
An official website of the United States government
Here’s how you know
The .gov means it’s official. Federal government websites often end in .gov or .mil. Before sharing sensitive information, make sure you’re on a federal government site.
The site is secure. The https:// ensures that you are connecting to the official website and that any information you provide is encrypted and transmitted securely.

Take action
- Report an antitrust violation
- File adjudicative documents
- Find banned debt collectors
- View competition guidance
- Competition Matters Blog
Slow the Roll-up: Help Shine a Light on Serial Acquisitions
View all Competition Matters Blog posts
We work to advance government policies that protect consumers and promote competition.
View Policy
Search or browse the Legal Library
Find legal resources and guidance to understand your business responsibilities and comply with the law.
Browse legal resources
- Find policy statements
- Submit a public comment
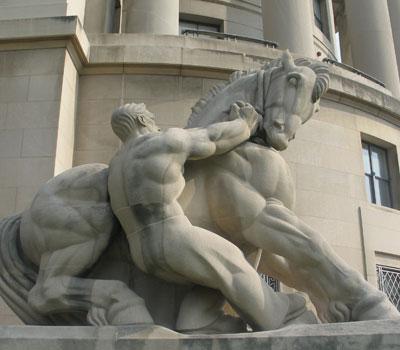
Vision and Priorities
Memo from Chair Lina M. Khan to commission staff and commissioners regarding the vision and priorities for the FTC.
Technology Blog
Avoiding outages and preventing widespread system failures.
View all Technology Blog posts
Advice and Guidance
Learn more about your rights as a consumer and how to spot and avoid scams. Find the resources you need to understand how consumer protection law impacts your business.
- Report fraud
- Report identity theft
- Register for Do Not Call
- Sign up for consumer alerts
Get Business Blog updates
- Get your free credit report
- Find refund cases
- Order bulk publications
- Consumer Advice
- Shopping and Donating
- Credit, Loans, and Debt
- Jobs and Making Money
- Unwanted Calls, Emails, and Texts
- Identity Theft and Online Security
- Business Guidance
- Advertising and Marketing
- Credit and Finance
- Privacy and Security
- By Industry
- For Small Businesses
- Browse Business Guidance Resources
- Business Blog
Servicemembers: Your tool for financial readiness
Visit militaryconsumer.gov
Get consumer protection basics, plain and simple
Visit consumer.gov
Learn how the FTC protects free enterprise and consumers
Visit Competition Counts
Looking for competition guidance?
- Competition Guidance
News and Events
Latest news, ftc staff issue report on multi-level marketing income disclosures.
View News and Events
Upcoming Event
Informal hearing on the proposed amendments to the energy labeling rule.
View more Events
Sign up for the latest news
Follow us on social media

Noncompete Rule: What You Should Know
Visit the Noncompetes feature page for more information, including factsheets featuring stories on how the rule can benefit Americans.
Latest Data Visualization
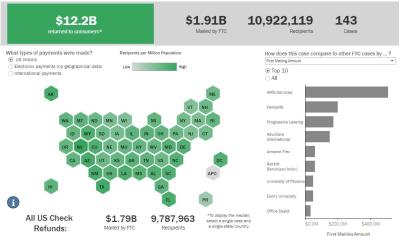
FTC Refunds to Consumers
Explore refund statistics including where refunds were sent and the dollar amounts refunded with this visualization.
About the FTC
Our mission is protecting the public from deceptive or unfair business practices and from unfair methods of competition through law enforcement, advocacy, research, and education.
Learn more about the FTC
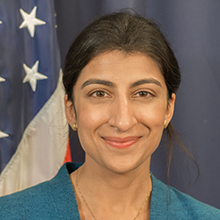
Meet the Chair
Lina M. Khan was sworn in as Chair of the Federal Trade Commission on June 15, 2021.
Chair Lina M. Khan
Looking for legal documents or records? Search the Legal Library instead.
- Cases and Proceedings
- Premerger Notification Program
- Merger Review
- Anticompetitive Practices
- Competition and Consumer Protection Guidance Documents
- Warning Letters
- Consumer Sentinel Network
- Criminal Liaison Unit
- FTC Refund Programs
- Notices of Penalty Offenses
- Advocacy and Research
- Advisory Opinions
- Cooperation Agreements
- Federal Register Notices
- Public Comments
- Policy Statements
- International
- Office of Technology Blog
- Military Consumer
- Consumer.gov
- Bulk Publications
- Data and Visualizations
- Stay Connected
- Commissioners and Staff
- Bureaus and Offices
- Budget and Strategy
- Office of Inspector General
- Careers at the FTC

Social media: a golden goose for scammers
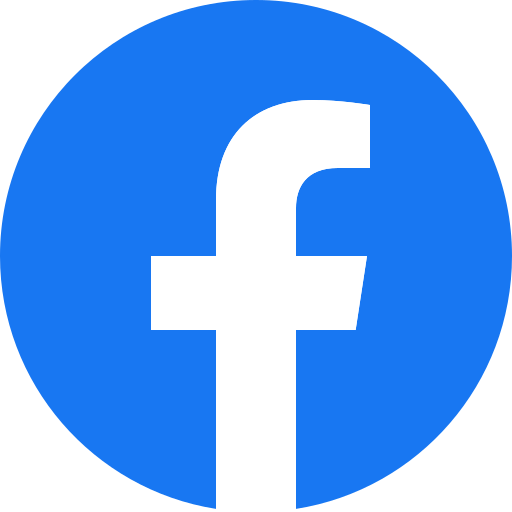
Scammers are hiding in plain sight on social media platforms and reports to the FTC’s Consumer Sentinel Network point to huge profits. One in four people who reported losing money to fraud since 2021 said it started on social media. [1] Reported losses to scams on social media during the same period hit a staggering $2.7 billion, far higher than any other method of contact. And because the vast majority of frauds are not reported, this figure reflects just a small fraction of the public harm. [2]
Social media gives scammers an edge in several ways. They can easily manufacture a fake persona, or hack into your profile, pretend to be you, and con your friends. They can learn to tailor their approach from what you share on social media. And scammers who place ads can even use tools available to advertisers to methodically target you based on personal details, such as your age, interests, or past purchases. All of this costs them next to nothing to reach billions of people from anywhere in the world.
Reports show that scams on social media are a problem for people of all ages, but the numbers are most striking for younger people. In the first six months of 2023, in reports of money lost to fraud by people 20-29, social media was the contact method more than 38% of the time. For people 18-19, that figure was 47%. [3] The numbers decrease with age, consistent with generational differences in social media use. [4]
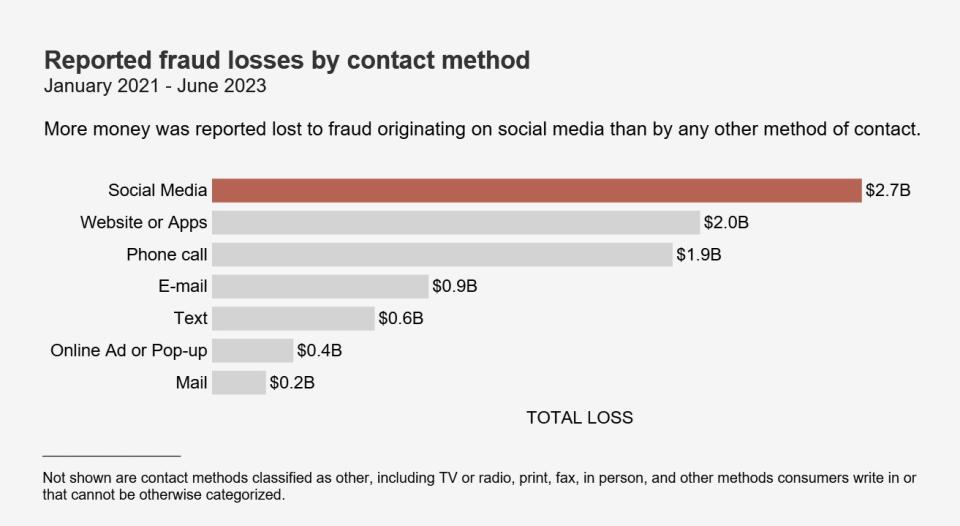
The most frequently reported fraud loss in the first half of 2023 was from people who tried to buy something marketed on social media, coming in at a whopping 44% of all social media fraud loss reports. Most of these reports are about undelivered goods, with no-show clothing and electronics topping the list. [5] According to reports, these scams most often start with an ad on Facebook or Instagram. [6]
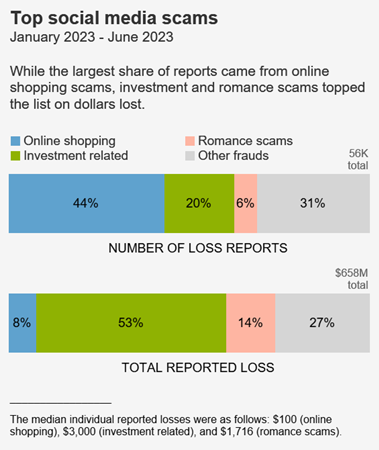
While online shopping scams have the highest number of reports, the largest share of dollar losses are to scams that use social media to promote fake investment opportunities. [7] In the first six months of 2023, more than half the money reported lost to fraud on social media went to investment scammers. To draw people in, these scammers promote their own supposed investment success, often trying to lure people to investment websites and apps that turn out to be bogus. They make promises of huge returns, and even make it look like an “investment” is growing. But if people invest, and reports say it’s usually in cryptocurrency, [8] they end up empty handed.
After investment scams, reports point to romance scams as having the second highest losses on social media. In the first six months of 2023, half of people who said they lost money to an online romance scam said it began on Facebook, Instagram, or Snapchat. [9] These scams often start with a seemingly innocent friend request from a stranger followed by love bombing and the inevitable request for money.
Here are some ways to steer clear of scams on social media:
- Limit who can see your posts and information on social media. All platforms collect information about you from your activities on social media, but visit your privacy settings to set some restrictions.
- If you get a message from a friend about an opportunity or an urgent need for money, call them. Their account may have been hacked—especially if they ask you to pay by cryptocurrency, gift card, or wire transfer. That’s how scammers ask you to pay.
- If someone appears on your social media and rushes you to start a friendship or romance, slow down. Read about romance scams . And never send money to someone you haven’t met in person.
- Before you buy, check out the company . Search online for its name plus “scam” or “complaint.”
[1] This figure excludes reports that did not specify a contact method. Including reports directly to the FTC and reports provided by Sentinel data contributors, 257,945 reports about money lost to fraud originating on social media were filed from January 2021 through June 2023.
[2] See Anderson, K. B., To Whom Do Victims of Mass-Market Consumer Fraud Complain? at 1 (May 2021), available at https://papers.ssrn.com/sol3/papers.cfm?abstract_id=3852323 (study showed only 4.8% of people who experienced mass-market consumer fraud complained to a Better Business Bureau or a government entity).
[3] These figures exclude reports that did not specify a contact method and reports that did not include age information.
[4] See Pew Research Center, Social Media Use in 2021 (April 2021), available at https://www.pewresearch.org/internet/2021/04/07/social-media-use-in-2021/ (study showed people ages 18-29 reported the highest social media use at 84%, followed by ages 30-49 at 81%, ages 50-64 at 73% and 65 and over at 45%). In the first 6 months of 2023, the share of loss reports indicating social media as the contact method by age was as follows: 47% (18-19), 38% (20-29), 32% (30-39), 28% (40-49), 26% (50-59), 21% (60-69), 15% (70-79), 9% (80 and over). Social media was the top contact method ranked by fraud loss reports for all age groups under age 70, while phone call was the top contact method for the 70-79 and 80 and over age groups.
[5] The top undelivered items were identified by hand-coding a random sample of 400 reports that contained a narrative description identifying the items ordered.
[6] In the first 6 months of 2023, people reported undelivered merchandise in 61% of loss reports about online shopping fraud originating on social media. Facebook was identified as the social media platform in 60% of these reports, and Instagram was identified in 24%. This excludes reports that did not identify a platform.
[7] The top platforms identified in these reports were Instagram (30%), Facebook (26%), WhatsApp (13%), and Telegram (9%). Reports that did not indicate a platform are excluded from these calculations.
[8] In the first 6 months of 2023, cryptocurrency was identified as the payment method in 53% of investment-related fraud reports that indicated social media as the method of contact. This excludes reports that did not specify a payment method.
[9] Facebook and Instagram were each identified in 21% of these reports, followed by Snapchat at 8%. This excludes reports that did not specify the platform, website, or app.
File Social media: a golden goose for scammers (318.84 KB)
More from the Data Spotlight
Bitcoin atms: a payment portal for scammers, who’s who in scams: a spring roundup, impersonation scams: not what they used to be, iykyk: the top text scams of 2022.
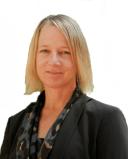
Social Media Use and Fraud
Employment scams and the telegram app..
Posted February 11, 2022 | Reviewed by Ekua Hagan
- What Is a Career
- Take our Procrastination Test
- Find a career counselor near me
- Social media fraud is exploding: Per the FTC, 1 in 4 scams start on social media.
- Younger adults aged 18-39 were more than twice as likely as older adults to report losing money to social media scams in 2021.
- Young workers who are new to the job market can be especially vulnerable to employment scams.
A recent report by the Federal Trade Commission (FTC, January 25, 2022) highlights the shift to social media as the platform of choice for con artists. Per the FTC, 1 in 4 scams start on social media, and it is the most profitable venue for reaching victims than any other format.
These scams may start with an ad, a post, or a message using a number of platforms. Losses initiated on social media are estimated to be in the range of $770 million for 2021 alone. The scams range from romance scams to investment scams with a massive increase in cryptocurrency scams. As a result of this shift, younger adults aged 18-39 were more than twice as likely as older adults to report losing money to these scams in 2021.
Younger adults and employment scams
Younger adults have different vulnerabilities than seniors. For example, new workers who need jobs and have limited professional experience are more likely to fall prey to employment scams.
These scams often start with either a bogus job posting on legitimate job boards (like Indeed or ZipRecruiter) or are initiated by third parties that contact job seekers indicating they had seen their resume on Indeed or a similar platform. In a typical example, the scammer suggests using a messaging tool such as Telegram or WhatsApp and conducts most or all of the business using text messaging.
I was contacted by a recent college graduate who was willing to speak to me about their experience with this type of scam. The individual graduated last May and was still looking for work in the area of a remote IT help desk or similar position. They were contacted by a recruitment manager who indicated they had seen their resume and indicated falsely that they were from a well-known supplement company. The position was a remote IT-type job and the rate was a tad higher than other entry-level jobs, but not out of the range.
The manager immediately indicated that the interview and training would take place on Telegram, and asked the applicant to download the app to proceed. Telegram is an instant messaging service similar to WhatsApp or Facebook messaging. It has been implicated in cryptocurrency scams and is also used by those seeking secrecy as individuals can communicate without exchanging phone numbers.
Besides being on Telegram, the interview questions appeared routine and professional related to work experience and logistics. Once “hired,” they were asked to give a social security number and other personal information. The big red flag, however, was the requirement that they deposit a $1400 check in their bank account to be used to purchase expensive equipment. At this point in time, the individual contacted me and I advised them to contact their banking institution to cancel the check and initiate a fraud investigation. I also recommended initiating a service to monitor credit reports and threats of identity theft.
If allowed to proceed, the scammer may ask the victim to “buy” expensive software with their own funds, and in the meantime, the initial check bounces. Or sometimes, they ask the individual to purchase crypto and send it to other accounts as part of the job and the applicant becomes unwittingly involved in money laundering. Or both—they become both a victim and have their bank accounts used by the scammers for other purposes. Usually, the banking institution will catch the fraudulent transactions and freeze the account or block online banking after a few suspicious transactions.
Law enforcement recommends contacting your bank and credit card companies, contacting credit monitoring companies, and contacting the national consumer credit line in the US (1-888-567-8688) if you suspect you have been the victim of an employment scam.
Scam victims may also need support. Already discouraged from a difficult job search, younger consumers may become less confident and more anxious regarding their prospects. Like any crime victim, they need support and to be assured that these incidents could literally happen to anyone.
https://www.ftc.gov/news-events/blogs/data-spotlight/2022/01/social-med…
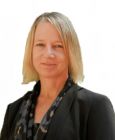
Stacey Wood, Ph.D. is the Molly Mason Jones Professor of Psychology at Scripps College and a national expert on elder fraud issues.
- Find a Therapist
- Find a Treatment Center
- Find a Psychiatrist
- Find a Support Group
- Find Online Therapy
- United States
- Brooklyn, NY
- Chicago, IL
- Houston, TX
- Los Angeles, CA
- New York, NY
- Portland, OR
- San Diego, CA
- San Francisco, CA
- Seattle, WA
- Washington, DC
- Asperger's
- Bipolar Disorder
- Chronic Pain
- Eating Disorders
- Passive Aggression
- Personality
- Goal Setting
- Positive Psychology
- Stopping Smoking
- Low Sexual Desire
- Relationships
- Child Development
- Self Tests NEW
- Therapy Center
- Diagnosis Dictionary
- Types of Therapy
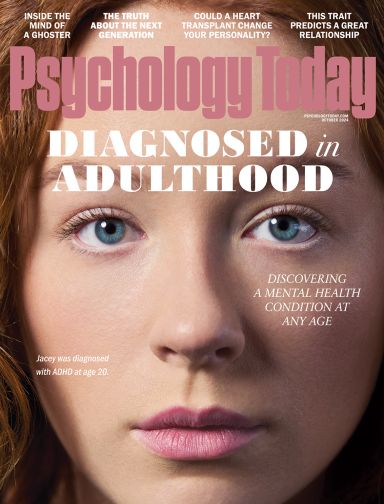
It’s increasingly common for someone to be diagnosed with a condition such as ADHD or autism as an adult. A diagnosis often brings relief, but it can also come with as many questions as answers.
- Emotional Intelligence
- Gaslighting
- Affective Forecasting
- Neuroscience
Worried you have been impacted by the latest data breach? Get protection now .
Worried you have been impacted by the
latest data breach? Get protection now .
The Worst Social Media Scams of 2024 & How To Avoid Them
Social media scams are running rampant. Learn what to look out for and how to avoid the worst social media scams out there right now.
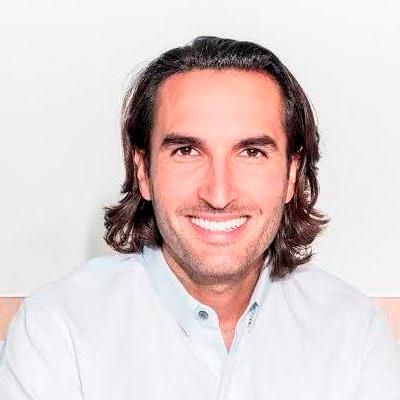
Yaniv Masjedi
Contributing Cybersecurity Writer
Yaniv Masjedi is the CMO at Nextiva, a provider of cloud-based, unified communication services. Previously, he headed the marketing department at Aura. Yaniv studied Political Science and History at UCLA. Follow him on Twitter: @YanivMasjedi.
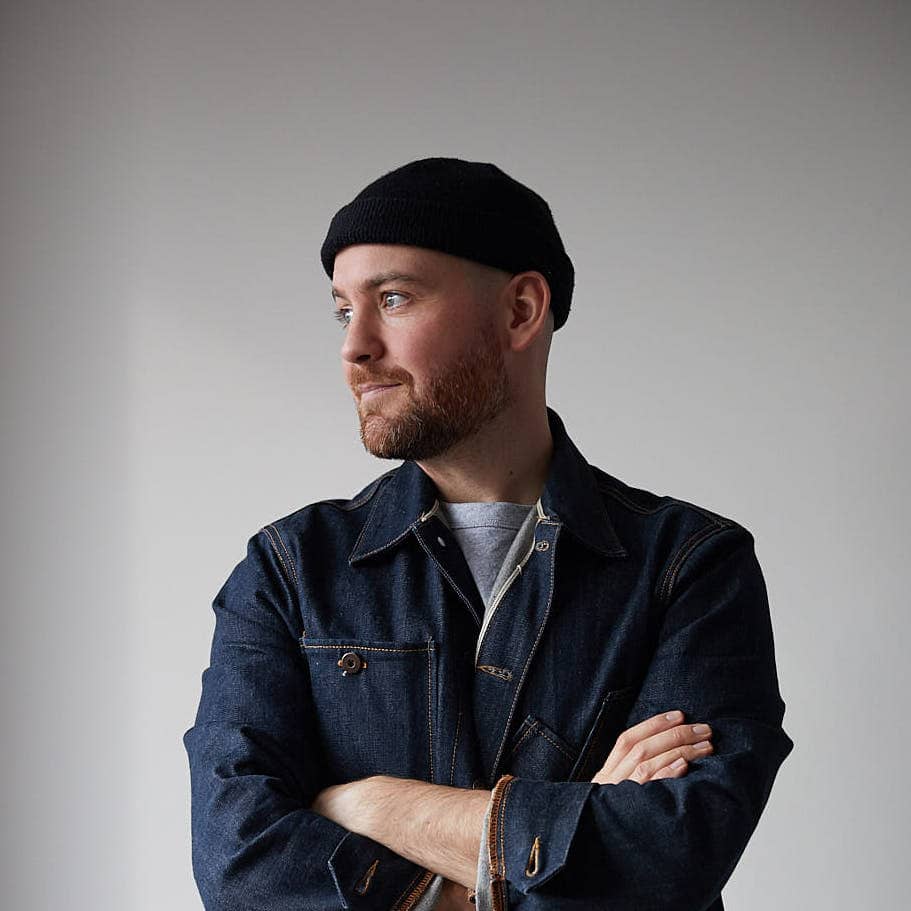
Jory MacKay
Aura Cybersecurity Editor
Jory MacKay is a writer and award-winning editor with over a decade of experience for online and print publications. He has a bachelor's degree in journalism from the University of Victoria and a passion for helping people identify and avoid fraud.

Aura’s digital security app keeps your family safe from scams, fraud, and identity theft.
Do You Know How To Spot a Social Media Scammer?
After Georgina’s husband passed away, she turned to Facebook to feel more connected to her family. Soon after joining, she received a friend request from a man named “Jim” — an attractive stranger who was serving in the military overseas [ * ].
The two hit it off, quickly building an online relationship. Jim had dreams of one day opening a gemstone business after his current service duty ended. But as that date drew closer, he started having serious legal troubles. He needed money to get home — and Georgina was more than happy to help.
When her family and the police finally found out what was going on, it was too late. There was no “Jim” — only a scammer to whom Georgina had sent more than $100,000.
Social media provides prime hunting grounds for scammers.
Last year, one out of every four fraud victims said the scam started with either a social media direct message (DM), ad, or post — with fraud losses hitting $770 million, according to the Federal Trade Commission (FTC) [ * ].
If you or a loved one use social media, you need to be more vigilant than ever to avoid scams.
In this guide, we’ll cover how social media scams work, how to quickly identify a scammer on social media, and 10 of the latest scams to watch out for.
{{show-toc}}
What Are Social Media Scams? How Do People Get Scammed on Social Media?
Social media scams are a type of fraud that is committed on social networking sites. Scammers often create fake profiles, befriend innocent people, and send spam messages or links that lead to malicious websites.
But that’s only several of the ways that scammers can use social media to target you.
Other tactics include:
- Sending you malicious links that infect your devices with malware.
- Running online dating scams and coercing you into sending money or signing up for fake investment platforms. (The latest version of this scam, known as the “ pig butchering scam ” has cost victims over $10 billion .)
- Posting ads to fake stores that steal your personal information or money.
- Using social engineering tactics to trick you into giving scammers access to your social media accounts or sending them money and cryptocurrency.
- Using surveys and quizzes to gather sensitive information that they can use to steal your identity.
- Impersonating brands, celebrities, and people you know — and tricking you into giving them money or personal information.
Scammers can create an endless number of fake profiles and ads, putting billions of social media users at risk. So, how do you spot a scammer before it’s too late?
⛳️ Related: How To Protect your Personal Information on Social Media →
Here’s How To Quickly Identify a Scammer on Social Media
- Their messages include a lot of grammar and spelling errors. Many scammers aren’t native English speakers and may use poor spelling, strange and unnatural language, or awkward formatting. Be especially cautious when someone’s claimed background (where they were born, education, etc.) doesn’t match up with how they write.
- It’s a brand new social media profile with little content or few friends . The average Facebook user has around 200–250 friends [ * ]. Regardless of the platform, if an account has fewer followers than that or is very new, it could be a scammer.
- The profile belongs to someone with whom you thought you were already friends. Scammers create “cloned” profiles to impersonate your friends and contacts.
- You receive a random message with a link in it. Never click on links or engage with unsolicited direct messages (DMs). This is how scammers trick you into going to fake websites or downloading malware onto your device.
- You’re asked to send money online (via gift cards, wire transfers, payment apps, etc.) or invest in cryptocurrency . This is the #1 red flag that you’re dealing with a social media scammer.
- Posts or ads promoting a deal that seems too good to be true. Low prices or hard-to-find items that are readily available are major warning signs of a scam.
- You’re sent to an online store that depicts signs of a scam. Beware of sites that offer good deals but are missing basic information (like shipping times and costs, the company’s address, and direct contact information). When in doubt, follow best practices on how to shop online safely .
- The person insists on taking the conversation off social media and asks you to text them. This allows them to bypass the security measures provided on most social media sites (or continue the scam if their account gets reported and banned).
The 10 Latest Social Media Scams in 2023
- Investment and cryptocurrency scams
- Romance scams
- Social media account takeover fraud
- Authentication code scams
- Social media ads promoting fake online stores
- Impersonator accounts
- “Is this you in this photo/video?”, other link scams
- Social media quizzes
- Lottery, sweepstakes, and giveaway scams
- Job scams on social media
Cybercriminals will stop at nothing to get you to give up your hard-earned money and personal data.
Keep an eye out for these common social media scams to help stay safe from fraudsters:
1. Investment and cryptocurrency scams
Fake cryptocurrency and investment opportunities are among the biggest scams happening on social media right now. It’s estimated that 37% of all social media scam losses last year were due to investment scams — with the majority being cryptocurrency scams [ * ].
The con starts when a scammer reaches out to you, typically via direct social media message. They’ll start off by trying to build a relationship but then quickly share information about a “great investment opportunity” that helped them “make so much money so fast.”

