” (p. 189). This opportunity for narration and self-reflexivity is even stronger in QLR because the same researcher usually conducts several interviews, and interviewers integrate past interview contents into new ones (Thomson and Holland, 2003 ). Consequently, in these settings, a bond of trust can grow over time, and the researcher might endorse the dual role of researcher and career counselor—as suggested by Fleet et al. ( 2016 ) for research on clinical psychology interventions. These conditions eventually facilitate awareness and new insights into the transition challenges and create a safe space for repeated shared moments that can transform a person’s relationship with their transitional experience. Such scenarios are corroborated by contemporary research and approaches that stress the relevance of narrative interventions in career guidance and counseling (Rossier et al., 2021 ; Savickas, 2019 ).
The challenges of qualitative longitudinal research in vocational psychology
According to Neale ( 2021b ), beyond its many strengths, conducting QLR also involves several challenges. First, efforts must be made to maintain contact and a bond with participants to ensure a high participation rate over time (Solomon et al., 2020 ). Second, analyzing data over several points in time, articulating cases, themes, and processes, and addressing evolving research questions turns out to be complex and calls for iterative adjustments of analysis strategies (Neale and Tarrant, 2024 ; Vogl et al., 2018 ). Third, QLR implies a longitudinal ethic, consisting of establishing researcher–participant reciprocity, maintaining professional boundaries, and designing an ethical closure for the study. Fourth, since it extends over time, QLR requires more resources than cross-sectional studies (Vogl et al., 2018 ). Fifth, the risk of data overload (Saldaña, 2003 ) and extended data collection require careful design of data management to facilitate data comparisons and connections through time so that researchers have time to conduct analyses between waves (Solomon et al., 2020 ). Sixth, there is a risk that the results will come out too late to address the original research or social problem. While the last three challenges relate similarly to QLR in vocational psychology and QLR in any other field, the first three challenges underpin additional issues specific to the field, calling for targeted methodological strategies.
First, maintaining a high participation rate over time can be particularly difficult when studying career transitions. Indeed, such transitions sometimes involve a change of living place and contact details (phone number or e-mail). Moreover, transition processes can be demanding, which can demotivate participants to make time for long-lasting interviews. Self-selection processes can also be insidious (Thomson and Holland, 2003 ), with participants who have difficulty managing their transition possibly tempted to withdraw from the study, in contrast to those with a smooth transition path. This entails the risk of accessing only successful stories and thus gaining a partial picture of the issues involved in a career transition. A range of strategies can be considered to reduce the risk of study withdrawal, such as engaging in systematic debriefing with participants at the end of each interview, being attentive and sensitive to participants’ emotional states and maintaining contact with them between waves of data collection (e.g., by sharing interim reports, cf. Brazier et al., 2023b ). Regarding self-selection, ensuring transparency about the project’s aims and being explicit about the interest in accessing any transitional experience, whether successful or not, is paramount. The therapeutic value of QLR fieldwork (Thomson and Holland, 2003 ) can also encourage enduring commitments from the most vulnerable participants. Involvement in the research may give them a voice and a sense of usefulness and represent relief from loneliness.
In addition, having to iteratively adjust the analytical strategy in order to address evolving research questions is another major challenge of QLR in vocational psychology because of a scientific context where qualitative researchers are often expected to rely on preestablished templates. Footnote 3 Justifying analytical flexibility can, therefore, prove complex in a field that is not necessarily accustomed to such an approach. In fact, the challenge of moving away from “rigid sets of procedures” is not specific to qualitative research in vocational psychology but concerns psychology in general (Levitt et al., 2017 , p. 6), as well as nursing (SmithBattle et al., 2018 ) and rehabilitation studies (Solomon et al., 2020 ). Demonstrating the relevance of methodological and analytical flexibility is essential to overcome this risk. The recent articles by Pratt et al. ( 2022 ) and Richardson et al. ( 2022 ) supporting the value of “methodological bricolage” in guaranteeing trustworthiness for qualitative organizational studies and careers research are exemplary in this sense.
Finally, the longitudinal ethic for QLR in vocational psychology involves at least two specific features, the first being the threat to confidentiality. Indeed, access to potential participants in a vocational psychology study is often gained through public or para-public institutions offering diverse forms of support to the target population. In exchange, this usually involves sharing results with these institutions (e.g., Brazier et al., 2023b ). In these exchanges, finding the right balance between reporting results that are embodied enough to be meaningful to professionals but sufficiently anonymized to prevent participants from being identified can be problematic. To ensure that participants feel free to share their experiences without fearing repercussions on the support they receive, particular care must be taken when disseminating QLR results. The second ethical challenge that seems salient for QLR in vocational psychology refers to the blurred boundaries between the roles of researcher and counselor. As researchers in vocational psychology are often also trained in career guidance and counseling, they may be tempted to “switch hats” when confronted with accounts of difficult experiences and engage in counseling to support participants needing help. If, as several qualitative researchers maintain (e.g., Birch and Miller, 2000 ; Fleet et al., 2016 ), playing a dual role is not problematic in itself, it is crucial to establish precise rules to gather insightful data while promoting participants’ wellbeing (e.g., Thomson and Holland, 2003 ). For example, role changes during the interview should be made explicit with the participants. Discussions within the research team are also needed to ensure that how these two roles are understood is appropriate and adjusted on a case-by-case basis and to define the limits beyond which it is advisable to refer to other professionals (e.g., psychotherapists or social workers). As with any qualitative interview with vulnerable populations, the goal is to prevent ethical tensions by establishing and maintaining a “just right” relationship in an in-between position on a continuum ranging from under-rapport to over-rapport (Schmid et al., 2024 ).
Conclusions
Longitudinal qualitative research has great promise for better understanding career development and vocational behavior in a context of multiform, changing, and increasingly unpredictable careers. This methodological approach complements longitudinal quantitative research and cross-sectional qualitative research. However, several major issues are associated with its implementation, including a need to consolidate the relevance of qualitative research in the field and a more flexible approach to the rigor criteria for such studies. This suggests a paradigmatic shift in approach to research in vocational psychology. The reflections proposed in this paper are intended to accelerate this shift, which is now essential in respect of the complexity and vulnerability of contemporary careers.
In line with Blustein et al. ( 2019 ), we define vocational psychology as “the scholarly study of work or career-based behavior and development across the life span” (p. 170).
For an overview of interdisciplinary and international developments, see Neale ( 2021b ).
Templates refer to “standardized ways of conducting research that are used as formulas for shaping the methods themselves, especially data collection and analysis.” (Pratt et al., 2022 , p. 212).
Akkermans, J., da Motta Veiga, S. P., Hirschi, A., & Marciniak, J. (2024). Career transitions across the lifespan: A review and research agenda. Journal of Vocational Behavior, 148 , 103957. https://doi.org/10.1016/j.jvb.2023.103957
Article Google Scholar
Akkermans, J., Lee, C. I. S. G., Nijs, S., & Oostrom, J. K. (2021). Mapping methods in careers research: A review and future research agenda. In W. Murphy & J. Tosti-Kharas (Eds.), Handbook of research methods in careers (pp. 9–32). Edward Elgar Publishing.
Google Scholar
Audulv, Å., Westergren, T., Ludvigsen, M. S., Pedersen, M. K., Fegran, L., Hall, E. O. C., Aagaard, H., Robstad, N., & Kneck, Å. (2023). Time and change: A typology for presenting research findings in qualitative longitudinal research. BMC Medical Research Methodology, 23 (1), 284. https://doi.org/10.1186/s12874-023-02105-1
Bidart, C., Longo, M., & Mendez, A. (2013). Time and process: An operational framework for processual analysis. European Sociological Review, 29 (4), 743–751. https://doi.org/10.1093/esr/jcs053
Birch, M., & Miller, T. (2000). Inviting intimacy: The interview as therapeutic opportunity. International Journal of Social Research Methodology, 3 (3), 189–202. https://doi.org/10.1080/13645570050083689
Blustein, D. L., Ali, S. R., & Flores, L. Y. (2019). Vocational psychology: Expanding the vision and enhancing the impact. The Counseling Psychologist, 47 (2), 166–221. https://doi.org/10.1177/0011000019861213
Blustein, D. L., Kenna, A. C., Murphy, K. A., DeVoy, J. E., & DeWine, D. B. (2005). Qualitative research in career development: Exploring the center and margins of discourse about careers and working. Journal of Career Assessment, 13 (4), 351–370. https://doi.org/10.1177/1069072705278047
Braun, V., & Clarke, V. (2019). Reflecting on reflexive thematic analysis. Qualitative Research in Sport, Exercise and Health, 11 (4), 589–597. https://doi.org/10.1080/2159676X.2019.1628806
Brazier, C. É., Masdonati, J., & Parmentier, M. (2023a). Chronicles of involuntary career change: A longitudinal qualitative analysis . [Manuscript submitted for publication]. Institute of Psychology, University of Lausanne.
Brazier, C. É., Parmentier, M., Oliveira Borges, A., & Masdonati, J. (2023b). Les reconversions professionnelles involontaires: Bilan de la deuxième vague d’entretiens [Involuntary career changes: Report on the second wave of interviews]. University of Lausanne.
Brazier, C. É., Masdonati, J., Oliveira Borges, A., Fedrigo, L., & Cerantola, M. (2024). Drivers of involuntary career changes: A qualitative study of push, pull, anti-push, and anti-pull factors. Journal of Career Development, 51 (3), 303–326. https://doi.org/10.1177/08948453241246720
Chen, C. P., & Bansal, J. (2022). Assisting athletes facing career transitions post-injury. International Journal for Educational and Vocational Guidance, 22 , 1–21. https://doi.org/10.1007/s10775-021-09469-0
Chudzikowski, K. (2012). Career transitions and career success in the “new” career era. Journal of Vocational Behavior, 81 (2), 298–306. https://doi.org/10.1016/j.jvb.2011.10.005
Conroy, S. A., & O’Leary-Kelly, A. M. (2014). Letting go and moving on: Work-related identity loss and recovery. Academy of Management Review, 39 , 67–87. https://doi.org/10.5465/amr.2011.0396
Dall, T., & Danneris, S. (2019). Re-considering “what works” in welfare to work with the vulnerable unemployed: The potential of relational causality as an alternative approach. Social Policy and Society, 18 (4), 583–596. https://doi.org/10.1017/S1474746419000186
Danneris, S. (2018). Ready to work (yet)? Unemployment trajectories among vulnerable welfare recipients. Qualitative Social Work, 17 (3), 355–372. https://doi.org/10.1177/1473325016672916
Dlouhy, K., & Biemann, T. (2017). Methodische Herausforderungen in der Karriere- und Laufbahnforschung [Methodological challenges in career and career pathway research]. In S. Kauffeld & D. Spurk (Eds), Handbuch Karriere und Laufbahnmanagement . Springer Reference Psychologie. https://doi.org/10.1007/978-3-662-45855-6_40-1
Farr, J., & Nizza, I. E. (2019). Longitudinal Interpretative Phenomenological Analysis (LIPA): A review of studies and methodological considerations. Qualitative Research in Psychology, 16 (2), 199–217. https://doi.org/10.1080/14780887.2018.1540677
Fleet, D., Burton, A., Reeves, A., & DasGupta, M. P. (2016). A case for taking the dual role of counsellor-researcher in qualitative research. Qualitative Research in Psychology, 13 (4), 328–346. https://doi.org/10.1080/14780887.2016.1205694
Fouad, N. A., & Bynner, J. (2008). Work transitions. American Psychologist, 63 (4), 241–251. https://doi.org/10.1037/0003-066X.63.4.241
Gati, I., & Levin, N. (2015). Making better career decisions. In P. J. Hartung, M. L. Savickas, & W. B. Walsh (Eds.), APA handbook of career intervention, Vol. 2. Applications (pp. 193–207). American Psychological Association. https://doi.org/10.1037/14439-015
George, M. M., Wittman, S., & Rockmann, K. W. (2022). Transitioning the study of role transitions: From an attribute-based to an experience-based approach. Academy of Management Annals, 16 (1), 102–133. https://doi.org/10.5465/annals.2020.0238
Heppner, P. P., Wampold, B. E., Owen, J., Thompson, M. N., & Wang, K. T. (2016). Research design in counseling (4th ed.). Cengage Learning.
Hermanowicz, J. C. (2009). Lives in Science. Chicago University Press . https://doi.org/10.7208/9780226327761
Hodkinson, P., Sparkes, A., & Hodkinson, H. (1996). Triumphs and tears: Young people, markets and the transition from school to work . Routledge.
Ibarra, H., & Petriglieri, J. L. (2010). Identity work and play. Journal of Organizational Change Management, 23 , 10–25. https://doi.org/10.1108/09534811011017180
Keskinen, K., Lumme-Sandt, K., & Nikander, P. (2023). Turning age into agency: A qualitative longitudinal investigation into older jobseekers’ agentic responses to ageism. Journal of Aging Studies, 65 , 101136. https://doi.org/10.1016/j.jaging.2023.101136
Kulkarni, M. (2020). Holding on to let go: Identity work in discontinuous and involuntary career transitions. Human Relations, 73 (10), 1415–1438. https://doi.org/10.1177/0018726719871087
Lent, R. W. (2013). Career-life preparedness: Revisiting career planning and adjustment in the new workplace. The Career Development Quarterly, 61 (1), 2–14. https://doi.org/10.1002/j.2161-0045.2013.00031.x
Lent, R. W., & Brown, S. D. (2020). Career decision making, fast and slow: Toward an integrative model of intervention for sustainable career choice. Journal of Vocational Behavior, 120 , 103448. https://doi.org/10.1016/j.jvb.2020.103448
Levin, N., & Lipshits-Braziler, Y. (2022). Facets of adaptability in career decision-making. International Journal for Educational and Vocational Guidance, 22 , 535–556. https://doi.org/10.1007/s10775-021-09489-w
Levitt, H. M., Motulsky, S. L., Wertz, F. J., Morrow, S. L., & Ponterotto, J. G. (2017). Recommendations for designing and reviewing qualitative research in psychology: Promoting methodological integrity. Qualitative Psychology, 4 (1), 2–22. https://doi.org/10.1037/qup0000082
Masdonati, J., Frésard, C. É., & Parmentier, M. (2022). Involuntary career changes: A lonesome social experience. Frontiers in Psychology, 13 , 899051. https://doi.org/10.3389/fpsyg.2022.899051
Mehlhouse, K., Johnsen, K. B., & Erford, B. T. (2023). A meta-study of the Journal of Career Development: An analysis of publication characteristics from 2000 to 2019. Journal of Career Development, 50 (3), 534–546. https://doi.org/10.1177/08948453221112110
McCoy, L. K. (2017). Longitudinal qualitative research and interpretative phenomenological analysis: Philosophical connections and practical considerations. Qualitative Research in Psychology, 14 (4), 442–458. https://doi.org/10.1080/14780887.2017.1340530
Millar, J. (2007). The dynamics of poverty and employment: The contribution of qualitative longitudinal research to understanding transitions, adaptations and trajectories. Social Policy and Society, 6 (4), 533–544. https://doi.org/10.1017/S1474746407003879
Neale, B. (2021a). Fluid enquiry, complex causality, policy processes: Making a difference with qualitative longitudinal research. Social Policy and Society, 20 (4), 653–669. https://doi.org/10.1017/S1474746421000142
Neale, B. (2021b). The craft of qualitative longitudinal research . Sage.
Neale, B., & Davies, L. (2016). Becoming a young breadwinner? The education, employment and training trajectories of young fathers. Social Policy and Society, 15 (1), 85–98. https://doi.org/10.1017/S1474746415000512
Neale, B., & Tarrant, A. (2024). The dynamics of young fatherhood: Understanding the parenting journeys and support needs of young fathers . Policiy Press. https://doi.org/10.56687/9781447351726
Book Google Scholar
Neary, J., Katikireddi, S. V., McQuaid, R. W., Macdonald, E. B., & Thomson, H. (2021). Using candidacy theory to explore unemployed over-50s perceptions of suitability of a welfare to work programme: A longitudinal qualitative study. Social Policy and Administration, 55 (4), 589–605. https://doi.org/10.1111/spol.12644
Olry-Louis, I., Cocandeau-Bellanger, L., Fournier, G., & Masdonati, J. (2022). Temporality: A fruitful concept for studying, understanding, and supporting people in transition. The Career Development Quarterly, 70 (4), 256–270. https://doi.org/10.1002/cdq.12306
Patrick, R. (2017). For whose benefit?: The everyday realities of welfare reform. Bristol University Press . https://doi.org/10.2307/j.ctt1t896vj
Patton, W., & McMahon, M. (2015). The systems theory framework of career development: 20 years of contribution to theory and practice. Australian Journal of Career Development, 24 (3), 141–147. https://doi.org/10.1177/1038416215579944
Perkins, D. F., Davenport, K. E., Morgan, N. R., et al. (2023). The influence of employment program components upon job attainment during a time of identity and career transition. International Journal for Educational and Vocational Guidance, 23 , 695–717. https://doi.org/10.1007/s10775-022-09527-1
Pinnock, H., Kendall, M., Murray, S., Worth, A., Levack, P., MacNee, W., & Sheikh, A. (2011). Living and dying with severe chronic obstructive pulmonary disease: A multi-perspective longitudinal qualitative study. BMJ . https://doi.org/10.1136/bmj.d142
Ponterotto, J. G. (2005). Qualitative research in counseling psychology: A primer on research paradigms and philosophy of science. Journal of Counseling Psychology, 52 (2), 126–136. https://doi.org/10.1037/0022-0167.52.2.126
Pratt, M. G., Sonenshein, S., & Feldman, M. S. (2022). Moving beyond templates: A bricolage approach to conducting trustworthy qualitative research. Organizational Research Methods, 25 (2), 211–238. https://doi.org/10.1177/1094428120927466
Richardson, J., O’Neil, D. A., & Thorn, K. (2022). Exploring careers through a qualitative lens: An investigation and invitation. Career Development International, 27 (1), 99–112. https://doi.org/10.1108/CDI-08-2021-0197
Rossier, J., Cardoso, P. M., & Duarte, M. E. (2021). The narrative turn in career Development theories: An integrative perspective. In P. Robertson, T. Hooley & P. McCash (Eds.), The Oxford Handbook of Career Development (pp. 169–180). Oxford University Press. https://doi.org/10.1093/oxfordhb/9780190069704.013.13
Rudolph, C. W. (2021). Improving careers science: Ten recommendations to enhance the credibility of vocational behavior research. Journal of Vocational Behavior, 126 , 103560. https://doi.org/10.1016/j.jvb.2021.103560
Saldaña, J. (2003). Longitudinal qualitative research: Analyzing change through time . AltaMira.