But if you invest, you’ll be sending money or crypto directly to a scammer.
Warning signs of a social media investment scam:
- Promises of high returns with zero risk.
- Professional-looking investment websites or crypto exchanges with little to no information about the company.
- The scammer offers to walk you through your first few trades and claims to have insider knowledge of the market.
Don’t get scammed. Do this instead:
- Conduct a thorough online search and/or contact your state’s Department of Financial Institutions (DFI) to see whether or not the person offering you this opportunity is a real investment banker.
- Don’t share any personal information until you’ve verified whether the company is legitimate or not.
- Do not send money to anyone who has reached out directly over social media.
⛳️ Related: The 11 Latest Facebook Scams You Didn't Know About (Until Now) →
2. Romance scams
Romance scams are common on dating sites, but many scammers also turn to social media to find victims.
In these scams, fraudsters create fake profiles using stolen photos of attractive people to lure in unsuspecting social media users. Once they initiate a relationship, they’re very forward and “love bomb” their victims — quickly telling them that they’re in love and want to meet up.
Eventually, the catfisher will mention financial troubles and ask for help. Too many people have fallen victim to this, with romance scams comprising 24% of all social media scams [ * ].
Warning signs of a romance scam:
- The person wants to quickly move from the social media site to WhatsApp or texting.
- They promise to meet in person but come up with excuses for why they can’t.
- They repeatedly ask for personal information, like your location or pet’s name.
- The scammer professes their love for you early in the conversation.
- They ask for money or gift cards.
- Be conscious of what you post publicly online. Scammers can use your posts, tweets, or updates to craft a personalized approach that makes you think you’ve found the “perfect partner.”
- Be safe and always meet people you meet online in public places.
- Don’t send money to people you haven’t met in person.
⛳️ Related: These 10 WhatsApp Scams Are as Unnerving as They Look →
3. Social media account takeover fraud
Account takeover fraud occurs when hackers gain access to someone’s social media profile. They may trick you into giving up access, use a phishing attack to steal your password, or simply buy your login information off the Dark Web.
Once they gain access, scammers will use these accounts to:
- Post about fake investment opportunities.
- Share links to phishing sites or fake apps .
- Gather personal details from their victim’s friends and family members.
- Gain access to other online accounts (for example, by using “sign in with Facebook”).
Warning signs of an account takeover scam:
- Your friend is randomly sending messages that don’t fully seem like actual things they would say.
- Your friend is randomly posting about investment opportunities or great deals that they just found.
Secure your accounts with strong and unique passwords, and enable two-factor authentication (2FA) whenever possible.
If you receive a message or see a social media post from a friend that doesn’t seem quite right — no matter what platform it’s on — message them on a different platform (or via text/phone call) to double-check that their account didn’t get hacked.
4. Authentication code scams
Two-factor and multi-factor authentication (2FA and MFA) offer additional security for your online accounts by requiring confirmation of a special code along with your password. These codes are usually sent via text or email, making it hard for hackers to steal them.
Scammers on social media pretend to be friends or contacts who need “help” getting their account back and will ask to send a code to your phone or email.
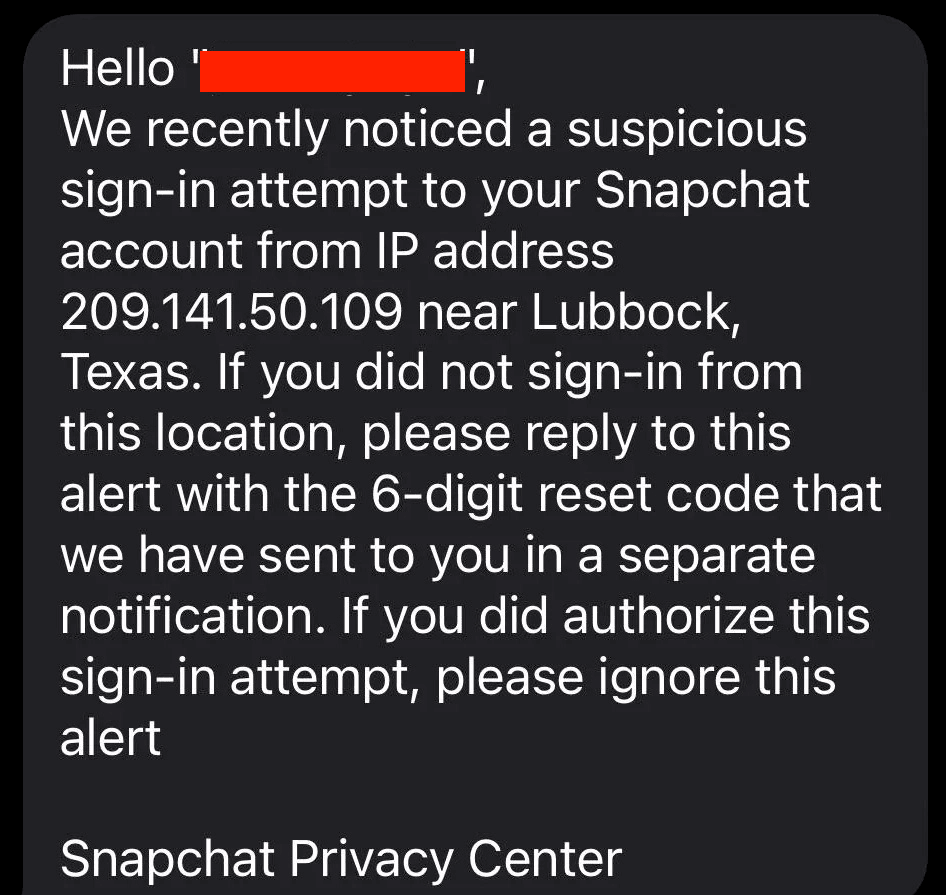
In reality, they’re requesting a 2FA code for your account . If you send the code back to them, they’ll gain access to your online accounts.
Warning signs of an authentication code scam:
- You’ve received a random text with an authentication code for one of your accounts.
- A stranger is texting or messaging you and asking for an authentication code.
- Some scammers claim the code is a way to “tell you’re legitimate” on Facebook Marketplace (or other platforms) as a ruse to get you to send them your code.
- Never give a stranger an authentication code that has been texted or emailed to you. Legitimate companies will never ask for your password or 2FA code.
- Ignore any requests for 2FA codes, and immediately change your passwords for the affected accounts.
⛳️ Related: How To Recover a Hacked Facebook Account →
5. Social media ads promoting fake online stores or counterfeit products
Scammers often use social media ads to promote fake products or stores on social media. The Better Business Bureau (BBB) has received thousands of complaints about misleading Facebook and Instagram ads [ * ].
These online shopping ads try to capture your attention by saying the proceeds are going to charity; or they list items at unbelievable prices.

However, the ads are just fronts to get your money or information, and the scammers do not intend to fulfill the order.
Warning signs of a social media ad scam:
- Poor-quality product images are the center point of the ad.
- Price points are exponentially lower than what other retailers are charging.
- There are spelling and grammatical errors in the ad copy.
If a deal seems too good to be true, it probably is. To be safe, do a Google search of the brand or product to check reviews. Consider searching for “[brand name] + [scam/reviews/legit]” to see if anything comes up.
⛳️ Related: How To Avoid Facebook Marketplace Scams →
6. Impersonator accounts
Scammers create imposter social media accounts using someone else's name, photos, and other identifying information.
Impersonator accounts may request money, send links for phishing scams, or post fake giveaways and prizes.
Scammers have also started impersonating celebrities. Several people have shared their experiences on social media about celebrities supposedly contacting them for financial assistance [ * ] or claiming they’re raising money for charities.
Warning signs of an impersonator scam:
- The account is not verified — especially if it normally would be (i.e., a celebrity or influencer).
- A celebrity or someone you don’t know well is requesting money.
- A “lookalike” social media handle misleadingly seems like it could belong to the real person.
A celebrity or influencer is likely not messaging you to ask for financial help. Always conduct an additional search to see if you can find a verified account for this person, or an account that displays more followers, content, and engagement.
⛳️ Related: How To Properly Set Up Your Social Media Privacy Settings →
7. “Is this you in this photo/video?” and other link scams
This scam is another version of a hacked account scam. You might receive a message from a friend or stranger that says something like, “Is this you in this photo?!” alongside a link.
While reading a message like this can be nerve-racking (or pique your curiosity), don’t click on the link. If you do, it will most likely take you to a fake social media login page designed to steal your password.
Warning signs of a link scam:
- You receive a random message with a strange-looking link or a threatening message.
- When clicking on a link, you’re prompted to log in to a website.
Never click on a suspicious-looking link. Check in with the friend from whom you received the link; but use a different platform or method of communication to either see if it’s legitimate or to let them know that their social media account has been hacked.
If you’re ever asked to log in to an account via a link, check that the page is secure and has a valid security certificate (issued to the site that you think you’re logging in to).
8. Social media quizzes
Scammers use quizzes on social media to steal your personal information and break into your accounts.
These quizzes start with innocent-sounding questions, such as “What car did you pass your driver’s test with?” or “What is your mother’s maiden name?” or “What street did you grow up on?”
But these are common security questions to access your bank account and other financial institutions.
Warning signs of a social media quiz scam:
- A quiz poses unrelated and deeply personal questions.
- You recognize the questions from options you’ve had for security questions.
- The quiz requests your phone number in order for you to view the results.
If a quiz starts asking strange questions, stop there. Don’t answer further questions, and immediately report the account to the social media platform.
9. Lottery, sweepstakes, and giveaway scams
In this type of scam, fraudsters DM you to say you've won a prize. But to receive it, you must first pay or provide financial information [ * ].
Everyone wants to win a big prize. But if you haven't entered any giveaways, you shouldn't receive congratulatory messages in your DMs.
Warning signs of a lottery, sweepstakes, or giveaway scam:
- You’re being asked to pay to receive your prize (i.e., taxes, shipping, processing fees).
- You’re told that paying increases your chances of winning.
- You’re asked to provide financial account information or a phone number to claim your prize.
Do not pay an account that DMs you. No credible lottery or sweepstakes requires you to pay. It’s illegal to request money for sweepstakes .
If you really have entered the lottery or sweepstakes, ensure that the person contacting you about your prize is not asking for money upfront.
⛳️ Related: How To Spot (and Avoid) Publishers Clearing House Scams →
10. Job scams on social media
The number of job scams have rocketed in the last few years as more Americans are working from home or exclusively online.
Fraudsters create fake social media accounts to promote amazing remote job opportunities, promising that you can make tons of money. Scammers have two objectives when running a job scam:
- Get money from you. A scammer will give you the job, but only if you “buy the equipment” first.
- Get information from you. Scammers will send you a job application in hopes that you’ll fill it out and give away private information, such as your Social Security number and home address.
Warning signs of a job scam:
- The job pays extremely well for not much work.
- The supposed employer wants you to pay for your own equipment (legitimate companies should provide you with everything you need).
- You’re sent a check for a large amount and told to deposit it and then send some of the money back to the employer. This is a classic bank scam .
Always research companies to which you’re applying, and make sure they’re legitimate. You can check reviews on sites like Glassdoor, or search for the company name on the Better Business Bureau (BBB) website. In all cases, you should never pay for equipment, training, or supplies upfront for a new job.
Did You Fall for a Social Media Scam? Do This
With 25% of all fraud victims getting scammed on social media, there’s a good chance that you could become a victim. Here’s what to do if you’ve been scammed on social media.
If scammers took over your social media account:
- Request a password reset email from the social media service. Each site and app has a different process for recovering a hacked account. For example, here’s how to recover a hacked Instagram account .
- Once you regain access, force any unfamiliar sessions to log out. For example, check your “login activity” and look for devices or locations that you don’t recognize.
- Then, update the email and phone number associated with your account, and change your passwords.
- Enable 2FA on your account and use an authenticator app such as Authy (instead of text or SMS).
If you sent a social media scammer money or crypto:
- Try to cancel the transaction by contacting the financial institution or crypto exchange that you used.
- Freeze your credit. This stops scammers from using your financial information to open new accounts or take out loans.
- Report the fraud to the social media platform and to the FTC at ReportFraud.ftc.gov.
- If you have any information that could lead to the arrest of the scammer, you should also file a police report with your local law enforcement.
If you clicked on a strange link or gave scammers personal information:
- Report the fraud to the social media platform. Collect as much as information as you can, including screenshots of conversations and the scammer’s profile.
- File an official identity theft report with the FTC at IdentityTheft.gov. This is an essential step if you need to dispute fraudulent transactions or prove that you were the victim of identity theft.
- Report the fraud to the FBI’s Internet Crime Complaint Center ( IC3 ). This will help the authorities track current scams and go after the fraudsters.
- Do a full scan of your device with antivirus software, and follow the steps of what to do if you think you’ve been hacked .
- Consider signing up for Aura’s #1-rated identity theft protection. Try Aura free for 14 days and see if it’s right for you →
How To Stay Safe and Avoid Social Media Scams
With billions of people using social media, it’s impossible to completely avoid scammers. But if you’re vigilant and do your due diligence, you can stay safe and social at the same time.
Whenever you’re using social media, make sure to follow these best practices:
- Never click on pop-up messages or links from unsolicited, private messages.
- Don’t give out personal information unless you know the website you’re on is legitimate and secure.
- Adjust your social media privacy settings to ensure that your posts are not visible to strangers.
- Don’t respond to strangers messaging you on social media.
- Create strong, unique passwords for each social media account.
- Use a password manager to securely store your passwords and warn you if your account has been compromised.
- Activate two-factor authentication (2FA) for your accounts.
- If you suspect a friend or company has been hacked, contact them directly through trusted channels (such as their phone number).
- Never send money to someone you’ve only met on social media.
For added protection, consider signing up for Aura’s all-in-one digital security solution to keep you and your family safe from scams.
With Aura, you get #1-rated identity theft protection, 24/7 credit monitoring, proactive digital security tools — including antivirus software, virtual private network (VPN), password manager, and more — as well as $1 million in insurance coverage for eligible losses due to identity theft.
Stop scammers in their tracks. Try Aura free for 14 days →
Editorial note: Our articles provide educational information for you to increase awareness about digital safety. Aura’s services may not provide the exact features we write about, nor may cover or protect against every type of crime, fraud, or threat discussed in our articles. Please review our Terms during enrollment or setup for more information. Remember that no one can prevent all identity theft or cybercrime.
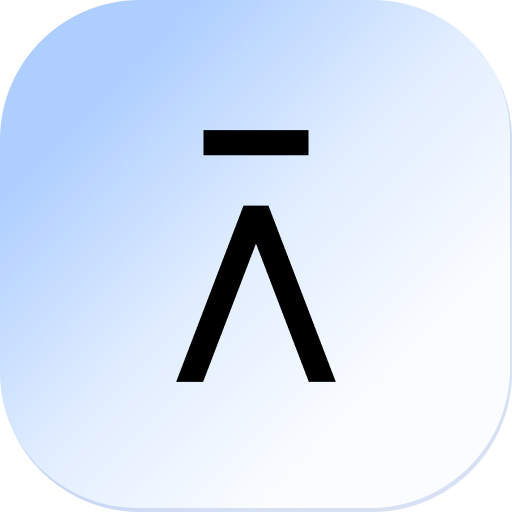
Award-winning identity theft protection with AI-powered digital security tools, 24/7 White Glove support, and more. Try Aura for free .
Related Articles

10 Text Message Scams You Didn't Know About (Until Now)
Scammers are everywhere — even in your text message inbox. Here are the 10 latest text message scams to be aware of (and how to avoid them).

How To Protect Yourself From Account Takeover Fraud (ATO)
Account takeover fraud is when scammers gain access to your online accounts — social media, online banking, etc. Learn how to keep your accounts secure.
Try Aura—14 Days Free
Start your free trial today**
14 days FREE of All-In-One Protection
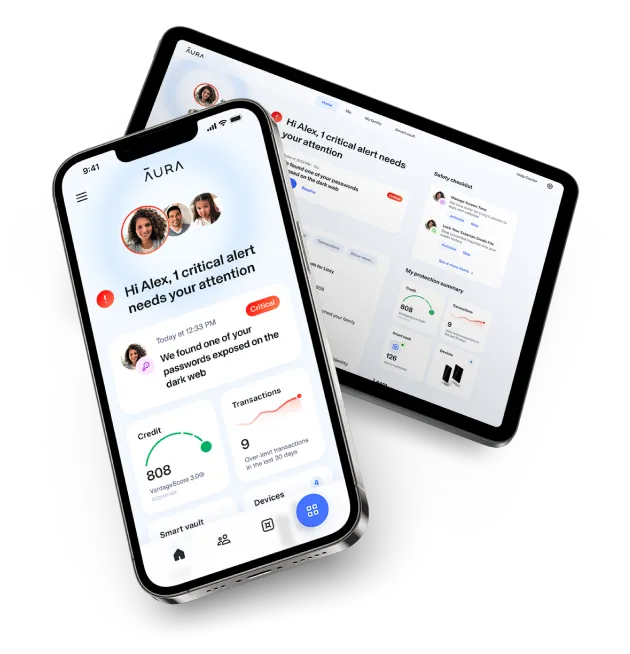
4.7 as of March 2024
The Psychology of Internet Fraud Victimisation: a Systematic Review
- Open access
- Published: 02 July 2019
- Volume 34 , pages 231–245, ( 2019 )
Cite this article
You have full access to this open access article
- Gareth Norris ORCID: orcid.org/0000-0002-7828-5857 1 ,
- Alexandra Brookes 1 &
- David Dowell 2
57k Accesses
57 Citations
96 Altmetric
11 Mentions
Explore all metrics
Existing theories of fraud provide some insight into how criminals target and exploit people in the online environment; whilst reference to psychological explanations is common, the actual use of established behavioural theories and/or methods in these studies is often limited. In particular, there is less understanding of why certain people/demographics are likely to respond to fraudulent communications. This systematic review will provide a timely synthesis of the leading psychologically based literature to establish the key theories and empirical research that promise to impact on anti-fraud policies and campaigns. Relevant databases and websites were searched using terms related to psychology and fraud victimisation. A total of 44 papers were extracted and 34 included in the final analysis. The studies range in their scope and methods; overall, three main factors were identified: message ( n = 6), experiential ( n = 7), and dispositional ( n = 21), although there was some overlap between these (for example, mapping message factors onto the dispositional traits of the victim). Despite a growing body of research, the total number of studies able to identify specific psychological processes associated with increased susceptibility to online fraud victimisation was limited. Messages are targeted to appeal to specific psychological vulnerabilities, the most successful linking message with human factors, for example, time-limited communications designed to enact peripheral rather than central information processing. Suggestions for future research and practical interventions are discussed.
Similar content being viewed by others
Understanding Internet Fraud: Denial of Risk Theory Perspective
Meeting the Challenges of Fraud in a Digital World
The Online Behaviour and Victimization Study: The Development of an Experimental Research Instrument for Measuring and Explaining Online Behaviour and Cybercrime Victimization
Explore related subjects.
- Artificial Intelligence
Avoid common mistakes on your manuscript.
Introduction
The FBI’s Internet Crime Complaint Center (IC3) recently reported figures that show Internet-enabled theft, fraud, and exploitation being responsible for $2.7 billion in financial losses in 2018 (FBI 2018 ). The annual Internet Crime Report shows that IC3 received 351,936 complaints last year—nearly 1000 per day—with non-payment/non-delivery scams, extortion, and personal data breaches the most frequently reported. The most financially costly were business email compromise, romance or confidence fraud, and investment scams. Internet-based fraud was the fastest growing crime in the UK in 2015–2016, with 3.25 million victims each year and an annual combined loss of £3.6 billion (Button et al. 2016 ). Estimates indicate 4.7 million incidents of fraud and computer misuse were experienced by adults aged 16 and over in England and Wales for the survey year ending September 2017 (ONS, 2017 ). Button and Cross ( 2017 ; p. 23) provide a summary on the rising role of technology in perpetuating these crimes: ‘[i]ndeed it is estimated globally there are 29 billion spam emails daily and that the email virus rate is 1 in 196 and phishing emails are 1 in 392’. The on-going infiltration and reliance on technology into our daily lives is likely to see this trend increase in the short-to-medium term until we develop suitable strategies to stay secure online.
However, despite current efforts to educate individuals on the way in which criminals operate online, millions of these fraudulent activities—from phishing attempts to ‘lonely hearts’ scams—are responded to each year (NAO 2017 ); inherent human weaknesses for incentive-driven behaviours seemingly make many of these scams too alluring to resist. For example, priming individuals with images of money has been shown to reduce helpfulness towards others and increase isolation in tasks involving new acquaintances (Vohs et al. 2006 ). Similarly, financial decisions elicit different brain structures to similar non-financial rewards (Knutson et al. 2000 ). Anecdotally, we know that fraud-related messages are designed to exploit certain behavioural and demographic ‘weaknesses’, for example, impulsiveness and/or loneliness (Duffield and Grabosky 2001 ). Button et al. ( 2009 ) note that when considering the perpetrators of fraud, ‘[…] there is only limited data available. Even the law enforcement community does not always know the background of the perpetrators.’ (p. 13). Significantly, the existing fraud literature is limited in scope in terms of exploring the ‘how’ and the ‘why’—in precisely what way they influence individual decision-making processes? Thus, this systematic review aims to connect some of these methodological and conceptual links to establish how message, experiential, and dispositional factors may influence an individual’s cognitive processing associated with increased likelihood for Internet fraud victimisation.
Previous Reviews
There are a number of reviews in the wider online/consumer fraud area, although the focus for many is age as a risk factor. Jackson’s ( 2017 ) evaluation is predominantly aimed at methodological and prevalence issues and suggests a lack of knowledge of risk factors in the financial exploitation of older people increases propensity for fraud. More recently, a review by Burnes et al. ( 2017 ) expands upon many of these points to also include susceptibility to web scams. Incorporating the wider issue of consumer fraud, Ross et al. ( 2014 ) attempt to dispel some of the myths regarding age-related victimisation and increased vulnerability. They document six key areas where older people are more likely to be disproportionately exploited by fraudsters, for example, slower cognitive processing and increased trust. However, Ross et al. suggest that age can also act as a protective factor in the sense that older people are less likely to use the Internet for financial transactions. In particular, they caution that vulnerability does not equal prevalence; Ross et al. conclude that psychological research in this area must not overly stereotype older people in the sense that policies designed to reduce victimisation mistakenly create further opportunities for crime.
A recently published report evaluation of fraud typologies and victims by the UK National Fraud Authority (NFA) highlights how victims are selected, approach strategies, and profiles of victims (Button et al. 2016 ). This report identifies a number of research articles which indicate that targeting individual susceptibility to fraud is a key feature of many scams; for example, using time-limited responses to limit the amount of deliberation. Risk taking and low self-control are also identified as additional personality factors linked to characteristics of fraud victims. The report also goes some way to dispel the myth that older people are more probable victims (although they are more likely to experience fraud than theft or robbery). Lower levels of reporting may be more apparent in older victims—whether they knew the fraud had taken place or not—with those who blamed themselves also being less likely to report. Significantly, active social networks encouraged reporting; these may be less extensive in some older populations. Ultimately, Button et al. caution that: ‘[…] what is striking about of [sic] the scams is that the profiles cover almost everybody; hence almost anyone could become the victim of a scam’ (p. 24). Consequently, although we can observe some small variations in demographics of fraud victims (e.g. age, gender, SES), it appears that individual psychological differences are likely to be the key factor in explaining why some people are more likely to arrive at erroneous decisions in responding to fraudulent online messages.
Theoretical and Conceptual Issues
The majority of previous research conducted in this area predominantly focus on the persuasive influence of the scam message employed by the fraudster (see Chang and Chong 2010 ) or the knowledge of scams held by the potential victim (see Harrison et al. 2016a ). The purpose of this systematic review is to extend that focus to incorporate variables related to individual psychological differences, i.e. those which make people more vulnerable to be deceived by fraudulent communications (see Judges et al. 2017 ). Research by Modic and colleagues has highlighted individual differences to scam compliance through the lens of susceptibility to persuasion and wider theoretical links with social influence (see Modic et al. 2018 ; Modic and Lea 2013 ). The development of the Susceptibility to Persuasion (StP) scale has demonstrated good construct validity in relation to self-report scam plausibility across large samples. The second iteration (StP-II; see Modic et al. 2018 ) incorporates 10 subscales measuring individual differences in a range of mechanisms, including sensation seeking, risk preferences, and social influence. However, we are still some way from achieving a robust and testable model of online fraud susceptibility.
Dispositional factors currently assessed in the literature predominantly focus on demographic factors, such as age, gender, income, and education (Purkait et al. 2014 ), in conjunction with individual characteristics, such as low self-control (Holtfreter et al. 2008 ), high levels of perceived loneliness (Buchanan and Whitty 2014 ), and impulsivity (Pattinson et al. 2011 ). The application of Petty and Cacioppo’s ( 1986 ) elaboration likelihood model (ELM) to explain how psychological mechanisms impact deception likelihood is common (see Vishwanath et al. 2011 ), although few have applied this theoretical model to explore how dispositional factors influence an individual’s cognitive processing associated with victimisation. Similarly, there are a limited number of experimental designs or use of large secondary data sets in this field, both of which would provide the vital understanding of ‘how’ these influences occur. Upon reflection, much of the literature exploring dispositional factors and vulnerability to fraud is limited in scope in terms of understanding the psychological mechanisms that lead people to become victims of these scams. Without sufficient grounding in established psychological mechanisms, attempts to prevent or limit victimisation will likely underperform. The aim of this systematic review is to collate and analyse the key research in relation to the psychology of Internet fraud to ascertain the baseline theoretical and research knowledge in this growing area, focusing on established psychological theories and empirically based methodologies.
Methodology
To examine the extent to which psychological theories have been empirically tested to explain Internet fraud victimisation through a systematic review of the literature. The primary focus is upon understanding the literature which relates to how victims respond to fraudulent communications as opposed to the offender. However, as Button, Lewis, and Tapley ( 2009 , p. 15) note: ‘[t]he growing literature upon different types of fraud provides much information on the techniques of fraudsters. These diverse range of tactics used [can] be considered under three sub-headings, victim selection techniques, perpetration strategies and finally detection avoiding strategies’:
Victim selection techniques concern the strategies that fraudsters use to contact their victims, e.g. email or virus.
Perpetration strategies: once the victim has been identified, these are the techniques used by fraudsters to secure money or identity, e.g. legitimate appearance of an email.
Detection avoidance techniques: techniques used by fraudsters that would minimise their risk of getting caught/sentenced, e.g. making reporting unlikely if ask for a small sum of money.
It is the first two of these that is the focus of this review and primarily the aim is to consolidate our understanding of the psychological mechanisms by which perpetrator (message) and victim (respondent) interact.
Search Methods
Multiple investigators (GN and AB) independently screened both titles and abstracts and relevant full-text articles from the following databases: PsychINFO, ProQuest, International Bibliography of the Social Sciences; Applied Social Science Index and Abstracts, Sociological Abstracts; Sage Criminology; Criminal Justice Abstracts, alongside grey literature from Dissertation Abstracts Online and COS Conference Paper Index. Figure 1 shows the flow diagram outlining the search and exclusion process conforming to the Preferred Reporting Items for Systematic Reviews and Meta-Analyses (PRISMA) guidelines (Moher et al. 2009 ). Full technical data for the systematic review is included in Appendix A .
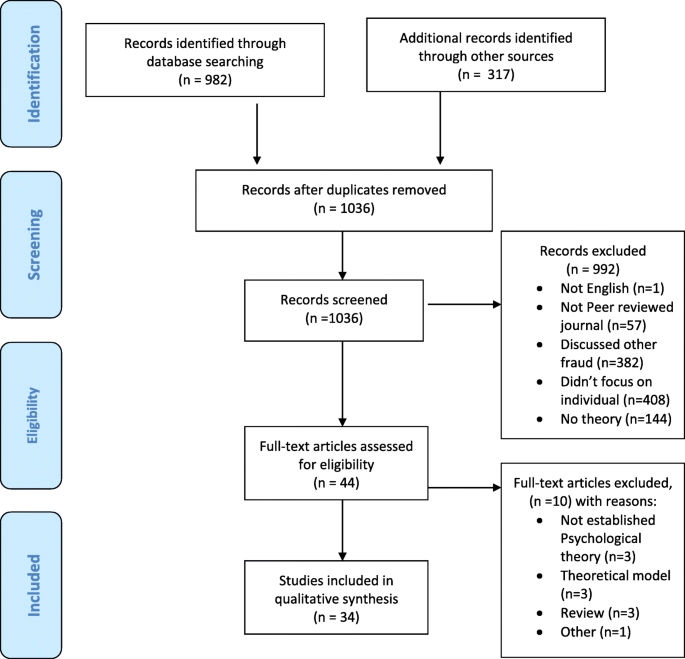
PRISMA flow diagram for identifying psychologically based studies into Internet-based fraud
Inclusion Criteria
The key inclusion criteria were that the paper should be an empirical examination of an established psychological theory relating to online fraud. In order to minimise more general commentary and published statistics articles, we restricted our search criteria to peer-reviewed journal articles, conference presentations, and book chapters in English. Both quantitative and qualitative studies were acceptable, but the latter should employ a recognised analysis technique, for example, interpretive phenomenological analysis (IPA), as opposed to more anecdotal commentaries of cases, scams, etc.
Exclusion Criteria
There were a large number of articles extracted and screened full text before being rejected as not fulfilling the inclusion criteria ( n = 1036). The majority of these articles purported to include psychological theories and/or measures (for example, personality). Additional exclusions included other fraud types (e.g. corporate or academic fraud), those not focusing on the individual or individual factors (e.g. socialisation), and did not include at least one established and testable psychological theory (e.g. loneliness).
Data Collection and Analysis
Main results.
A total of 1299 initial papers were extracted; 39 papers were included in the final search after the exclusion criteria were applied and an additional 5 equivocal items also added ( n = 44) (see Fig. 1 ). From this, a further 10 were excluded by a third author (DD) due to not including an established psychological theory and/or were theoretical models or existing reviews (i.e. not empirical studies). The final number of reviewed articles was 34. The studies range in their scope and methods; overall, three main factors were identified: message, experiential, and dispositional (see Fig. 2 ).
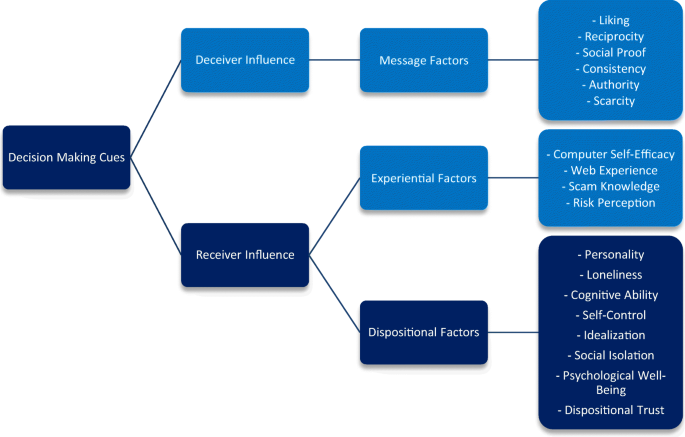
Summary diagram of the variables and processes which influence an individual’s ability to correctly identify fraudulent communications
Meta-analysis
Given the diverse nature of the theoretical background and unrelated outcome measures from each study, a meta-analysis of the findings is not appropriate.
Summary of Studies
Modic and Lea ( 2013 ) regard Internet fraud as a staged process, involving: ‘[…] plausibility, interaction with a fraudster, and losing utility to fraud’ (p. 15); once an offer is deemed plausible, the later stages are therefore more likely to be forthcoming. The review highlighted some broad groupings under which the empirical research in this area has been targeted. The key variables associated with decisions as to whether or not to decide whether information via the internet is plausible can be divided into two key areas: deceiver and receiver influence (see Fig. 1 ). These categories represent both the content of the message and the way in which it interacts with the target. The receiver characteristics can also be further divided into two distinct elements: experiential and dispositional factors. Experiential factors relate to the person’s knowledge and experience of computers and knowledge of fraudulent activity. Dispositional factors include personality, heuristics, and cognitive ability.
Message Factors
The 6 papers classified into this category primarily focused on how the fraudulent message was framed in order to maximise the potential for enticing a victim (Table 1 ). In these articles, only limited mapping onto demographic or individual factors was made. Experimental designs included ‘fake’ phishing emails sent out to university staff and students purporting to be from ‘Information Services’ requesting account verification (Luo et al. 2013 ). Follow-up studies of respondent demographics and personality features in these ‘real-world’ experiments would potentially yield important results for understanding fraud victims’ behaviour, although ethically they may present some limitations.
Fischer et al. ( 2013 ) highlight four key factors that make people more likely to respond to fraudulent communications: (1) high motivation triggers in the size of the reward; (2) trust by focusing on interaction rather than message content, often generated by using ‘official’ notices, logos etc.; (3) social influence, including liking and reciprocation, designed to gain compliance; and (4) the scarcity or urgency of the opportunity. Utilising several waves of quantitative and qualitative studies, Fischer et al. found mixed support for these four elements associated with the message factors and indeed concluded that a fifth factor—personality—may indeed be more indicative of those people likely to predict victimisation. Fischer et al. suggest that this could be in some way linked to ‘self-confidence’ and an increased belief in one’s ability to detect scams. Scam compliance was linked to decision-making errors—exploitation of heuristics (judgement inaccuracies)—and hence limits the exploration of message factors alone as a viable explanation of fraud. It appears that individual differences are more relevant to understanding the way messages are constructed and what processes they are likely to exploit. For example, in what way does a ‘time-limited’ message interact with certain individual’s decision-making processes that make them more likely to respond.
The review highlighted that the content of the message was important to ‘hook’ the target to engaging with the deception. For example, Luo et al. ( 2013 ) demonstrated that messages with high source credibility and quality arguments are particularly effective in ‘spear phishing’ Footnote 1 attacks. Wang et al. ( 2012 ) also found that ‘time-limited’ messages (those which required a quick response) were more likely to be responded to than those which appeared less urgent; it suggests that these ‘visceral triggers’ reduce the cognitive effort expended upon assessing the authenticity of the message. Vishwanath ( 2016 ) extends this perspective to the use of smartphones as a means of reducing cognitive involvement in email filtering, alongside usage variables such as habituation. Responding to fraudulent messages on smartphones was found to be more probable, potentially due to increased cognitive demands and further impacted by the presentation on smaller screens and routine engagement with continued email demands whilst on the move. Certainly, fraudulent responding on smartphones is one potential additional variable to be included in future research.
Experiential Factors
A total of 7 papers were classified into the experiential category, focusing primarily on the experience and expertise of the end-user (Table 2 ). Knowledge of internet scams was one way in which people showed some resilience to victimisation; for example, Wright and Marett ( 2010 ) indicated that people with higher levels of computer self-efficacy, web experience, and security knowledge were less susceptible to phishing attempts. However, Internet use itself was not a protective factor; for some, usage patterns predicted whether they were likely to respond to fraudulent requests, with those people dealing with significantly high email traffic more likely to respond to messages (van Wilsem 2011 ; see also Vishwanath 2015 ). Self-control was identified as a key predictor in whether people were able to withhold responses to fraudulent requests in van Wilsem’s study; what did emerge was a promising underlying pathway that linked low self-control to engaging in more online consumer behaviour generally. Interestingly, Vishwanath ( 2015 ) proposes that email behaviour—particularly habitual use—is linked to low social and emotional control and predictive of increased likelihood to respond to phishing emails.
Harrison et al. ( 2016a ) demonstrate that individual processing styles were also indicative of the likelihood of fraud responding, although this link was moderated significantly by individual factors linked to email knowledge and experience. Similarly, Zielinska et al. ( 2015 ) compared experts and novices in their ability to conceptually link phishing characteristics, discovering the latter used much simpler mental processes in evaluating how a message might be a phishing attempt. Using a novel neurological pathway design, Zielinska and colleagues demonstrate how semantic connections become more sophisticated following experience with how phishing attacks are executed and how to take steps to avoid fraudulent victimisation. The implications for interventions are evident; in addition, the prospects to map these novice reactions to phishing attempts enable a deeper understanding of the way in which people become victims, i.e. the personal factors that limit the way in which people optimise their decision-making strategies.
Hence, a person’s own competency with Internet safety cannot alone explain how they become victims of web-based fraud. Rather, it is an interaction between their ability and usage of the web and general dispositional factors, such as more deliberate and controlled information processing, which are possibly more fruitful avenues of future research in this domain. Potentially, habitual email users are susceptible—feasibly through low social control—to the way in which fraudulent messages are framed, for example, through the use of time-limited rewards, particularly when using mobile devices. It appears, however, that whilst message content and Internet experience have some predictive ability, the key mediating factor is individual dispositional factors that demonstrate the way in which message and experiential factors are processed.
Dispositional Factors
In reviewing the literature in the previous sections, it becomes apparent that the individual is central to the fraud victimisation process. Fischer et al. ( 2013 ) posit the question as to: ‘[w]hy do so many people all over the world, so often, react to completely worthless scam offers?’ (p. 2060). Likewise, despite the investment in firewalls and anti-virus software, the so called semantic attacks exploit inerrant weaknesses in the system—the individual—to divulge sensitive information (Harrison et al. 2016a , b ; p. 265). Workman ( 2008 ) formalises this process of social engineering as: ‘[…] techniques used to manipulate people into performing actions or divulging confidential information’ (p. 662). Subsequently, the key mediating factor between the message(s) and whether experience/expertise in detecting fraud is likely to be practical are individual and personality variables.
One of the most cited papers in this domain is an early examination by Langenderfer and Shimp ( 2001 ) (Table 3 ). Although not focused solely on Internet-based fraud, it nonetheless identifies the ‘visceral influences’ that make individuals vulnerable to scams, through a process that reduces the cognitive deliberation when faced with a message. Notably, Langenderfer and Shrimp utilise Petty and Cacioppo’s ( 1986 ) theory of persuasion: the elaboration likelihood model (ELM). In essence, ELM suggests that individuals who are motivated to respond to the content contained in a fraudulent message are likely to focus and be persuaded by the key messages. On the other hand, those less motivated by the content are more likely to be influenced by peripheral cues. Hence, motivation is likely to be negatively correlated with scam victimisation; the higher the level of motivation, the more likely attention will be expended upon aspects of the message and cues to deception identified. However, although widely cited, Langenderfer and Shimp ( 2001 ) rely heavily on largely anecdotal evidence for their ELM-based theory of scam compliance. Additional studies examining the relevance of ELM have found mixed support for the relevance of this individual factor relating to fraud victimisation (see Whitty 2013 ; Chang 2008 ), although Vishwanath et al. ( 2011 ) do support the ELM approach in conjunction with message and experiential influences.
In the previous section, the link between computer knowledge and self-control are identified by van Wilsem ( 2011 ). Results from Dickman’s ( 1990 ) Impulsivity Inventory (DII)—as a measure of self-control—support the expected link between increased levels of fraud susceptibility. Pattinson et al. ( 2011 ) examine cognitive impulsivity alongside personality and computer familiarity. Personality was less predictive of fraud susceptibility—with the exception of agreeableness—than familiarity with computers generally (see the ‘ Experiential Factors ” section). With regard to impulsivity, however, there was only a small relationship; generally speaking, less impulsive respondents are more able to manage potentially fraudulent messages. Using willingness to take risky investments as a proxy for low self-control, Chen et al. ( 2017 ) identify the role impulsivity has in susceptibility to responding to phishing messages, particularly those promising financial gains. Chen et al. advocate the ‘unpacking’ of the way in which Internet scams exploit impulsive individuals through financial rewards. Reisig and Holtfreter ( 2013 ) add additional support for the notion that lower levels of self-control are correlated to fraud victimisation.
Wider ‘personality’ correlates with fraud susceptibility are often featured in studies, yet many of these fail to incorporate established psychological theories from personality research and/or validated instruments. From those studies that did meet the inclusion criteria, a number attempt more exploratory research into the Big 5 personality characteristics. Hong et al. ( 2013 ) record negative correlations for openness to experience and introversion being more likely to delete legitimate emails. Hence, although these respondents were less prone to being victims of phishing messages, lower levels of trust (also measured) were predictive of general suspicion and potential rejection of genuine communication as a result. In contrast, only agreeableness was identified as a risk factor in Pattinson et al.’s ( 2011 ) research. Alternative personality inventories, for example, the HEXACO Personality Inventory (Judges et al. 2017 ) and the DISC Personality Questionnaire (Chuchuen and Chanvarasuth 2015 ), provide additional evidence for general personality influence in fraud susceptibility. Whilst some small links with potential to increase victimisation and personality factors emerge from these and other studies—for example, conscientiousness (victims have lower scores)—lead Chuchuen and Chanvarasuth ( 2015 ) caution that given the wide-range of phishing and fraudulent message content, no one personality feature is likely to predict susceptibility in isolation: ‘[…] there is relatively little information about the relationship between personality types and phishing techniques. However, there is some interesting literature on the relationship between decision-making that could reflect upon this area’ (p. 332).
The ELM/schema models suggest that central and peripheral decision strategies are key to understanding how cues to fraudulent messages are neglected (Langenderfer and Shimp 2001 ). Additional heuristics and potential judgement errors have also been examined: through a content analysis of phishing emails, Chang and Chong ( 2010 ) identify the representative, availability, and affect heuristics as possible sources of decision errors. Similarly, anchoring—the tendency to use previous information as a base line for later decision processing—compromised the ability to identify fraudulent websites (Iuga et al. 2016 ). Other dispositional factors, include executive functioning (Gavett et al. 2017 ), theory of deception (a decision-making model; Alseadoon et al. 2012 ; Alseadoon et al. 2013 ), and cognitive health and well-being (Lichtenberg et al. 2013 ; James et al. 2014 ). Despite the obvious links to fraud, judgement and decision-making would appear to be a relatively underexplored area of research that potentially can link message and received factors in a meaningful way.
The preamble to this review highlighted the limited use of established psychological theories in explaining Internet fraud susceptibility. From the 34 papers that met our inclusion criteria, there was still a lack of coherence in the selection of appropriate psychological principles with which to explain the increased likelihood of victimisation. In addition, there was a lack of consistency in developing useful ways in which these established psychological constructs added to our understanding of fraud conducted via the Internet. In attempting to identify the methods used by criminals and how they are targeted at specific individuals, there is a need to accurately map aspects of the message to individual differences, including Internet usage and psychological factors. This task is made more complex due to many of the papers reviewed here incorporating two or more of the three identified decision-making factors (message, experiential, and/or dispositional).
Personality theories appear to tell us very little about how people are more likely to respond to fraudulent communication via the Internet. Extravert individuals might be prone to higher levels of risk taking, but there was no clear pathway linking extraversion and fraud susceptibility (Pattinson et al. 2011 ). Time-limited messages might appeal to those with lower levels of social control (Reisig and Holtfreter 2013 ). Similarly, neuroticism increases fraud susceptibility (Cho et al. 2016 ), whereas conscientiousness decreases this tendency (Judges et al. 2017 ). These observations only loosely map onto plausible individual level explanation. In reality, it seems that the targeting of fraudulent emails—whether for phishing attacks, romance scams, or bank frauds—is done largely at random, through a high volume of communications. However, the mass release of phishing scams disguises somewhat the purposely considered message that is designed to appeal to people of specific dispositions.
What is less clear is how these messages—of which receivers negotiate several times per day—are only sometimes successful, even amongst rational and computer savvy individuals. Central versus peripheral processing may provide the most useful way to understand why people fall for scams, particularly messages that emulate official and/or genuine communications. For example, Vishwanath et al. ( 2011 ) produce a convincing account of the way in which message factors are linked to individual processing through the ELM. In addition, domain-specific knowledge also regulates the ELM process, with increased scam knowledge being linked to the attention given to email cues, i.e. a high level of elaboration likelihood. Schwarz ( 1990 ) reviews the evidence on the effect of mood upon visual information processing more generally and concluded that sad moods decreased global processing, whereas those of a happier disposition focused more on local factors. Specifically, when faced with ambiguous stimuli, mood states influenced how quickly people were likely to process information, particularly when the information was relevant to them. Additionally, people in a happy mood are more likely to pay attention to positive messages (for example, fake lottery wins). Current theories (e.g. elaboration likelihood model (ELM); see Petty and Briñol 2015 ) associated with mood influences on information processing suggest that happy individuals structure their response to stimuli in a top-down manner, relying more on heuristics and schemas to aid in understanding (Gasper 2004 ). The contrasting bottom up approach of those in less happy mood states would focus on the stimulus details more closely. Hence, we can see for our understanding of Internet fraud vulnerability that mood could be one key factor that influences how we process potentially fraudulent communications, but as yet has not received significant attention from researchers.
Practical implications concern the ability to identify individuals most at risk of fraud and provide targeted consumer education measures to help prevent victimisation. We know less about the financial situation and other background variables of fraud victims that might increase their risk of victimization. For example, does financial hardship lead people to take bigger chances with regard to false promises of prizes? Similarly, are those with physical and/or mental health problems likely to engage in dialogue with fraudsters through social isolation, anxiety, and other similar issues? Perhaps people with a predisposition for extraversion and/or risk taking may be ‘happier’, less likely to attend to the peripheral aspects of messages (cues to deception), and therefore be at a greater chance of being fraud victims (Gasper, 2004 ). Additional research with a theoretically and practically informed agenda is necessary in this important and growing field. The search terms and inclusion/exclusion criteria employed in this review clearly focused on a relatively narrow band of studies; wider reviews of what we know about the offender and how they target victims specifically can add value to this debate. It would appear that the most used ‘spam/phishing’ email, however, is largely indiscriminately aimed at a wide audience hoping to catch individuals not fully processing the possibility these communications are fraudulent.
Currently, issues arise in protecting specific groups of individuals, as a high proportion of any general awareness campaign maybe targeted on people unlikely to ever fall victim, for example, elderly non-Internet users (Lea et al. 2009 ). This research may help bridge this gap, in that if the more vulnerable groups are identified—or are encouraged to self-identify—prevention material can be specifically targeted at them. For example, the UK National Policing ‘4 P’s’ to tackle fraud and cyber-crime; specifically, elements concerning ‘protection’ and ‘preparation’ of potential fraud victims (City of London Police 2015 ). Similarly, the current ‘Take 5’ campaign developed by The Metropolitan Police with the support of the Financial Fraud Action UK (FFA UK) highlights the importance of not immediately responding to messages. Creating a time-buffer to avoid the peripheral/heuristic interpretation of potentially fraudulent requests could potentially limit the number of responses. Experimental examinations of how people can best control their responses would appear to be a fruitful avenue on the research agenda.
Methodological Limitations
Any systematic review will undoubtedly contain some bias in terms of the search parameters employed; hence, there may be papers which are not included here that others might see as an omission. A number of papers were rejected, most notably through the stipulation that there be an established psychological theory. The question as to what was deemed ‘established’ is somewhat equivocal; for example, research by Van Wyk and Mason ( 2001 ) was not included because the measures for ‘risk taking’ and ‘socialisation’ were not from published scales. Similarly, Button et al. ( 2014 ) acknowledged that ‘[…] previous research studies have identified certain psychological traits […] This was beyond the remit of this research’ (p. 400). Notably the research by Modic and colleagues is absent from the reviewed articles due to the search parameters employed here; the development of the StP-II did not fully match our criteria. Empirical examinations on the predictive validity of the StP-II are forthcoming (see Modic et al. 2018 , p. 16) and if successful will provide a way of understanding and mapping personality characteristics onto fraudulent activity.
There are also some methodological considerations to be accounted for in regard to the studies themselves and in particular their ecological validity in respects to accounting for behaviour in the real world. Role play scenarios, in which participants are asked to access the account of a character and decide how they would deal with a number of emails, may suffer from expectancy/observer effects. Jones et al. ( 2015 ) argue:
[…] that the way in which these types of tasks are constructed may still prompt socially desirable responses. For example, when given the option ‘type the URL into a browser window’, may subsequently alert participants that this is the most sensible option compared to other options such as ‘click on the link’. Parsons et al. ( 2014 ) demonstrated—using a role-play task as a measure of susceptibility—that knowledge of the nature of the study affected behaviour. Participants identified phishing emails more successfully when they had been alerted to look out for them. Such subject expectancy effects might affect the integrity of a study even more than any socially desirable bias (p. 20).
An example of a study using a role-play scenario included in the systematic review is by Pattinson et al. ( 2011 ). Jones et al. ( 2015 ) argue ‘possibly, the assessment of vulnerability with the highest face validity, but clearly the most ethically challenging, would be to stimulate a genuine phishing attack by sending a fake phishing email to participants and recording whether or not they respond’ (p. 22). Two examples of studies that use this method in the systematic review are by Luo et al. ( 2013 ) and Vishwanath ( 2016 ). Hence, although many studies suffer from a potential lack of ecological validity and generalizability, there is a growing corpus of studies which at the very least recognise the limitations inherent in this research domain.
The purpose of this systematic review was to examine the range of psychological factors associated with Internet-based fraud victimisation to identify the way in which Internet scams exploit inherently compromised human decision-making. The majority of the studies reviewed focused on ‘phishing’ and examined a range of factors from personality through to heuristics. Additionally, this included aspects of the message itself, although accurately mapping these two aspects together is potentially less successful. The majority of evidence and subsequent beliefs we have regarding the psychological factors associated with vulnerability to online fraud are at best anecdotal and at worst in danger of creating misleading myths (e.g. older people are ‘easy’ targets). Policies designed to limit the extent and impact of fraud should clearly recognise the universal nature of compliance and that no one demographic is necessarily more or less vulnerable (Button et al. 2016 ). Additionally, whilst we have a steady source of material in terms of fraudulent emails, we know less about which are successful and/or why. Online fraud is relatively unique in that examples of potential criminal activity are openly available. Seemingly we are unable to stop this onslaught, but we can limit their effectiveness by increasing awareness and understanding. Through gaining an insight into how they work and with whom, the potential for law enforcement to create general and targeted crime prevention initiatives is enhanced.
Seemingly, much of the existing literature on the prevalence and prevention of Internet fraud has limited scope in terms of understanding the psychological mechanisms that lead people to become victims of these scams. Without sufficient grounding in established psychological mechanisms, it is likely that attempts to limit victimisation will be potentially flawed and/or underperform. There are a limited number of experimental designs in this field; these provide a vital understanding of how fraudulent attempts made via the Internet are able to exploit innate human frailties in decision-making. General models of risk, on the other hand, largely fail to explain why people withhold responses to very specific requests and what heuristics they use to differentiate real and fraudulent messages. Largely unexplored temporal effects, such as mood and emotion (see Gasper, 2004 ), provide a platform for broader contextual understanding of the fraud process.
‘Spear phishing’ differs from ‘phishing’ in that it is targeted at particular individuals and/or groups; the message is highly relevant and mirrors official communication styles and presentation.
Alseadoon I, Chan T, Foo E, Gonzales Nieto J (2012) Who is more susceptible to phishing emails?: a Saudi Arabian study. ACIS 2012: Location, location, location: Proceedings of the 23rd Australasian Conference on Information Systems 2012 (pp. 1–11). ACIS
Alseadoon IM, Othman MFI, Foo E, Chan T (2013) Typology of phishing email victims based on their behavioural response. AMCIS 2013: Anything, anywhere, anytime: Proceedings of the 19th Americas Conference on Information Systems, 5, 3716–3624
Buchanan T, Whitty MT (2014) The online dating romance scam: causes and consequences of victimhood. Psychol Crime Law 20(3):261–283
Article Google Scholar
Burnes D, Henderson CR, Sheppard C, Zhao R, Pillemer K, Lachs MS (2017) Prevalence of financial fraud and scams among older adults in the United States: a systematic review and meta-analysis. Am J Public Health 107(8):13–21
Button M, Cross C (2017) Technology and fraud: the ‘Fraudogenic’ consequences of the internet revolution. In: McGuire M, Holt T (eds) The Routledge handbook of technology, crime and justice. Routledge, London, pp 1–5
Google Scholar
Button M, Lewis C, Tapley J (2009) Fraud typologies and the victims of fraud: literature review. National Fraud Authority, London
Button M, McNaughton Nicholls C, Kerr J, Owen R (2014) Online frauds: learning from victims why they fall for these scams. Aust N Z J Criminol 47(3):391–408
Button M, Lewis C, Tapley J (2016) Fraud typologies and victims of fraud. National Fraud Authority, London
Chang JJ (2008) An analysis of advance fee fraud on the internet. J Financ Crime 15(1):71–81
Chang JJ, Chong MD (2010) Psychological influences in e-mail fraud. J Financ Crime 17(3):337–350
Chen H, Beaudoin CE, Hong T (2017) Securing online privacy: an empirical test on Internet scam victimization, online privacy concerns, and privacy protection behaviors. Comput Hum Behav 70:291–302
Cho JH, Cam H, Oltramari A (2016) Effect of personality traits on trust and risk to phishing vulnerability: modeling and analysis. Presented at the Proceedings of the IEEE CogSIMA 2016 International Multi-Disciplinary Conference on Cognitive Methods in Situation Awareness and Decision Support, San Diego, CA, (pp. 7–13)
Chuchuen C, Chanvarasuth P (2015) Relationship between phishing techniques and user personality model of Bangkok Internet users. Kasetsart Journal Social Sciences 36(2):322–334
City of London Police, (2015). National Policing Fraud Strategy. Available at: http://democracy.cityoflondon.gov.uk/documents/s50106/Pol_24-15_Appendix_1_Draft%20Police%20Fraud%20Strategy%20v%202.2.pdf . Accessed 24 Jun 2019
Dickman SJ (1990) Functional and dysfunctional impulsivity: personality and cognitive correlates. J Pers Soc Psychol 58:95–102
Article PubMed Google Scholar
Duffield GM, Grabosky PN (2001) The psychology of fraud. Trends and Issues in Crime and Criminal Justice, 199, 1–6
Federal Bureau of Investigation (2018) Internet crime report 2018. Washington: Internet Crime Complaint Center. Available at: https://www.ic3.gov/media/annualreport/2018_IC3Report.pdf (5/6/19)
Fischer P, Lea SE, Evans KM (2013) Why do individuals respond to fraudulent scam communications and lose money? The psychological determinants of scam compliance. J Appl Soc Psychol 43(10):2060–2072
Gasper K (2004) Do you see what I see? Affect and visual information. Cognit Emot 18:405–421
Gavett BE, Zhao R, John SE, Bussell CA, Roberts JR, Yue C (2017) Phishing suspiciousness in older and younger adults: the role of executive functioning. PLoS One 12(2):e0171620
Article PubMed PubMed Central Google Scholar
Harrison B, Svetieva E, Vishwanath A (2016a) Individual processing of phishing emails: how attention and elaboration protect against phishing. Online Inf Rev 40(2):265–281
Harrison B, Vishwanath A, Rao R (2016b) A user-centered approach to phishing susceptibility: the role of a suspicious personality in protecting against phishing. In System Sciences (HICSS), Proceedings of the 49th Hawaii International Conference on System Sciences (pp. 5628–5634). IEEE
Holtfreter K, Reisig MD, Pratt TC (2008) Low self-control, routine activities, and fraud victimization. Criminology 46(1):189–220
Hong KW, Kelley CM, Tembe R, Murphy-Hill E, Mayhorn CB (2013) Keeping up with the Joneses: assessing phishing susceptibility in an email task. In Proceedings of the Human Factors and Ergonomics Society Annual Meeting (Vol. 57, No. 1, pp. 1012–1016). Sage CA: Los Angeles, CA: SAGE Publications, 57, 1012, 1016
Iuga C, Nurse JR, Erola A (2016) Baiting the hook: factors impacting susceptibility to phishing attacks. Hum-Cent Comput Info 6(1):8
Jackson SL (2017) GAO reports and senate committee on aging hearings. In: Dong X (ed) Elder abuse: research, practice, and policy. Springer, New York, pp 595–613
Chapter Google Scholar
James BD, Boyle PA, Bennett DA (2014) Correlates of susceptibility to scams in older adults without dementia. J Elder Abuse Negl 26(2):107–122
Jones H, Towse J, Race N (2015) Susceptibility to email fraud: a review of psychological perspectives, data-collection methods, and ethical considerations. International Journal of Cyber Behavior, Psychology and Learning 5(3):13–29
Judges RA, Gallant SN, Yang L, Lee K (2017) The role of cognition, personality, and trust in fraud victimization in older adults. Front Psychol 8:588
Knutson B, Westdorp A, Kaiser E, Hommer D (2000) FMRI visualization of brain activity during a monetary incentive delay task. NeuroImage 12:20–27
Langenderfer J, Shimp TA (2001) Consumer vulnerability to scams, swindles, and fraud: a new theory of visceral influences on persuasion. Psychol Mark 18(7):763–783
Lea, S.E.G. Fischer, P. and Evans, K.M. (2009). The psychology of scams: provoking and committing errors of judgement, report for the office of fair trading. Available at: www.oft.gov.uk/shared_oft/reports/consumer_protection/oft1070.pdf . Accessed 24 Jun 2019
Lichtenberg PA, Stickney L, Paulson D (2013) Is psychological vulnerability related to the experience of fraud in older adults? Clin Gerontol 36(2):132–146
Luo XR, Zhang W, Burd S, Seazzu A (2013) Investigating phishing victimization with the heuristic-systemic model: a theoretical framework and an exploration. Comput Secur 38(C):28–38
Modic D, Lea S (2013) Scam compliance and the psychology of persuasion. Soc Sci Res Netw. Online: https://doi.org/10.2139/ssrn.2364464
Modic D, Anderson R, Palomäki J (2018) We will make you like our research: the development of a susceptibility-to-persuasion scale. PLoS One 13(3):e0194119
Moher D, Liberati A, Tetzlaff J, Altman DG (2009) Preferred Reporting Items for Systematic Reviews and Meta-Analyses: the PRISMA statement. PLoS Med 6(7):e1000097
National Audit Office (2017) Online fraud. National Audit Office, London
ONS, (2017). Overview of fraud and computer misuse statistics for England and Wales. Office for National Statistics: https://www.ons.gov.uk/peoplepopulationandcommunity/crimeandjustice/articles/overviewoffraudandcomputermisusestatisticsforenglandandwales/2018-01-25 . Accessed 24 Jun 2019
Parsons K, McCormac A, Pattinson M, Butavicius M, Jerram C (2014) A study of information security awareness in Australian government organisations. Inf Manag Comput Secur 22(4):334–345
Pattinson MR, Jerram C, Parsons K, McCormac A, Butavicius MA (2011) Managing phishing emails: a scenario-based experiment. Paper presented at the Proceedings of the Fifth International Symposium on Human Aspects of the Information Security & Assurance HAISA (pp. 74–85)
Petty R, Cacioppo J (1986) The elaboration likelihood model of persuasion. Adv Exp Soc Psychol 19:123–205
Petty RE, Briñol P (2015) Emotion and persuasion: cognitive and meta-cognitive processes impact attitudes. Cognit Emot 29(1):1–26
Purkait S, Kumar De S, Suar D (2014) An empirical investigation of the factors that influence internet user’s ability to correctly identify a phishing website. Inf Manag Comput Secur 22(3):194–234
Reisig MD, Holtfreter K (2013) Shopping fraud victimization among the elderly. J Financ Crime 20(3):324–337
Ross M, Grossmann I, Schryer E (2014) Contrary to psychological and popular opinion, there is no compelling evidence that older adults are disproportionately victimized by consumer fraud. Perspect Psychol Sci 9(4):427–442
Schwarz N (1990) Feelings as information: informational and mo- tivational functions of affective states. In: Higgins ET, Sorrentino R (eds) Handbook of motivation and cognition: foundations of social behavior, vol 2. Guilford, New York, pp 527–561
Van Wyk J, Mason KA (2001) Investigating vulnerability and reporting behavior for consumer fraud victimization. J Contemp Crim Justice 17(4):328–345
Vishwanath A (2015) Examining the distinct antecedents of e-mail habits and its influence on the outcomes of a phishing attack. J Comput-Mediat Commun 20(5):570–584
Vishwanath A (2016) Mobile device affordance: explicating how smartphones influence the outcome of phishing attacks. Comput Hum Behav 63:198–207
Vishwanath A, Herath T, Chen R, Wang J, Rao HR (2011) Why do people get phished? Testing individual differences in phishing vulnerability within an integrated, information processing model. Decis Support Syst 51(3):576–586
Vohs KD, Mead NL, Goode MR (2006) The psychological consequences of money. Science 314(5802):1154–1156
Wang J, Herath T, Chen R, Vishwanath A, Rao HR (2012) Research article phishing susceptibility: an investigation into the processing of a targeted spear phishing email. IEEE Trans Prof Commun 55(4):345–362
Whitty MT (2013) The scammers persuasive techniques model: development of a stage model to explain the online dating romance scam. Br J Criminol 53(4):665–684
Wright RT, Marett K (2010) The influence of experiential and dispositional factors in phishing: an empirical investigation of the deceived. J Manag Inf Syst 27(1):273–303
Zielinska OA, Welk AK, Mayhorn CB, Murphy-Hill E (2015) Exploring expert and novice mental models of phishing. In Proceedings of the Human Factors and Ergonomics Society Annual Meeting (Vol. 59, No. 1, pp. 1132–1136). Sage CA: Los Angeles, CA: SAGE Publications
Download references
Author information
Authors and affiliations.
Aberystwyth University, Aberystwyth, UK
Gareth Norris & Alexandra Brookes
St. Andrews University, St. Andrews, UK
David Dowell
You can also search for this author in PubMed Google Scholar
Corresponding author
Correspondence to Gareth Norris .
Ethics declarations
Conflict of interest.
The authors declare that they have no conflict of interest.
Ethical Approval
Ethical approval was awarded by the Department of Psychology at Aberystwyth University (#6549GGN17).
Informed Consent
N/A (systematic review/analysis of existing research)
Additional information
Publisher’s note.
Springer Nature remains neutral with regard to jurisdictional claims in published maps and institutional affiliations.
Appendix Systematic Review Technical Data
Review title:
i. Psychology, fraud, and risk
Review question
i. How have psychological mechanisms been applied to help understand the individual determinants of consumer susceptibility to online fraud victimisation?
Search terms
Offence type : (fraud; scam; phishing; swindles; advance-fee)
Offence subtype : (consumer; online; internet; cyber; door; telephone; email)
Focus on victim not offender : (victim; victimisation; victimization; victimhood; victimology; vulnerability; susceptibility; risk)
Employing psychology : (persuasion; heuristics; decision-making; elaboration; attention; bias; social-engineering; judgement; influence; personality; mental-models; psychology; cognition)
Search database input fields
TITLE(fraud OR scam OR phishing OR swindles OR “advance fee”) AND ALL(consumer OR online OR internet OR cyber OR door OR telephone OR email) AND ALL(victim OR victimisation OR victimization OR victimhood OR victimology OR vulnerability OR susceptibility OR risk) AND ALL(persuasion OR heuristics OR “decision making” OR elaboration OR attention OR bias OR “social engineering” OR judgement OR influence OR personality OR mental-models OR psychology OR cognition)
Possible databases
Social science databases: PsychINFO; Psych Articles; Web of knowledge core collections; social science premium collection via ProQuest; EBSCOhost; Science Direct; Wiley Online library; Scopus; PubMed; International bibliography of the social sciences; Applied social science index and abstract; Periodicals archive online via ProQuest
Criminology databases: Criminology collection via ProQuest; Sociological abstracts; Sage criminology; Criminal justice abstracts
Grey literature: British library collections; Google scholar; British library direct; Dissertation abstracts online; COS conference paper index; open grey www.opengrey.eu/ ; EthOS; WorldCat
Search results
Search source | Number of items | Field code used |
---|---|---|
Science Direct | 356 | TITLE (only offence type) |
Scopus | 526 | TITLE (only offence type) |
PsycARTICLES | 3 | TITLE (only offence type) |
PubMed | 9 | TITLE (only offence type) |
Web of knowledge core collection | 74 | TITLE (only offence type) and TS (Topic all others) |
Wiley Online library | 235 | TITLE (only offence type) |
Periodicals archive online | 8 | TITLE (only offence type) |
EBSCOhost | 88 | TITLE (only offence type) |
British library direct | 0 | TITLE (only offence type) |
Open grey | 0 | TITLE (only offence type) |
EthOS | 0 | TITLE (only offence type) |
Google scholar | 101,000 | TITLE (only offence type) |
WorldCat | 1,567,061 | TITLE (only offence type) |
British library collections | 20 | TITLE (only offence type) |
Total number of articles collected | 1299 |
Exclusion criteria applied to screening results
Exclusion criteria | Number of papers excluded |
---|---|
EXCLUDE 1. Duplicate article | 263 |
EXCLUDE 2. Not published in English | 1 |
EXCLUDE 3. Not a peer-reviewed journal article or an article found within the specified grey literature (i.e. book chapters or book reviews) | 57 |
EXCLUDE 4. Discussed a fraud type other than online consumer fraud (i.e. mail fraud, telemarketing, corporate, intellectual property, or academic fraud) | 382 |
EXCLUDE 5. Did not focus on the individual victims of consumer fraud (e.g. focused on technology prevention methods or the offenders) | 408 |
EXCLUDE 6. Did not discuss individual factors (including decision-making, cognition, and personality) associated with susceptibility to fraud targeting and/or victimisation | 123 |
EXCLUDE 7. Did not refer to an established scientifically based psychological theory (e.g. the Big 5 personality model) | 31 |
Total number of papers included | 34 |
Rights and permissions
Open Access This article is distributed under the terms of the Creative Commons Attribution 4.0 International License (http://creativecommons.org/licenses/by/4.0/), which permits unrestricted use, distribution, and reproduction in any medium, provided you give appropriate credit to the original author(s) and the source, provide a link to the Creative Commons license, and indicate if changes were made.
Reprints and permissions
About this article
Norris, G., Brookes, A. & Dowell, D. The Psychology of Internet Fraud Victimisation: a Systematic Review. J Police Crim Psych 34 , 231–245 (2019). https://doi.org/10.1007/s11896-019-09334-5
Download citation
Published : 02 July 2019
Issue Date : 15 September 2019
DOI : https://doi.org/10.1007/s11896-019-09334-5
Share this article
Anyone you share the following link with will be able to read this content:
Sorry, a shareable link is not currently available for this article.
Provided by the Springer Nature SharedIt content-sharing initiative
- Internet fraud
- Find a journal
- Publish with us
- Track your research