Savickas, M. L. (2019). Career counseling (2nd ed.). American Psychological Association.
Savickas, M. L. (2020). Career construction theory and counseling model. In R.W. Lent & S. D. Brown (Eds), Career development and counseling: Putting theory and research to work (3rd Ed., pp. 155–200). Wiley. https://doi.org/10.1002/9781394258994.ch6
Schmid, E., Garrels, V., & Skåland, B. (2024). The continuum of rapport: Ethical tensions in qualitative interviews with vulnerable participants. Qualitative Research . https://doi.org/10.1177/14687941231224600
SmithBattle, L., Lorenz, R., Reangsing, C., Palmerm, J. L., & Pitroff, G. (2018). A methodological review of qualitative longitudinal research in nursing. Nursing Inquiry, 25 (4), e12248. https://doi.org/10.1111/nin.12248
Solomon, P., Nixon, S., Bond, V., Cameron, C., & Gervais, N. (2020). Two approaches to longitudinal qualitative analyses in rehabilitation and disability research. Disability and Rehabilitation, 42 (24), 3566–3572. https://doi.org/10.1080/09638288.2019.1602850
Stead, G. B., Perry, J. C., Munka, L. M., Bonnett, H. R., Shiban, A. P., & Care, E. (2012). Qualitative research in career development: Content analysis from 1990 to 2009. International Journal for Educational and Vocational Guidance, 12 , 105–122. https://doi.org/10.1007/s10775-011-9196-1
Sullivan, S. E., & Al Ariss, A. (2021). Making sense of different perspectives on career transitions: A review and agenda for future research. Human Resource Management Review, 31 (1), 100727. https://doi.org/10.1016/j.hrmr.2019.100727
Sullivan, S. E., & Al Ariss, A. (2022). A conservation of resources approach to inter-role career transitions. Human Resource Management Review, 32 (3), 100852. https://doi.org/10.1016/j.hrmr.2021.100852
Super, D. E., Savickas, M. L., & Super, C. M. (1996). The life-span, life-space approach to careers. In D. Brown, L. Brooks, & Associates (Eds.), Career choice and development (3rd Ed.) (pp. 121–178). Jossey-Bass.
Thomson, R., & Holland, J. (2003). Hindsight, foresight and insight: The challenges of longitudinal qualitative research. International Journal of Social Research Methodology, 6 (3), 233–244. https://doi.org/10.1080/1364557032000091833
Torregrosa, M., Ramis, Y., Pallarés, S., Azócar, F., & Selva, C. (2015). Olympic athletes back to retirement: A qualitative longitudinal study. Psychology of Sport and Exercise, 21 , 50–56. https://doi.org/10.1016/j.psychsport.2015.03.003
Treanor, M. C., Patrick, R., & Wenham, A. (2021). Qualitative longitudinal research: From monochrome to technicolour. Social Policy and Society, 20 (4), 635–651. https://doi.org/10.1017/S1474746421000270
Udayar, S., Toscanelli, C., & Massoudi, K. (2024). Sustainable career trajectories in Switzerland: The role of psychological resources and sociodemographic characteristics. Journal of Career Assessment . https://doi.org/10.1177/10690727241234929
Vogl, S., Zartler, U., Schmidt, E.-M., & Rieder, I. (2018). Developing an analytical framework for multiple perspective, qualitative longitudinal interviews (MPQLI). International Journal of Social Research Methodology, 21 (2), 177–190. https://doi.org/10.1080/13645579.2017.1345149
Zittoun, T. (2009). Dynamics of life-course transitions: A methodological reflection. In J. Valsiner, P. C. M. Molenaar, M. C. D. P. Lyra, & N. Chaudhary (Eds.), Dynamic process methodology in the social and developmental sciences (pp. 405–430). New Springer Science and Business Media. https://doi.org/10.1007/978-0-387-95922-1_1
Download references
Open access funding provided by University of Lausanne.
Author information
Authors and affiliations.
Institute of Psychology, University of Lausanne, UNIL-Mouline, 1015, Géopolis, Lausanne, Switzerland
J. Masdonati, C. É. Brazier, M. Kekki & M. Parmentier
Swiss National Centre of Competence in Research LIVES – Overcoming vulnerability: life course perspectives (NCCR LIVES), University of Lausanne, Lausanne, Switzerland
J. Masdonati & C. É. Brazier
Management School, HEC Liège, University of Liège, Liège, Belgium
M. Parmentier
School of Sociology and Social Policy, University of Leeds, Leeds, UK
You can also search for this author in PubMed Google Scholar
Corresponding author
Correspondence to J. Masdonati .
Ethics declarations
Conflict of interest.
On behalf of all authors, the corresponding author states that there is no conflict of interest.
Additional information
Publisher’s note.
Springer Nature remains neutral with regard to jurisdictional claims in published maps and institutional affiliations.
Rights and permissions
Open Access This article is licensed under a Creative Commons Attribution 4.0 International License, which permits use, sharing, adaptation, distribution and reproduction in any medium or format, as long as you give appropriate credit to the original author(s) and the source, provide a link to the Creative Commons licence, and indicate if changes were made. The images or other third party material in this article are included in the article's Creative Commons licence, unless indicated otherwise in a credit line to the material. If material is not included in the article's Creative Commons licence and your intended use is not permitted by statutory regulation or exceeds the permitted use, you will need to obtain permission directly from the copyright holder. To view a copy of this licence, visit http://creativecommons.org/licenses/by/4.0/ .
Reprints and permissions
About this article
Masdonati, J., Brazier, C.É., Kekki, M. et al. Qualitative longitudinal research in vocational psychology: a methodological approach to enhance the study of contemporary careers. Int J Educ Vocat Guidance (2024). https://doi.org/10.1007/s10775-024-09692-5
Download citation
Received : 08 April 2024
Accepted : 11 July 2024
Published : 09 August 2024
DOI : https://doi.org/10.1007/s10775-024-09692-5
Share this article
Anyone you share the following link with will be able to read this content:
Sorry, a shareable link is not currently available for this article.
Provided by the Springer Nature SharedIt content-sharing initiative
- Qualitative methods
- Qualitative longitudinal research
- Vocational psychology
- Career development
- Career transitions
- Find a journal
- Publish with us
- Track your research
Thank you for visiting nature.com. You are using a browser version with limited support for CSS. To obtain the best experience, we recommend you use a more up to date browser (or turn off compatibility mode in Internet Explorer). In the meantime, to ensure continued support, we are displaying the site without styles and JavaScript.
- View all journals
- Explore content
- About the journal
- Publish with us
- Sign up for alerts
- Open access
- Published: 02 August 2024
Longitudinal study on the multifactorial public health risks associated with sewage reclamation
- Inés Girón-Guzmán 1 ,
- Santiago Sánchez-Alberola 1 , 2 ,
- Enric Cuevas-Ferrando ORCID: orcid.org/0000-0002-0799-009X 1 ,
- Irene Falcó 1 , 3 ,
- Azahara Díaz-Reolid 1 ,
- Pablo Puchades-Colera ORCID: orcid.org/0009-0009-5692-3406 1 ,
- Sandra Ballesteros 4 ,
- Alba Pérez-Cataluña 1 ,
- José María Coll 1 ,
- Eugenia Núñez ORCID: orcid.org/0000-0002-1852-3374 1 , 2 ,
- María José Fabra 1 , 2 ,
- Amparo López-Rubio 1 , 2 na1 &
- Gloria Sánchez 1 na1
npj Clean Water volume 7 , Article number: 72 ( 2024 ) Cite this article
454 Accesses
7 Altmetric
Metrics details
- Environmental sciences
- Water resources
This year-long research analyzed emerging risks in influent, effluent wastewaters and biosolids from six wastewater treatment plants in Spain’s Valencian Region. Specifically, it focused on human enteric and respiratory viruses, bacterial and viral faecal contamination indicators, extended-spectrum beta-lactamases-producing Escherichia coli , and antibiotic-resistance genes. Additionally, particles and microplastics in biosolid and wastewater samples were assessed. Human enteric viruses were prevalent in influent wastewater, with limited post-treatment reduction. Wastewater treatment effectively eliminated respiratory viruses, except for low levels of SARS-CoV-2 in effluent and biosolid samples, suggesting minimal public health risk. Antibiotic resistance genes and microplastics were persistently found in effluent and biosolids, thus indicating treatment inefficiencies and potential environmental dissemination. This multifaced research sheds light on diverse contaminants present after water reclamation, emphasizing the interconnectedness of human, animal, and environmental health in wastewater management. It underscores the need for a One Health approach to address the United Nations Sustainable Development Goals.
Similar content being viewed by others
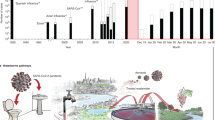
Rethinking wastewater risks and monitoring in light of the COVID-19 pandemic
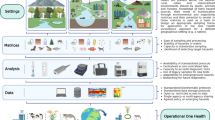
Realising a global One Health disease surveillance approach: insights from wastewater and beyond
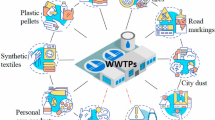
Research progress on the origin, fate, impacts and harm of microplastics and antibiotic resistance genes in wastewater treatment plants
Introduction.
Water is a fundamental resource for human life, being also essential for crops and livestock production. However, the increasing global population and limited freshwater resources pose significant challenges to meeting the demands of various sectors, including agriculture. Water reuse has emerged as a sustainable solution to preserve freshwater resources and reduce environmental pressure. Reclaimed water, also known as recycled water or effluent from wastewater treatment plants (WWTPs), refers to the treated wastewater that undergoes a series of physical, chemical, and biological processes to remove contaminants and pathogens. The reclaimed water is then suitable for non-potable uses, such as irrigation, industrial processes, and groundwater recharge according to national regulations 1 .
Water reuse has become increasingly important in agriculture due to the limited freshwater resources and the growing demand for food production. Agriculture accounts for approximately 70% of global freshwater withdrawals and the water demand for crops and livestock is projected to increase in the coming decades 2 . Reclaimed water offers a sustainable solution to reduce the demand for freshwater resources and ensure the availability of water for irrigation while reducing the discharge of treated wastewater into the environment and the cost of water supply. However, water reuse also poses several challenges, particularly in terms of microbiological and chemical safety. Reclaimed water may contain a variety of contaminants, including bacteria, viruses, protozoa, and emerging pollutants, such as microplastics (MPs), antibiotic resistant genes (ARGs), and pharmaceuticals 3 .
In particular, human enteric viruses are responsible for causing viral gastroenteritis, hepatitis, and various illnesses primarily transmitted through the faecal-oral route 4 . The spread of these viruses is primarily linked to person-to-person contact and the consumption of contaminated food and water. Enteric viruses are excreted in substantial quantities, up to 10 13 particles per gram of stool, by both symptomatic and asymptomatic individuals 5 , 6 . Major causative agents of waterborne viral gastroenteritis and hepatitis outbreaks worldwide include rotaviruses (RVs), norovirus genogroups I (HuNoV GI) and II (HuNoV GII), hepatitis A and E viruses (HAV and HEV), and human astroviruses 5 (HAstVs). In this context, and related to microbiological risks dissemination, a new European regulation (EC, 2020/741) on minimum quality criteria (MQR) for water reuse is in place since June 2023, outlining the guidelines for the use of reclaimed water for agricultural irrigation 7 . However, questions have arisen concerning potential non-compliance scenarios in European water reuse systems 8 , 9 , 10 , 11 , 12 . According to EC 2020/741 regulation, validation monitoring needs to assess whether the performance targets reductions are met. Monitoring of pathogen elimination in the water reclamation process is necessary to assess the suitability of reclaimed water in its secondary uses. In this respect, the WHO has suggested that another problem to be tackled in the framework of “One Health” is the rise of antibiotic resistance (AR) 13 . AR is frequent in places where antibiotics are employed, but antibiotic resistant bacteria (ARB) and ARGs are also widely prevalent in water environments 14 , 15 . According to several reports, surface water and reclaimed wastewater used for irrigation are significant sources of ARBs and ARGs 16 . Due to inadequate removal of ARGs, which are crucial in the growth of extremely unfavourable drug-resistant superbugs, reuse of WWTP effluents may be harmful to human health 17 .
On the other hand, plastic pollution is currently one of the most important environmental problems that humanity must face. The exponential growth of plastic production since 1950s (up to 368 million of tons were produced in 2019) and the massive use of plastics, together with insufficient/inadequate waste management/disposal strategies, are the main causes of the global presence of plastics in every environmental compartment 18 . The European Commission has recently published an amending Annex to Regulation (EC) No 1907/2006 concerning the Registration, Evaluation, Authorization, and Restriction of Chemicals (REACH) as regards synthetic polymer microparticles, where the intentional use of microplastics in commercial products is prohibited 19 .
Current research is showing that one of the main concerns about plastics, apart from the fact that they persist in the environment for an extremely long time, is their constant fragmentation into even smaller particles called microplastics (MPs, 1 μm–5 mm) or nanoplastics (< 1 μm), depending on their final dimensions, though they are also released as such 20 .
MPs are emerging global threats as they can end up in our bodies through water and food ingestion or by air inhalation 21 . The larger MPs can cause mechanical damage to the intestinal epithelium, while the smaller particles can cross the epithelial barrier 22 and end up in the lung 23 , colon 24 , placenta 25 , and even blood 26 .
MPs can transport pathogens over long distances, due to their ability to harbor biofilms on the surface, which can lead to the spread of pathogenic viruses and bacteria to new areas where they were not previously found 27 . Another of the main risks associated with MPs is that plastic materials include approximately 4% by weight of additives 28 , some of them declared as possible human carcinogens, and most of them considered endocrine disruptors 29 . In addition, MPs also contain traces of persistent organic pollutants (COPs), such as polychlorinated biphenyls (PCBs), polycyclic aromatic hydrocarbons (PAHs), and organochlorine pesticides 22 .
It is important to highlight that depending on the performance of WWTPs high amounts of pathogens, MPs and ARGs can be released on a daily basis into rivers, lakes, and oceans 9 , 14 , 30 . On the other hand, the sludge generated as well as the effluent water from the WWTPs are generally used in agriculture as a fertilizer and for irrigation respectively, and, therefore, the presence of emerging contaminants in these biosolids and reclaimed waters can favour the propagation of plastic particles, emerging pathogens, and ARGs through agricultural soils which could reach cultivated vegetables and ultimately the human body through the trophic chain.
In overall terms, understanding the distinct risk factors involved in the water reclamation process is critical to ensuring the safety of water reuse in agriculture and other sectors, and the analysis of the water reclamation process can serve as an important risk assessment tool. Moreover, by analysing wastewater, we gain valuable insights into the collective health of a community, as it contains traces of chemical pollutants, pathogens, and biomarkers from human and animal sources. Thus, monitoring wastewater helps identifying trends in the prevalence of diseases, antibiotic resistance patterns, zoonotic pathogens, and exposure to environmental pollutants as MPs, providing early warning and valuable data for public health interventions. This integration of environmental, human, and animal health data underscores the significance of wastewater analysis in promoting a comprehensive and proactive “One Health” approach to public health and the well-being of both the planet and its inhabitants.
Incidence of human enteric viruses, respiratory viruses, and viral faecal indicators in influent and effluent wastewater samples
The presence of human enteric viruses, including HuNoV GI, HuNoV GII, HAstV, HAV, HEV, and RV, was analysed, along with novel viral faecal contamination indicators pepper mild mottle virus (PMMoV), crAssphage and somatic coliphages in influent, effluent and biosolid samples from six different WWTPs in the Valencian region of Spain (Figs. 1 and 2 ).
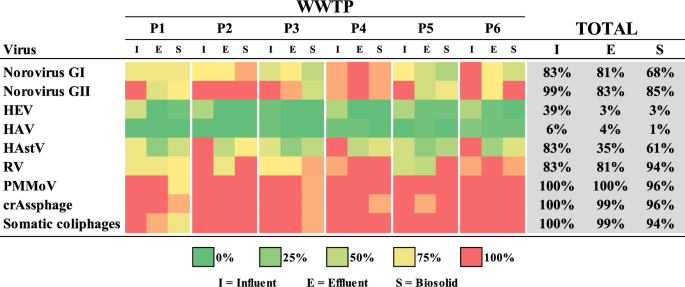
HEV hepatitis E virus, HAV hepatitis A virus, HAstV human astrovirus, RV rotavirus, PMMoV pepper mild mottle virus.