4 Case Studies in Fraud: Social Media and Identity Theft
Does over-sharing leave you open to the risk of identity theft.
Generally speaking, social media is a pretty nifty tool for keeping in touch. Platforms including Facebook, Twitter, Instagram, and LinkedIn offer us a thousand different ways in which we can remain plugged in at all times.
However, our seemingly endless capacity for sharing, swiping, liking, and retweeting has some negative ramifications, not the least of which is that it opens us up as targets for identity theft.
Identity Theft Over the Years
Identity theft isn’t a new criminal activity; in fact, it’s been around for years. What’s new is the method criminals are using to part people from their sensitive information.
Considering how long identity theft has been a consumer threat, it’s unlikely that we’ll be rid of this inconvenience any time soon.
Living Our Lives Online
The police have been using fake social media accounts in order to conduct surveillance and investigations for years. If the police have gotten so good at it, just imagine how skilled the fraudsters must be who rely on stealing social media users’ identities for a living.
People are often surprised at how simple it is for fraudsters to commit identity theft via social media. However, we seem to forget just how much personal information goes onto social media – our names, location, contact info, and personal details – all of this is more than enough for a skilled fraudster to commit identity theft.
In many cases, a fraudster might not even need any personal information at all.
Case Study #1: The Many Sarah Palins
Former Alaska governor Sarah Palin is no stranger to controversy, nor to impostor Twitter accounts. Back in 2011, Palin’s official Twitter account at the time, AKGovSarahPalin (now @SarahPalinUSA ), found itself increasingly lost in a sea of fake accounts.
In one particularly notable incident, a Palin impersonator tweeted out an open invite to Sarah Palin’s family home for a barbecue. As a result, Palin’s security staff had to be dispatched to her Alaska residence to deter would-be partygoers.
This phenomenon is not limited only to Sarah Palin. Many public figures and politicians, particularly controversial ones like the 2016 presidential candidate Donald Trump, have a host of fake accounts assuming their identity.
Case Study #2: Dr. Jubal Yennie
As demonstrated by the above incident, it doesn’t take much information to impersonate someone via social media. In the case of Dr. Jubal Yennie, all it took was a name and a photo.
In 2013, 18-year-old Ira Trey Quesenberry III, a student of the Sullivan County School District in Sullivan County, Tennessee, created a fake Twitter account using the name and likeness of the district superintendent, Dr. Yennie.
After Quesenberry sent out a series of inappropriate tweets using the account, the real Dr. Yennie contacted the police, who arrested the student for identity theft.
Case Study #3: Facebook Security Scam
While the first two examples were intended as (relatively) harmless pranks, this next instance of social media fraud was specifically designed to separate social media users from their money.
In 2012, a scam involving Facebook developed as an attempt to use social media to steal financial information from users.
Hackers hijacked users’ accounts, impersonating Facebook security. These accounts would then send fake messages to other users, warning them that their account was about to be disabled and instructing the users to click on a link to verify their account. The users would be directed to a false Facebook page that asked them to enter their login info, as well as their credit card information to secure their account.
Case Study #4: Desperate Friends and Family
Another scam circulated on Facebook over the last few years bears some resemblance to more classic scams such as the “Nigerian prince” mail scam, but is designed to be more believable and hit much closer to home.
In this case, a fraudster hacked a user’s Facebook profile, then message one of the user’s friends with something along the lines of:
“Help! I’m traveling outside the country right now, but my bag was stolen, along with all my cash, my phone, and my passport. I’m stranded somewhere in South America. Please, please wire me $500 so I can get home!”
Family members, understandably not wanting to leave their loved ones stranded abroad, have obliged, unwittingly wiring the money to a con artist.
Simple phishing software or malware can swipe users’ account information without their having ever known that they were targeted, thus leaving all of the user’s friends and family vulnerable to such attacks.
How to Defend Against Social Media Fraud
For celebrities, politicians, CEOs, and other well-known individuals, it can be much more difficult to defend against social media impersonators, owing simply to the individual’s notoriety. However, for your everyday user, there are steps that we can take to help prevent this form of fraud.
- Make use of any security settings offered by social media platforms. Examples of these include privacy settings, captcha puzzles, and warning pages informing you that you are being redirected offsite.
- Do not share login info, not even with people you trust. Close friends and family might still accidentally make you vulnerable if they are using your account.
- Be wary of what information you share. Keep your personal info under lock and key, and never give out highly sensitive information like your social security number or driver’s license number.
- Do not reuse passwords. Have a unique password for every account you hold.
- Consider changing inessential info. You don’t have to put your real birthday on Facebook.
- Only accept friend requests from people who seem familiar.
Antivirus software, malware blockers, and firewalls can only do so much. In the end, your discretion is your best line of defense against identity fraud.
You might also enjoy a great Motivational Speaker Video for social media safety tips
Jessica Velasco
Tech Company Pulls Ads from Olympics Over Controversial Opening Ceremony Performance C Spire, a Mississippi-based tech company, withdrew its Olympi…
Hurricane Beryl, Caitlin Clark Triple-Double, French Elections
Nba draft 2024, rivian stock, rapidan dam, subscribe to the skinny.
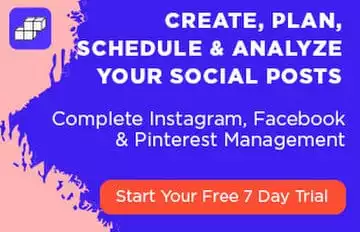
The Viral Sweep: Mapping the Social Media Buzz Around Online Minesweeper Challenges
How surveys can increase b2b lead generation, how to use ai to predict trends and increase sales, uber fined $324 million by the dutch data protection authority, the rise of ai in the workplace, use ai to help you, not replace you, the future of mobile marketing: staying powered in a digital world, the symbiotic relationship between investors, mentors, and startups with fabien dureuil .

An official website of the United States government
Here’s how you know
The .gov means it’s official. Federal government websites often end in .gov or .mil. Before sharing sensitive information, make sure you’re on a federal government site.
The site is secure. The https:// ensures that you are connecting to the official website and that any information you provide is encrypted and transmitted securely.
View all Consumer Alerts
Get Consumer Alerts
Credit, Loans, and Debt
Learn about getting and using credit, borrowing money, and managing debt.
View Credit, Loans, and Debt
Jobs and Making Money
What to know when you're looking for a job or more education, or considering a money-making opportunity or investment.
View Jobs and Making Money
Unwanted Calls, Emails, and Texts
What to do about unwanted calls, emails, and text messages that can be annoying, might be illegal, and are probably scams.
View Unwanted Calls, Emails, and Texts

Identity Theft and Online Security
How to protect your personal information and privacy, stay safe online, and help your kids do the same.
View Identity Theft and Online Security
- Search Show/hide Search menu items Items per page 20 50 100 Filters Fulltext search
Scams that start on social media
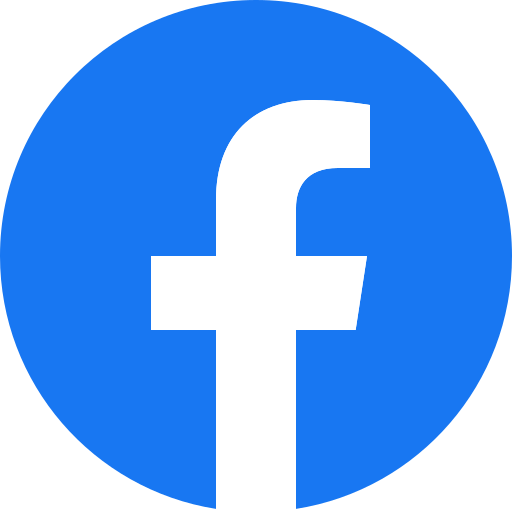
Scammers are hiding out on social media, using ads and offers to market their scams, according to people’s reports to the FTC and a new Data Spotlight . In the first six months of 2020, people reported losing a record high of almost $117 million to scams that started on social media. People sent money to online sellers that didn’t deliver, to romance scammers, and for phony offers of financial help.
The biggest group of reports were about online sellers that didn’t deliver the goods. They were more than one-quarter of all reports about scams that started on social media in the first half of 2020. Next came reports of romance scams: about half of all romance scams reported since 2019 started on social media, usually on Facebook or Instagram. People also told the FTC about social media messages that pretended to offer grants and other financial relief because of the pandemic — but were really trying to get money, personal information or both.
Scammers can hide behind phony profiles on social media. They can take over an account or join a virtual community you trust to encourage you to trust them. But you can make it harder for scammers to target you :
- Review your social media privacy settings and limit what you share publicly.
- Before you buy based on an ad or post, check out the company . Type its name in a search engine with words like or “scam” or “complaint.”
- If someone appears on your social media and rushes you to start a friendship or romance, slow down. Read about romance scams.
- If you get a message from a friend about a grant or financial relief, call them. Did they really send that message? If not, their account may have been hacked. Check it out before you act.
If you spot a scam, report it to the social media site and the FTC at ftc.gov/complaint .
Read Our Privacy Act Statement
It is your choice whether to submit a comment. If you do, you must create a user name, or we will not post your comment. The Federal Trade Commission Act authorizes this information collection for purposes of managing online comments. Comments and user names are part of the Federal Trade Commission’s (FTC) public records system, and user names also are part of the FTC’s computer user records system. We may routinely use these records as described in the FTC’s Privacy Act system notices . For more information on how the FTC handles information that we collect, please read our privacy policy .
Read Our Comment Policy
The purpose of this blog and its comments section is to inform readers about Federal Trade Commission activity, and share information to help them avoid, report, and recover from fraud, scams, and bad business practices. Your thoughts, ideas, and concerns are welcome, and we encourage comments. But keep in mind, this is a moderated blog. We review all comments before they are posted, and we won’t post comments that don’t comply with our commenting policy. We expect commenters to treat each other and the blog writers with respect.
- We won’t post off-topic comments, repeated identical comments, or comments that include sales pitches or promotions.
- We won’t post comments that include vulgar messages, personal attacks by name, or offensive terms that target specific people or groups.
- We won’t post threats, defamatory statements, or suggestions or encouragement of illegal activity.
- We won’t post comments that include personal information, like Social Security numbers, account numbers, home addresses, and email addresses. To file a detailed report about a scam, go to ReportFraud.ftc.gov.
We don't edit comments to remove objectionable content, so please ensure that your comment contains none of the above. The comments posted on this blog become part of the public domain. To protect your privacy and the privacy of other people, please do not include personal information. Opinions in comments that appear in this blog belong to the individuals who expressed them. They do not belong to or represent views of the Federal Trade Commission.
Joann October 21, 2020 I'm embarrassed to have been scammed on Facebook buying shoes from a sham company in China or Taiwan advertising on the site. There are multiple companies of this type advertising there, & I learned, along with hundreds of others, not to buy products from the site. Doesn't Facebook vet their vendors?
Gma4 October 21, 2020 I’ve had people try to log into my social media accounts. But I have really good protection software that alerted me and blocked them.
Red1228 October 21, 2020 How do you help to get back money ppl lost?
In reply to How do you help to get back by Red1228
The FTC enforces consumer protection laws to stop illegal business practices and get refunds to people who lost money.
389361 October 21, 2020 This is a good message. I was scammed last year. Had to close my checking account and open a new one; filed a police report and the same people called me back trying to do the same thing. I do not answer my phone because of this!
Suspicions October 22, 2020 I've noticed increasing friend requests from handsome older- looking men on Facebook. When I look at their public FB page, it becomes clear we have no friends in common and there is very little other info. I do NOT friend them!
Beth October 23, 2020 I was scammed by someone posing as LauraLee Bell reached out to thanking me for being a fan. We struck up a friendship for 6 weeks and we talked about meeting. Through her supposed Management company I got an invoice to pay before we meet for over $18,000. We had to communicate through google hangout, and described her day to day activities & family info, that seem relevant. But - I realized the management company was not a company, just a working email. Had no address where to send payment, a phone number that was v-mail account. Thank god - I did not send this money for something that was likely not going to occur. Also for a meet & Greet I thought 18,795 was pretty hefty. The person is still trying to convince me it is herself - LauraLee Bell, and her management company watches everything she does, and unable to even send me a quick picture of herself. She said I don’t get this money - it’s my management company. Now the person is using emotional strategies - about our close friendship. I am sure other fans or followers are being targeted and hope they don’t for it. I am amazed how a scammer can set up an email address under a celebrities name and have fraudulent Instagram accounts. But this is almost an emotional scam where a relationship is developed and then in order to meet to have to extraordinary fee.
JasminO October 30, 2020 I too was scammed on FB marketplace. Cashapp won't refund amount and on a separate scam, pll would not refund money. Not right as these scammed block you and keep reselling the items.
Bookie October 31, 2020 I have been scammed twice this year which has put me so far behind that I can’t breathe
Pikas March 19, 2021 I've had multiple people try to scam me.... they keep trying to get me to mail phones for them. Today I received 2 new iPhone 12 pro max phones!!!! I caught on pretty quick to what was happening since my grandpa was in the military and I have friends in the military as well.
Confused September 17, 2021 I've just been friend request by a handsome guy called Wood Edward does this name ring a bell
This website requires Javascript. Cifas
Necessary cookies
Necessary cookies enable core functionality such as security, network management, and accessibility. You may disable these by changing your browser settings, but this may affect how the website functions.
User Experience cookies
We'd like to set user experience cookies to help us to improve your experience using this website.
Our use of cookies
We use necessary cookies to make our site work. We'd also like to set optional analytics cookies to help us improve it. We won't set optional cookies unless you enable them. Using this tool will set a cookie on your device to remember your preferences.
For more detailed information about the cookies we use, see our Cookies page