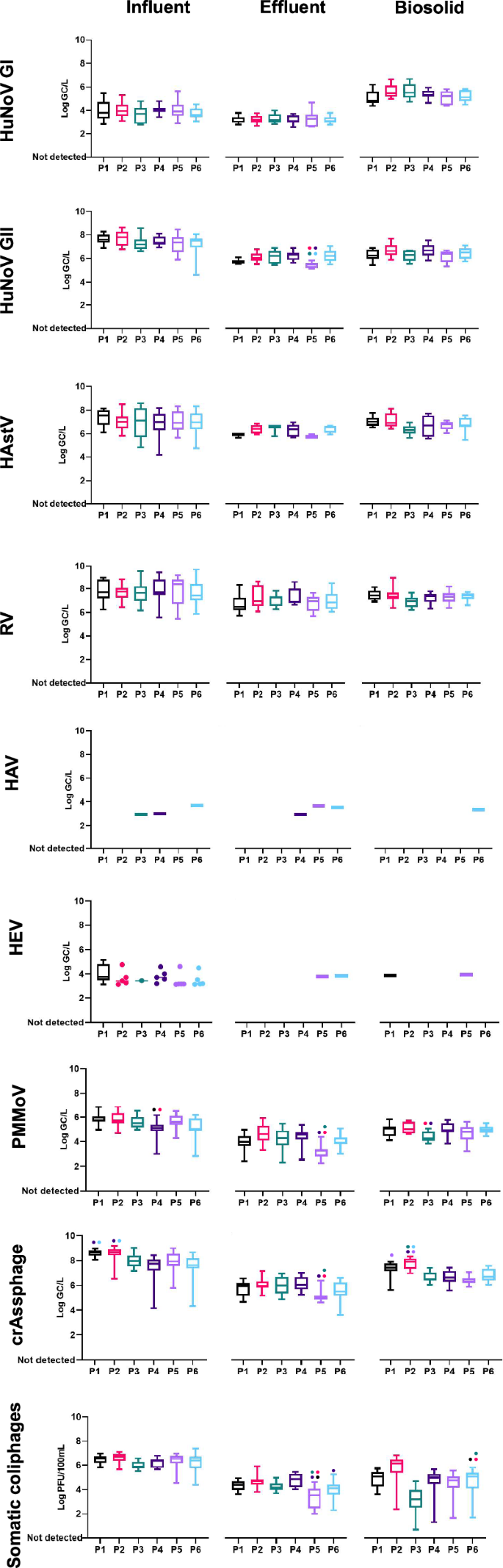
Whiskers in box are drawn min. to max., box extends from the 25th to 75th percentiles, and line within the box represents the median. Coloured circles above a box indicate significant differences between that box and the box with that same colour ( p < 0.05). GC genome copies, PFU plate forming units, RV rotavirus, HuNoV human norovirus, HAstV human astrovirus, HAV hepatitis A virus, HEV hepatitis E virus, PMMoV pepper mild mottle virus.
In influent wastewater samples, the mean highest levels of viruses were observed for RV (8.55 log genome copies, GC/L), followed by HuNoV GII (7.80 log GC/L) and HAstV (7.72 log GC/L). The lowest concentration levels were detected for HuNoV GI (4.46 log GC/L), HEV (4.13 log GC/L), and HAV (3.47 log GC/L) (Fig. 2 ). HAV was only detected in 4 out of 72 influent wastewater samples (Fig. 1 ). PMMoV and crAssphage were detected in all influent samples, with mean levels of 5.95 log GC/L and 8.44 log GC/L, respectively.
In the effluent wastewater samples, the titres of all viruses decreased after the water reclamation process. HuNoV GI, HuNoV GII, HAstV, and RV showed mean concentrations titers of 3.51, 6.25, 6.35, and 7.69 Log GC/L when detected, respectively (Fig. 2 ). On the contrary, HEV was not detected in any of the effluent samples. In the case of faecal viral indicators, PMMoV (4.72 Log GC/L) and crAssphage (6.23 Log GC/L) were present in all effluent samples. The highest reduction in virus levels were observed for HEV, with a reduction of 4 Log GC/L, even though the vast majority of viruses’ reduction levels were below 2 Logs GC/L (Supplementary Fig. 1 ). Interestingly, viable somatic coliphages were found at levels of 4.73 Log plaque forming units (PFU)/100 mL in effluent waters, with a mean reduction of 1.83 Log PFU/100 mL compared to the influent waters (6.54 Log PFU/100 mL) when testing positive.
As for biosolid samples, HuNoV GI, HuNoV GII, HAstV, and RV showed the highest mean concentrations, with titers ranging from 5.37 (HuNoV GI) to 7.27 Log GC/L (RV) when detected (Fig. 2 ). HAV and HEV rendered lower mean concentrations of 3.24 and 3.91 Log GC/L, respectively. Besides, proposed viral faecal indicators yielded mean concentrations levels of 7.06 Log GC/L for crAssphage, 4.85 Log GC/L for PMMoV. and 5.63 Log PFU/100 mL for somatic coliphages (Fig. 2 ).
Regarding respiratory viruses, respiratory syncytial virus (RSV) showed a remarkable seasonality, with almost all positive samples being collected on November and December 2022 (Fig. 3 ). Influenza A virus (IAV) was intermittently detected over the year, with the most noteworthy peaks taking place in spring and winter (Fig. 3 ). Finally, SARS-CoV-2 was present in 99% and 32% of the influent and effluent samples, respectively. When testing positive, mean concentration values for RSV, IAV, and SARS-CoV-2 were 4.57, 6.20, and 5.27 Log GC/L, respectively. Notably, any of the analysed effluent wastewater samples tested positive for either RSV or IAV.
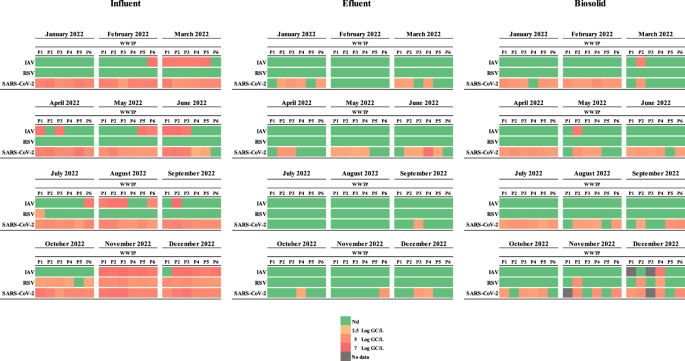
Nd not detected, GC genome copies, SARS-CoV-2 severe acute respiratory syndrome coronavirus 2, RSV respiratory syncytial virus, IA Influenza A virus.
Regarding biosolid samples, SARS-CoV-2 was found positive in 71% of the samples at a mean concentration of 4.44 Log GC/L, while RSV and IAV only tested positive in three biosolid samples.
In general, no significant differences were found among the six different WWTPs analysed neither for enteric or respiratory viruses.
Quantification of Escherichia coli , Extended Spectrum Beta-Lactamases-producing E. coli , and ARGs in wastewater and biosolids samples
In influent wastewater samples, the mean concentration of E. coli and ESBL- E. coli were 7.08 Log colony forming units (CFU)/100 mL and 6.19 Log CFU/100 mL, respectively (Fig. 4 ). After the wastewater treatment process, the mean concentrations of E. coli , and ESBL- E. coli in the effluent wastewater samples were significantly reduced, with mean concentrations of 5.43 Log CFU/100 mL, and 4.76 Log CFU/100 mL, respectively.
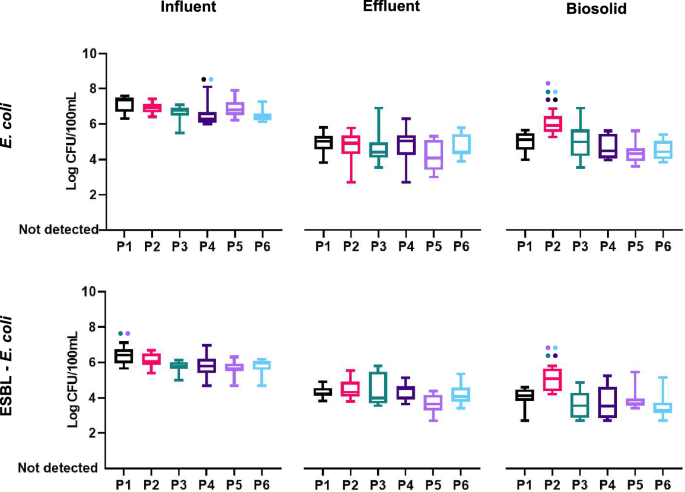
Whiskers in box are drawn min. to max., box extends from the 25th to 75th percentiles, and the line within the box represents the median. Coloured circles above a box indicate significant differences between that box and the box with that same colour ( p < 0.05). CFU colony forming unit ESBL- E. coli extended spectrum beta-lactamases producing Escherichia coli .
Regarding biosolid samples, the mean concentration of E. coli was 5.64 Log CFU/100 mL, while ESBL- E. coli yielded a mean concentration of 4.89 Log CFU/100 mL.
Furthermore, a deeper analysis of the ARGs present in effluent and biosolids samples was performed due to the high levels of ESBL- E. coli in biosolids and the observed low performance of the water reclamation process (less than 2 log reduction; Fig. 4 ). ARGs including tetPB_3 , tetA_1 , and qacA_1 were not detected in effluent wastewater and biosolids. ARG sul1_1 , sul2_1 , pbp2b , bla CTX-M , cmlA_2 , nimE , and ermB were detected in effluent samples at mean concentrations of 9.20, 8.78, 8.57, 8.42, 8.31, 8,24, and 8.39 Log GC/100 mL, respectively (Fig. 5 ).
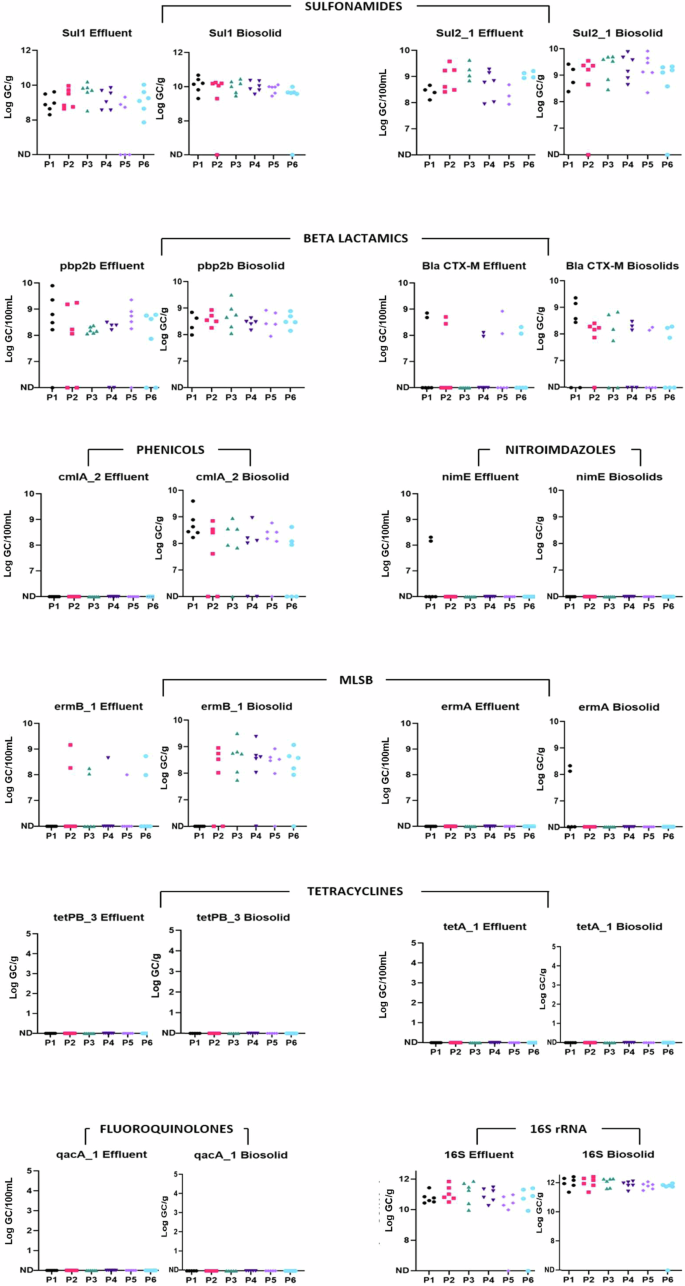
Each different symbol type represents a different WWTP. ND Not detected, MLSB Macrolide-lincosamide-streptogramin B group antibiotics, GC genome copies.
ARGs were identified in biosolids, with the following values: 9.87, 9.25, 8.58, 8.42, 8.50, 8.64, 8.28 Log GC/100 mL for sul1_1 , sul2_1 , pbp2b , bla CTX-M , cmlA_2 , erm B, and ermA , respectively. Notably, nimE was not found in any of analysed biosolids.
Quantification of particles and microplastics present in biosolids and reclaimed water samples
The presence of solid particles and microplastics was bi-monthly analysed in both influent and effluent wastewater samples. In general, a great reduction in both the number of particles between 1 μm and 5 mm or (T)-P and particles larger than 300 µm or (S)-P was observed after the wastewater treatment process (Fig. 6 ). Although there was not a clear effect derived from seasonality, WWTPs were slightly less efficient in removing (T)-P in January and March.
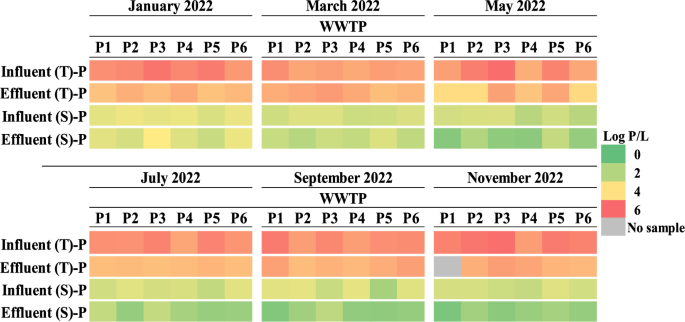
Concentration (log P/L) of total particles (T)-P and sieved particles (>300 μm, (S)-P) in influent and effluent wastewater samples in even months over a one-year period in six different WWTPs (P1-P6).
The efficiency of each WWTPs regarding the reduction of (T)-P and (S)-P particles was determined considering the average number of particles in the influent and effluent wastewater samples (Fig. 7 ). At the WWTP level, the calculated efficiency in (T)-P reduction was approximately 84, 68, 69, 46, 80 and 71%, for the different WWTPs (P1-P6) samples analysed. Notably, the efficiency in removing (S)-P was higher than in removing (T)-P, with the most noteworthy reduction taking place for P2 and P6 wastewater samples (91 and 93% approximately, and respectively), while the lowest efficiency in (T)-P reduction was approximately 40% for P5.
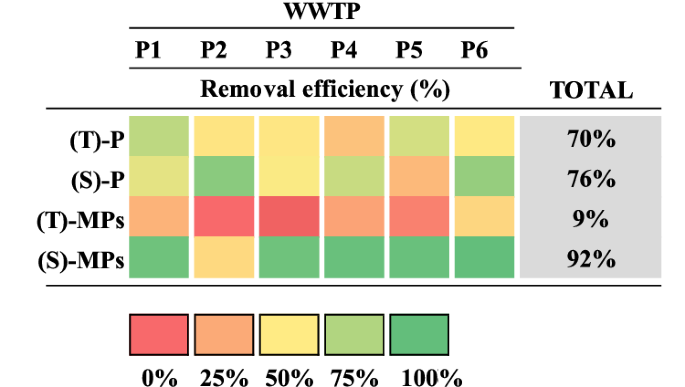
Removal efficiency (%) of all solid particles (P) and microplastics (MPs) between influent and effluent samples collected from six different WWTPs (P1-P6) after both pre-treatment protocols. Total Particles (T) and Sieved > 300 µm (S).
Once (T)-P and (S)-P particles were quantified, all samples were spectroscopically characterized in order to identify the presence of MPs derived from synthetic polymer particles, fibres, and films. In general terms, the highest reduction was observed in (S)-MPs as compared to (T)-MPs, thus suggesting the lower efficiency of wastewater treatments in removing microplastics smaller than 300 μm (Fig. 7 ). It should be highlighted that the efficiency of WWTPs for removing MPs of smaller particle size or (T)-MPs was lower than for removing all solid particles or (T)-P, being 59% the highest (T)-MPs efficiency (sample P6). In general, a higher efficiency in reducing (S)-MPs was observed (around 98-100%) in all samples, except in P2 (77%) (Fig. 7 ).
Considering the pre-treatment (T), the annual average MPs concentration in influent samples was around 1816 MPs/L which was slightly reduced in effluent samples (1724 MPs/L). In contrast, the annual average concentration of (S)-MPs (larger than 300 µm) in influent samples was 198 MPs/L and it was significantly reduced in effluent wastewater samples until 11 MPs/L in average (Fig. 8 ).
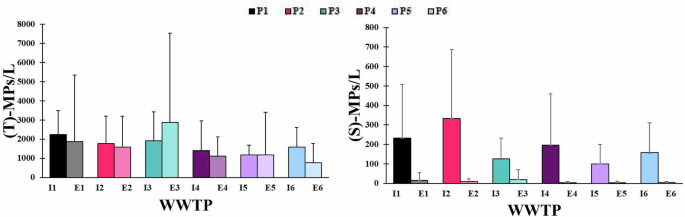
Average concentration (mean + standard deviation) of MPs in influent (I) and effluent (E) after (T) (left) and (S) (right) protocols collected from six different WWTPs. T total particles, S Sieved > 300 µm.
The annual average percentage of MPs with respect to all solid particles in influent and effluent wastewater samples and biosolids was also determined (Supplementary Fig. 2 ). It is worth mentioning that, regarding the particles larger than 300 μm, the MPs/all solid particles ratio in biosolid samples was similar to the MPs/all solid particles ratio in influent wastewater samples, reaching values up to 35 in some of the WWTPs (Supplementary Fig. 2 ).
In all the analysed biosolid samples a significant number of (S)-P was also detected, and no significant effects due to seasonality were found (Fig. 9 ). The average highest concentration of (S)-MPs was 122 MPs/g and 99 MPs/g for P1 and P2, respectively. In contrast, the lowest level of MPs was detected for P3 (23 MPs/g) (Supplementary Fig. 3 ).
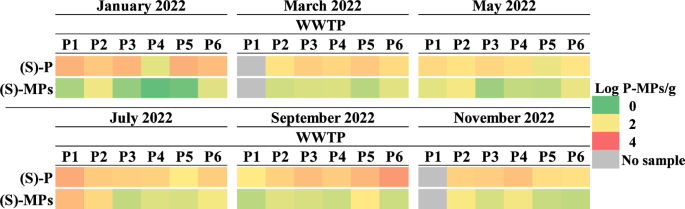
Concentration (in log/g) of (S)-P and (S)-MPs in biosolids in even months over a one-year period in six different WWTPs (P1-P6).
Analysing the morphology and type of MPs identified in the WWTPs samples may help to understand the origin of water pollution (Supplementary Figs. 5 and 6 ). As depicted in Fig. 10 , the majority of MPs existing in influent wastewater samples had the shape of fragments ( ∼ 86%), percentage that was further increased in effluent wastewater samples. The percentage of particles identified as films was negligible both in influent or effluent samples. Most of the MPs found in influent samples were between 0 and 100 µm ( ∼ 61%) in size, percentage that was increased in effluents (up to 73%), and a small fraction of MPs ( ∼ 3-5%) were larger than 300 µm in size, in agreement with the results commented above (Fig. 8 ). It is hypothesized that, during sieving, particles smaller than 300 µm may aggregate and become retained, but following oxidative digestion, they break down into smaller particles. The composition of the MPs was dominated by common polymers, whereas the PS, PA, PVC, and PET were greatly decreased in effluent samples (Fig. 10 ). It is worth mentioning that the distribution of polymer type was quite different when comparing wastewater and biosolids samples. PE was dominant in all samples, accounting for 56, 46 and 57% of the total MPs, for wastewater (T)-MPs and (S)-MPs, and for biosolids (S)-MPs, respectively (Supplementary Fig. 4 ). The amount of PA was more than two-fold higher in (T)-MPs samples from wastewater than in (S)-MPs from biosolids (31% vs. 12%, respectively). PET represented around 21–28% of the (S)-MPs in wastewater and biosolid samples. Other polymers such as PS, polytetrafluoroethylene PTFE, PVC, and PS were detected in lower amounts.
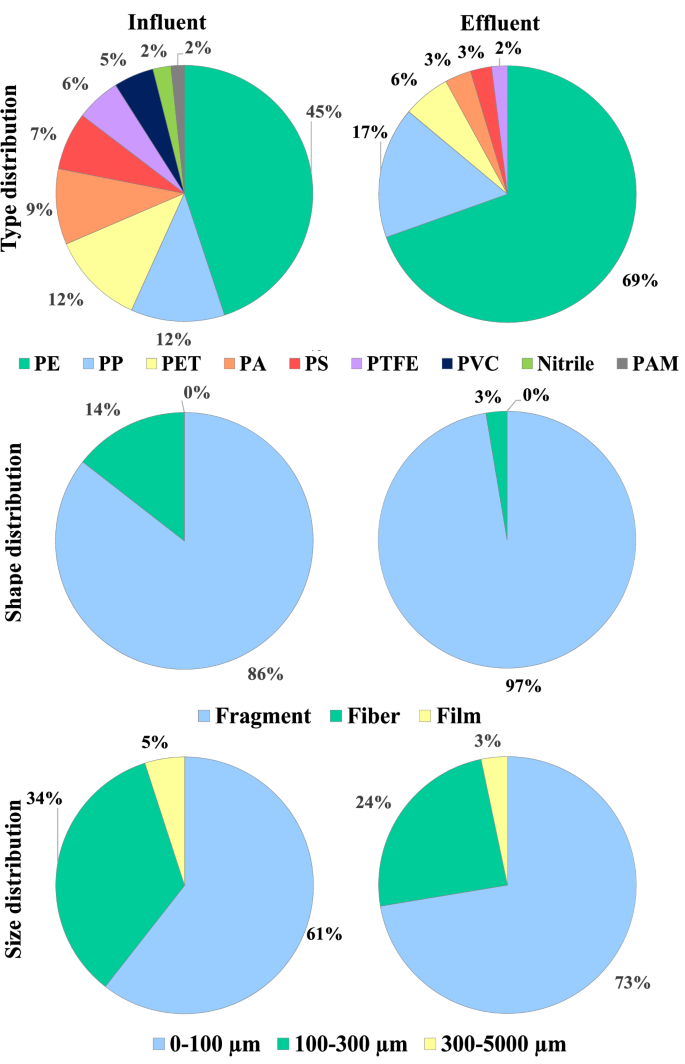
PE polyethylene, PET polyethylene terephthalate, PA polyamide, PP polypropylene, PS polystyrene, PVC polyvinyl chloride, PTFE polytetrafluoroethylene, PAM polyacrylamide.
Reuse of effluent wastewater and biosolids in agriculture is essential to face the increasing demand of water and agricultural products in combination with global warming and water scarcity 31 . Effluent wastewater and biosolids, however, are sources of emerging contaminants of concern such as viral pathogens, antibiotic resistance genes, and microplastics. The reuse of water and the release of reclaimed water into the environment may compromise public health due to the combination of several risk factors. In recent years, several publications have pointed out the low efficiency of WWTPs in removing viral pathogens 9 . While decay rates of human enteric viruses in effluents wastewater samples are frequently studied, very few studies have reported the incidence of respiratory viruses, MPs, and ARGs in effluent wastewater and biosolids, with the potential of being used in agriculture.
The present study investigated the presence of human enteric viruses, including HuNoV GI and GII, HAstV, HEV, and RV, as well as ARBs, ARGs, MPs and two novel viral faecal contamination indicators (PMMoV and crAssphage) in influent, effluent and biosolids samples. Consistent with findings from earlier research, influent wastewater samples exhibited elevated concentrations of human enteric viruses, MPs and ARBs 14 , 32 (Figs. 1 , 2 , 4 , 6 , and 8 ).
Following the water reclamation process, the concentrations of all analysed viruses decreased in the effluent samples. However, it is worth noting that the reductions for HuNoV GI, HuNoV GII, HAstV, and RV (when detected in effluent) were below 2 Logs, suggesting the persistence of these viruses to a relevant extent after being exposed to either UV or chlorination treatments. Only HEV was not detected in any of the analysed effluent samples thus resulting in higher reductions (> 4 Log GC). The reductions observed for human enteric viruses along the year substantially differ from current European legislation (Regulation (EU) 2020/741, 2020) on water reuse, which indicates the need for ≥ 6 Log decreases on the presence of these pathogens 7 . Even though enteric viruses’ presence detected by RT-qPCR in this study might not correspond with infectious particles, several publications have pointed out the presence of infectious enteric viruses in reclaimed waters by capsid-integrity or cell culture approaches 8 , 9 , 10 , 11 , 33 .
Owing to the microbiological risk that the presence of enteric viruses in these waters could entail, this study also aimed to assess the levels of somatic coliphages and E. coli in influent and effluent wastewater samples, as well as biosolid samples. Coliphages have been found in locations where faecal contamination is present 34 , 35 , and numerous studies have suggested utilizing coliphages as markers for enteric viruses’ presence 34 , 35 , 36 , 37 , 38 , 39 . Following the water treatment process, reductions of 1.83 Log PFU and 1.65 Log CFU were observed for somatic coliphages and E. coli , respectively. These reductions, which are far from those stipulated by the legislation EU 2020/741, 2020, highlight the low performance of the investigated WWTPs in decreasing the microbial load and mitigating the potential risks associated with these pathogens (pathogenicity and antibiotic resistance transmission) 7 . The high prevalence of viruses in reclaimed waters and biosolids, attributed to their high stability, poses a significant risk when applied to agricultural fields, particularly for products such as leafy greens and berries, which are often consumed raw and are unlikely to undergo extensive processing 40 . Shellfish are highly susceptible to viral contamination due to their efficient water filtration capacity, and they are commonly consumed raw or with minimal processing, making them a potential source of viral outbreak.
For somatic coliphages and E. coli , obtained counts in biosolids were similar to those obtained in effluent wastewater samples, pointing out the risk of using biosolids without any further treatment in agriculture. Besides, in recent years, both crAssphage and PMMoV have been proposed as viral indicators of faecal contamination in water bodies and as a virus model to assess the performance of WWTPs 41 , 42 , 43 , 44 , 45 , 46 , 47 . Regarding effluent samples, the mean concentration of crAssphage detected in reclaimed waters was 6.25 Log GC/L, which consistently matches the reported mean concentrations of 6.5 Log GC/L in high-income countries as reviewed by Adnan et al. 48 . PMMoV concentrations in effluent wastewater samples are in line with existing bibliography, which reports mean concentration values of ~ 4 Log GC/L 49 , 50 , 51 . Notably, obtained mean concentrations of PMMoV in influent wastewaters (5.95 Log GC/L) are slightly under-average when compared with previously reported data, as the common concentration values of PMMoV published in influent wastewater samples range from 6 to 10 Log GC/L 49 , 50 , 51 , 52 , 53 , 54 , 55 . Interestingly, to our knowledge, this study includes the first report on PMMoV levels in biosolid samples. This finding suggests a potential risk for the dissemination of this plant pathogen, which can infect solanaceous plants, ultimately leading to reduced productivity.
As for respiratory viruses, SARS-CoV-2, and IAV were detected at mean titres similar to those reported in the US, Canada, Australia, and other regions in Spain covering the same time period, while RSV levels were at least one Log GC/L over the reported in the aforementioned studies 56 , 57 , 58 , 59 , 60 , 61 . In recent years, the possibility of transmission of various respiratory viruses through food and water consumption has been discussed 62 . The absence of RSV and IAV in all effluent samples analysed in this study indicates an almost non-existent risk of transmissibility caused by ineffective water treatment, a finding of significant relevance, especially given the current situation where IAV H5N1 has been detected in sewage 63 . Nevertheless, the high presence of SARS-CoV-2 in effluent samples, together with the presence of these respiratory viruses in several of the analysed biosolids samples and the lack of studies regarding non-respiratory routes of transmission, warrant the need for further studies to assess public health risks.
Recently, a new proposal by The Urban Wastewater Treatment Directive (UWWTD), requested that member states should monitor antibiotic resistance at WWTPs serving over 100,000 individuals 19 . As this monitoring has been proposed to be performed for both influent and effluent wastewater samples, it should tackle both environmental transmission risks arising from WWTPs and provide insights into resistance patterns within specific regional areas.
In this study, ESBL- E. coli levels in influent samples were very high, with 6.63 Log CFU /100 mL on average, with no statistical differences among the different WWTPs and along the year. When analysing the reclamation treatment applied by the WWTPs, only mean reductions of 1.43 Log were observed for ESBL- E. coli , with 4.30 Log counts on average in effluent samples, which surpasses by 3 Logs the levels reported in other studies, suggesting the important role of effluent water in the dissemination of ARB in the food chain if used for irrigation and the need to improve water reclamation processes 14 , 64 , 65 . Similarly, the high levels of ESBL- E. coli in biosolids, suggest the need for further treatments before application in agriculture.
As well as resistant bacteria, the spread of ARGs needs to be addressed worldwide 13 . Thus, it is important to understand and mitigate their occurrence in different ecological systems. This study has shown the prevalence of 11 different ARGs belonging to 7 of the most widely used antibiotic groups in effluent water and biosolids 66 . Our study revealed that sulfonamide ARGs ( sul1 and sul2 ) were the genes with higher concentrations in effluents and biosolid samples. In line with previous studies, levels of sulfonamide resistance genes in effluent samples were higher than macrolide, tetracycline, and quinolone resistance genes 66 , 67 . Furthermore, sulfonamide gene levels were higher in biosolids than effluents (Fig. 5 ) as in the Mao et al. 2015 aforementioned study, highlighting the risk of biosolids as carriers of ARGs 64 . Levels of bla CTX-M , ARG that confer resistance to beta-lactamase, were 4 Log higher than levels of viable ESBL- E. coli , which could be explained by the longer persistence of DNA 68 , the presence of extracellular genetic material with bacterial surfaces, colloids, and bacteriophages, which shields it from nucleases 69 , 70 , 71 , 72 . This fact supports the idea that the dissemination of ARGs is not only carried out by viable bacteria but also by being found free in the environment or carried by other microorganisms such as bacteriophages 73 .
ARGs profiles were comparable in effluents and biosolids despite gene concentration differences except for cmlA_2 and ermB_1 . The cmlA_2 gene, which confer resistance to phenicol, was not found in any effluent samples indicating that environmental conditions, microbial populations, or the presence of contaminants in water treatment facilities may have impacted effluents but not biosolids. In March–May 2022, the ermB_1 gene was only detected in effluent samples, whereas the ermA gene, conferring resistance to macrolide-lincosamide-streptogramin B group antibiotic, was only detected in biosolid samples collected in January, consistent with previously reported data, whereas erm genes were only detected in biosolids 74 . Cold stress, which is linked with low temperatures, may increase horizontal gene transfer of ARGs, explaining this fluctuation along the year 75 . The significant presence of the ARGs and ESBL- E. coli supports assertions that land application of biosolids may disseminate ARGs to soil bacteria and demonstrate their potential introduction to food products via both irrigation and amendment 76 . Furthermore, from a One Health perspective, the dissemination of ARGs in aquatic environments may have implications for both animal and human health, underscoring the importance of enhancing reclamation processes through innovative strategies such as membrane bioreactors.
The extensive presence of MPs in wastewater sources significantly contributes to environmental contamination and poses considerable risks. In this sense, WWTPs play an important role in hindering MPs from entering water environments 77 . As observed in this work, the concentration of MPs in wastewater decreased in effluent samples as compared to influent samples, being the water treatment more efficient in removing higher size particles. The number of MPs found in the different samples agreed with those reported in the literature. Previous works investigated the abundance of MPs in urban WWTPs, with ranges of 0.28 to 3.14 × 10 4 particles/L in the influent, which significant differed from 0.01 to 2.97 × 10 2 particles/L in the effluent 78 . However, they did not refer to the removal efficiency depending on the particle size. In this work, a higher efficiency in reducing MPs (between 77-100%) of higher particle size (S)-MPs has been observed, which was similar to the 88–94% efficiency of municipal WWTPs previously reported 79 . However, this value was significantly reduced for MPs with smaller particle size (S)-MPs and presented a great variability depending on the WWTP studied (4-59%). Deng et al. (2023) reported that the removal efficiency of MPs in a petrochemical WWTPs reached ̴ 92% and highlighted that the primary treatment removed most of the MPs 80 (87.5%). Talvitie et al. (2015) also stated that the primary treatment could remove most of the MPs, although they did not refer to their particle size 81 . They reported that the major part of the fibers can be removed already in primary sedimentation process, which agreed with the lower proportion of fibers (as compared to fragments) found in these samples. While some authors have indicated that removing MPs from wastewater is technically feasible and cost-effective, suggesting that membrane bioreactors and sludge incineration are the best options, further research is necessary to enhance processes within a circular economy framework 82 .
Concerning the type of polymers detected, there is a higher prevalence of PE, PET, PS, and PA, as it has been previously reported for drinking water and petrochemical and urban WWTPs 80 , 83 , 84 , 85 . Furthermore, WWTPs were more efficient in removing polymers with higher density such as PA and PET, probably during the density separation step, favouring a significant reduction of these polymers in the effluent wastewater. Furthermore, the size of more than 90% of microplastic particles detected in WWTPs ranged between 1 and 300 μm and fragments were found to be the most prevalent shape of microplastics, in agreement with other works 86 .
Within this context, MPs release into the environment through sludge and effluent wastewater can also pose another risk, since MPs can accumulate/transport harmful pollutants, posing concerns about their role in treatment resistance and disease spread 87 . Bacteria and viruses have been reported to adsorb onto MPs, forming plastispheres 88 . Pathogenic bacteria, including those harmful to humans and fish, have also been found in communities of MPs 89 , 90 , 91 . Regarding viruses, the primary interaction with MPs involves electrostatic adhesion, increasing the risk of waterborne viral transmission. These viral or bacterial plastispheres not only resist UV treatment but can also promote infections, as shown for polystyrene MPs, which have been observed to facilitate IAV infection of host cells 91 , 92 . Additionally, the persistence of pathogen-carrying MPs in aquatic environments raises concerns about reverse zoonosis, where these plastispheres might be ingested by aquatic organisms, potentially endangering human populations through the food chain 93 . In summary, MPs can act as carriers for pathogenic bacteria and viruses in municipal sewage, intensifying concerns about public health and the environment.
The wide distribution of MPs in wastewater sources and the capability of some viruses to remain intact after traditional tertiary treatment disinfection processes (UV and chlorination) undoubtedly bring about environmental pollution and risk. Regarding MPs, their removal before reaching environmental water courses is highly recommended. To overcome these problems, several researchers are focused on finding cutting-edge methods to improve the efficiency of microplastic removal rates in WWTPs, although the literature is still scarce. Nasir et al. 2024, have recently reviewed innovative technologies for the removal of microplastics, highlighting the use of a membrane bioreactor system which combines biological treatments (aerobic, anaerobic) with membrane technology, thus improving sludge separation and effluent quality as compared to traditional methods 94 . Al-Amir et al., 2024 proposed the use of ultrafiltration in WWTP. It consists on a low-pressure (1–10 bar) method that removes particles using perforated asymmetric membranes up to 1–100 μm 95 . In the case of viruses, over the past few decades, as reviewed by Ibrahim et al. 2021 and Al-Hazmi et al. 2022, several efforts have been made to employ membrane-based and other hybrid technologies to effectively eliminate waterborne enteric viruses 96 , 97 . Technologies such as microfiltration (MF), ultrafiltration (UF), and membrane bioreactors (MBR) have been widely applied. The major concerns with these technologies are the factors impacting membrane performance regarding virus removal efficiency and sustainable operation, including physical sieving, adsorption, cake layer formation, and changes in membrane fouling. Additionally, microalgae-based approaches have emerged as a biological alternative to energy-intensive and expensive disinfection techniques 98 . Utilising microalgal processes, in conjunction with natural temperature, pH, or light conditions in treatment systems, may facilitate the complete removal of viruses from wastewater. Also, enhancing systems to filter out particles of extremely small sizes, such as MPs or viruses, from reclaimed water increases protection against other potentially harmful contaminants, including pathogenic bacteria. Finally, despite these treatment methods having various advantages and disadvantages, combining these systems aims to overcome their known technical and economic limitations
Overall, the findings of this research underscore the potential threats to public health associated with the reuse and release of reclaimed water, particularly concerning microbiological pathogens and environmental pollutants like microplastics, as well as the release of emerging contaminants into the environment and food chain through the use of biosolids in agriculture. These risk factors, including the persistence of enteric viruses, the inadequate reduction of microbial load and antibiotic resistance genes, and the prevalent presence of microplastics, emphasize the need for a holistic approach in addressing health concerns. Integrating these insights from wastewater analysis as well as human epidemic respiratory viruses monitoring into the broader One Health framework is crucial for devising effective policies, improving water treatment processes, and safeguarding both human and ecosystem health in a sustainable manner.