- I'm an Individual and I need help I want to know what information Cifas holds on me I want to apply for protective registration I want help or advice on scams Identity Protection Advice Victim of Impersonation Cifas information for consumers Advice for Young People
- Solutions for Business Data Sharing Membership of the National Fraud Database (External Threat Protect) National Fraud Database Members Insider Threat Protect Solution Insider Threat Database Members Intelligence Sharing Membership Organised Fraud Intelligence Group Cifas Intelligence Service Principles Cifas Identity Check (for orgs) Cifas for the Public Sector
- Fraud and Cyber Academy
- Our Community What is Cifas? Governance Cifas Member Organisations App Victim Check Relationships and Collaboration Annual Reports Reports and Trends Fraud and Risk Focus Blog Public Affairs and Policy Anti-Fraud Lesson Plans Fighting Fraud and Corruption Locally Slipping Through the Net Failure to Prevent - How can Cifas help? Events
- / Our Community
- / Fraud and Risk Focus Blog
How is social media used to commit fraud?
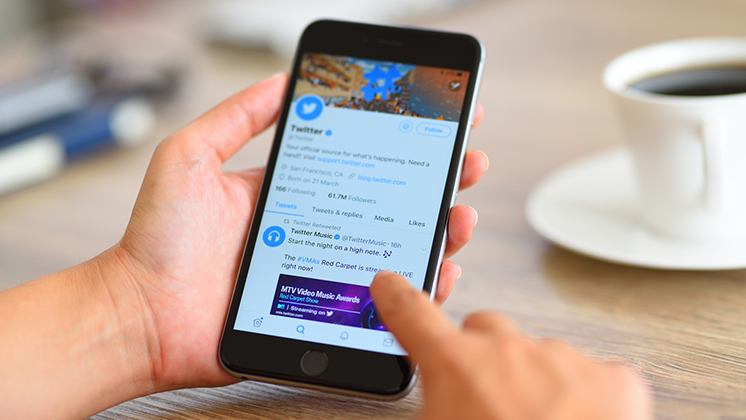
I don’t know what proportion of social media users would query why there’s a piece on social media on a blog about fraud and financial crime, but I suspect it’s high.
The fact is, social media has long been a favourite medium for cybercriminals to do their research and facilitate their crimes, whether financial fraud, identity theft … or both. And they’re becoming increasingly devious and convincing.
Here are some of the ways that financial crime committed via social media, and what can people do to safeguard themselves against it.
Piecing together information
Information that some users post or include in their profiles can represent a goldmine for criminals. The personal details requested by some platforms just when setting up an account – such as date of birth, address and mother’s maiden name – could all be used to steal a user’s identity. This goes hand-in-hand with the ubiquitous practice of using details like family, pets’ or football club names in passwords, because posting photos of the family dog or a day out at Manchester City provides big clues for the jigsaw puzzle.
And, of course, remember that Facebook has been constantly in the news for the way it uses and shares member data.
Research reveals that thankfully, our ‘Generation Z’ young people are being considerably more guarded about the information they reveal online. Many older users, however, despite warnings about oversharing, remain guilty as charged.
Our advice: Don’t overshare … personal should mean personal. If you really need to supply your birthday when registering for a social media account, use someone else’s. The same goes for your mother’s maiden name. Never post pics of your driving licence or passport; they’re an identity thief’s dream. Lock down your privacy settings, but remember there’s no guarantee that your information won’t be shared.
Holiday time
In a similar vein, social media is the modern burglar’s best friend and that includes the status and photos people share when they’re away on holiday, having left the house empty for a week or two. If your home is ransacked while you’re away, not only could insurance companies not settle claims if they find you’ve announced your absence on social media, but the burglar will have a field day with your bank statements and other confidential papers.
Our advice: however tempted you are to share your holiday good times online, think twice before you do.
Phishing via email is still by far the most common initiator of online financial crime, but phishing by social media is getting up there too.
With billions of active users, it represents a rich vein of income for fraudsters. Innocent looking links in Tweets, Facebook posts or on photo or video sharing sites – or in direct messages – can be used to bait users into clicking through to websites which either invite them to enter confidential details or are laden with malware. There’s a multitude of risks, from being duped into revealing logins to having your device infected with any kind of malware – whether it’s ransomware, spyware, a key logger or a bot. All this, just from clicking on a link.
Our advice: don’t click on spurious links in posts, comments or DMs and avoid QR codes for the same reason.
Fake Twitter support accounts
Another commonplace scam involves criminals creating a convincing but fake Twitter customer service account with a handle similar to a bank or other financial services provider’s real one. They wait for customer help request tweets at the bank’s genuine handle, then hijack the conversation by responding with a fraudulent support link sent from a fake support page. The victim is directed to a convincing but fake login page designed to capture their confidential details.
Our advice: if you’re asked for login or other confidential information online, don’t supply it, but call the bank or other organisation concerned on the phone number you know to be correct.
Being befriended by a fraudster
Being deceived out of money to help out someone who says they’re desperate isn’t confined to online dating. Online friendships which begin on social media can grow very fast. Most are genuine, but some fraudsters take advantage of this and begin to ask for money to help them out of a desperate situation, with the amounts steadily growing.
Our advice: never send money or reveal bank account details to anybody you’ve met online, however convincing their story.
It’s scary, but only if the safety rules aren’t followed. At Get Safe Online, we applaud social media for its many positives.
Report fraud to Action Fraud at www.actionfraud.police.uk or on 0300 123 2040
Tim is Content Director at Get Safe Online .
Fraud as a service: holiday accommodation ad scams
With holiday accommodation scams on the rise due to fraud being sold as a service, it's become more important than ever before to make sure your holiday bookings are legitimate.
Tackling first party fraud: busting the myths
What’s meant by ‘first party fraud’ and how can industry reduce the costs to consumers and business? Cifas and WPI Economics released a report covering the different types of first party fraud and the patterns of offenders, then using the data to identify interventions.
- Organisations Advice (40)
- Consumer Advice (35)
- Fraud Education (21)
- Policy (16)
- Identity Fraud (10)

An official website of the United States government
The .gov means it’s official. Federal government websites often end in .gov or .mil. Before sharing sensitive information, make sure you’re on a federal government site.
The site is secure. The https:// ensures that you are connecting to the official website and that any information you provide is encrypted and transmitted securely.
- Publications
- Account settings
Preview improvements coming to the PMC website in October 2024. Learn More or Try it out now .
- Advanced Search
- Journal List
- Springer Nature - PMC COVID-19 Collection

Fake news, disinformation and misinformation in social media: a review
Esma aïmeur.
Department of Computer Science and Operations Research (DIRO), University of Montreal, Montreal, Canada
Sabrine Amri
Gilles brassard, associated data.
All the data and material are available in the papers cited in the references.
Online social networks (OSNs) are rapidly growing and have become a huge source of all kinds of global and local news for millions of users. However, OSNs are a double-edged sword. Although the great advantages they offer such as unlimited easy communication and instant news and information, they can also have many disadvantages and issues. One of their major challenging issues is the spread of fake news. Fake news identification is still a complex unresolved issue. Furthermore, fake news detection on OSNs presents unique characteristics and challenges that make finding a solution anything but trivial. On the other hand, artificial intelligence (AI) approaches are still incapable of overcoming this challenging problem. To make matters worse, AI techniques such as machine learning and deep learning are leveraged to deceive people by creating and disseminating fake content. Consequently, automatic fake news detection remains a huge challenge, primarily because the content is designed in a way to closely resemble the truth, and it is often hard to determine its veracity by AI alone without additional information from third parties. This work aims to provide a comprehensive and systematic review of fake news research as well as a fundamental review of existing approaches used to detect and prevent fake news from spreading via OSNs. We present the research problem and the existing challenges, discuss the state of the art in existing approaches for fake news detection, and point out the future research directions in tackling the challenges.
Introduction
Context and motivation.
Fake news, disinformation and misinformation have become such a scourge that Marcia McNutt, president of the National Academy of Sciences of the United States, is quoted to have said (making an implicit reference to the COVID-19 pandemic) “Misinformation is worse than an epidemic: It spreads at the speed of light throughout the globe and can prove deadly when it reinforces misplaced personal bias against all trustworthy evidence” in a joint statement of the National Academies 1 posted on July 15, 2021. Indeed, although online social networks (OSNs), also called social media, have improved the ease with which real-time information is broadcast; its popularity and its massive use have expanded the spread of fake news by increasing the speed and scope at which it can spread. Fake news may refer to the manipulation of information that can be carried out through the production of false information, or the distortion of true information. However, that does not mean that this problem is only created with social media. A long time ago, there were rumors in the traditional media that Elvis was not dead, 2 that the Earth was flat, 3 that aliens had invaded us, 4 , etc.
Therefore, social media has become nowadays a powerful source for fake news dissemination (Sharma et al. 2019 ; Shu et al. 2017 ). According to Pew Research Center’s analysis of the news use across social media platforms, in 2020, about half of American adults get news on social media at least sometimes, 5 while in 2018, only one-fifth of them say they often get news via social media. 6
Hence, fake news can have a significant impact on society as manipulated and false content is easier to generate and harder to detect (Kumar and Shah 2018 ) and as disinformation actors change their tactics (Kumar and Shah 2018 ; Micallef et al. 2020 ). In 2017, Snow predicted in the MIT Technology Review (Snow 2017 ) that most individuals in mature economies will consume more false than valid information by 2022.
Recent news on the COVID-19 pandemic, which has flooded the web and created panic in many countries, has been reported as fake. 7 For example, holding your breath for ten seconds to one minute is not a self-test for COVID-19 8 (see Fig. 1 ). Similarly, online posts claiming to reveal various “cures” for COVID-19 such as eating boiled garlic or drinking chlorine dioxide (which is an industrial bleach), were verified 9 as fake and in some cases as dangerous and will never cure the infection.

Fake news example about a self-test for COVID-19 source: https://cdn.factcheck.org/UploadedFiles/Screenshot031120_false.jpg , last access date: 26-12-2022
Social media outperformed television as the major news source for young people of the UK and the USA. 10 Moreover, as it is easier to generate and disseminate news online than with traditional media or face to face, large volumes of fake news are produced online for many reasons (Shu et al. 2017 ). Furthermore, it has been reported in a previous study about the spread of online news on Twitter (Vosoughi et al. 2018 ) that the spread of false news online is six times faster than truthful content and that 70% of the users could not distinguish real from fake news (Vosoughi et al. 2018 ) due to the attraction of the novelty of the latter (Bovet and Makse 2019 ). It was determined that falsehood spreads significantly farther, faster, deeper and more broadly than the truth in all categories of information, and the effects are more pronounced for false political news than for false news about terrorism, natural disasters, science, urban legends, or financial information (Vosoughi et al. 2018 ).
Over 1 million tweets were estimated to be related to fake news by the end of the 2016 US presidential election. 11 In 2017, in Germany, a government spokesman affirmed: “We are dealing with a phenomenon of a dimension that we have not seen before,” referring to an unprecedented spread of fake news on social networks. 12 Given the strength of this new phenomenon, fake news has been chosen as the word of the year by the Macquarie dictionary both in 2016 13 and in 2018 14 as well as by the Collins dictionary in 2017. 15 , 16 Since 2020, the new term “infodemic” was coined, reflecting widespread researchers’ concern (Gupta et al. 2022 ; Apuke and Omar 2021 ; Sharma et al. 2020 ; Hartley and Vu 2020 ; Micallef et al. 2020 ) about the proliferation of misinformation linked to the COVID-19 pandemic.
The Gartner Group’s top strategic predictions for 2018 and beyond included the need for IT leaders to quickly develop Artificial Intelligence (AI) algorithms to address counterfeit reality and fake news. 17 However, fake news identification is a complex issue. (Snow 2017 ) questioned the ability of AI to win the war against fake news. Similarly, other researchers concurred that even the best AI for spotting fake news is still ineffective. 18 Besides, recent studies have shown that the power of AI algorithms for identifying fake news is lower than its ability to create it Paschen ( 2019 ). Consequently, automatic fake news detection remains a huge challenge, primarily because the content is designed to closely resemble the truth in order to deceive users, and as a result, it is often hard to determine its veracity by AI alone. Therefore, it is crucial to consider more effective approaches to solve the problem of fake news in social media.
Contribution
The fake news problem has been addressed by researchers from various perspectives related to different topics. These topics include, but are not restricted to, social science studies , which investigate why and who falls for fake news (Altay et al. 2022 ; Batailler et al. 2022 ; Sterret et al. 2018 ; Badawy et al. 2019 ; Pennycook and Rand 2020 ; Weiss et al. 2020 ; Guadagno and Guttieri 2021 ), whom to trust and how perceptions of misinformation and disinformation relate to media trust and media consumption patterns (Hameleers et al. 2022 ), how fake news differs from personal lies (Chiu and Oh 2021 ; Escolà-Gascón 2021 ), examine how can the law regulate digital disinformation and how governments can regulate the values of social media companies that themselves regulate disinformation spread on their platforms (Marsden et al. 2020 ; Schuyler 2019 ; Vasu et al. 2018 ; Burshtein 2017 ; Waldman 2017 ; Alemanno 2018 ; Verstraete et al. 2017 ), and argue the challenges to democracy (Jungherr and Schroeder 2021 ); Behavioral interventions studies , which examine what literacy ideas mean in the age of dis/mis- and malinformation (Carmi et al. 2020 ), investigate whether media literacy helps identification of fake news (Jones-Jang et al. 2021 ) and attempt to improve people’s news literacy (Apuke et al. 2022 ; Dame Adjin-Tettey 2022 ; Hameleers 2022 ; Nagel 2022 ; Jones-Jang et al. 2021 ; Mihailidis and Viotty 2017 ; García et al. 2020 ) by encouraging people to pause to assess credibility of headlines (Fazio 2020 ), promote civic online reasoning (McGrew 2020 ; McGrew et al. 2018 ) and critical thinking (Lutzke et al. 2019 ), together with evaluations of credibility indicators (Bhuiyan et al. 2020 ; Nygren et al. 2019 ; Shao et al. 2018a ; Pennycook et al. 2020a , b ; Clayton et al. 2020 ; Ozturk et al. 2015 ; Metzger et al. 2020 ; Sherman et al. 2020 ; Nekmat 2020 ; Brashier et al. 2021 ; Chung and Kim 2021 ; Lanius et al. 2021 ); as well as social media-driven studies , which investigate the effect of signals (e.g., sources) to detect and recognize fake news (Vraga and Bode 2017 ; Jakesch et al. 2019 ; Shen et al. 2019 ; Avram et al. 2020 ; Hameleers et al. 2020 ; Dias et al. 2020 ; Nyhan et al. 2020 ; Bode and Vraga 2015 ; Tsang 2020 ; Vishwakarma et al. 2019 ; Yavary et al. 2020 ) and investigate fake and reliable news sources using complex networks analysis based on search engine optimization metric (Mazzeo and Rapisarda 2022 ).
The impacts of fake news have reached various areas and disciplines beyond online social networks and society (García et al. 2020 ) such as economics (Clarke et al. 2020 ; Kogan et al. 2019 ; Goldstein and Yang 2019 ), psychology (Roozenbeek et al. 2020a ; Van der Linden and Roozenbeek 2020 ; Roozenbeek and van der Linden 2019 ), political science (Valenzuela et al. 2022 ; Bringula et al. 2022 ; Ricard and Medeiros 2020 ; Van der Linden et al. 2020 ; Allcott and Gentzkow 2017 ; Grinberg et al. 2019 ; Guess et al. 2019 ; Baptista and Gradim 2020 ), health science (Alonso-Galbán and Alemañy-Castilla 2022 ; Desai et al. 2022 ; Apuke and Omar 2021 ; Escolà-Gascón 2021 ; Wang et al. 2019c ; Hartley and Vu 2020 ; Micallef et al. 2020 ; Pennycook et al. 2020b ; Sharma et al. 2020 ; Roozenbeek et al. 2020b ), environmental science (e.g., climate change) (Treen et al. 2020 ; Lutzke et al. 2019 ; Lewandowsky 2020 ; Maertens et al. 2020 ), etc.
Interesting research has been carried out to review and study the fake news issue in online social networks. Some focus not only on fake news, but also distinguish between fake news and rumor (Bondielli and Marcelloni 2019 ; Meel and Vishwakarma 2020 ), while others tackle the whole problem, from characterization to processing techniques (Shu et al. 2017 ; Guo et al. 2020 ; Zhou and Zafarani 2020 ). However, they mostly focus on studying approaches from a machine learning perspective (Bondielli and Marcelloni 2019 ), data mining perspective (Shu et al. 2017 ), crowd intelligence perspective (Guo et al. 2020 ), or knowledge-based perspective (Zhou and Zafarani 2020 ). Furthermore, most of these studies ignore at least one of the mentioned perspectives, and in many cases, they do not cover other existing detection approaches using methods such as blockchain and fact-checking, as well as analysis on metrics used for Search Engine Optimization (Mazzeo and Rapisarda 2022 ). However, in our work and to the best of our knowledge, we cover all the approaches used for fake news detection. Indeed, we investigate the proposed solutions from broader perspectives (i.e., the detection techniques that are used, as well as the different aspects and types of the information used).
Therefore, in this paper, we are highly motivated by the following facts. First, fake news detection on social media is still in the early age of development, and many challenging issues remain that require deeper investigation. Hence, it is necessary to discuss potential research directions that can improve fake news detection and mitigation tasks. However, the dynamic nature of fake news propagation through social networks further complicates matters (Sharma et al. 2019 ). False information can easily reach and impact a large number of users in a short time (Friggeri et al. 2014 ; Qian et al. 2018 ). Moreover, fact-checking organizations cannot keep up with the dynamics of propagation as they require human verification, which can hold back a timely and cost-effective response (Kim et al. 2018 ; Ruchansky et al. 2017 ; Shu et al. 2018a ).
Our work focuses primarily on understanding the “fake news” problem, its related challenges and root causes, and reviewing automatic fake news detection and mitigation methods in online social networks as addressed by researchers. The main contributions that differentiate us from other works are summarized below:
- We present the general context from which the fake news problem emerged (i.e., online deception)
- We review existing definitions of fake news, identify the terms and features most commonly used to define fake news, and categorize related works accordingly.
- We propose a fake news typology classification based on the various categorizations of fake news reported in the literature.
- We point out the most challenging factors preventing researchers from proposing highly effective solutions for automatic fake news detection in social media.
- We highlight and classify representative studies in the domain of automatic fake news detection and mitigation on online social networks including the key methods and techniques used to generate detection models.
- We discuss the key shortcomings that may inhibit the effectiveness of the proposed fake news detection methods in online social networks.
- We provide recommendations that can help address these shortcomings and improve the quality of research in this domain.
The rest of this article is organized as follows. We explain the methodology with which the studied references are collected and selected in Sect. 2 . We introduce the online deception problem in Sect. 3 . We highlight the modern-day problem of fake news in Sect. 4 , followed by challenges facing fake news detection and mitigation tasks in Sect. 5 . We provide a comprehensive literature review of the most relevant scholarly works on fake news detection in Sect. 6 . We provide a critical discussion and recommendations that may fill some of the gaps we have identified, as well as a classification of the reviewed automatic fake news detection approaches, in Sect. 7 . Finally, we provide a conclusion and propose some future directions in Sect. 8 .
Review methodology
This section introduces the systematic review methodology on which we relied to perform our study. We start with the formulation of the research questions, which allowed us to select the relevant research literature. Then, we provide the different sources of information together with the search and inclusion/exclusion criteria we used to select the final set of papers.
Research questions formulation
The research scope, research questions, and inclusion/exclusion criteria were established following an initial evaluation of the literature and the following research questions were formulated and addressed.
- RQ1: what is fake news in social media, how is it defined in the literature, what are its related concepts, and the different types of it?
- RQ2: What are the existing challenges and issues related to fake news?
- RQ3: What are the available techniques used to perform fake news detection in social media?
Sources of information
We broadly searched for journal and conference research articles, books, and magazines as a source of data to extract relevant articles. We used the main sources of scientific databases and digital libraries in our search, such as Google Scholar, 19 IEEE Xplore, 20 Springer Link, 21 ScienceDirect, 22 Scopus, 23 ACM Digital Library. 24 Also, we screened most of the related high-profile conferences such as WWW, SIGKDD, VLDB, ICDE and so on to find out the recent work.
Search criteria
We focused our research over a period of ten years, but we made sure that about two-thirds of the research papers that we considered were published in or after 2019. Additionally, we defined a set of keywords to search the above-mentioned scientific databases since we concentrated on reviewing the current state of the art in addition to the challenges and the future direction. The set of keywords includes the following terms: fake news, disinformation, misinformation, information disorder, social media, detection techniques, detection methods, survey, literature review.
Study selection, exclusion and inclusion criteria
To retrieve relevant research articles, based on our sources of information and search criteria, a systematic keyword-based search was carried out by posing different search queries, as shown in Table 1 .
List of keywords for searching relevant articles
Fake news + social media |
Fake news + disinformation |
Fake news + misinformation |
Fake news + information disorder |
Fake news + survey |
Fake news + detection methods |
Fake news + literature review |
Fake news + detection techniques |
Fake news + detection + social media |
Disinformation + misinformation + social media |
We discovered a primary list of articles. On the obtained initial list of studies, we applied a set of inclusion/exclusion criteria presented in Table 2 to select the appropriate research papers. The inclusion and exclusion principles are applied to determine whether a study should be included or not.
Inclusion and exclusion criteria
Inclusion criterion | Exclusion criterion |
---|---|
Peer-reviewed and written in the English language | Articles in a different language than English. |
Clearly describes fake news, misinformation and disinformation problems in social networks | Does not focus on fake news, misinformation, or disinformation problem in social networks |
Written by academic or industrial researchers | Short papers, posters or similar |
Have a high number of citations | |
Recent articles only (last ten years) | |
In the case of equivalent studies, the one published in the highest-rated journal or conference is selected to sustain a high-quality set of articles on which the review is conducted | Articles not following these inclusion criteria |
Articles that propose methodologies, methods, or approaches for fake news detection online social networks |
After reading the abstract, we excluded some articles that did not meet our criteria. We chose the most important research to help us understand the field. We reviewed the articles completely and found only 61 research papers that discuss the definition of the term fake news and its related concepts (see Table 4 ). We used the remaining papers to understand the field, reveal the challenges, review the detection techniques, and discuss future directions.
Classification of fake news definitions based on the used term and features
Fake news | Misinformation | Disinformation | False information | Malinformation | Information disorder | |
---|---|---|---|---|---|---|
Intent and authenticity | Shu et al. ( ), Sharma et al. ( ), Mustafaraj and Metaxas ( ), Klein and Wueller ( ), Potthast et al. ( ), Allcott and Gentzkow ( ), Zhou and Zafarani ( ), Zhang and Ghorbani ( ), Conroy et al. ( ), Celliers and Hattingh ( ), Nakov ( ), Shu et al. ( ), Tandoc Jr et al. ( ), Abu Arqoub et al. ( ),Molina et al. ( ), de Cock Buning ( ), Meel and Vishwakarma ( ) | Wu et al. ( ), Shu et al. ( ), Islam et al. ( ), Hameleers et al. ( ) | Kapantai et al. ( ), Shu et al. ( ), Shu et al. ( ),Kumar et al. ( ), Jungherr and Schroeder ( ), Starbird et al. ( ), de Cock Buning ( ), Bastick ( ), Bringula et al. ( ), Tsang ( ), Hameleers et al. ( ), Wu et al. ( ) | – | Shu et al. ( ), Di Domenico et al. ( ), Dame Adjin-Tettey ( ) | Wardle and Derakhshan ( ), Wardle Wardle ( ), Derakhshan and Wardle ( ), Shu et al. ( ) |
Intent or authenticity | Jin et al. ( ), Rubin et al. ( ), Balmas ( ),Brewer et al. ( ), Egelhofer and Lecheler ( ), Lazer et al. ( ), Allen et al. ( ), Guadagno and Guttieri ( ), Van der Linden et al. ( ), ERGA ( ) | Pennycook and Rand ( ), Shao et al. ( ), Shao et al. ( ),Micallef et al. ( ), Ha et al. ( ), Singh et al. ( ), Wu et al. ( ) | Marsden et al. ( ), Ireton and Posetti ( ), ERGA ( ), Baptista and Gradim ( ) | Habib et al. ( ) | Carmi et al. ( ) | – |
Intent and knowledge | Weiss et al. ( ) | – | Bhattacharjee et al. ( ), Khan et al. ( ) | Kumar and Shah ( ), Guo et al. ( ) | – | – |
A brief introduction of online deception
The Cambridge Online Dictionary defines Deception as “ the act of hiding the truth, especially to get an advantage .” Deception relies on peoples’ trust, doubt and strong emotions that may prevent them from thinking and acting clearly (Aïmeur et al. 2018 ). We also define it in previous work (Aïmeur et al. 2018 ) as the process that undermines the ability to consciously make decisions and take convenient actions, following personal values and boundaries. In other words, deception gets people to do things they would not otherwise do. In the context of online deception, several factors need to be considered: the deceiver, the purpose or aim of the deception, the social media service, the deception technique and the potential target (Aïmeur et al. 2018 ; Hage et al. 2021 ).
Researchers are working on developing new ways to protect users and prevent online deception (Aïmeur et al. 2018 ). Due to the sophistication of attacks, this is a complex task. Hence, malicious attackers are using more complex tools and strategies to deceive users. Furthermore, the way information is organized and exchanged in social media may lead to exposing OSN users to many risks (Aïmeur et al. 2013 ).
In fact, this field is one of the recent research areas that need collaborative efforts of multidisciplinary practices such as psychology, sociology, journalism, computer science as well as cyber-security and digital marketing (which are not yet well explored in the field of dis/mis/malinformation but relevant for future research). Moreover, Ismailov et al. ( 2020 ) analyzed the main causes that could be responsible for the efficiency gap between laboratory results and real-world implementations.
In this paper, it is not in our scope of work to review online deception state of the art. However, we think it is crucial to note that fake news, misinformation and disinformation are indeed parts of the larger landscape of online deception (Hage et al. 2021 ).
Fake news, the modern-day problem
Fake news has existed for a very long time, much before their wide circulation became facilitated by the invention of the printing press. 25 For instance, Socrates was condemned to death more than twenty-five hundred years ago under the fake news that he was guilty of impiety against the pantheon of Athens and corruption of the youth. 26 A Google Trends Analysis of the term “fake news” reveals an explosion in popularity around the time of the 2016 US presidential election. 27 Fake news detection is a problem that has recently been addressed by numerous organizations, including the European Union 28 and NATO. 29
In this section, we first overview the fake news definitions as they were provided in the literature. We identify the terms and features used in the definitions, and we classify the latter based on them. Then, we provide a fake news typology based on distinct categorizations that we propose, and we define and compare the most cited forms of one specific fake news category (i.e., the intent-based fake news category).
Definitions of fake news
“Fake news” is defined in the Collins English Dictionary as false and often sensational information disseminated under the guise of news reporting, 30 yet the term has evolved over time and has become synonymous with the spread of false information (Cooke 2017 ).
The first definition of the term fake news was provided by Allcott and Gentzkow ( 2017 ) as news articles that are intentionally and verifiably false and could mislead readers. Then, other definitions were provided in the literature, but they all agree on the authenticity of fake news to be false (i.e., being non-factual). However, they disagree on the inclusion and exclusion of some related concepts such as satire , rumors , conspiracy theories , misinformation and hoaxes from the given definition. More recently, Nakov ( 2020 ) reported that the term fake news started to mean different things to different people, and for some politicians, it even means “news that I do not like.”
Hence, there is still no agreed definition of the term “fake news.” Moreover, we can find many terms and concepts in the literature that refer to fake news (Van der Linden et al. 2020 ; Molina et al. 2021 ) (Abu Arqoub et al. 2022 ; Allen et al. 2020 ; Allcott and Gentzkow 2017 ; Shu et al. 2017 ; Sharma et al. 2019 ; Zhou and Zafarani 2020 ; Zhang and Ghorbani 2020 ; Conroy et al. 2015 ; Celliers and Hattingh 2020 ; Nakov 2020 ; Shu et al. 2020c ; Jin et al. 2016 ; Rubin et al. 2016 ; Balmas 2014 ; Brewer et al. 2013 ; Egelhofer and Lecheler 2019 ; Mustafaraj and Metaxas 2017 ; Klein and Wueller 2017 ; Potthast et al. 2017 ; Lazer et al. 2018 ; Weiss et al. 2020 ; Tandoc Jr et al. 2021 ; Guadagno and Guttieri 2021 ), disinformation (Kapantai et al. 2021 ; Shu et al. 2020a , c ; Kumar et al. 2016 ; Bhattacharjee et al. 2020 ; Marsden et al. 2020 ; Jungherr and Schroeder 2021 ; Starbird et al. 2019 ; Ireton and Posetti 2018 ), misinformation (Wu et al. 2019 ; Shu et al. 2020c ; Shao et al. 2016 , 2018b ; Pennycook and Rand 2019 ; Micallef et al. 2020 ), malinformation (Dame Adjin-Tettey 2022 ) (Carmi et al. 2020 ; Shu et al. 2020c ), false information (Kumar and Shah 2018 ; Guo et al. 2020 ; Habib et al. 2019 ), information disorder (Shu et al. 2020c ; Wardle and Derakhshan 2017 ; Wardle 2018 ; Derakhshan and Wardle 2017 ), information warfare (Guadagno and Guttieri 2021 ) and information pollution (Meel and Vishwakarma 2020 ).
There is also a remarkable amount of disagreement over the classification of the term fake news in the research literature, as well as in policy (de Cock Buning 2018 ; ERGA 2018 , 2021 ). Some consider fake news as a type of misinformation (Allen et al. 2020 ; Singh et al. 2021 ; Ha et al. 2021 ; Pennycook and Rand 2019 ; Shao et al. 2018b ; Di Domenico et al. 2021 ; Sharma et al. 2019 ; Celliers and Hattingh 2020 ; Klein and Wueller 2017 ; Potthast et al. 2017 ; Islam et al. 2020 ), others consider it as a type of disinformation (de Cock Buning 2018 ) (Bringula et al. 2022 ; Baptista and Gradim 2022 ; Tsang 2020 ; Tandoc Jr et al. 2021 ; Bastick 2021 ; Khan et al. 2019 ; Shu et al. 2017 ; Nakov 2020 ; Shu et al. 2020c ; Egelhofer and Lecheler 2019 ), while others associate the term with both disinformation and misinformation (Wu et al. 2022 ; Dame Adjin-Tettey 2022 ; Hameleers et al. 2022 ; Carmi et al. 2020 ; Allcott and Gentzkow 2017 ; Zhang and Ghorbani 2020 ; Potthast et al. 2017 ; Weiss et al. 2020 ; Tandoc Jr et al. 2021 ; Guadagno and Guttieri 2021 ). On the other hand, some prefer to differentiate fake news from both terms (ERGA 2018 ; Molina et al. 2021 ; ERGA 2021 ) (Zhou and Zafarani 2020 ; Jin et al. 2016 ; Rubin et al. 2016 ; Balmas 2014 ; Brewer et al. 2013 ).
The existing terms can be separated into two groups. The first group represents the general terms, which are information disorder , false information and fake news , each of which includes a subset of terms from the second group. The second group represents the elementary terms, which are misinformation , disinformation and malinformation . The literature agrees on the definitions of the latter group, but there is still no agreed-upon definition of the first group. In Fig. 2 , we model the relationship between the most used terms in the literature.