Methods for viruses and ARGs in wastewater and biosolid samples
Grab influent ( n = 72) and effluent ( n = 72) wastewater samples were collected monthly along with dehydrated biosolid samples ( n = 72) from 6 different urban WWTPs over a one-year period (January 2022–December 2022). Samples were grabbed early in the morning (8 am) by collecting ~500 mL of wastewater in sterile HDPE plastic containers (Labbox Labware, Spain). Collected samples were transferred on ice to the laboratory, kept refrigerated at 4 °C, and concentrated within 24 h. Samples were artificially contaminated with 10 6 PCR units (PCRU) of porcine epidemic diarrhea virus (PEDV) strain CV777, serving as a coronavirus model. Additionally, 10 6 PCRU of mengovirus (MgV) vMC 0 (CECT 100,000) were used as a non-enveloped counterpart for recovery efficiency assessment. Effluent wastewater samples were concentrated through a previously validated aluminium-based adsorption-precipitation method 11 , 99 . Briefly, 200 mL of sample was adjusted to pH 6.0 and Al(OH) 3 precipitate formed by adding 1 part 0.9 N AlCl 3 solution to 100 parts of sample. Then, pH was readjusted to 6.0 and sample mixed using an orbital shaker at 150 rpm for 15 min at room temperature. Next, viruses and ARGs were collected by centrifugation at 1700 × g for 20 min. The pellet was resuspended in 10 mL of 3% beef extract pH 7.4, and samples were shaken for 10 min at 150 rpm. Finally, the concentrate was recovered by centrifugation at 1900 × g for 30 min and the pellet was resuspended in 1 ml of phosphate buffer saline (PBS) and stored at −80 °C. Alternatively, 40 mL of influent wastewater samples were processed with the Enviro Wastewater TNA Kit (Promega Corp., Spain) vacuum concentration system following the manufacturer’s instructions 100 . For biosolid samples, 0.1 g of biosolid were resuspended in 900 µL PBS for nucleic acid extraction prior to PCR analyses.
Nucleic acid extraction from influent and effluent wastewater concentrates and biosolid suspensions was performed by using the Maxwell® RSC Instrument (Promega, Spain) with the Maxwell RSC Pure Food GMO for viral and ARG extraction. Specific programs, namely ‘Maxwell RSC Viral Total Nucleic Acid’ and ‘PureFood GMO and Authentication,’ were employed for viral and ARG extractions, respectively.
Virus detection and quantification
The detection of process control viruses, PEDV and MgV, was carried out through RT-qPCR using the One Step PrimeScript™ RT-PCR Kit (Perfect Real Time) (Takara Bio Inc., USA) as detailed elsewhere 101 . Levels of HuNoV GI and GII, HAstV, RV, HAV, and HEV were determined using the RNA UltraSense One-Step kit (Invitrogen, USA), following previously described procedures 9 , 11 . The occurrence of crAssphage was established using the qPCR Premix Ex Taq™ kit (Takara Bio Inc) 102 . PMMoV detection was determined using the PMMoV Fecal Indicator RT-qPCR Kit (Promega, Spain) following the manufacturer’s instructions. SARS-CoV-2 detection was performed by targeting the N1 region of the nucleocapsid gene. The One Step PrimeScript™ RT-PCR Kit (Perfect Real Time) was used with N1 primers and conditions described by CDC 103 . IAV detection followed the protocol described by CDC (2009) using primers from CDC (2020) and the One Step PrimeScript™ RT-PCR Kit (Perfect Real Time) 104 .
Different controls were used in all assays: negative process control consisting of PBS; whole process control to monitor the process efficiency of each sample (spiked with PEDV and MgV); and positive (targeted gene reference material) and negative (RNase-free water) RT-qPCR controls. The recoveries of PEDV and MgV, spiked as enveloped and non-enveloped viral process controls, respectively, ranged between 6.31 and 59.65% (data not included). The validation of results for targeted viruses adhered the criteria specified in ISO 15216-1:2017, where a recovery of the process control of ≥1% is required 105 .
Commercially available gBlock synthetic gene fragments (Integrated DNA Technologies, Inc., USA) of HuNoVs GI and GII, HAstV, RV, HAV, HEV, and crAssphage were used to prepare standard curves for quantification. For IAV and RSV quantification, Twist Synthetic InfluenzaV H1N1 RNA control (Twist BioScience, South San Francisco, CA, USA), and purified RNA of RSV (Vircell, S.L., Spain) were used. The PMMoV Fecal Indicator RT-qPCR Kit (Promega) provided PMMoV RNA for generating a standard curve. A table, featuring primers, probes, PCR conditions, limit of quantification (LOQ/L), and limit of detection (LOD/L) for all targeted viruses in this work is available in the Supplementary materials (Supplementary Table 1 ).
Quantification of viable somatic coliphages, E. coli , and Extended Spectrum Beta-Lactamases producing E. coli
One mL of influent and effluent samples was filtered through sterile 0.45 μm pore syringe filters (Labbox Labware, S.L., Spain) to remove bacteria and fungus 106 . Phage enumeration was performed by plaque counting using the commercial Bluephage Easy Kit for Enumeration of Somatic Coliphages (Bluephage S.L., Spain), following manufacturer’s instructions. For biosolid samples, 1 g of biosolid was resuspended in 100 mL PBS for both somatic coliphages and E. coli enumeration.
For all water and biosolid samples, E. coli and ESBL- E. coli enumeration was assessed by using selective culture media Chromocult coliform agar (Merck, Darmstadt, Germany) and CHROMagar ESBL (CHROMagar, Paris, France), respectively. Spread plating (0.1 mL) or membrane filtration (200 mL) was used depending on the anticipated bacterial concentration. Influent wastewater samples were diluted serially, and 0.1 mL aliquots were spread-plated. Effluent samples were filtered through a 0.45 μm cellulose nitrate membrane filter (Sartorius, Madrid, Spain). Following incubation at 37 °C for 24 hours, results were interpreted, with. dark blue-violet colonies considered positive for E. coli and dark pink-reddish colonies considered positive for ESBL- E. coli . The analysis was performed in duplicate, and the results were expressed as CFU/100 mL. The detection limit (LOD) for E. coli and ESBL- E. coli counts in the influent and biosolid samples was 2.0 Log CFU/100 mL (100 CFU/100 mL), while in the effluents, the LOD was 0 Log CFU/100 mL (1 CFU/100 mL).
Detection and quantification of antimicrobial resistance genes in effluent waters and biosolids
In this study, 11 ARGs that confer resistance to Sulfonamides ( sul1 , sul2_ 1), beta-lactamase ( pbp2b , bla CTX- M ), phenicols ( cmlA_2 ), nitroimidazoles ( nimE ), MLSB ( ermB_ 1, ermA ), tetracyclines ( tetPB_3 , tetA_1 ) and fluoroquinolones ( qacA_1 ), were only detected in effluent waters and biosolids. The 16 S rRNA gene was used as positive control for qPCR measurement. Quantification of the 12 selected genes was performed by high-throughput quantitative PCR (HT-qPCR) using the SmartChip™ Real-Time PCR system (TakaraBio, CA, USA) by Resistomap Oy (Helsinki, Finland). qPCR cycling conditions and processing of raw data were described elsewhere 107 , 108 , 109 , 110 . Each DNA sample was analysed in duplicate. Data processing and analysis were performed by using a python-based script by Resistomap Oy (Helsinki, Finland) 100 , 111 .
Digestion of organic material and isolation of MPs
Initial steps consisted on optimizing the protocol for the removal of organic material and the isolation of the maximum number of MPs from wastewater and biosolid samples. Different volumes of water, amounts of biosolids and digestion strategies for organic biomass removal were tested to remove the greatest amount of organic material without compromising the integrity of the MPs. Avoiding filter clogging was a requirement during the methodology development, to facilitate further identification of MPs. To reduce the risk of external contamination by MPs, laboratory consumables made of glass were used, the reagents were purified by filtering through a 0.2 µm pore size nitrocellulose filter (Whatman, Maidstone, UK), 100% cotton lab aprons were used, samples were processed in a laminar flow cabinet, the beakers were covered with a watch glass, disposable nitrile gloves were used and, before and after using the material, all used materials were rinsed thoroughly with deionized water. In order to assure that the isolation of MPs was effective and external contamination did not occur, a negative control (NC) was included every month and a positive control (PC) was carried out every 3 months. The positive control was made with fluorescent polystyrene microspheres (Invitrogen, Waltham, USA) of 1 µm in diameter. Specifically, a solution of 1000 beads/20 µL was prepared and 20 µL of this solution was incorporated before the pre-treatment and, the number of remaining microbeads after the digestion protocol was determined to calculate the percentage of recovery. The average value of particle recovery was 93.9%.
Two different pre-treatment protocols were finally defined:
(1) Sieved > 300 µm or (S): With this pre-treatment, all solid particles (including MPs) larger than 300 µm were isolated from 2 L of wastewater or 5 g of biosolid samples after sieving, oxidative digestion, and filtration steps.
(2) Total Particles or (T): With this pre-treatment all solid particles (including MPs) with a size between 1 µm and 5 mm were isolated from a 10 mL aliquot of wastewater after oxidative digestion, density separation, and filtration steps.
Through protocol (S), a larger and more representative amount of wastewater was treated, but particles smaller than 300 µm were lost. In the other hand, protocol (T) allowed the analysis of particles down to 1 µm in size, but the amount of analysed wastewater was much smaller to avoid filter clogging.
In both protocols (S) and (T), oxidative digestion was performed to remove organic material, adapting the method described by the National Oceanic and Atmospheric Administration (NOAA) 112 .
In the case of the Sieved 300 µm or (S) protocol (Figs. 11 ), 2L of wastewater or 5 g of biosolids were treated. The 5 g of biosolids were previously dispersed in 100 mL of ultrapure MilliQ water by applying stirring and heat during 30 minutes at 30 °C. The wastewater or biosolid dispersion were subsequently poured through a 300 µm mesh stainless steel sieve. The retained particles were collected by washing with MilliQ water into a beaker and digested by adding an equivalent volume of NaClO (14%, VWR chemical, USA). After heating at 75 °C for 3 h under stirring, the sample was sieved again to remove the disaggregated smallest particles. The particles retained on the sieve were collected by washing with MilliQ water on a 0.8 µm pore size nitrocellulose filter (Whatman, USA). The filter was protected from external contamination between a microscope glass slide and a glass cover, and finally dried at 40 °C for 24 h in a convection oven.
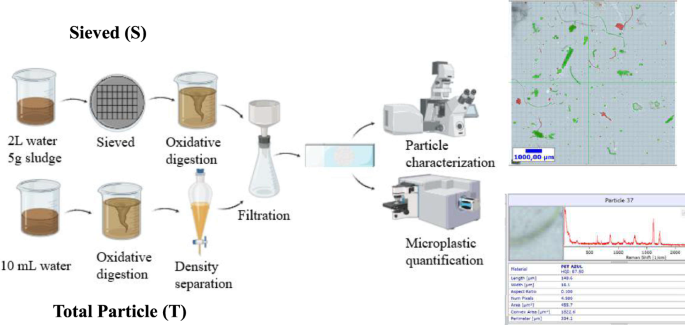
Scheme summary of the methodology used for the isolation, quantification, and identification of microplastics (MPs).
In the case of the Total Particles or (T) protocol, an oxidative digestion (Fenton reaction) was performed on a 10 mL wastewater sample by adding 20 mL of a H 2 O 2 (30%, Sigma- Aldrich, USA) solution and 20 mL of a 0.05 M Fe (II) solution prepared by mixing FeSO 4 (Sigma- Aldrich, USA), H 2 SO 4 (96%, PanReac AppliChem, ITW Reagents, USA) and deionized water. The sample was then heated at 75 °C for 30 min under stirring. The digestion step was repeated if any remaining organic material was visually. Thereafter, a density separation was performed after adding NaCl (99.5%, Sigma- Aldrich, USA) until saturation. Subsequently, the sample was left to sediment for 30 min in a separatory funnel and the supernatant was filtered through a 0.8 µm pore size nitrocellulose filter (Whatman, USA) under vacuum. The filter was also protected between glass slide and coverslip and dried at 40 °C for 24 hours.
Characterization of particles present in biosolid and wastewater samples
Filters obtained after pre-treatment protocols (S) and (T) were photographed using an EVOCAM II macrophotography equipment (Vision Engineering, Woking, UK) and the ViPlus software (2018, Vision Engineering). Two partially overlapping 2MPx color photos were taken for each filter, always at 20x magnification, with half of the filter appearing in each photo. These images were fused by digital stitching techniques using the mosaic J command of the FIJI software (ImageJ 1.49q Software, National Institutes of Health, USA). Each image showed a 25*15 mm field of view. The pixel size was 13.3 microns, obtaining an image to calibrate in each photo session to have precise external calibration data. A rough quantification was performed, and all particles, including MPs, were characterized using the Nis Elements BR 3.2 software (Nikon Corporation, Japan). To achieve this, a macro of programmed actions was designed in which, firstly, the pixel size was calibrated in the complete image of the filter, then a matrix-iterative detection tool for particles less bright than the filter was applied, which facilitated a binary segmentation by brightness levels and achieve the selection of the particles of each filter in an automated way, only in the filtration zone. Finally, the data of all the particles were exported to obtain the count and the different morphological values of numerous parameters and perform the statistical calculations.
For the characterization, the particles were classified into 3 size ranges of 1–100 µm, 100–300 µm and 300-5000 µm. The particles were also classified according to their circularity, calculated from the measured perimeter and area of each particle according to Eq. 1 , in 3 ranges: 0-0.4, 0.4–0.8, and 0.8-1. A circularity value of 1.0 indicates a perfect circle. As the value approaches 0.0, it indicates an increasingly elongated polygon. Particles with a circularity less than 0.4 were considered as fibers.
In addition, the efficiency of WWTPs in removing particles was calculated according to the following equation:
Where: Efficiency = particle removal efficiency (%); influent = number of particles detected at the WWTP influent; effluent = number of particles detected at the WWTP effluent.
Quantification of microplastics present in biosolid and wastewater samples
Quantification, identification, and characterization of MPs was carried out only on samples from the odd months. The analysis was performed using an automated Raman microscope Alpha300 apyron (Witec, Ulm, Germany). First, each filter was mapped by acquiring a total of 1089 images, which after reconstruction represented a 27% of the filter area or 1 cm 2 . The present particles were detected and selected by performing image analysis using the ParticleScout 6.0 software in automatic mode.
After particle selection, analysis on each particle by Raman spectroscopy and subsequent identification were carried out. The optimal conditions for Raman spectra acquisition were as follows: 785 nm laser which facilitates to identify fluorescent particles, 300 lines/mm diffraction grating opening, spectral range between 0 and 3000 cm –1 , 10 accumulations, 0.2 second acquisition time, and 40 mW laser power. The spectrum of each particle was registered and compared with an in-house build spectral library of polymers. The reference polymer materials included in the spectral library were polyethylene (PE), polyethylene terephthalate (PET), polyamide (PA), polypropylene (PP), polystyrene (PS), polyvinyl chloride (PVC), polytetrafluoroethylene (PTFE), polyacrylamide (PAM), Polyarylsulfones (PSU), Polymethylmethacrylate (PMMA), nitrile rubber (NBR), Cellophane and Melamine. Particles that had a 75% or better match (HQI) between the sample and reference spectra were identified as composed of the same material or of a similar chemical nature. In addition, a visual inspection was carried out and the spectrum acquisition was repeated on the particles where a clear identification was not initially possible. Three rules were considered to discriminate between plastics and non-plastics and to prioritize the particles to be analysed: (i) the object must not show cellular or natural organic structures; (ii) the fibre thickness must be uniform along the entire length; (iii) the colour of the particles must be clear and homogeneous 113 . The MPs already identified were classified based on material type, size, morphology, and area.
Statistical analysis
Results were statistically analysed and significance of differences was determined on the ranks with a one-way analysis of variance (ANOVA) and Tukey’s multiple comparison tests. In all cases, a value of p < 0.05 (confidence interval 95%) was deemed significant.
Data availability
The datasets used and/or analysed during the current study available from the corresponding author on reasonable request.
Barcelo, D., & Petrovic, M. The Handbook of Environmental Chemistry (D. Barcel & Andrey G. Kostianoy, Eds.; Springer) (2011).
FAO. Water for Sustainable Food and Agriculture A report produced for the G20 Presidency of Germany. Retrieved 17 October 2023, from www.fao.org/publications (2017).
K Mishra, R., Mentha, S. S., Misra, Y., & Dwivedi, N. Emerging pollutants of severe environmental concern in water and wastewater: A comprehensive review on current developments and future research. https://doi.org/10.1016/j.wen.2023.08.002 (2023).
Oude Munnink, B. B., & van der Hoek, L. Viruses causing gastroenteritis: the known, the new and those beyond. Viruses, 8 . https://doi.org/10.3390/v8020042 (2016).
Bosch, A., Guix, S., Sano, D. & Pintó, R. M. New tools for the study and direct surveillance of viral pathogens in water. Curr. Opin. Biotechnol. 19 , 295–301 (2008).
Article CAS Google Scholar
Okoh, A. I., Sibanda, T. & Gusha, S. S. Inadequately treated wastewater as a source of human enteric viruses in the environment. Int. J. Environ. Res. Public Health 7 , 2620–2637 (2010).
EC, E. C. Regulation (EU) 2020/741 of The European Parliament and of the Council of 25 May 2020 on minimum requirements for water reuse (Text with EEA relevance). Off. J. Eur. Union , L, 177 , 32–55(63) (2020).
Canh, V. D., Torii, S., Furumai, H. & Katayama, H. Application of Capsid Integrity (RT-)qPCR to assessing occurrence of intact viruses in surface water and tap water in Japan. Water Res. 189 , 116674 (2021).
Cuevas-Ferrando, E., Pérez-Cataluña, A., Falcó, I., Randazzo, W., & Sánchez, G. Monitoring human viral pathogens reveals potential hazard for treated wastewater discharge or reuse. Front. Microbiol., 13 . https://doi.org/10.3389/FMICB.2022.836193 (2022).
Gyawali, P. & Hewitt, J. Detection of infectious noroviruses from wastewater and seawater using PEMAXTM treatment combined with RT-qPCR. Water 10 , 841 (2018).
Article Google Scholar
Randazzo, W. et al. Interlaboratory comparative study to detect potentially infectious human enteric viruses in influent and effluent waters. Food Environ. Virol. 11 , 350–363 (2019).
Truchado, P. et al. Monitoring of human enteric virus and coliphages throughout water reuse system of wastewater treatment plants to irrigation endpoint of leafy greens. Sci. Total Environ. 782 , 146837 (2021).
One Health Initiative (n.d.). Retrieved 17 October 2023, from www.archive.onehealthinitiative.com/index.php .
Oliveira, M. et al. Surveillance on ESBL- Escherichia coli and Indicator ARG in wastewater and reclaimed water of four regions of Spain: Impact of different disinfection treatments. Antibiotics , 12. https://doi.org/10.3390/ANTIBIOTICS12020400 (2023).
Schwartz, T., Kohnen, W., Jansen, B. & Obst, U. Detection of antibiotic-resistant bacteria and their resistance genes in wastewater, surface water, and drinking water biofilms. FEMS Microbiol. Ecol. 43 , 325–335 (2003).
Koutsoumanis, K. et al. Role played by the environment in the emergence and spread of antimicrobial resistance (AMR) through the food chain. EFSA J. 19 , e06651 (2021).
CAS Google Scholar
Gajdoš, S. et al. Synergistic removal of pharmaceuticals and antibiotic resistance from ultrafiltered WWTP effluent: Free-floating ARGs exceptionally susceptible to degradation. J. Environ. Manag. 340 , 117861 (2023).
PlasticsEurope. Plastics-the Facts 2020. An analysis of European plastics production, demand, and waste data. Retrieved 12 September 2023. https://plasticseurope.org/wp-content/uploads/2021/09/Plastics_the_facts-WEB-2020_versionJun21_final.pdf (2020).
EC. Proposal for a revised Urban Wastewater Treatment Directive. Retrieved 10 November 2023, from https://environment.ec.europa.eu/publications/proposal-revised-urban-wastewater-treatment-directive_en (2022).
Wang, L. et al. Environmental fate, toxicity and risk management strategies of nanoplastics in the environment: Current status and future perspectives. J. Hazard. Mater. 401 , 123415 (2021).
Pironti, C., et al. Microplastics in the environment: intake through the food web, human exposure and toxicological effects. Toxics , 9 . https://doi.org/10.3390/TOXICS9090224 (2021).
Fackelmann, G. & Sommer, S. Microplastics and the gut microbiome: How chronically exposed species may suffer from gut dysbiosis. Mar. Pollut. Bull. 143 , 193–203 (2019).
Jenner, L. C. et al. Detection of microplastics in human lung tissue using μFTIR spectroscopy. Sci. Total Environ. 831 , 154907 (2022).
Ibrahim, Y. S. et al. Detection of microplastics in human colectomy specimens. JGH Open 5 , 116–121 (2021).
Zhu, L. et al. Identification of microplastics in human placenta using laser direct infrared spectroscopy. Sci. Total Environ. 856 , 159060 (2023).
Leslie, H. A. et al. Discovery and quantification of plastic particle pollution in human blood. Environ. Int. 163 , 107199 (2022).
Bowley, J., Baker-Austin, C., Porter, A., Hartnell, R. & Lewis, C. Oceanic Hitchhikers – assessing pathogen risks from marine microplastic. Trends Microbiol. 29 , 107–116 (2021).
Bouwmeester, H., Hollman, P. C. & Peters, R. J. Potential health impact of environmentally released micro- and nanoplastics in the human food production chain: experiences from nanotoxicology. Environ. Sci. Technol. 49 , 8932–8947 (2015).
Grindler, N. M. et al. Exposure to Phthalate, an endocrine disrupting chemical, alters the first trimester placental methylome and transcriptome in women. Sci. Rep 8 , 1–9 (2018).
Sadia, M. et al. Microplastics pollution from wastewater treatment plants: A critical review on challenges, detection, sustainable removal techniques and circular economy. Environ. Technol. Innov. 28 , 102946 (2022).
Zhu, L. et al. Quantifying health risks of plastisphere antibiotic resistome and deciphering driving mechanisms in an urbanizing watershed. Water Res. 245 , 120574 (2023).
Vermi, M., et al. Viruses in wastewater: occurrence, abundance and detection methods. https://doi.org/10.1016/j.scitotenv.2020.140910 (2020).
Simmons, F. J. & Xagoraraki, I. Release of infectious human enteric viruses by full-scale wastewater utilities. Water Res. 45 , 3590–3598 (2011).
AWPRC. Bacteriophages as model viruses in water quality controlag. Water Res. 25 , 529–545 (1991).
Grabow, W. O. K. Bacteriophages: update on application as models for viruses in water. Water SA 27 , 251–268 (2001).
Google Scholar
Funderburg, S. W. & Sorber, C. A. Coliphages as indicators of enteric viruses in activated sludge. Water Res. 19 , 547–555 (1985).
Kott1, Y. Estimation of low numbers of Escherichia coli bacteriophage by use of the most probable number method. Appl. Microbiol . https://journals.asm.org/journal/am (1966).
Lucena, F. et al. Reduction of bacterial indicators and bacteriophages infecting faecal bacteria in primary and secondary wastewater treatments. J. Appl. Microbiol. 97 , 1069–1076 (2004).
Ueda, T. & Horan, N. J. Fate of indigenous bacteriophage in a membrane bioreactor. Water Res. 34 , 2151–2159 (2000).
Sánchez G., Bosch A. Survival of enteric viruses in the environment and food. Viruses Foods . 26 :367–392. https://doi.org/10.1007/978-3-319-30723-7_13 (2016).
Bivins, A. et al. Cross-assembly phage and pepper mild mottle virus as viral water quality monitoring tools—potential, research gaps, and way forward. Curr. Opin. Environ. Sci. Health 16 , 54–61 (2020).
Farkas, K. et al. Critical evaluation of CrAssphage as a molecular marker for human-derived wastewater contamination in the aquatic environment. Food Environ. Virol. 11 , 113–119 (2019).
García-Aljaro, C., Ballesté, E., Muniesa, M. & Jofre, J. Determination of crAssphage in water samples and applicability for tracking human faecal pollution. Microb. Biotechnol. 10 , 1775–1780 (2017).
Kitajima, M., Iker, B. C., Pepper, I. L. & Gerba, C. P. Relative abundance and treatment reduction of viruses during wastewater treatment processes - Identification of potential viral indicators. Sci. Total Environ. 488–489 , 290–296 (2014).
Symonds, E. M., Rosario, K. & Breitbart, M. Pepper mild mottle virus: Agricultural menace turned effective tool for microbial water quality monitoring and assessing (waste)water treatment technologies. PLOS Pathog. 15 , e1007639 (2019).
Tandukar, S., Sherchan, S. P. & Haramoto, E. Applicability of crAssphage, pepper mild mottle virus, and tobacco mosaic virus as indicators of reduction of enteric viruses during wastewater treatment. Sci. Rep. 10 , 3616 (2020).
Wu, Z., Greaves, J., Arp, L., Stone, D. & Bibby, K. Comparative fate of CrAssphage with culturable and molecular fecal pollution indicators during activated sludge wastewater treatment. Environ. Int. 136 , 105452 (2020).
Sabar, M. A., Honda, R. & Haramoto, E. CrAssphage as an indicator of human-fecal contamination in water environment and virus reduction in wastewater treatment. Water Res 221 , 118827 (2022).
Kitajima, M., Sassi, H. P. & Torrey, J. R. Pepper mild mottle virus as a water quality indicator. Npj Clean. Water 2018 1 , 1–9 (2018).
Rosario, K., Symonds, E. M., Sinigalliano, C., Stewart, J., & Breitbart, M. Pepper Mild Mottle Virus as an indicator of fecal pollution. Appl. Environ. Microbiol. , 75 , 7261. https://doi.org/10.1128/AEM.00410-09 (2009a).
Symonds, E. M., Nguyen, K. H., Harwood, V. J. & Breitbart, M. Pepper mild mottle virus: A plant pathogen with a greater purpose in (waste)water treatment development and public health management. Water Res. 144 , 1–12 (2018).
Hamza, I. A., Jurzik, L., Überla, K. & Wilhelm, M. Evaluation of pepper mild mottle virus, human picobirnavirus and Torque teno virus as indicators of fecal contamination in river water. Water Res. 45 , 1358–1368 (2011).
Gyawali, P., Croucher, D., Ahmed, W., Devane, M. & Hewitt, J. Evaluation of pepper mild mottle virus as an indicator of human faecal pollution in shellfish and growing waters. Water Res. 154 , 370–376 (2019).
Kuroda, K. et al. Pepper mild mottle virus as an indicator and a tracer of fecal pollution in water environments: Comparative evaluation with wastewater-tracer pharmaceuticals in Hanoi, Vietnam. Sci. Total Environ. 506–507 , 287–298 (2015).
Schmitz, B. W., Kitajima, M., Campillo, M. E., Gerba, C. P. & Pepper, I. L. Virus Reduction during Advanced Bardenpho and Conventional Wastewater Treatment Processes. Environ. Sci. Technol. 50 , 9524–9532 (2016) .
Ando, H. et al. Impact of the COVID-19 pandemic on the prevalence of influenza A and respiratory syncytial viruses elucidated by wastewater-based epidemiology. Sci. Total Environ. , 880 . https://doi.org/10.1016/J.SCITOTENV.2023.162694 (2023).
Boehm, A. B. et al. More than a Tripledemic: Influenza A Virus, Respiratory Syncytial Virus, SARS-CoV-2, and human metapneumovirus in wastewater during winter 2022-2023. Environ. Sci. Technol. Lett. 10 , 622–627 (2023).
Hughes, B. et al. Respiratory Syncytial Virus (RSV) RNA in Wastewater Settled Solids Reflects RSV Clinical Positivity Rates. Environ. Sci. Technol. Lett. 9 , 173–178 (2022).
Mercier, E. et al. (123 C.E.). Municipal and neighbourhood level wastewater surveillance and subtyping of an influenza virus outbreak. Sci. Rep. , 12 , 15777.
Toribio-Avedillo, D. et al. Monitoring influenza and respiratory syncytial virus in wastewater. Beyond COVID-19. Sci. Total Environ. 892 , 164495 (2023).
Wolfe, M. K. et al. Wastewater-based detection of two influenza outbreaks. Environ. Sci. Technol. Lett. 2022 , 687–692 (2022).
Guo, M., Tao, W., Flavell, R. A. & Zhu, S. Potential intestinal infection and faecal-oral transmission of SARS-CoV-2. Nat. Rev. Gastroenterol. Hepatol. 18 , 269–283 (2021).
Detection of hemagglutinin H5 influenza A virus sequence in municipal wastewater solids at wastewater treatment plants with increases in influenza A in spring, 2024. Marlene K. Wolfe, Dorothea Duong, Bridgette Shelden, View ORCID ProfileElana M. G. Chan, Vikram Chan-Herur, Stephen Hilton, Abigail Harvey Paulos, Alessandro Zulli, Bradley J. White, View ORCID ProfileAlexandria B. Boehm. https://doi.org/10.1101/2024.04.26.24306409 .
Nzima, B. et al. Resistotyping and extended-spectrum beta-lactamase genes among Escherichia coli from wastewater treatment plants and recipient surface water for reuse in South Africa. https://doi.org/10.1016/j.nmni.2020.100803 (2020).
Raven, K. E., et al. Genomic surveillance of Escherichia coli in municipal wastewater treatment plants as an indicator of clinically relevant pathogens and their resistance genes. Microb. Genomics , 5. https://doi.org/10.1099/mgen.0.000267 (2019).
Mao, D. et al. Prevalence and proliferation of antibiotic resistance genes in two municipal wastewater treatment plants. Water Res. 85 , 458–466 (2015).
Christgen, B. et al. Metagenomics shows that low-energy anaerobic−aerobic treatment reactors reduce antibiotic resistance gene levels from domestic wastewater. https://doi.org/10.1021/es505521w (2015).
Gekenidis, M. T., Rigotti, S., Hummerjohann, J., Walsh, F. & Drissner, D. Long-term persistence of blaCTX-M-15 in soil and lettuce after introducing extended-Spectrum β-Lactamase (ESBL)-producing Escherichia coli via manure or water. Microorganisms 2020 8 , 1646 (2020).
Bryzgunova, O. E. et al. Redistribution of free- and cell-surface-bound DNA in blood of benign and malignant prostate tumor patients. Acta Nat. 7 , 115 (2015). /pmc/articles/PMC4463421/.
Laktionov, P. P. et al. Cell-surface-bound nucleic acids: Free and cell-surface-bound nucleic acids in blood of healthy donors and breast cancer patients. Ann. N. Y. Acad. Sci. 1022 , 221–227 (2004).
Nagler, M., Insam, H., Pietramellara, G. & Ascher-Jenull, J. Extracellular DNA in natural environments: features, relevance and applications. Appl. Microbiol. Biotechnol. 2018 102 , 6343–6356 (2018).
Zhang, Y., Snow, D. D., Parker, D., Zhou, Z. & Li, X. Intracellular and extracellular antimicrobial resistance genes in the sludge of livestock waste management structures. Environ. Sci. Technol. 47 , 10206–10213 (2013).
Muniesa, M., Colomer-Lluch, M. & Jofre, J. Potential impact of environmental bacteriophages in spreading antibiotic resistance genes. Future Microbiol 8 , 739–751 (2013).
Szczepanowski, R. et al. Detection of 140 clinically relevant antibiotic-resistance genes in the plasmid metagenome of wastewater treatment plant bacteria showing reduced susceptibility to selected antibiotics. Microbiology 155 , 2306–2319 (2009).
Miller, J. H., Novak, J. T., Knocke, W. R. & Pruden, A. Elevation of antibiotic resistance genes at cold temperatures: implications for winter storage of sludge and biosolids. Lett. Appl. Microbiol. 59 , 587–593 (2014).
Xu, S., Liu, Y., Wang, R., Zhang, T. & Lu, W. Behaviors of antibiotic resistance genes (ARGs) and metal resistance genes (MRGs) during the pilot-scale biophysical drying treatment of sewage sludge: Reduction of ARGs and enrichment of MRGs. Sci. Total Environ. 809 , 152221 (2022).
Enfrin, M., Dumée, L. F. & Lee, J. Nano/microplastics in water and wastewater treatment processes – Origin, impact and potential solutions. Water Res. 161 , 621–638 (2019).
Liu, W. et al. A review of the removal of microplastics in global wastewater treatment plants: Characteristics and mechanisms. Environ. Int. 146 , 106277 (2021).
Iyare, P. U., Ouki, S. K. & Bond, T. Microplastics removal in wastewater treatment plants: a critical review. Environ. Sci.: Water Res. Technol. 6 , 2664–2675 (2020).
Deng, L. et al. The destiny of microplastics in one typical petrochemical wastewater treatment plant. Sci. Total Environ. 896 , 165274 (2023).
Talvitie, J. et al. Do wastewater treatment plants act as a potential point source of microplastics? Preliminary study in the coastal Gulf of Finland, Baltic Sea. Water Sci. Technol. 72 , 1495–1504 (2015).
Larissa VuoriMarkku Ollikainen How to remove microplastics in wastewater? A cost-effectiveness analysis. Ecol. Econ. 192 , 107246 (2022).
Koelmans, A. A. et al. Microplastics in freshwaters and drinking water: Critical review and assessment of data quality. Water Res. 155 , 410–422 (2019).
Schymanski, D., Goldbeck, C., Humpf, H. U. & Fürst, P. Analysis of microplastics in water by micro-Raman spectroscopy: Release of plastic particles from different packaging into mineral water. Water Res. 129 , 154–162 (2018).
Senathirajah, K. et al. Estimation of the mass of microplastics ingested – A pivotal first step towards human health risk assessment. J. Hazard. Mater. 404 , 124004 (2021).
Lee, J. H. et al. Detection of microplastic traces in four different types of municipal wastewater treatment plants through FT-IR and TED-GC-MS. Environ. Pollut. 333 , 122017 (2023).
Issac, M. N. & Kandasubramanian, B. Effect of microplastics in water and aquatic systems. Environ. Sci. Pollut. Res. 2021 28 , 19544–19562 (2021).