Modeling of the relationship between terms related to fake news
The terms most used in the literature to refer, categorize and classify fake news can be summarized and defined as shown in Table 3 , in which we capture the similarities and show the differences between the different terms based on two common key features, which are the intent and the authenticity of the news content. The intent feature refers to the intention behind the term that is used (i.e., whether or not the purpose is to mislead or cause harm), whereas the authenticity feature refers to its factual aspect. (i.e., whether the content is verifiably false or not, which we label as genuine in the second case). Some of these terms are explicitly used to refer to fake news (i.e., disinformation, misinformation and false information), while others are not (i.e., malinformation). In the comparison table, the empty dash (–) cell denotes that the classification does not apply.
A comparison between used terms based on intent and authenticity
Term | Definition | Intent | Authenticity |
---|---|---|---|
False information | Verifiably false information | – | False |
Misinformation | False information that is shared without the intention to mislead or to cause harm | Not to mislead | False |
Disinformation | False information that is shared to intentionally mislead | To mislead | False |
Malinformation | Genuine information that is shared with an intent to cause harm | To cause harm | Genuine |
In Fig. 3 , we identify the different features used in the literature to define fake news (i.e., intent, authenticity and knowledge). Hence, some definitions are based on two key features, which are authenticity and intent (i.e., news articles that are intentionally and verifiably false and could mislead readers). However, other definitions are based on either authenticity or intent. Other researchers categorize false information on the web and social media based on its intent and knowledge (i.e., when there is a single ground truth). In Table 4 , we classify the existing fake news definitions based on the used term and the used features . In the classification, the references in the cells refer to the research study in which a fake news definition was provided, while the empty dash (–) cells denote that the classification does not apply.

The features used for fake news definition
Fake news typology
Various categorizations of fake news have been provided in the literature. We can distinguish two major categories of fake news based on the studied perspective (i.e., intention or content) as shown in Fig. 4 . However, our proposed fake news typology is not about detection methods, and it is not exclusive. Hence, a given category of fake news can be described based on both perspectives (i.e., intention and content) at the same time. For instance, satire (i.e., intent-based fake news) can contain text and/or multimedia content types of data (e.g., headline, body, image, video) (i.e., content-based fake news) and so on.

Most researchers classify fake news based on the intent (Collins et al. 2020 ; Bondielli and Marcelloni 2019 ; Zannettou et al. 2019 ; Kumar et al. 2016 ; Wardle 2017 ; Shu et al. 2017 ; Kumar and Shah 2018 ) (see Sect. 4.2.2 ). However, other researchers (Parikh and Atrey 2018 ; Fraga-Lamas and Fernández-Caramés 2020 ; Hasan and Salah 2019 ; Masciari et al. 2020 ; Bakdash et al. 2018 ; Elhadad et al. 2019 ; Yang et al. 2019b ) focus on the content to categorize types of fake news through distinguishing the different formats and content types of data in the news (e.g., text and/or multimedia).
Recently, another classification was proposed by Zhang and Ghorbani ( 2020 ). It is based on the combination of content and intent to categorize fake news. They distinguish physical news content and non-physical news content from fake news. Physical content consists of the carriers and format of the news, and non-physical content consists of the opinions, emotions, attitudes and sentiments that the news creators want to express.
Content-based fake news category
According to researchers of this category (Parikh and Atrey 2018 ; Fraga-Lamas and Fernández-Caramés 2020 ; Hasan and Salah 2019 ; Masciari et al. 2020 ; Bakdash et al. 2018 ; Elhadad et al. 2019 ; Yang et al. 2019b ), forms of fake news may include false text such as hyperlinks or embedded content; multimedia such as false videos (Demuyakor and Opata 2022 ), images (Masciari et al. 2020 ; Shen et al. 2019 ), audios (Demuyakor and Opata 2022 ) and so on. Moreover, we can also find multimodal content (Shu et al. 2020a ) that is fake news articles and posts composed of multiple types of data combined together, for example, a fabricated image along with a text related to the image (Shu et al. 2020a ). In this category of fake news forms, we can mention as examples deepfake videos (Yang et al. 2019b ) and GAN-generated fake images (Zhang et al. 2019b ), which are artificial intelligence-based machine-generated fake content that are hard for unsophisticated social network users to identify.
The effects of these forms of fake news content vary on the credibility assessment, as well as sharing intentions which influences the spread of fake news on OSNs. For instance, people with little knowledge about the issue compared to those who are strongly concerned about the key issue of fake news tend to be easier to convince that the misleading or fake news is real, especially when shared via a video modality as compared to the text or the audio modality (Demuyakor and Opata 2022 ).
Intent-based Fake News Category
The most often mentioned and discussed forms of fake news according to researchers in this category include but are not restricted to clickbait , hoax , rumor , satire , propaganda , framing , conspiracy theories and others. In the following subsections, we explain these types of fake news as they were defined in the literature and undertake a brief comparison between them as depicted in Table 5 . The following are the most cited forms of intent-based types of fake news, and their comparison is based on what we suspect are the most common criteria mentioned by researchers.
A comparison between the different types of intent-based fake news
Intent to deceive | Propagation | Negative Impact | Goal | |
---|---|---|---|---|
Clickbait | High | Slow | Low | Popularity, Profit |
Hoax | High | Fast | Low | Other |
Rumor | High | Fast | High | Other |
Satire | Low | Slow | Low | Popularity, Other |
Propaganda | High | Fast | High | Popularity |
Framing | High | Fast | Low | Other |
Conspiracy theory | High | Fast | High | Other |
Clickbait refers to misleading headlines and thumbnails of content on the web (Zannettou et al. 2019 ) that tend to be fake stories with catchy headlines aimed at enticing the reader to click on a link (Collins et al. 2020 ). This type of fake news is considered to be the least severe type of false information because if a user reads/views the whole content, it is possible to distinguish if the headline and/or the thumbnail was misleading (Zannettou et al. 2019 ). However, the goal behind using clickbait is to increase the traffic to a website (Zannettou et al. 2019 ).
A hoax is a false (Zubiaga et al. 2018 ) or inaccurate (Zannettou et al. 2019 ) intentionally fabricated (Collins et al. 2020 ) news story used to masquerade the truth (Zubiaga et al. 2018 ) and is presented as factual (Zannettou et al. 2019 ) to deceive the public or audiences (Collins et al. 2020 ). This category is also known either as half-truth or factoid stories (Zannettou et al. 2019 ). Popular examples of hoaxes are stories that report the false death of celebrities (Zannettou et al. 2019 ) and public figures (Collins et al. 2020 ). Recently, hoaxes about the COVID-19 have been circulating through social media.
The term rumor refers to ambiguous or never confirmed claims (Zannettou et al. 2019 ) that are disseminated with a lack of evidence to support them (Sharma et al. 2019 ). This kind of information is widely propagated on OSNs (Zannettou et al. 2019 ). However, they are not necessarily false and may turn out to be true (Zubiaga et al. 2018 ). Rumors originate from unverified sources but may be true or false or remain unresolved (Zubiaga et al. 2018 ).
Satire refers to stories that contain a lot of irony and humor (Zannettou et al. 2019 ). It presents stories as news that might be factually incorrect, but the intent is not to deceive but rather to call out, ridicule, or to expose behavior that is shameful, corrupt, or otherwise “bad” (Golbeck et al. 2018 ). This is done with a fabricated story or by exaggerating the truth reported in mainstream media in the form of comedy (Collins et al. 2020 ). The intent behind satire seems kind of legitimate and many authors (such as Wardle (Wardle 2017 )) do include satire as a type of fake news as there is no intention to cause harm but it has the potential to mislead or fool people.
Also, Golbeck et al. ( 2018 ) mention that there is a spectrum from fake to satirical news that they found to be exploited by many fake news sites. These sites used disclaimers at the bottom of their webpages to suggest they were “satirical” even when there was nothing satirical about their articles, to protect them from accusations about being fake. The difference with a satirical form of fake news is that the authors or the host present themselves as a comedian or as an entertainer rather than a journalist informing the public (Collins et al. 2020 ). However, most audiences believed the information passed in this satirical form because the comedian usually projects news from mainstream media and frames them to suit their program (Collins et al. 2020 ).
Propaganda refers to news stories created by political entities to mislead people. It is a special instance of fabricated stories that aim to harm the interests of a particular party and, typically, has a political context (Zannettou et al. 2019 ). Propaganda was widely used during both World Wars (Collins et al. 2020 ) and during the Cold War (Zannettou et al. 2019 ). It is a consequential type of false information as it can change the course of human history (e.g., by changing the outcome of an election) (Zannettou et al. 2019 ). States are the main actors of propaganda. Recently, propaganda has been used by politicians and media organizations to support a certain position or view (Collins et al. 2020 ). Online astroturfing can be an example of the tools used for the dissemination of propaganda. It is a covert manipulation of public opinion (Peng et al. 2017 ) that aims to make it seem that many people share the same opinion about something. Astroturfing can affect different domains of interest, based on which online astroturfing can be mainly divided into political astroturfing, corporate astroturfing and astroturfing in e-commerce or online services (Mahbub et al. 2019 ). Propaganda types of fake news can be debunked with manual fact-based detection models such as the use of expert-based fact-checkers (Collins et al. 2020 ).
Framing refers to employing some aspect of reality to make content more visible, while the truth is concealed (Collins et al. 2020 ) to deceive and misguide readers. People will understand certain concepts based on the way they are coined and invented. An example of framing was provided by Collins et al. ( 2020 ): “suppose a leader X says “I will neutralize my opponent” simply meaning he will beat his opponent in a given election. Such a statement will be framed such as “leader X threatens to kill Y” and this framed statement provides a total misrepresentation of the original meaning.
Conspiracy Theories
Conspiracy theories refer to the belief that an event is the result of secret plots generated by powerful conspirators. Conspiracy belief refers to people’s adoption and belief of conspiracy theories, and it is associated with psychological, political and social factors (Douglas et al. 2019 ). Conspiracy theories are widespread in contemporary democracies (Sutton and Douglas 2020 ), and they have major consequences. For instance, lately and during the COVID-19 pandemic, conspiracy theories have been discussed from a public health perspective (Meese et al. 2020 ; Allington et al. 2020 ; Freeman et al. 2020 ).
Comparison Between Most Popular Intent-based Types of Fake News
Following a review of the most popular intent-based types of fake news, we compare them as shown in Table 5 based on the most common criteria mentioned by researchers in their definitions as listed below.
- the intent behind the news, which refers to whether a given news type was mainly created to intentionally deceive people or not (e.g., humor, irony, entertainment, etc.);
- the way that the news propagates through OSN, which determines the nature of the propagation of each type of fake news and this can be either fast or slow propagation;
- the severity of the impact of the news on OSN users, which refers to whether the public has been highly impacted by the given type of fake news; the mentioned impact of each fake news type is mainly the proportion of the negative impact;
- and the goal behind disseminating the news, which can be to gain popularity for a particular entity (e.g., political party), for profit (e.g., lucrative business), or other reasons such as humor and irony in the case of satire, spreading panic or anger, and manipulating the public in the case of hoaxes, made-up stories about a particular person or entity in the case of rumors, and misguiding readers in the case of framing.
However, the comparison provided in Table 5 is deduced from the studied research papers; it is our point of view, which is not based on empirical data.
We suspect that the most dangerous types of fake news are the ones with high intention to deceive the public, fast propagation through social media, high negative impact on OSN users, and complicated hidden goals and agendas. However, while the other types of fake news are less dangerous, they should not be ignored.
Moreover, it is important to highlight that the existence of the overlap in the types of fake news mentioned above has been proven, thus it is possible to observe false information that may fall within multiple categories (Zannettou et al. 2019 ). Here, we provide two examples by Zannettou et al. ( 2019 ) to better understand possible overlaps: (1) a rumor may also use clickbait techniques to increase the audience that will read the story; and (2) propaganda stories, as a special instance of a framing story.
Challenges related to fake news detection and mitigation
To alleviate fake news and its threats, it is crucial to first identify and understand the factors involved that continue to challenge researchers. Thus, the main question is to explore and investigate the factors that make it easier to fall for manipulated information. Despite the tremendous progress made in alleviating some of the challenges in fake news detection (Sharma et al. 2019 ; Zhou and Zafarani 2020 ; Zhang and Ghorbani 2020 ; Shu et al. 2020a ), much more work needs to be accomplished to address the problem effectively.
In this section, we discuss several open issues that have been making fake news detection in social media a challenging problem. These issues can be summarized as follows: content-based issues (i.e., deceptive content that resembles the truth very closely), contextual issues (i.e., lack of user awareness, social bots spreaders of fake content, and OSN’s dynamic natures that leads to the fast propagation), as well as the issue of existing datasets (i.e., there still no one size fits all benchmark dataset for fake news detection). These various aspects have proven (Shu et al. 2017 ) to have a great impact on the accuracy of fake news detection approaches.
Content-based issue, deceptive content
Automatic fake news detection remains a huge challenge, primarily because the content is designed in a way that it closely resembles the truth. Besides, most deceivers choose their words carefully and use their language strategically to avoid being caught. Therefore, it is often hard to determine its veracity by AI without the reliance on additional information from third parties such as fact-checkers.
Abdullah-All-Tanvir et al. ( 2020 ) reported that fake news tends to have more complicated stories and hardly ever make any references. It is more likely to contain a greater number of words that express negative emotions. This makes it so complicated that it becomes impossible for a human to manually detect the credibility of this content. Therefore, detecting fake news on social media is quite challenging. Moreover, fake news appears in multiple types and forms, which makes it hard and challenging to define a single global solution able to capture and deal with the disseminated content. Consequently, detecting false information is not a straightforward task due to its various types and forms Zannettou et al. ( 2019 ).
Contextual issues
Contextual issues are challenges that we suspect may not be related to the content of the news but rather they are inferred from the context of the online news post (i.e., humans are the weakest factor due to lack of user awareness, social bots spreaders, dynamic nature of online social platforms and fast propagation of fake news).
Humans are the weakest factor due to the lack of awareness
Recent statistics 31 show that the percentage of unintentional fake news spreaders (people who share fake news without the intention to mislead) over social media is five times higher than intentional spreaders. Moreover, another recent statistic 32 shows that the percentage of people who were confident about their ability to discern fact from fiction is ten times higher than those who were not confident about the truthfulness of what they are sharing. As a result, we can deduce the lack of human awareness about the ascent of fake news.
Public susceptibility and lack of user awareness (Sharma et al. 2019 ) have always been the most challenging problem when dealing with fake news and misinformation. This is a complex issue because many people believe almost everything on the Internet and the ones who are new to digital technology or have less expertise may be easily fooled (Edgerly et al. 2020 ).
Moreover, it has been widely proven (Metzger et al. 2020 ; Edgerly et al. 2020 ) that people are often motivated to support and accept information that goes with their preexisting viewpoints and beliefs, and reject information that does not fit in as well. Hence, Shu et al. ( 2017 ) illustrate an interesting correlation between fake news spread and psychological and cognitive theories. They further suggest that humans are more likely to believe information that confirms their existing views and ideological beliefs. Consequently, they deduce that humans are naturally not very good at differentiating real information from fake information.
Recent research by Giachanou et al. ( 2020 ) studies the role of personality and linguistic patterns in discriminating between fake news spreaders and fact-checkers. They classify a user as a potential fact-checker or a potential fake news spreader based on features that represent users’ personality traits and linguistic patterns used in their tweets. They show that leveraging personality traits and linguistic patterns can improve the performance in differentiating between checkers and spreaders.
Furthermore, several researchers studied the prevalence of fake news on social networks during (Allcott and Gentzkow 2017 ; Grinberg et al. 2019 ; Guess et al. 2019 ; Baptista and Gradim 2020 ) and after (Garrett and Bond 2021 ) the 2016 US presidential election and found that individuals most likely to engage with fake news sources were generally conservative-leaning, older, and highly engaged with political news.
Metzger et al. ( 2020 ) examine how individuals evaluate the credibility of biased news sources and stories. They investigate the role of both cognitive dissonance and credibility perceptions in selective exposure to attitude-consistent news information. They found that online news consumers tend to perceive attitude-consistent news stories as more accurate and more credible than attitude-inconsistent stories.
Similarly, Edgerly et al. ( 2020 ) explore the impact of news headlines on the audience’s intent to verify whether given news is true or false. They concluded that participants exhibit higher intent to verify the news only when they believe the headline to be true, which is predicted by perceived congruence with preexisting ideological tendencies.
Luo et al. ( 2022 ) evaluate the effects of endorsement cues in social media on message credibility and detection accuracy. Results showed that headlines associated with a high number of likes increased credibility, thereby enhancing detection accuracy for real news but undermining accuracy for fake news. Consequently, they highlight the urgency of empowering individuals to assess both news veracity and endorsement cues appropriately on social media.
Moreover, misinformed people are a greater problem than uninformed people (Kuklinski et al. 2000 ), because the former hold inaccurate opinions (which may concern politics, climate change, medicine) that are harder to correct. Indeed, people find it difficult to update their misinformation-based beliefs even after they have been proved to be false (Flynn et al. 2017 ). Moreover, even if a person has accepted the corrected information, his/her belief may still affect their opinion (Nyhan and Reifler 2015 ).
Falling for disinformation may also be explained by a lack of critical thinking and of the need for evidence that supports information (Vilmer et al. 2018 ; Badawy et al. 2019 ). However, it is also possible that people choose misinformation because they engage in directionally motivated reasoning (Badawy et al. 2019 ; Flynn et al. 2017 ). Online clients are normally vulnerable and will, in general, perceive web-based networking media as reliable, as reported by Abdullah-All-Tanvir et al. ( 2019 ), who propose to mechanize fake news recognition.
It is worth noting that in addition to bots causing the outpouring of the majority of the misrepresentations, specific individuals are also contributing a large share of this issue (Abdullah-All-Tanvir et al. 2019 ). Furthermore, Vosoughi et al. (Vosoughi et al. 2018 ) found that contrary to conventional wisdom, robots have accelerated the spread of real and fake news at the same rate, implying that fake news spreads more than the truth because humans, not robots, are more likely to spread it.
In this case, verified users and those with numerous followers were not necessarily responsible for spreading misinformation of the corrupted posts (Abdullah-All-Tanvir et al. 2019 ).
Viral fake news can cause much havoc to our society. Therefore, to mitigate the negative impact of fake news, it is important to analyze the factors that lead people to fall for misinformation and to further understand why people spread fake news (Cheng et al. 2020 ). Measuring the accuracy, credibility, veracity and validity of news contents can also be a key countermeasure to consider.
Social bots spreaders
Several authors (Shu et al. 2018b , 2017 ; Shi et al. 2019 ; Bessi and Ferrara 2016 ; Shao et al. 2018a ) have also shown that fake news is likely to be created and spread by non-human accounts with similar attributes and structure in the network, such as social bots (Ferrara et al. 2016 ). Bots (short for software robots) exist since the early days of computers. A social bot is a computer algorithm that automatically produces content and interacts with humans on social media, trying to emulate and possibly alter their behavior (Ferrara et al. 2016 ). Although they are designed to provide a useful service, they can be harmful, for example when they contribute to the spread of unverified information or rumors (Ferrara et al. 2016 ). However, it is important to note that bots are simply tools created and maintained by humans for some specific hidden agendas.
Social bots tend to connect with legitimate users instead of other bots. They try to act like a human with fewer words and fewer followers on social media. This contributes to the forwarding of fake news (Jiang et al. 2019 ). Moreover, there is a difference between bot-generated and human-written clickbait (Le et al. 2019 ).
Many researchers have addressed ways of identifying and analyzing possible sources of fake news spread in social media. Recent research by Shu et al. ( 2020a ) describes social bots use of two strategies to spread low-credibility content. First, they amplify interactions with content as soon as it is created to make it look legitimate and to facilitate its spread across social networks. Next, they try to increase public exposure to the created content and thus boost its perceived credibility by targeting influential users that are more likely to believe disinformation in the hope of getting them to “repost” the fabricated content. They further discuss the social bot detection systems taxonomy proposed by Ferrara et al. ( 2016 ) which divides bot detection methods into three classes: (1) graph-based, (2) crowdsourcing and (3) feature-based social bot detection methods.
Similarly, Shao et al. ( 2018a ) examine social bots and how they promote the spread of misinformation through millions of Twitter posts during and following the 2016 US presidential campaign. They found that social bots played a disproportionate role in spreading articles from low-credibility sources by amplifying such content in the early spreading moments and targeting users with many followers through replies and mentions to expose them to this content and induce them to share it.
Ismailov et al. ( 2020 ) assert that the techniques used to detect bots depend on the social platform and the objective. They note that a malicious bot designed to make friends with as many accounts as possible will require a different detection approach than a bot designed to repeatedly post links to malicious websites. Therefore, they identify two models for detecting malicious accounts, each using a different set of features. Social context models achieve detection by examining features related to an account’s social presence including features such as relationships to other accounts, similarities to other users’ behaviors, and a variety of graph-based features. User behavior models primarily focus on features related to an individual user’s behavior, such as frequency of activities (e.g., number of tweets or posts per time interval), patterns of activity and clickstream sequences.
Therefore, it is crucial to consider bot detection techniques to distinguish bots from normal users to better leverage user profile features to detect fake news.
However, there is also another “bot-like” strategy that aims to massively promote disinformation and fake content in social platforms, which is called bot farms or also troll farms. It is not social bots, but it is a group of organized individuals engaging in trolling or bot-like promotion of narratives in a coordinated fashion (Wardle 2018 ) hired to massively spread fake news or any other harmful content. A prominent troll farm example is the Russia-based Internet Research Agency (IRA), which disseminated inflammatory content online to influence the outcome of the 2016 U.S. presidential election. 33 As a result, Twitter suspended accounts connected to the IRA and deleted 200,000 tweets from Russian trolls (Jamieson 2020 ). Another example to mention in this category is review bombing (Moro and Birt 2022 ). Review bombing refers to coordinated groups of people massively performing the same negative actions online (e.g., dislike, negative review/comment) on an online video, game, post, product, etc., in order to reduce its aggregate review score. The review bombers can be both humans and bots coordinated in order to cause harm and mislead people by falsifying facts.
Dynamic nature of online social platforms and fast propagation of fake news
Sharma et al. ( 2019 ) affirm that the fast proliferation of fake news through social networks makes it hard and challenging to assess the information’s credibility on social media. Similarly, Qian et al. ( 2018 ) assert that fake news and fabricated content propagate exponentially at the early stage of its creation and can cause a significant loss in a short amount of time (Friggeri et al. 2014 ) including manipulating the outcome of political events (Liu and Wu 2018 ; Bessi and Ferrara 2016 ).
Moreover, while analyzing the way source and promoters of fake news operate over the web through multiple online platforms, Zannettou et al. ( 2019 ) discovered that false information is more likely to spread across platforms (18% appearing on multiple platforms) compared to real information (11%).
Furthermore, recently, Shu et al. ( 2020c ) attempted to understand the propagation of disinformation and fake news in social media and found that such content is produced and disseminated faster and easier through social media because of the low barriers that prevent doing so. Similarly, Shu et al. ( 2020b ) studied hierarchical propagation networks for fake news detection. They performed a comparative analysis between fake and real news from structural, temporal and linguistic perspectives. They demonstrated the potential of using these features to detect fake news and they showed their effectiveness for fake news detection as well.
Lastly, Abdullah-All-Tanvir et al. ( 2020 ) note that it is almost impossible to manually detect the sources and authenticity of fake news effectively and efficiently, due to its fast circulation in such a small amount of time. Therefore, it is crucial to note that the dynamic nature of the various online social platforms, which results in the continued rapid and exponential propagation of such fake content, remains a major challenge that requires further investigation while defining innovative solutions for fake news detection.
Datasets issue
The existing approaches lack an inclusive dataset with derived multidimensional information to detect fake news characteristics to achieve higher accuracy of machine learning classification model performance (Nyow and Chua 2019 ). These datasets are primarily dedicated to validating the machine learning model and are the ultimate frame of reference to train the model and analyze its performance. Therefore, if a researcher evaluates their model based on an unrepresentative dataset, the validity and the efficiency of the model become questionable when it comes to applying the fake news detection approach in a real-world scenario.
Moreover, several researchers (Shu et al. 2020d ; Wang et al. 2020 ; Pathak and Srihari 2019 ; Przybyla 2020 ) believe that fake news is diverse and dynamic in terms of content, topics, publishing methods and media platforms, and sophisticated linguistic styles geared to emulate true news. Consequently, training machine learning models on such sophisticated content requires large-scale annotated fake news data that are difficult to obtain (Shu et al. 2020d ).
Therefore, datasets are also a great topic to work on to enhance data quality and have better results while defining our solutions. Adversarial learning techniques (e.g., GAN, SeqGAN) can be used to provide machine-generated data that can be used to train deeper models and build robust systems to detect fake examples from the real ones. This approach can be used to counter the lack of datasets and the scarcity of data available to train models.
Fake news detection literature review
Fake news detection in social networks is still in the early stage of development and there are still challenging issues that need further investigation. This has become an emerging research area that is attracting huge attention.
There are various research studies on fake news detection in online social networks. Few of them have focused on the automatic detection of fake news using artificial intelligence techniques. In this section, we review the existing approaches used in automatic fake news detection, as well as the techniques that have been adopted. Then, a critical discussion built on a primary classification scheme based on a specific set of criteria is also emphasized.
Categories of fake news detection
In this section, we give an overview of most of the existing automatic fake news detection solutions adopted in the literature. A recent classification by Sharma et al. ( 2019 ) uses three categories of fake news identification methods. Each category is further divided based on the type of existing methods (i.e., content-based, feedback-based and intervention-based methods). However, a review of the literature for fake news detection in online social networks shows that the existing studies can be classified into broader categories based on two major aspects that most authors inspect and make use of to define an adequate solution. These aspects can be considered as major sources of extracted information used for fake news detection and can be summarized as follows: the content-based (i.e., related to the content of the news post) and the contextual aspect (i.e., related to the context of the news post).
Consequently, the studies we reviewed can be classified into three different categories based on the two aspects mentioned above (the third category is hybrid). As depicted in Fig. 5 , fake news detection solutions can be categorized as news content-based approaches, the social context-based approaches that can be divided into network and user-based approaches, and hybrid approaches. The latter combines both content-based and contextual approaches to define the solution.