Reeves, A. et al. Potential transmission of SARS-CoV-2 through microplastics in sewage: A wastewater-based epidemiological review ☆ . https://doi.org/10.1016/j.envpol.2023.122171 (2023).
Kruglova, A. et al. The dangerous transporters: A study of microplastic-associated bacteria passing through municipal wastewater treatment. https://doi.org/10.1016/j.envpol.2022.120316 (2023).
Lai, K. P. et al. Microplastics act as a carrier for wastewater-borne pathogenic bacteria in sewage. https://doi.org/10.1016/j.chemosphere.2022.134692 (2022).
Manoli, K. et al. Investigation of the effect of microplastics on the UV inactivation of antibiotic-resistant bacteria in water. Water Res. 222 , 43–1354 (2022).
Wang, C., et al. Polystyrene microplastics significantly facilitate influenza A virus infection of host cells. https://doi.org/10.1016/j.jhazmat.2022.130617 (2022).
Zhong, H. et al. The hidden risk of microplastic-associated pathogens in aquatic environments. Ecol. Environ. Health 2 , 142–151 (2023).
Nasir, M. S. et al. Innovative technologies for removal of micro plastic: A review of recent advances. Heliyon 10 , e25883 (2024).
Amri, A., Yavari, Z., Reza Nikoo, M. & Karimi, M. Microplastics removal efficiency and risk analysis of wastewater treatment plants in Oman. Chemosphere 359 , 142206 (2024).
Ibrahim, Y. et al. Detection and removal of waterborne enteric viruses from wastewater: A comprehensive review. J. Environ. Chem. Eng. 9 , 105613 (2021).
Al-Hazmi, H. E. et al. Recent advances in aqueous virus removal technologies. Chemosphere 305 , 135441 (2022).
Bhatt, A., Arora, P. & Prajapati, S. K. Occurrence, fates and potential treatment approaches for removal of viruses from wastewater: A review with emphasis on SARS-CoV-2. J. Environ. Chem. Eng. 8 , 104429 (2020) .
Pérez-Cataluña, A. et al. Comparing analytical methods to detect SARS-CoV-2 in wastewater. Sci. Total Environ. 758 , 143870 (2021).
Girón-Guzmán, I. et al. Evaluation of two different concentration methods for surveillance of human viruses in sewage and their effects on SARS-CoV-2 sequencing. Sci. Total Environ. 862 , 160914 (2023).
Puente, H., Randazzo, W., Falcó, I., Carvajal, A. & Sánchez, G. Rapid selective detection of potentially infectious porcine epidemic diarrhea coronavirus exposed to heat treatments using viability RT-qPCR. Front. Microbiol. 11 , 1911 (2020).
Stachler, E. et al. Quantitative CrAssphage PCR Assays for Human Fecal Pollution Measurement. Environ. Sci. Technol. 51 , 9146–9154 (2017).
CDC. CDC 2019-novel coronavirus (2019-nCoV) real-time RT-PCR diagnostic panel. https://www.Fda.Gov/Media/134922/Download . Accessed October 2020.
Sanghavi, S. K., Bullotta, A., Husain, S. & Rinaldo, C. R. Clinical evaluation of multiplex real-time PCR panels for rapid detection of respiratory viral infections. J. Med. Virol. 84 , 162–169 (2012).
Haramoto, E. et al. A review on recent progress in the detection methods and prevalence of human enteric viruses in water. Water Res 135 , 168–186 (2018).
Girón-Guzmán, I. et al. Urban wastewater-based epidemiology for multi-viral pathogen surveillance in the Valencian region, Spain. Water Res 255 , 121463 (2024).
Muurinen, J., et al. Influence of manure application on the environmental resistome under finnish agricultural practice with restricted antibiotic use. https://doi.org/10.1021/acs.est.7b00551 (2017).
Muziasari, W. I., et al. Aquaculture changes the profile of antibiotic resistance and mobile genetic element associated genes in Baltic Sea sediments. FEMS Microbiol. Ecol. , 92 . https://doi.org/10.1093/FEMSEC/FIW052 (2016).
Muziasari, W.I., et al. The resistome of farmed fish feces contributes to the enrichment of antibiotic resistance genes in sediments below baltic sea fish farms. Front. Microbiol. , 7 , 229367. https://doi.org/10.3389/FMICB.2016.02137/BIBTEX (2017).
Wang, F. H. et al. High throughput profiling of antibiotic resistance genes in urban park soils with reclaimed water irrigation. Environ. Sci. Technol. 48 , 9079–9085 (2014).
Yin Lai, F., Muziasari, W., Virta, M., Wiberg, K., & Ahrens, L. Profiles of environmental antibiotic resistomes in the urban aquatic recipients of Sweden using high-throughput quantitative PCR analysis ☆ . https://doi.org/10.1016/j.envpol.2021.117651 (2021).
Masura, J., Baker, J. E., 1959-, Foster, G. D. (Gregory D., Arthur, C., & Herring, C). Laboratory methods for the analysis of microplastics in the marine environment: recommendations for quantifying synthetic particles in waters and sediments. https://doi.org/10.25923/4X5W-8Z02 (2015).
Hidalgo-Ruz, V., Gutow, L., Thompson, R. F. & Thiel, M. Microplastics in the marine environment: A review of the methods used for identification and quantification. Environ. Sci. Technol. 46 , 3060–3075 (2012).
Download references
Acknowledgements
This research was supported by project the Lagoon project (PROMETEO/2021/044) and MCEC WATER (PID 2020 116789 RB C 42 AEI/FEDER, UE). IATA-CSIC is a Centre of Excellence Severo Ochoa (CEX2021-001189-S MCIN/AEI / 10.13039/ 501100011033). IF (MS21-006) and SB were supported by a postdoctoral contract grant for the requalification of the Spanish university system from the Ministry of Universities of the Government of Spain, financed by the European Union (NextGeneration EU).IG-G is recipient of a predoctoral contract from the Generalitat Valenciana (ACIF/2021/181), EC-F is recipient of a postdoctoral contract from the MICINN Call 2018 (PRE2018-083753) and AP-C is recipient of the contract Juan de la Cierva – Incorporación (IJC2020-045382-I) which is financed by MCIN/AEI/10.13039/501100011033 and the European Union “NextGenerationEU/PRTR”. The authors thank Andrea López de Mota, Arianna Pérez, Agustín Garrido Fernández, Mercedes Reyes Sanz, José Miguel Pedra Tellols, and Alcira Reyes Rovatti for their technical support.
Author information
These authors jointly supervised this work: Amparo López-Rubio, Gloria Sánchez.
Authors and Affiliations
Institute of Agrochemistry and Food Technology, IATA-CSIC, Paterna, Valencia, Spain
Inés Girón-Guzmán, Santiago Sánchez-Alberola, Enric Cuevas-Ferrando, Irene Falcó, Azahara Díaz-Reolid, Pablo Puchades-Colera, Alba Pérez-Cataluña, José María Coll, Eugenia Núñez, María José Fabra, Amparo López-Rubio & Gloria Sánchez
Interdisciplinary Platform for Sustainable Plastics towards a Circular Economy—Spanish National Research Council (SusPlast), CSIC, Madrid, Spain
Santiago Sánchez-Alberola, Eugenia Núñez, María José Fabra & Amparo López-Rubio
Department of Microbiology and Ecology, University of Valencia, Burjassot, Valencia, Spain
Irene Falcó
Department of Genetics and Microbiology, Faculty of Biosciences, Universitat Autònoma de Barcelona, Cerdanyola del Vallès, Barcelona, Spain
Sandra Ballesteros
You can also search for this author in PubMed Google Scholar
Contributions
All authors have provided substantial contributions to the conception or design of the work or the acquisition, analysis, or interpretation of the data, drafted the work or revised it critically for important intellectual content, have approved of the completed version, and are accountable for all aspects of the work in ensuring that questions related to the accuracy or integrity of any part of the work are appropriately investigated and resolved.
Corresponding author
Correspondence to Enric Cuevas-Ferrando .
Ethics declarations
Competing interests.
The authors declare no competing interests.
Additional information
Publisher’s note Springer Nature remains neutral with regard to jurisdictional claims in published maps and institutional affiliations.
Supplementary information
Supplementary material, rights and permissions.
Open Access This article is licensed under a Creative Commons Attribution 4.0 International License, which permits use, sharing, adaptation, distribution and reproduction in any medium or format, as long as you give appropriate credit to the original author(s) and the source, provide a link to the Creative Commons licence, and indicate if changes were made. The images or other third party material in this article are included in the article’s Creative Commons licence, unless indicated otherwise in a credit line to the material. If material is not included in the article’s Creative Commons licence and your intended use is not permitted by statutory regulation or exceeds the permitted use, you will need to obtain permission directly from the copyright holder. To view a copy of this licence, visit http://creativecommons.org/licenses/by/4.0/ .
Reprints and permissions
About this article
Cite this article.
Girón-Guzmán, I., Sánchez-Alberola, S., Cuevas-Ferrando, E. et al. Longitudinal study on the multifactorial public health risks associated with sewage reclamation. npj Clean Water 7 , 72 (2024). https://doi.org/10.1038/s41545-024-00365-y
Download citation
Received : 20 February 2024
Accepted : 23 July 2024
Published : 02 August 2024
DOI : https://doi.org/10.1038/s41545-024-00365-y
Share this article
Anyone you share the following link with will be able to read this content:
Sorry, a shareable link is not currently available for this article.
Provided by the Springer Nature SharedIt content-sharing initiative
Quick links
- Explore articles by subject
- Guide to authors
- Editorial policies
Sign up for the Nature Briefing: Anthropocene newsletter — what matters in anthropocene research, free to your inbox weekly.

Log in using your username and password
- Search More Search for this keyword Advanced search
- Latest content
- Current issue
- Browse by collection
- BMJ Journals
You are here
- Online First
- Detection of glaucoma progression on longitudinal series of en-face macular optical coherence tomography angiography images with a deep learning model
- Article Text
- Article info
- Citation Tools
- Rapid Responses
- Article metrics

- Vahid Mohammadzadeh 1 , 2 ,
- Youwei Liang 3 ,
- http://orcid.org/0000-0002-9375-4711 Sasan Moghimi 1 ,
- Pengtao Xie 3 ,
- http://orcid.org/0000-0002-8312-6623 Takashi Nishida 1 ,
- Golnoush Mahmoudinezhad 1 ,
- Medi Eslani 1 ,
- http://orcid.org/0000-0001-8566-0371 Evan Walker 1 ,
- Alireza Kamalipour 1 ,
- Eleonora Micheletti 4 ,
- http://orcid.org/0000-0002-9435-746X Jo-Hsuan Wu 1 ,
- Mark Christopher 1 ,
- http://orcid.org/0000-0002-1143-5224 Linda M Zangwill 1 ,
- Tara Javidi 3 ,
- http://orcid.org/0000-0001-9553-3202 Robert N Weinreb 1
- 1 Viterbi Family Department of Ophthalmology , University of California San Diego , La Jolla , California , USA
- 2 Ophthalmology and Vision Science , University of Louisville , Louisville , Kentucky , USA
- 3 Department of Electrical and Computer Engineering , University of California San Diego , La Jolla , California , USA
- 4 Department of Surgical & Clinical, Diagnostic and Pediatric Sciences, Section of Ophthalmology , University of Pavia , Pavia , Lombardia , Italy
- Correspondence to Dr Robert N Weinreb; rweinreb{at}ucsd.edu
Background/aims To design a deep learning (DL) model for the detection of glaucoma progression with a longitudinal series of macular optical coherence tomography angiography (OCTA) images.
Methods 202 eyes of 134 patients with open-angle glaucoma with ≥4 OCTA visits were followed for an average of 3.5 years. Glaucoma progression was defined as having a statistically significant negative 24-2 visual field (VF) mean deviation (MD) rate. The baseline and final macular OCTA images were aligned according to centre of fovea avascular zone automatically, by checking the highest value of correlation between the two images. A customised convolutional neural network (CNN) was designed for classification. A comparison of the CNN to logistic regression model for whole image vessel density (wiVD) loss on detection of glaucoma progression was performed. The performance of the model was defined based on the confusion matrix of the validation dataset and the area under receiver operating characteristics (AUC).
Results The average (95% CI) baseline VF MD was −3.4 (−4.1 to −2.7) dB. 28 (14%) eyes demonstrated glaucoma progression. The AUC (95% CI) of the DL model for the detection of glaucoma progression was 0.81 (0.59 to 0.93). The sensitivity, specificity and accuracy (95% CI) of DL model were 67% (34% to 78%), 83% (42% to 97%) and 80% (52% to 95%), respectively. The AUC (95% CI) for the detection of glaucoma progression based on the logistic regression model was lower than the DL model (0.69 (0.50 to 0.88)).
Conclusion The optimised DL model detected glaucoma progression based on longitudinal macular OCTA images showed good performance. With external validation, it could enhance detection of glaucoma progression.
Trial registration number NCT00221897 .
Data availability statement
Data are available upon reasonable request. The datasets generated and/or analysed during the current study are available from the corresponding author on reasonable request.
https://doi.org/10.1136/bjo-2023-324528
Statistics from Altmetric.com
Request permissions.
If you wish to reuse any or all of this article please use the link below which will take you to the Copyright Clearance Center’s RightsLink service. You will be able to get a quick price and instant permission to reuse the content in many different ways.
VM and YL are joint first authors.
VM and YL contributed equally.
Contributors VM involved in design and conduct of study, data collection, analysis and interpretation of data, writing, critical revision and approval of the manuscript. YL involved in design and conduct of study, analysis and interpretation of data, writing and critical revision. SM involved in design and conduct of study, data collection, analysis and interpretation of data, writing, critical revision and approval of the manuscript. PX involved in design and conduct of study, writing and critical revision. TN involved in data collection, analysis and interpretation of data, writing and critical revision. GM involved in data collection and writing. ME involved in data collection and writing. EW involved in analysis and interpretation of data and writing. AK involved in data collection and writing. EM involved in data collection and writing. J-HW involved in data collection and writing. MC involved in analysis and interpretation of data and writing. LMZ involved in design and conduct of study, data collection, analysis and interpretation of data, writing, critical revision and approval of the manuscript. TJ involved in design and conduct of study, analysis and interpretation of data, writing, critical revision and approval of the manuscript. RNW involved in design and conduct of study, data collection, analysis and interpretation of data, had access to the data, writing, critical revision and approval of the manuscript, controlled the decision to publish and overall guarantor of work.
Funding This work is supported by National Institutes of Health/National Eye Institute Grants R01EY034148, R01EY029058, R01EY011008, R01EY019869, R01EY027510, R01EY026574, P30EY022589; University of California Tobacco Related Disease Research Program (T31IP1511), Research to Prevent Blindness (an unrestricted grant), and participant retention incentive grants in the form of glaucoma medication at no cost from Novartis/Alcon Laboratories, Allergan, Akorn and Pfizer.
Disclaimer The sponsor or funding organisations had no role in the design or conduct of this research.
Competing interests VM: None; YL: None; SM: F: National Eye Institute; PX: None; TN: C: Topcon; GM: None; ME: None; EW: None; AK: F: Fight for Sight; EM: None; J-HW: None:, MC: F: National Eye Institute; LMZ: C: Abbvie Inc., Topcon; F: National Eye Institute, Carl Zeiss Meditec Inc., Heidelberg Engineering GmbH, Optovue Inc., Topcon Medical Systems Inc.; P: Zeiss Meditec, AISight Health (founder); TJ: None; RNW: C: Abbvie, Aerie Pharmaceuticals, Allergan, Amydis, Editas, Equinox, Eyenovia, Iantrek, Implandata, IOPtic, iSTAR Medical, Nicox, Santen, Tenpoint and Topcon; F: National Eye Institute, National Institute of Minority Health and Health Disparities, Heidelberg Engineering, Carl Zeiss Meditec, Konan Medical, Optovue, Zilia, Centervue, and Topcon; P: Toromedes, Carl Zeiss Meditec.
Provenance and peer review Not commissioned; externally peer reviewed.
Supplemental material This content has been supplied by the author(s). It has not been vetted by BMJ Publishing Group Limited (BMJ) and may not have been peer-reviewed. Any opinions or recommendations discussed are solely those of the author(s) and are not endorsed by BMJ. BMJ disclaims all liability and responsibility arising from any reliance placed on the content. Where the content includes any translated material, BMJ does not warrant the accuracy and reliability of the translations (including but not limited to local regulations, clinical guidelines, terminology, drug names and drug dosages), and is not responsible for any error and/or omissions arising from translation and adaptation or otherwise.
Read the full text or download the PDF:
Watch CBS News
Cancer deaths among men predicted to increase 93% by 2050, study finds
By Sara Moniuszko
Edited By Allison Elyse Gualtieri
Updated on: August 12, 2024 / 7:41 PM EDT / CBS News
Cancer cases and deaths among men are expected to surge globally by 2050, according to a new study.
In the study , published Monday in Cancer, a peer-reviewed journal of the American Cancer Society, researchers projected an 84% increase in cancer cases and a 93% increase in cancer deaths among men worldwide between between 2022 and 2050.
The increases were greater among men 65 and older and in countries and territories with a low or medium human development index. The index measures each country's development in health, knowledge and standard of living, according to the study.
Using data from the Global Cancer Observatory, the study analyzed more than 30 different types of cancers across 185 countries and territories worldwide to make demographic projections.
"We know from previous research in 2020 that cancer death rates around the world are about 43% higher in men than in women," said CBS News chief medical correspondent Dr. Jon LaPook. "So this study today looked at, OK, what do we expect over the next 25 years? And it turns out that it translates to about 5 million more deaths per year in men in 2050, compared to today."
This isn't the first study to paint a less-than-optimistic outlook at the future of cancer case numbers.
Earlier this year, the World Health Organization predicted we will see more than 35 million new cancer cases by 2050, a 77% increase from the estimated 20 million cases in 2022. The survey looked at both men and women in 115 countries.
The organization pointed to several factors behind the projected global cancer increase, including:
- Population aging and growth
- Changes to people's exposure to risk factors, with air pollution a key driver of environmental risk factors
- Tobacco and alcohol use
In the latest study, authors also pointed to smoking and alcohol consumption as modifiable risk factors prevalent among men.
"By far, not smoking is the single most important thing" people can do do reduce their risk, LaPook said.
Other factors that may help explain why men face higher rates of cancer compared to women include lower participation in cancer prevention activities and underuse of screening and treatment options, the study authors said.
Improving access to cancer prevention, screening, diagnosis and treatment options, especially for older men, could help improve cancer outcomes, lead author Habtamu Mellie Bizuayehu said in a news release .
Sara Moniuszko is a health and lifestyle reporter at CBSNews.com. Previously, she wrote for USA Today, where she was selected to help launch the newspaper's wellness vertical. She now covers breaking and trending news for CBS News' HealthWatch.
More from CBS News
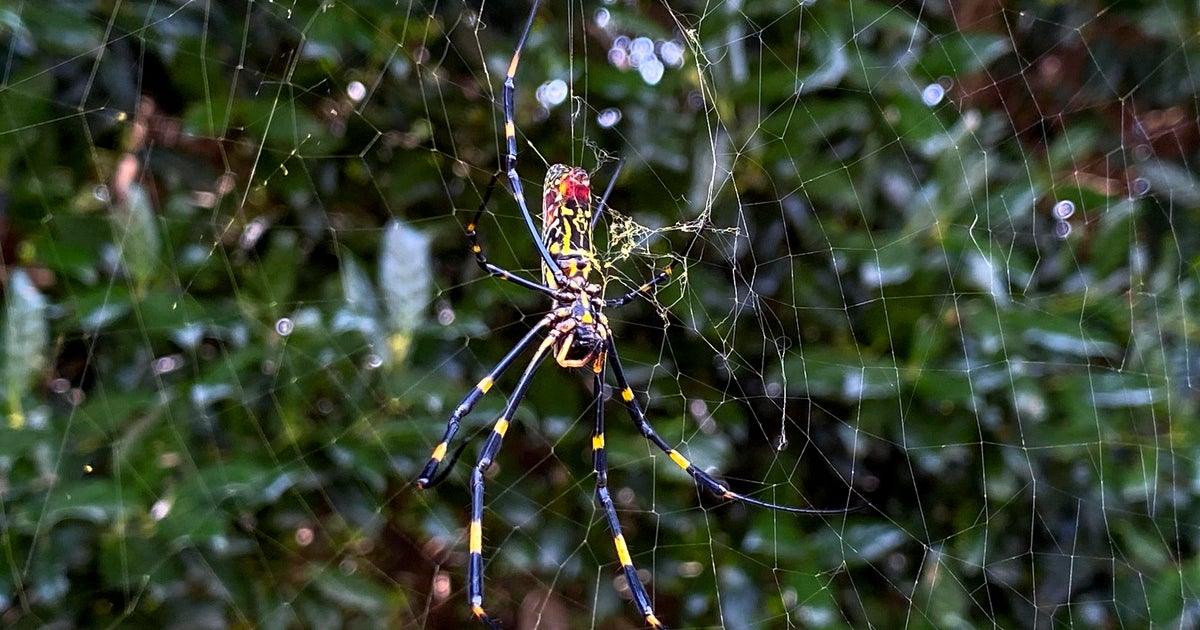
Joro spiders seem to know how to stay chill in big cities, study finds
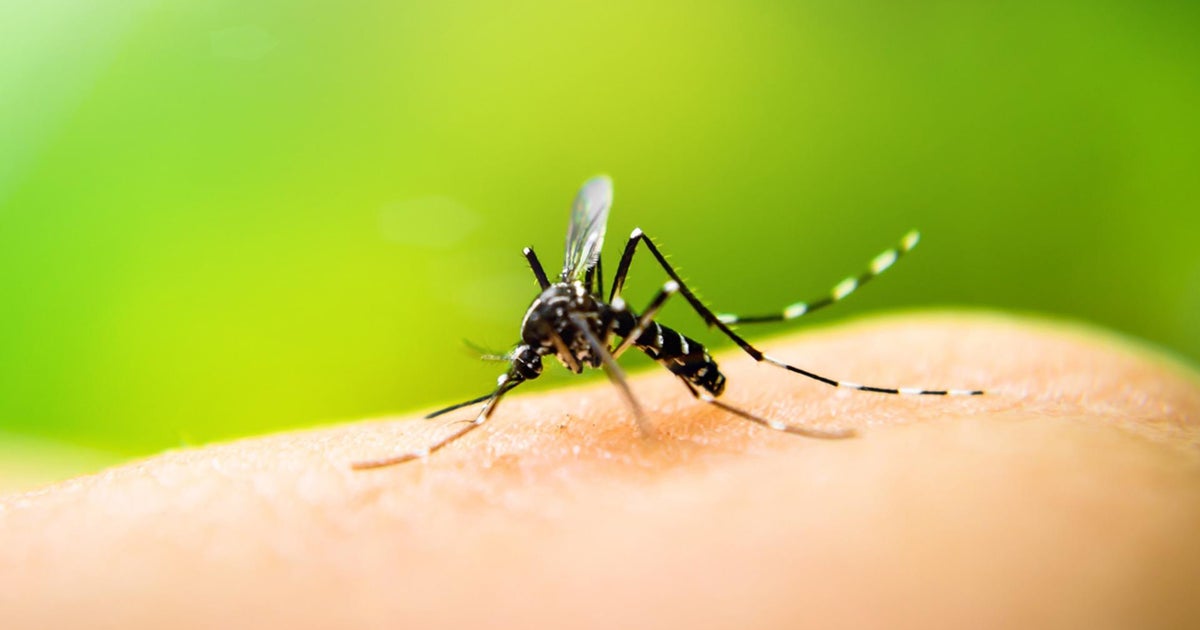
As mosquito season gets longer, how one city is working to prevent diseases
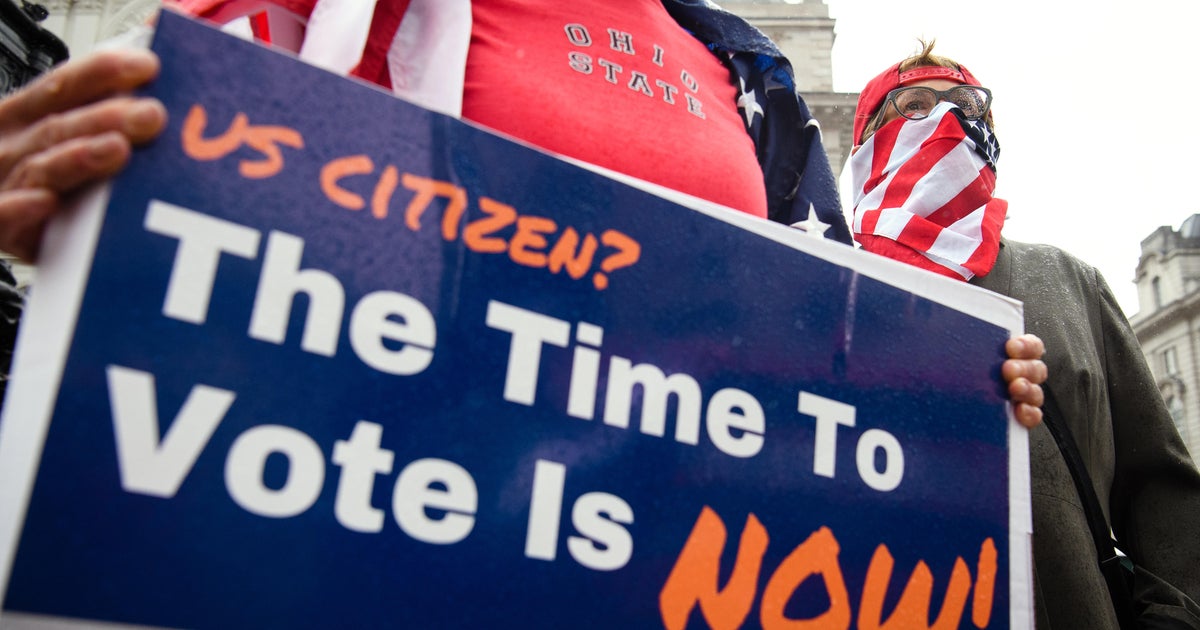
Democrats invest $300,000 to turn out voters living outside the U.S.
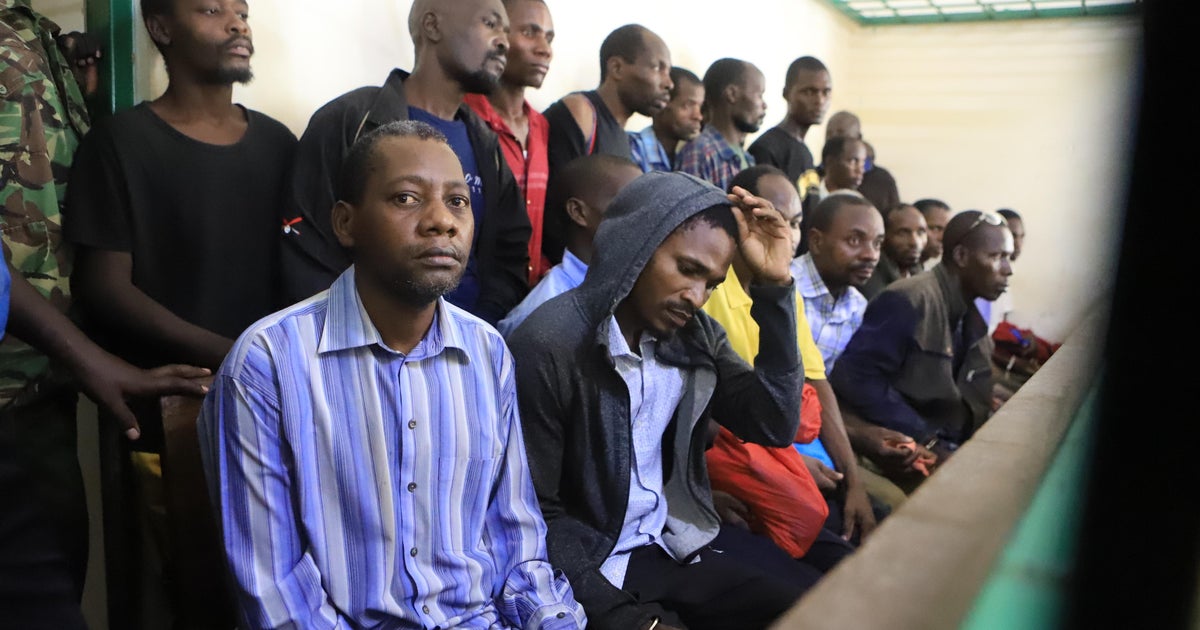
Kenya cult leader on trial for manslaughter over hundreds of deaths

IMAGES
COMMENTS
Longitudinal vs cross-sectional studies The opposite of a longitudinal study is a cross-sectional study. While longitudinal studies repeatedly observe the same participants over a period of time, cross-sectional studies examine different samples (or a "cross-section") of the population at one point in time.
A longitudinal study is a type of observational and correlational study that involves monitoring a population over an extended period of time. It allows researchers to track changes and developments in the subjects over time.
Introduction. Longitudinal studies employ continuous or repeated measures to follow particular individuals over prolonged periods of time—often years or decades. They are generally observational in nature, with quantitative and/or qualitative data being collected on any combination of exposures and outcomes, without any external influenced ...
A longitudinal study (or longitudinal survey, or panel study) is a research design that involves repeated observations of the same variables (e.g., people) over long periods of time (i.e., uses longitudinal data ). It is often a type of observational study, although it can also be structured as longitudinal randomized experiment.
A review and summary of studies on panel conditioning. In Menard S. (Ed.), Handbook of longitudinal research: Designs, measurement and analysis (pp. 123-138). New York: Academic Press. Common Cause & Lokniti—Centre for the Study of Developing Societies (2018).
A longitudinal study is a study that repeatedly measures observations (collects data) over time. It often involves following up with patients for a prolonged period, such as years, and measuring both explanatory and outcome variables at multiple points, usually more than two, of follow-up. Longitudinal studies are most commonly observational ...
Abstract A longitudinal study follows subjects over a certain time period and collects data at specific intervals. Longitudinal studies are powerful study designs. They are particularly useful in medicine as they enable researchers to determine important associations and answer questions regarding prognosis. For example, "when is an athlete able to return to play after a forearm fracture ...
Longitudinal studies, a type of correlational research, are usually observational, in contrast with cross-sectional research. Longitudinal research involves collecting data over an extended time, whereas cross-sectional research involves collecting data at a single point. To test this hypothesis, the researchers recruit participants who are in ...
This study found a greater incidence of dental caries in children breastfed for a period ≥24 months. This longitudinal study demonstrates how these studies repeatedly observe the same individual for changes over time. Example 3: Seven-Year Weight Trajectories and Health Outcomes in the Longitudinal Assessment of Bariatric Surgery Study 24.
A longitudinal study is an experimental design that takes repeated measurements of the same subjects over time. These studies can span years or even decades. Unlike cross-sectional studies, which analyze data at a single point, longitudinal studies track changes and developments, producing a more dynamic assessment.
Both cross-sectional and longitudinal studies are observational in nature, meaning that researchers measure variables of interest without manipulating them. Cross-sectional studies gather information and compare multiple population groups at a single point in time. They offer snapshots of the important current social phenomena.
Longitudinal research refers to any study that collects the same sample of data from the same group of people at different points in time. While time-consuming and potentially costly in terms of resources and effort, a longitudinal study has enormous utility in understanding complex phenomena that might change as time passes.
Keywords: longitudinal studies, observational studies, study design, data analysis Longitudinal observational studies have played a major role in geriatric research and in defining the scope of many health concerns in older adults, their risk factors, and their natural history.
A longitudinal study is a type of correlational research that involves regular observation of the same variables within the same subjects over a long or short period. These studies can last from a few weeks to several decades. Longitudinal studies are common in epidemiology, economics, and medicine. People also use them in other medical and ...
Aiming to encourage longitudinal studies in science education, we clarify conceptual and methodological aspects of longitudinal research. We use the studies that other articles in this issue describe to illustrate these aspects. The illustrations range from attempts to promote long-term change through experimental teaching to investigations that describe change without intending to affect it ...
Learn what a longitudinal study is, what the main advantages and disadvantages are, and whether you should use a longitudinal design.
Longitudinal studies and cross-sectional studies are two different types of research design. In a cross-sectional study you collect data from a population at a specific point in time; in a longitudinal study you repeatedly collect data from the same sample over an extended period of time. Longitudinal study.
10 Famous Examples of Longitudinal Studies. A longitudinal study is a study that observes a subject or subjects over an extended period of time. They may run into several weeks, months, or years. An examples is the Up Series which has been going since 1963. Longitudinal studies are deployed most commonly in psychology and sociology, where the ...
Longitudinal studies. Chapter 7. Longitudinal studies. More chapters in Epidemiology for the uninitiated. In a longitudinal study subjects are followed over time with continuous or repeated monitoring of risk factors or health outcomes, or both. Such investigations vary enormously in their size and complexity.
Longitudinal studies provide a unique vantage point, allowing researchers to observe and analyze how patterns of web streaming engagement evolve and their consequential effects on various aspects ...
What is a Longitudinal Study? A longitudinal study is a correlational research method that helps discover the relationship between variables in a specific target population. It is pretty similar to a cross-sectional study, although in its case, the researcher observes the variables for a longer time, sometimes lasting many years.
Most longitudinal studies are used in either clinical psychology or social-personality observations. They are useful when observing the rapid fluctuations of emotion, thoughts, or behaviors between two specific baseline points. Some researchers use them to study life events, compare generational behaviors, or review developmental trends across individual lifetimes.
The Indian Council of Social Science Research (ICSSR) invites proposals for Longitudinal Studies in Social and Human Sciences. The guidelines entailing details of framework for longitudinal studies, duration of the studies, eligibility criteria, how to apply, budget, remuneration and emoluments of project staff, joining and release of grant, monitoring of research studies and other conditions ...
The Dunedin Multidisciplinary Health and Development Study (also known as the Dunedin Study ... assessment, 94% of all living eligible study members, or 938 people, participated. This is unprecedented for a longitudinal study, with many others worldwide experiencing more than 40% drop-out rates. ... British birth cohort studies; References ...
Longitudinal qualitative research has great promise for better understanding career development and vocational behavior in a context of multiform, changing, and increasingly unpredictable careers. This methodological approach complements longitudinal quantitative research and cross-sectional qualitative research.
This multifaced research sheds light on diverse contaminants present after water reclamation, emphasizing the interconnectedness of human, animal, and environmental health in wastewater management.
Shandong Clinical Research Center of Diabetes and Metabolic Diseases, Jinan, Shandong 250021, China. Shandong Institute of Endocrine and Metabolic Diseases, Jinan, Shandong, 250021, China ... Longitudinal Cohort Study Highlights Cancer-preventive Benefits of Lipid-lowering Drugs.
Background/aims To design a deep learning (DL) model for the detection of glaucoma progression with a longitudinal series of macular optical coherence tomography angiography (OCTA) images. Methods 202 eyes of 134 patients with open-angle glaucoma with ≥4 OCTA visits were followed for an average of 3.5 years. Glaucoma progression was defined as having a statistically significant negative 24-2 ...
Cancer cases and deaths among men are expected to nearly double globally by 2050, according to a new study.
Previous research suggested that the upcoming collision between the two galaxies was inevitable, but a new study claims there's a 50% chance the Milky Way could narrowly avoid Andromeda.