Classification of fake news detection approaches
News Content-based Category
News content-based approaches are fake news detection approaches that use content information (i.e., information extracted from the content of the news post) and that focus on studying and exploiting the news content in their proposed solutions. Content refers to the body of the news, including source, headline, text and image-video, which can reflect subtle differences.
Researchers of this category rely on content-based detection cues (i.e., text and multimedia-based cues), which are features extracted from the content of the news post. Text-based cues are features extracted from the text of the news, whereas multimedia-based cues are features extracted from the images and videos attached to the news. Figure 6 summarizes the most widely used news content representation (i.e., text and multimedia/images) and detection techniques (i.e., machine learning (ML), deep Learning (DL), natural language processing (NLP), fact-checking, crowdsourcing (CDS) and blockchain (BKC)) in news content-based category of fake news detection approaches. Most of the reviewed research works based on news content for fake news detection rely on the text-based cues (Kapusta et al. 2019 ; Kaur et al. 2020 ; Vereshchaka et al. 2020 ; Ozbay and Alatas 2020 ; Wang 2017 ; Nyow and Chua 2019 ; Hosseinimotlagh and Papalexakis 2018 ; Abdullah-All-Tanvir et al. 2019 , 2020 ; Mahabub 2020 ; Bahad et al. 2019 ; Hiriyannaiah et al. 2020 ) extracted from the text of the news content including the body of the news and its headline. However, a few researchers such as Vishwakarma et al. ( 2019 ) and Amri et al. ( 2022 ) try to recognize text from the associated image.

News content-based category: news content representation and detection techniques
Most researchers of this category rely on artificial intelligence (AI) techniques (such as ML, DL and NLP models) to improve performance in terms of prediction accuracy. Others use different techniques such as fact-checking, crowdsourcing and blockchain. Specifically, the AI- and ML-based approaches in this category are trying to extract features from the news content, which they use later for content analysis and training tasks. In this particular case, the extracted features are the different types of information considered to be relevant for the analysis. Feature extraction is considered as one of the best techniques to reduce data size in automatic fake news detection. This technique aims to choose a subset of features from the original set to improve classification performance (Yazdi et al. 2020 ).
Table 6 lists the distinct features and metadata, as well as the used datasets in the news content-based category of fake news detection approaches.
The features and datasets used in the news content-based approaches
Feature and metadata | Datasets | Reference |
---|---|---|
The average number of words in sentences, number of stop words, the sentiment rate of the news measured through the difference between the number of positive and negative words in the article | Getting real about fake news , Gathering mediabiasfactcheck , KaiDMML FakeNewsNet , Real news for Oct-Dec 2016 | Kapusta et al. ( ) |
The length distribution of the title, body and label of the article | News trends, Kaggle, Reuters | Kaur et al. ( ) |
Sociolinguistic, historical, cultural, ideological and syntactical features attached to particular words, phrases and syntactical constructions | FakeNewsNet | Vereshchaka et al. ( ) |
Term frequency | BuzzFeed political news, Random political news, ISOT fake news | Ozbay and Alatas ( ) |
The statement, speaker, context, label, justification | POLITIFACT, LIAR | Wang ( ) |
Spatial vicinity of each word, spatial/contextual relations between terms, and latent relations between terms and articles | Kaggle fake news dataset | Hosseinimotlagh and Papalexakis ( ) |
Word length, the count of words in a tweeted statement | Twitter dataset, Chile earthquake 2010 datasets | Abdullah-All-Tanvir et al. ( ) |
The number of words that express negative emotions | Twitter dataset | Abdullah-All-Tanvir et al. ( ) |
Labeled data | BuzzFeed , PolitiFact | Mahabub ( ) |
The relationship between the news article headline and article body. The biases of a written news article | Kaggle: real_or_fake , Fake news detection | Bahad et al. ( ) |
Historical data. The topic and sentiment associated with content textual. The subject and context of the text, semantic knowledge of the content | Facebook dataset | Del Vicario et al. ( ) |
The veracity of image text. The credibility of the top 15 Google search results related to the image text | Google images, the Onion, Kaggle | Vishwakarma et al. ( ) |
Topic modeling of text and the associated image of the online news | Twitter dataset , Weibo | Amri et al. ( ) |
a https://www.kaggle.com/anthonyc1/gathering-real-news-for-oct-dec-2016 , last access date: 26-12-2022
b https://mediabiasfactcheck.com/ , last access date: 26-12-2022
c https://github.com/KaiDMML/FakeNewsNet , last access date: 26-12-2022
d https://www.kaggle.com/anthonyc1/gathering-real-news-for-oct-dec-2016 , last access date: 26-12-2022
e https://www.cs.ucsb.edu/~william/data/liar_dataset.zip , last access date: 26-12-2022
f https://www.kaggle.com/mrisdal/fake-news , last access date: 26-12-2022
g https://github.com/BuzzFeedNews/2016-10-facebook-fact-check , last access date: 26-12-2022
h https://www.politifact.com/subjects/fake-news/ , last access date: 26-12-2022
i https://www.kaggle.com/rchitic17/real-or-fake , last access date: 26-12-2022
j https://www.kaggle.com/jruvika/fake-news-detection , last access date: 26-12-2022
k https://github.com/MKLab-ITI/image-verification-corpus , last access date: 26-12-2022
l https://drive.google.com/file/d/14VQ7EWPiFeGzxp3XC2DeEHi-BEisDINn/view , last access date: 26-12-2022
Social Context-based Category
Unlike news content-based solutions, the social context-based approaches capture the skeptical social context of the online news (Zhang and Ghorbani 2020 ) rather than focusing on the news content. The social context-based category contains fake news detection approaches that use the contextual aspects (i.e., information related to the context of the news post). These aspects are based on social context and they offer additional information to help detect fake news. They are the surrounding data outside of the fake news article itself, where they can be an essential part of automatic fake news detection. Some useful examples of contextual information may include checking if the news itself and the source that published it are credible, checking the date of the news or the supporting resources, and checking if any other online news platforms are reporting the same or similar stories (Zhang and Ghorbani 2020 ).
Social context-based aspects can be classified into two subcategories, user-based and network-based, and they can be used for context analysis and training tasks in the case of AI- and ML-based approaches. User-based aspects refer to information captured from OSN users such as user profile information (Shu et al. 2019b ; Wang et al. 2019c ; Hamdi et al. 2020 ; Nyow and Chua 2019 ; Jiang et al. 2019 ) and user behavior (Cardaioli et al. 2020 ) such as user engagement (Uppada et al. 2022 ; Jiang et al. 2019 ; Shu et al. 2018b ; Nyow and Chua 2019 ) and response (Zhang et al. 2019a ; Qian et al. 2018 ). Meanwhile, network-based aspects refer to information captured from the properties of the social network where the fake content is shared and disseminated such as news propagation path (Liu and Wu 2018 ; Wu and Liu 2018 ) (e.g., propagation times and temporal characteristics of propagation), diffusion patterns (Shu et al. 2019a ) (e.g., number of retweets, shares), as well as user relationships (Mishra 2020 ; Hamdi et al. 2020 ; Jiang et al. 2019 ) (e.g., friendship status among users).
Figure 7 summarizes some of the most widely adopted social context representations, as well as the most used detection techniques (i.e., AI, ML, DL, fact-checking and blockchain), in the social context-based category of approaches.

Social context-based category: social context representation and detection techniques
Table 7 lists the distinct features and metadata, the adopted detection cues, as well as the used datasets, in the context-based category of fake news detection approaches.
The features, detection cues and datasets used int the social context-based approaches
Feature and metadata | Detection cues | Datasets | Reference |
---|---|---|---|
Users’ sharing behaviors, explicit and implicit profile features | User-based: user profile information | FakeNewsNet | Shu et al. ( ) |
Users’ trust level, explicit and implicit profile features of “experienced” users who can recognize fake news items as false and “naive” users who are more likely to believe fake news | User-based: user engagement | FakeNewsNet, BuzzFeed, PolitiFact | Shu et al. ( ) |
Users’ replies on fake content, the reply stances | User-based: user response | RumourEval, PHEME | Zhang et al. ( ) |
Historical user responses to previous articles | User-based: user response | Weibo, Twitter dataset | Qian et al. ( ) |
Speaker name, job title, political party affiliation, etc. | User-based: user profile information | LIAR | Wang et al. ( ) |
Latent relationships among users, the influence of the users with high prestige on the other users | Networks-based: user relationships | Twitter15 and Twitter16 | Mishra ( ) |
The inherent tri-relationships among publishers, news items and users (i.e., publisher-news relations and user-news interactions) | Networks-based: diffusion patterns | FakeNewsNet | Shu et al. ( ) |
Propagation paths of news stories constructed from the retweets of source tweets | Networks-based: news propagation path | Weibo, Twitter15, Twitter16 | Liu and Wu ( ) |
The propagation of messages in a social network | Networks-based: news propagation path | Twitter dataset | Wu and Liu ( ) |
Spatiotemporal information (i.e., location, timestamps of user engagements), user’s Twitter profile, the user engagement to both fake and real news | User-based: user engagement | FakeNewsNet, PolitiFact, GossipCop, Twitter | Nyow and Chua ( ) |
The credibility of information sources, characteristics of the user, and their social graph | User and network-based: user profile information and user relationships | Ego-Twitter | Hamdi et al. ( ) |
Number of follows and followers on social media (user followee/follower, The friendship network), users’ similarities | User and network-based: user profile information, user engagement and user relationships | FakeNewsNet | Jiang et al. ( ) |
a https://www.dropbox.com/s/7ewzdrbelpmrnxu/rumdetect2017.zip , last access date: 26-12-2022 b https://snap.stanford.edu/data/ego-Twitter.html , last access date: 26-12-2022
Hybrid approaches
Most researchers are focusing on employing a specific method rather than a combination of both content- and context-based methods. This is because some of them (Wu and Rao 2020 ) believe that there still some challenging limitations in the traditional fusion strategies due to existing feature correlations and semantic conflicts. For this reason, some researchers focus on extracting content-based information, while others are capturing some social context-based information for their proposed approaches.
However, it has proven challenging to successfully automate fake news detection based on just a single type of feature (Ruchansky et al. 2017 ). Therefore, recent directions tend to do a mixture by using both news content-based and social context-based approaches for fake news detection.
Table 8 lists the distinct features and metadata, as well as the used datasets, in the hybrid category of fake news detection approaches.
The features and datasets used in the hybrid approaches
Feature and metadata | Datasets | Reference |
---|---|---|
Features and textual metadata of the news content: title, content, date, source, location | SOT fake news dataset, LIAR dataset and FA-KES dataset | Elhadad et al. ( ) |
Spatiotemporal information (i.e., location, timestamps of user engagements), user’s Twitter profile, the user engagement to both fake and real news | FakeNewsNet, PolitiFact, GossipCop, Twitter | Nyow and Chua ( ) |
The domains and reputations of the news publishers. The important terms of each news and their word embeddings and topics. Shares, reactions and comments | BuzzFeed | Xu et al. ( ) |
Shares and propagation path of the tweeted content. A set of metrics comprising of created discussions such as the increase in authors, attention level, burstiness level, contribution sparseness, author interaction, author count and the average length of discussions | Twitter dataset | Aswani et al. ( ) |
Features extracted from the evolution of news and features from the users involved in the news spreading: The news veracity, the credibility of news spreaders, and the frequency of exposure to the same piece of news | Twitter dataset | Previti et al. ( ) |
Similar semantics and conflicting semantics between posts and comments | RumourEval, PHEME | Wu and Rao ( ) |
Information from the publisher, including semantic and emotional information in news content. Semantic and emotional information from users. The resultant latent representations from news content and user comments | Guo et al. ( ) | |
Relationships between news articles, creators and subjects | PolitiFact | Zhang et al. ( ) |
Source domains of the news article, author names | George McIntire fake news dataset | Deepak and Chitturi ( ) |
The news content, social context and spatiotemporal information. Synthetic user engagements generated from historical temporal user engagement patterns | FakeNewsNet | Shu et al. ( ) |
The news content, social reactions, statements, the content and language of posts, the sharing and dissemination among users, content similarity, stance, sentiment score, headline, named entity, news sharing, credibility history, tweet comments | SHPT, PolitiFact | Wang et al. ( ) |
The source of the news, its headline, its author, its publication time, the adherence of a news source to a particular party, likes, shares, replies, followers-followees and their activities | NELA-GT-2019, Fakeddit | Raza and Ding ( ) |
Fake news detection techniques
Another vision for classifying automatic fake news detection is to look at techniques used in the literature. Hence, we classify the detection methods based on the techniques into three groups:
- Human-based techniques: This category mainly includes the use of crowdsourcing and fact-checking techniques, which rely on human knowledge to check and validate the veracity of news content.
- Artificial Intelligence-based techniques: This category includes the most used AI approaches for fake news detection in the literature. Specifically, these are the approaches in which researchers use classical ML, deep learning techniques such as convolutional neural network (CNN), recurrent neural network (RNN), as well as natural language processing (NLP).
- Blockchain-based techniques: This category includes solutions using blockchain technology to detect and mitigate fake news in social media by checking source reliability and establishing the traceability of the news content.
Human-based Techniques
One specific research direction for fake news detection consists of using human-based techniques such as crowdsourcing (Pennycook and Rand 2019 ; Micallef et al. 2020 ) and fact-checking (Vlachos and Riedel 2014 ; Chung and Kim 2021 ; Nyhan et al. 2020 ) techniques.
These approaches can be considered as low computational requirement techniques since both rely on human knowledge and expertise for fake news detection. However, fake news identification cannot be addressed solely through human force since it demands a lot of effort in terms of time and cost, and it is ineffective in terms of preventing the fast spread of fake content.
Crowdsourcing. Crowdsourcing approaches (Kim et al. 2018 ) are based on the “wisdom of the crowds” (Collins et al. 2020 ) for fake content detection. These approaches rely on the collective contributions and crowd signals (Tschiatschek et al. 2018 ) of a group of people for the aggregation of crowd intelligence to detect fake news (Tchakounté et al. 2020 ) and to reduce the spread of misinformation on social media (Pennycook and Rand 2019 ; Micallef et al. 2020 ).
Micallef et al. ( 2020 ) highlight the role of the crowd in countering misinformation. They suspect that concerned citizens (i.e., the crowd), who use platforms where disinformation appears, can play a crucial role in spreading fact-checking information and in combating the spread of misinformation.
Recently Tchakounté et al. ( 2020 ) proposed a voting system as a new method of binary aggregation of opinions of the crowd and the knowledge of a third-party expert. The aggregator is based on majority voting on the crowd side and weighted averaging on the third-party site.
Similarly, Huffaker et al. ( 2020 ) propose a crowdsourced detection of emotionally manipulative language. They introduce an approach that transforms classification problems into a comparison task to mitigate conflation content by allowing the crowd to detect text that uses manipulative emotional language to sway users toward positions or actions. The proposed system leverages anchor comparison to distinguish between intrinsically emotional content and emotionally manipulative language.
La Barbera et al. ( 2020 ) try to understand how people perceive the truthfulness of information presented to them. They collect data from US-based crowd workers, build a dataset of crowdsourced truthfulness judgments for political statements, and compare it with expert annotation data generated by fact-checkers such as PolitiFact.
Coscia and Rossi ( 2020 ) introduce a crowdsourced flagging system that consists of online news flagging. The bipolar model of news flagging attempts to capture the main ingredients that they observe in empirical research on fake news and disinformation.
Unlike the previously mentioned researchers who focus on news content in their approaches, Pennycook and Rand ( 2019 ) focus on using crowdsourced judgments of the quality of news sources to combat social media disinformation.
Fact-Checking. The fact-checking task is commonly manually performed by journalists to verify the truthfulness of a given claim. Indeed, fact-checking features are being adopted by multiple online social network platforms. For instance, Facebook 34 started addressing false information through independent fact-checkers in 2017, followed by Google 35 the same year. Two years later, Instagram 36 followed suit. However, the usefulness of fact-checking initiatives is questioned by journalists 37 , as well as by researchers such as Andersen and Søe ( 2020 ). On the other hand, work is being conducted to boost the effectiveness of these initiatives to reduce misinformation (Chung and Kim 2021 ; Clayton et al. 2020 ; Nyhan et al. 2020 ).
Most researchers use fact-checking websites (e.g., politifact.com, 38 snopes.com, 39 Reuters, 40 , etc.) as data sources to build their datasets and train their models. Therefore, in the following, we specifically review examples of solutions that use fact-checking (Vlachos and Riedel 2014 ) to help build datasets that can be further used in the automatic detection of fake content.
Yang et al. ( 2019a ) use PolitiFact fact-checking website as a data source to train, tune, and evaluate their model named XFake, on political data. The XFake system is an explainable fake news detector that assists end users to identify news credibility. The fakeness of news items is detected and interpreted considering both content and contextual (e.g., statements) information (e.g., speaker).
Based on the idea that fact-checkers cannot clean all data, and it must be a selection of what “matters the most” to clean while checking a claim, Sintos et al. ( 2019 ) propose a solution to help fact-checkers combat problems related to data quality (where inaccurate data lead to incorrect conclusions) and data phishing. The proposed solution is a combination of data cleaning and perturbation analysis to avoid uncertainties and errors in data and the possibility that data can be phished.
Tchechmedjiev et al. ( 2019 ) propose a system named “ClaimsKG” as a knowledge graph of fact-checked claims aiming to facilitate structured queries about their truth values, authors, dates, journalistic reviews and other kinds of metadata. “ClaimsKG” designs the relationship between vocabularies. To gather vocabularies, a semi-automated pipeline periodically gathers data from popular fact-checking websites regularly.
AI-based Techniques
Previous work by Yaqub et al. ( 2020 ) has shown that people lack trust in automated solutions for fake news detection However, work is already being undertaken to increase this trust, for instance by von der Weth et al. ( 2020 ).
Most researchers consider fake news detection as a classification problem and use artificial intelligence techniques, as shown in Fig. 8 . The adopted AI techniques may include machine learning ML (e.g., Naïve Bayes, logistic regression, support vector machine SVM), deep learning DL (e.g., convolutional neural networks CNN, recurrent neural networks RNN, long short-term memory LSTM) and natural language processing NLP (e.g., Count vectorizer, TF-IDF Vectorizer). Most of them combine many AI techniques in their solutions rather than relying on one specific approach.

Examples of the most widely used AI techniques for fake news detection
Many researchers are developing machine learning models in their solutions for fake news detection. Recently, deep neural network techniques are also being employed as they are generating promising results (Islam et al. 2020 ). A neural network is a massively parallel distributed processor with simple units that can store important information and make it available for use (Hiriyannaiah et al. 2020 ). Moreover, it has been proven (Cardoso Durier da Silva et al. 2019 ) that the most widely used method for automatic detection of fake news is not simply a classical machine learning technique, but rather a fusion of classical techniques coordinated by a neural network.
Some researchers define purely machine learning models (Del Vicario et al. 2019 ; Elhadad et al. 2019 ; Aswani et al. 2017 ; Hakak et al. 2021 ; Singh et al. 2021 ) in their fake news detection approaches. The more commonly used machine learning algorithms (Abdullah-All-Tanvir et al. 2019 ) for classification problems are Naïve Bayes, logistic regression and SVM.
Other researchers (Wang et al. 2019c ; Wang 2017 ; Liu and Wu 2018 ; Mishra 2020 ; Qian et al. 2018 ; Zhang et al. 2020 ; Goldani et al. 2021 ) prefer to do a mixture of different deep learning models, without combining them with classical machine learning techniques. Some even prove that deep learning techniques outperform traditional machine learning techniques (Mishra et al. 2022 ). Deep learning is one of the most widely popular research topics in machine learning. Unlike traditional machine learning approaches, which are based on manually crafted features, deep learning approaches can learn hidden representations from simpler inputs both in context and content variations (Bondielli and Marcelloni 2019 ). Moreover, traditional machine learning algorithms almost always require structured data and are designed to “learn” to act by understanding labeled data and then use it to produce new results with more datasets, which requires human intervention to “teach them” when the result is incorrect (Parrish 2018 ), while deep learning networks rely on layers of artificial neural networks (ANN) and do not require human intervention, as multilevel layers in neural networks place data in a hierarchy of different concepts, which ultimately learn from their own mistakes (Parrish 2018 ). The two most widely implemented paradigms in deep neural networks are recurrent neural networks (RNN) and convolutional neural networks (CNN).
Still other researchers (Abdullah-All-Tanvir et al. 2019 ; Kaliyar et al. 2020 ; Zhang et al. 2019a ; Deepak and Chitturi 2020 ; Shu et al. 2018a ; Wang et al. 2019c ) prefer to combine traditional machine learning and deep learning classification, models. Others combine machine learning and natural language processing techniques. A few combine deep learning models with natural language processing (Vereshchaka et al. 2020 ). Some other researchers (Kapusta et al. 2019 ; Ozbay and Alatas 2020 ; Ahmed et al. 2020 ) combine natural language processing with machine learning models. Furthermore, others (Abdullah-All-Tanvir et al. 2019 ; Kaur et al. 2020 ; Kaliyar 2018 ; Abdullah-All-Tanvir et al. 2020 ; Bahad et al. 2019 ) prefer to combine all the previously mentioned techniques (i.e., ML, DL and NLP) in their approaches.
Table 11 , which is relegated to the Appendix (after the bibliography) because of its size, shows a comparison of the fake news detection solutions that we have reviewed based on their main approaches, the methodology that was used and the models.
Comparison of AI-based fake news detection techniques
Reference | Approach | Method | Model |
---|---|---|---|
Del Vicario et al. ( ) | An approach to analyze the sentiment associated with data textual content and add semantic knowledge to it | ML | Linear Regression (LIN), Logistic Regression (LOG), Support Vector Machine (SVM) with Linear Kernel, K-Nearest Neighbors (KNN), Neural Network Models (NN), Decision Trees (DT) |
Elhadad et al. ( ) | An approach to select hybrid features from the textual content of the news, which they consider as blocks, without segmenting text into parts (title, content, date, source, etc.) | ML | Decision Tree, KNN, Logistic Regression, SVM, Naïve Bayes with n-gram, LSVM, Perceptron |
Aswani et al. ( ) | A hybrid artificial bee colony approach to identify and segregate buzz in Twitter and analyze user-generated content (UGC) to mine useful information (content buzz/popularity) | ML | KNN with artificial bee colony optimization |
Hakak et al. ( ) | An ensemble of machine learning approaches for effective feature extraction to classify fake news | ML | Decision Tree, Random Forest and Extra Tree Classifier |
Singh et al. ( ) | A multimodal approach, combining text and visual analysis of online news stories to automatically detect fake news through predictive analysis to detect features most strongly associated with fake news | ML | Logistic Regression, Linear Discrimination Analysis, Quadratic Discriminant Analysis, K-Nearest Neighbors, Naïve Bayes, Support Vector Machine, Classification and Regression Tree, and Random Forest Analysis |
Amri et al. ( ) | An explainable multimodal content-based fake news detection system | ML | Vision-and-Language BERT (VilBERT), Local Interpretable Model-Agnostic Explanations (LIME), Latent Dirichlet Allocation (LDA) topic modeling |
Wang et al. ( ) | A hybrid deep neural network model to learn the useful features from contextual information and to capture the dependencies between sequences of contextual information | DL | Recurrent and Convolutional Neural Networks (RNN and CNN) |
Wang ( ) | A hybrid convolutional neural network approach for automatic fake news detection | DL | Recurrent and Convolutional Neural Networks (RNN and CNN) |
Liu and Wu ( ) | An early detection approach of fake news to classify the propagation path to mine the global and local changes of user characteristics in the diffusion path | DL | Recurrent and Convolutional Neural Networks (RNN and CNN) |
Mishra ( ) | Unsupervised network representation learning methods to learn user (node) embeddings from both the follower network and the retweet network and to encode the propagation path sequence | DL | RNN: (long short-term memory unit (LSTM)) |
Qian et al. ( ) | A Two-Level Convolutional Neural Network with User Response Generator (TCNN-URG) where TCNN captures semantic information from the article text by representing it at the sentence and word level. The URG learns a generative model of user responses to article text from historical user responses that it can use to generate responses to new articles to assist fake news detection | DL | Convolutional Neural Network (CNN) |
Zhang et al. ( ) | Based on a set of explicit features extracted from the textual information, a deep diffusive network model is built to infer the credibility of news articles, creators and subjects simultaneously | DL | Deep Diffusive Network Model Learning |
Goldani et al. ( ) | A capsule networks (CapsNet) approach for fake news detection using two architectures for different lengths of news statements and claims that capsule neural networks have been successful in computer vision and are receiving attention for use in Natural Language Processing (NLP) | DL | Capsule Networks (CapsNet) |
Wang et al. ( ) | An automated approach to distinguish different cases of fake news (i.e., hoaxes, irony and propaganda) while assessing and classifying news articles and claims including linguistic cues as well as user credibility and news dissemination in social media | DL, ML | Convolutional Neural Network (CNN), long Short-Term Memory (LSTM), logistic regression |
Abdullah-All-Tanvir et al. ( ) | A model to recognize forged news messages from twitter posts, by figuring out how to anticipate precision appraisals, in view of computerizing forged news identification in Twitter dataset. A combination of traditional machine learning, as well as deep learning classification models, is tested to enhance the accuracy of prediction | DL, ML | Naïve Bayes, Logistic Regression, Support Vector Machine, Recurrent Neural Network (RNN), Long Short-Term Memory (LSTM) |
Kaliyar et al. ( ) | An approach named (FNDNet) based on the combination between unsupervised learning algorithm GloVe and deep convolutional neural network for fake news detection | DL, ML | Deep Convolutional Neural Network (CNN), Global Vectors (GloVe) |
Zhang et al. ( ) | A hybrid approach to encode auxiliary information coming from people’s replies alone in temporal order. Such auxiliary information is then used to update a priori belief generating a posteriori belief | DL, ML | Deep Learning Model, Long Short-Term Memory Neural Network (LSTM) |
Deepak and Chitturi ( ) | A system that consists of live data mining in addition to the deep learning model | DL, ML | Feedforward Neural Network (FNN) and LSTM Word Vector Model |
Shu et al. ( ) | A multidimensional fake news data repository “FakeNewsNet” and conduct an exploratory analysis of the datasets to evaluate them | DL, ML | Convolutional Neural Network (CNN), Support Vector Machines (SVMs), Logistic Regression (LR), Naïve Bayes (NB) |
Vereshchaka et al. ( ) | A sociocultural textual analysis, computational linguistics analysis, and textual classification using NLP, as well as deep learning models to distinguish fake from real news to mitigate the problem of disinformation | DL, NLP | Short-Term Memory (LSTM), Recurrent Neural Network (RNN) and Gated Recurrent Unit (GRU) |
Kapusta et al. ( ) | A sentiment and frequency analysis using both machine learning and NLP in what is called text mining to processing news content sentiment analysis and frequency analysis to compare basic text characteristics of fake and real news articles | ML, NLP | The Natural Language Toolkit (NLTK), TF-IDF |
Ozbay and Alatas ( ) | A hybrid approach based on text analysis and supervised artificial intelligence for fake news detection | ML, NLP | Supervised algorithms: BayesNet, JRip, OneR, Decision Stump, ZeroR, Stochastic Gradient Descent (SGD), CV Parameter Selection (CVPS), Randomizable Filtered Classifier (RFC), Logistic Model Tree (LMT). NLP: TF weighting |
Ahmed et al. ( ) | A machine learning and NLP text-based processing to identify fake news. Various features of the text are extracted through text processing and after that those features are incorporated into classification | ML, NLP | Machine learning classifiers (i.e., Passive-aggressive, Naïve Bayes and Support Vector Machine) |
Abdullah-All-Tanvir et al. ( ) | A hybrid neural network approach to identify authentic news on popular Twitter threads which would outperform the traditional neural network architecture’s performance. Three traditional supervised algorithms and two Deep Neural are combined to train the defined model. Some NLP concepts were also used to implement some of the traditional supervised machine learning algorithms over their dataset | ML, DL, NLP | Traditional supervised algorithm (i.e., Logistic Regression, Bayesian Classifier and Support Vector Machine). Deep Neural Networks (i.e., Recurrent Neural Network, Long Short-Term Memory LSTM). NLP concepts such as Count vectorizer and TF-IDF Vectorizer |
Kaur et al. ( ) | A hybrid method to identify news articles as fake or real through finding out which classification model identifies false features accurately | ML, DL, NLP | Neural Networks (NN) and Ensemble Models. Supervised Machine Learning Classifiers such as Naïve Bayes (NB), Decision Tree (DT), Support Vector Machine (SVM), Linear Models. Term Frequency-Inverse Document Frequency (TF-IDF), Count-Vectorizer (CV), Hashing-Vectorizer (HV) |
Kaliyar ( ) | A fake news detection approach to classify the news article or other documents into certain or not. Natural language processing, machine learning and deep learning techniques are used to implement the defined models and to predict the accuracy of different models and classifiers | ML, DL, NLP | Machine Learning Models: Naïve Bayes, K-nearest Neighbors, Decision Tree, Random Forest. Deep Learning Networks: Shallow Convolutional Neural Networks (CNN), Very Deep Convolutional Neural Network (VDCNN), Long Short-Term Memory Network (LSTM), Gated Recurrent Unit Network (GRU). A combination of Convolutional Neural Network with Long Short-Term Memory (CNN-LSTM) and Convolutional Neural Network with Gated Recurrent Unit (CNN-LSTM) |
Mahabub ( ) | An intelligent detection system to manage the classification of news as being either real or fake | ML, DL, NLP | Machine Learning: Naïve Bayes, KNN, SVM, Random Forest, Artificial Neural Network, Logistic Regression, Gradient Boosting, AdaBoost |
Bahad et al. ( ) | A method based on Bi-directional LSTM-recurrent neural network to analyze the relationship between the news article headline and article body | ML, DL, NLP | Unsupervised Learning algorithm: Global Vectors (GloVe). Bi-directional LSTM-recurrent Neural Network |
Blockchain-based Techniques for Source Reliability and Traceability
Another research direction for detecting and mitigating fake news in social media focuses on using blockchain solutions. Blockchain technology is recently attracting researchers’ attention due to the interesting features it offers. Immutability, decentralization, tamperproof, consensus, record keeping and non-repudiation of transactions are some of the key features that make blockchain technology exploitable, not just for cryptocurrencies, but also to prove the authenticity and integrity of digital assets.
However, the proposed blockchain approaches are few in number and they are fundamental and theoretical approaches. Specifically, the solutions that are currently available are still in research, prototype, and beta testing stages (DiCicco and Agarwal 2020 ; Tchechmedjiev et al. 2019 ). Furthermore, most researchers (Ochoa et al. 2019 ; Song et al. 2019 ; Shang et al. 2018 ; Qayyum et al. 2019 ; Jing and Murugesan 2018 ; Buccafurri et al. 2017 ; Chen et al. 2018 ) do not specify which fake news type they are mitigating in their studies. They mention news content in general, which is not adequate for innovative solutions. For that, serious implementations should be provided to prove the usefulness and feasibility of this newly developing research vision.
Table 9 shows a classification of the reviewed blockchain-based approaches. In the classification, we listed the following:
- The type of fake news that authors are trying to mitigate, which can be multimedia-based or text-based fake news.
- The techniques used for fake news mitigation, which can be either blockchain only, or blockchain combined with other techniques such as AI, Data mining, Truth-discovery, Preservation metadata, Semantic similarity, Crowdsourcing, Graph theory and SIR model (Susceptible, Infected, Recovered).
- The feature that is offered as an advantage of the given solution (e.g., Reliability, Authenticity and Traceability). Reliability is the credibility and truthfulness of the news content, which consists of proving the trustworthiness of the content. Traceability aims to trace and archive the contents. Authenticity consists of checking whether the content is real and authentic.
A checkmark ( ✓ ) in Table 9 denotes that the mentioned criterion is explicitly mentioned in the proposed solution, while the empty dash (–) cell for fake news type denotes that it depends on the case: The criterion was either not explicitly mentioned (e.g., fake news type) in the work or the classification does not apply (e.g., techniques/other).
A classification of popular blockchain-based approaches for fake news detection in social media
Reference | Fake News Type | Techniques | Feature | |
---|---|---|---|---|
Multimedia | Text | |||
Shae and Tsai ( ) | AI | Reliability | ||
Ochoa et al. ( ) | – | Data Mining, Truth-Discovery | Reliability | |
Huckle and White ( ) | – | Preservation Metadata | Reliability | |
Song et al. ( ) | – | – | – | Traceability |
Shang et al. ( ) | – | – | – | Traceability |
Qayyum et al. ( ) | – | – | Semantic Similarity | Reliability |
Jing and Murugesan ( ) | – | – | AI | Reliability |
Buccafurri et al. ( ) | – | – | Crowd-Sourcing | Reliability |
Chen et al. ( ) | – | – | SIR Model | Reliability |
Hasan and Salah ( ) | – | – | Authenticity | |
Tchechmedjiev et al. ( ) | – | – | Graph theory | Reliability |
After reviewing the most relevant state of the art for automatic fake news detection, we classify them as shown in Table 10 based on the detection aspects (i.e., content-based, contextual, or hybrid aspects) and the techniques used (i.e., AI, crowdsourcing, fact-checking, blockchain or hybrid techniques). Hybrid techniques refer to solutions that simultaneously combine different techniques from previously mentioned categories (i.e., inter-hybrid methods), as well as techniques within the same class of methods (i.e., intra-hybrid methods), in order to define innovative solutions for fake news detection. A hybrid method should bring the best of both worlds. Then, we provide a discussion based on different axes.
Fake news detection approaches classification
Artificial Intelligence | Crowdsourcing (CDS) | Blockchain (BKC) | Fact-checking | Hybrid | |||
---|---|---|---|---|---|---|---|
ML | DL | NLP | |||||
Content | Del Vicario et al. ( ), Hosseinimotlagh and Papalexakis ( ), Hakak et al. ( ), Singh et al. ( ), Amri et al. ( ) | Wang ( ), Hiriyannaiah et al. ( ) | Zellers et al. ( ) | Kim et al. ( ), Tschiatschek et al. ( ), Tchakounté et al. ( ), Huffaker et al. ( ), La Barbera et al. ( ), Coscia and Rossi ( ), Micallef et al. ( ) | Song et al. ( ) | Sintos et al. ( ) | ML, DL, NLP: Abdullah-All-Tanvir et al. ( ), Kaur et al. ( ), Mahabub ( ), Bahad et al. ( ) Kaliyar ( ) |
ML, DL: | |||||||
Abdullah-All-Tanvir et al. ( ), Kaliyar et al. ( ), Deepak and Chitturi ( ) | |||||||
DL, NLP: Vereshchaka et al. ( ) | |||||||
ML, NLP: Kapusta et al. ( ), Ozbay and Alatas Ozbay and Alatas ( ), Ahmed et al. ( ) | |||||||
BKC, CDS: Buccafurri et al. ( ) | |||||||
Context | – | Qian et al. ( ), Liu and Wu ( ), Hamdi et al. ( ), Wang et al. ( ), Mishra ( ) | – | Pennycook and Rand ( ) | Huckle and White ( ), Shang et al. ( ) | Tchechmedjiev et al. ( ) | ML, DL: Zhang et al. ( ), Shu et al. ( ), Shu et al. ( ), Wu and Liu ( ) |
BKC, AI: Ochoa et al. ( ) | |||||||
BKC, SIR: Chen et al. ( ) | |||||||
Hybrid | Aswani et al. ( ), Previti et al. ( ), Elhadad et al. ( ), Nyow and Chua ( ) | Ruchansky et al. ( ), Wu and Rao ( ), Guo et al. ( ), Zhang et al. ( ) | Xu et al. ( ) | – | Qayyum et al. ( ), Hasan and Salah ( ), Tchechmedjiev et al. ( ) | Yang et al. ( ) | ML, DL: Shu et al. ( ), Wang et al. ( ) |
BKC, AI: Shae and Tsai ( ), Jing and Murugesan ( ) |
News content-based methods
Most of the news content-based approaches consider fake news detection as a classification problem and they use AI techniques such as classical machine learning (e.g., regression, Bayesian) as well as deep learning (i.e., neural methods such as CNN and RNN). More specifically, classification of social media content is a fundamental task for social media mining, so that most existing methods regard it as a text categorization problem and mainly focus on using content features, such as words and hashtags (Wu and Liu 2018 ). The main challenge facing these approaches is how to extract features in a way to reduce the data used to train their models and what features are the most suitable for accurate results.
Researchers using such approaches are motivated by the fact that the news content is the main entity in the deception process, and it is a straightforward factor to analyze and use while looking for predictive clues of deception. However, detecting fake news only from the content of the news is not enough because the news is created in a strategic intentional way to mimic the truth (i.e., the content can be intentionally manipulated by the spreader to make it look like real news). Therefore, it is considered to be challenging, if not impossible, to identify useful features (Wu and Liu 2018 ) and consequently tell the nature of such news solely from the content.
Moreover, works that utilize only the news content for fake news detection ignore the rich information and latent user intelligence (Qian et al. 2018 ) stored in user responses toward previously disseminated articles. Therefore, the auxiliary information is deemed crucial for an effective fake news detection approach.
Social context-based methods
The context-based approaches explore the surrounding data outside of the news content, which can be an effective direction and has some advantages in areas where the content approaches based on text classification can run into issues. However, most existing studies implementing contextual methods mainly focus on additional information coming from users and network diffusion patterns. Moreover, from a technical perspective, they are limited to the use of sophisticated machine learning techniques for feature extraction, and they ignore the usefulness of results coming from techniques such as web search and crowdsourcing which may save much time and help in the early detection and identification of fake content.
Hybrid approaches can simultaneously model different aspects of fake news such as the content-based aspects, as well as the contextual aspect based on both the OSN user and the OSN network patterns. However, these approaches are deemed more complex in terms of models (Bondielli and Marcelloni 2019 ), data availability, and the number of features. Furthermore, it remains difficult to decide which information among each category (i.e., content-based and context-based information) is most suitable and appropriate to be used to achieve accurate and precise results. Therefore, there are still very few studies belonging to this category of hybrid approaches.
Early detection
As fake news usually evolves and spreads very fast on social media, it is critical and urgent to consider early detection directions. Yet, this is a challenging task to do especially in highly dynamic platforms such as social networks. Both news content- and social context-based approaches suffer from this challenging early detection of fake news.
Although approaches that detect fake news based on content analysis face this issue less, they are still limited by the lack of information required for verification when the news is in its early stage of spread. However, approaches that detect fake news based on contextual analysis are most likely to suffer from the lack of early detection since most of them rely on information that is mostly available after the spread of fake content such as social engagement, user response, and propagation patterns. Therefore, it is crucial to consider both trusted human verification and historical data as an attempt to detect fake content during its early stage of propagation.
Conclusion and future directions
In this paper, we introduced the general context of the fake news problem as one of the major issues of the online deception problem in online social networks. Based on reviewing the most relevant state of the art, we summarized and classified existing definitions of fake news, as well as its related terms. We also listed various typologies and existing categorizations of fake news such as intent-based fake news including clickbait, hoax, rumor, satire, propaganda, conspiracy theories, framing as well as content-based fake news including text and multimedia-based fake news, and in the latter, we can tackle deepfake videos and GAN-generated fake images. We discussed the major challenges related to fake news detection and mitigation in social media including the deceptiveness nature of the fabricated content, the lack of human awareness in the field of fake news, the non-human spreaders issue (e.g., social bots), the dynamicity of such online platforms, which results in a fast propagation of fake content and the quality of existing datasets, which still limits the efficiency of the proposed solutions. We reviewed existing researchers’ visions regarding the automatic detection of fake news based on the adopted approaches (i.e., news content-based approaches, social context-based approaches, or hybrid approaches) and the techniques that are used (i.e., artificial intelligence-based methods; crowdsourcing, fact-checking, and blockchain-based methods; and hybrid methods), then we showed a comparative study between the reviewed works. We also provided a critical discussion of the reviewed approaches based on different axes such as the adopted aspect for fake news detection (i.e., content-based, contextual, and hybrid aspects) and the early detection perspective.
To conclude, we present the main issues for combating the fake news problem that needs to be further investigated while proposing new detection approaches. We believe that to define an efficient fake news detection approach, we need to consider the following:
- Our choice of sources of information and search criteria may have introduced biases in our research. If so, it would be desirable to identify those biases and mitigate them.
- News content is the fundamental source to find clues to distinguish fake from real content. However, contextual information derived from social media users and from the network can provide useful auxiliary information to increase detection accuracy. Specifically, capturing users’ characteristics and users’ behavior toward shared content can be a key task for fake news detection.
- Moreover, capturing users’ historical behavior, including their emotions and/or opinions toward news content, can help in the early detection and mitigation of fake news.
- Furthermore, adversarial learning techniques (e.g., GAN, SeqGAN) can be considered as a promising direction for mitigating the lack and scarcity of available datasets by providing machine-generated data that can be used to train and build robust systems to detect the fake examples from the real ones.
- Lastly, analyzing how sources and promoters of fake news operate over the web through multiple online platforms is crucial; Zannettou et al. ( 2019 ) discovered that false information is more likely to spread across platforms (18% appearing on multiple platforms) compared to valid information (11%).
Appendix: A Comparison of AI-based fake news detection techniques
This Appendix consists only in the rather long Table 11 . It shows a comparison of the fake news detection solutions based on artificial intelligence that we have reviewed according to their main approaches, the methodology that was used, and the models, as explained in Sect. 6.2.2 .
Author Contributions
The order of authors is alphabetic as is customary in the third author’s field. The lead author was Sabrine Amri, who collected and analyzed the data and wrote a first draft of the paper, all along under the supervision and tight guidance of Esma Aïmeur. Gilles Brassard reviewed, criticized and polished the work into its final form.
This work is supported in part by Canada’s Natural Sciences and Engineering Research Council.
Availability of data and material
Declarations.
On behalf of all authors, the corresponding author states that there is no conflict of interest.
1 https://www.nationalacademies.org/news/2021/07/as-surgeon-general-urges-whole-of-society-effort-to-fight-health-misinformation-the-work-of-the-national-academies-helps-foster-an-evidence-based-information-environment , last access date: 26-12-2022.
2 https://time.com/4897819/elvis-presley-alive-conspiracy-theories/ , last access date: 26-12-2022.
3 https://www.therichest.com/shocking/the-evidence-15-reasons-people-think-the-earth-is-flat/ , last access date: 26-12-2022.
4 https://www.grunge.com/657584/the-truth-about-1952s-alien-invasion-of-washington-dc/ , last access date: 26-12-2022.
5 https://www.journalism.org/2021/01/12/news-use-across-social-media-platforms-in-2020/ , last access date: 26-12-2022.
6 https://www.pewresearch.org/fact-tank/2018/12/10/social-media-outpaces-print-newspapers-in-the-u-s-as-a-news-source/ , last access date: 26-12-2022.
7 https://www.buzzfeednews.com/article/janelytvynenko/coronavirus-fake-news-disinformation-rumors-hoaxes , last access date: 26-12-2022.
8 https://www.factcheck.org/2020/03/viral-social-media-posts-offer-false-coronavirus-tips/ , last access date: 26-12-2022.
9 https://www.factcheck.org/2020/02/fake-coronavirus-cures-part-2-garlic-isnt-a-cure/ , last access date: 26-12-2022.
10 https://www.bbc.com/news/uk-36528256 , last access date: 26-12-2022.
11 https://en.wikipedia.org/wiki/Pizzagate_conspiracy_theory , last access date: 26-12-2022.
12 https://www.theguardian.com/world/2017/jan/09/germany-investigating-spread-fake-news-online-russia-election , last access date: 26-12-2022.
13 https://www.macquariedictionary.com.au/resources/view/word/of/the/year/2016 , last access date: 26-12-2022.
14 https://www.macquariedictionary.com.au/resources/view/word/of/the/year/2018 , last access date: 26-12-2022.
15 https://apnews.com/article/47466c5e260149b1a23641b9e319fda6 , last access date: 26-12-2022.
16 https://blog.collinsdictionary.com/language-lovers/collins-2017-word-of-the-year-shortlist/ , last access date: 26-12-2022.
17 https://www.gartner.com/smarterwithgartner/gartner-top-strategic-predictions-for-2018-and-beyond/ , last access date: 26-12-2022.
18 https://www.technologyreview.com/s/612236/even-the-best-ai-for-spotting-fake-news-is-still-terrible/ , last access date: 26-12-2022.
19 https://scholar.google.ca/ , last access date: 26-12-2022.
20 https://ieeexplore.ieee.org/ , last access date: 26-12-2022.
21 https://link.springer.com/ , last access date: 26-12-2022.
22 https://www.sciencedirect.com/ , last access date: 26-12-2022.
23 https://www.scopus.com/ , last access date: 26-12-2022.
24 https://www.acm.org/digital-library , last access date: 26-12-2022.
25 https://www.politico.com/magazine/story/2016/12/fake-news-history-long-violent-214535 , last access date: 26-12-2022.
26 https://en.wikipedia.org/wiki/Trial_of_Socrates , last access date: 26-12-2022.
27 https://trends.google.com/trends/explore?hl=en-US &tz=-180 &date=2013-12-06+2018-01-06 &geo=US &q=fake+news &sni=3 , last access date: 26-12-2022.
28 https://ec.europa.eu/digital-single-market/en/tackling-online-disinformation , last access date: 26-12-2022.
29 https://www.nato.int/cps/en/natohq/177273.htm , last access date: 26-12-2022.
30 https://www.collinsdictionary.com/dictionary/english/fake-news , last access date: 26-12-2022.
31 https://www.statista.com/statistics/657111/fake-news-sharing-online/ , last access date: 26-12-2022.
32 https://www.statista.com/statistics/657090/fake-news-recogition-confidence/ , last access date: 26-12-2022.
33 https://www.nbcnews.com/tech/social-media/now-available-more-200-000-deleted-russian-troll-tweets-n844731 , last access date: 26-12-2022.
34 https://www.theguardian.com/technology/2017/mar/22/facebook-fact-checking-tool-fake-news , last access date: 26-12-2022.
35 https://www.theguardian.com/technology/2017/apr/07/google-to-display-fact-checking-labels-to-show-if-news-is-true-or-false , last access date: 26-12-2022.
36 https://about.instagram.com/blog/announcements/combatting-misinformation-on-instagram , last access date: 26-12-2022.
37 https://www.wired.com/story/instagram-fact-checks-who-will-do-checking/ , last access date: 26-12-2022.
38 https://www.politifact.com/ , last access date: 26-12-2022.
39 https://www.snopes.com/ , last access date: 26-12-2022.
40 https://www.reutersagency.com/en/ , last access date: 26-12-2022.
Publisher's Note
Springer Nature remains neutral with regard to jurisdictional claims in published maps and institutional affiliations.
Contributor Information
Esma Aïmeur, Email: ac.laertnomu.ori@ruemia .
Sabrine Amri, Email: [email protected] .
Gilles Brassard, Email: ac.laertnomu.ori@drassarb .
- Abdullah-All-Tanvir, Mahir EM, Akhter S, Huq MR (2019) Detecting fake news using machine learning and deep learning algorithms. In: 7th international conference on smart computing and communications (ICSCC), IEEE, pp 1–5 10.1109/ICSCC.2019.8843612
- Abdullah-All-Tanvir, Mahir EM, Huda SMA, Barua S (2020) A hybrid approach for identifying authentic news using deep learning methods on popular Twitter threads. In: International conference on artificial intelligence and signal processing (AISP), IEEE, pp 1–6 10.1109/AISP48273.2020.9073583
- Abu Arqoub O, Abdulateef Elega A, Efe Özad B, Dwikat H, Adedamola Oloyede F. Mapping the scholarship of fake news research: a systematic review. J Pract. 2022; 16 (1):56–86. doi: 10.1080/17512786.2020.1805791. [ CrossRef ] [ Google Scholar ]
- Ahmed S, Hinkelmann K, Corradini F. Development of fake news model using machine learning through natural language processing. Int J Comput Inf Eng. 2020; 14 (12):454–460. [ Google Scholar ]
- Aïmeur E, Brassard G, Rioux J. Data privacy: an end-user perspective. Int J Comput Netw Commun Secur. 2013; 1 (6):237–250. [ Google Scholar ]
- Aïmeur E, Hage H, Amri S (2018) The scourge of online deception in social networks. In: 2018 international conference on computational science and computational intelligence (CSCI), IEEE, pp 1266–1271 10.1109/CSCI46756.2018.00244
- Alemanno A. How to counter fake news? A taxonomy of anti-fake news approaches. Eur J Risk Regul. 2018; 9 (1):1–5. doi: 10.1017/err.2018.12. [ CrossRef ] [ Google Scholar ]
- Allcott H, Gentzkow M. Social media and fake news in the 2016 election. J Econ Perspect. 2017; 31 (2):211–36. doi: 10.1257/jep.31.2.211. [ CrossRef ] [ Google Scholar ]
- Allen J, Howland B, Mobius M, Rothschild D, Watts DJ. Evaluating the fake news problem at the scale of the information ecosystem. Sci Adv. 2020 doi: 10.1126/sciadv.aay3539. [ PMC free article ] [ PubMed ] [ CrossRef ] [ Google Scholar ]
- Allington D, Duffy B, Wessely S, Dhavan N, Rubin J. Health-protective behaviour, social media usage and conspiracy belief during the Covid-19 public health emergency. Psychol Med. 2020 doi: 10.1017/S003329172000224X. [ PMC free article ] [ PubMed ] [ CrossRef ] [ Google Scholar ]
- Alonso-Galbán P, Alemañy-Castilla C (2022) Curbing misinformation and disinformation in the Covid-19 era: a view from cuba. MEDICC Rev 22:45–46 10.37757/MR2020.V22.N2.12 [ PubMed ] [ CrossRef ]
- Altay S, Hacquin AS, Mercier H. Why do so few people share fake news? It hurts their reputation. New Media Soc. 2022; 24 (6):1303–1324. doi: 10.1177/1461444820969893. [ CrossRef ] [ Google Scholar ]
- Amri S, Sallami D, Aïmeur E (2022) Exmulf: an explainable multimodal content-based fake news detection system. In: International symposium on foundations and practice of security. Springer, Berlin, pp 177–187. 10.1109/IJCNN48605.2020.9206973
- Andersen J, Søe SO. Communicative actions we live by: the problem with fact-checking, tagging or flagging fake news-the case of Facebook. Eur J Commun. 2020; 35 (2):126–139. doi: 10.1177/0267323119894489. [ CrossRef ] [ Google Scholar ]
- Apuke OD, Omar B. Fake news and Covid-19: modelling the predictors of fake news sharing among social media users. Telematics Inform. 2021; 56 :101475. doi: 10.1016/j.tele.2020.101475. [ PMC free article ] [ PubMed ] [ CrossRef ] [ Google Scholar ]
- Apuke OD, Omar B, Tunca EA, Gever CV. The effect of visual multimedia instructions against fake news spread: a quasi-experimental study with Nigerian students. J Librariansh Inf Sci. 2022 doi: 10.1177/09610006221096477. [ CrossRef ] [ Google Scholar ]
- Aswani R, Ghrera S, Kar AK, Chandra S. Identifying buzz in social media: a hybrid approach using artificial bee colony and k-nearest neighbors for outlier detection. Soc Netw Anal Min. 2017; 7 (1):1–10. doi: 10.1007/s13278-017-0461-2. [ CrossRef ] [ Google Scholar ]
- Avram M, Micallef N, Patil S, Menczer F (2020) Exposure to social engagement metrics increases vulnerability to misinformation. arXiv preprint arxiv:2005.04682 , 10.37016/mr-2020-033
- Badawy A, Lerman K, Ferrara E (2019) Who falls for online political manipulation? In: Companion proceedings of the 2019 world wide web conference, pp 162–168 10.1145/3308560.3316494
- Bahad P, Saxena P, Kamal R. Fake news detection using bi-directional LSTM-recurrent neural network. Procedia Comput Sci. 2019; 165 :74–82. doi: 10.1016/j.procs.2020.01.072. [ CrossRef ] [ Google Scholar ]
- Bakdash J, Sample C, Rankin M, Kantarcioglu M, Holmes J, Kase S, Zaroukian E, Szymanski B (2018) The future of deception: machine-generated and manipulated images, video, and audio? In: 2018 international workshop on social sensing (SocialSens), IEEE, pp 2–2 10.1109/SocialSens.2018.00009
- Balmas M. When fake news becomes real: combined exposure to multiple news sources and political attitudes of inefficacy, alienation, and cynicism. Commun Res. 2014; 41 (3):430–454. doi: 10.1177/0093650212453600. [ CrossRef ] [ Google Scholar ]
- Baptista JP, Gradim A. Understanding fake news consumption: a review. Soc Sci. 2020 doi: 10.3390/socsci9100185. [ CrossRef ] [ Google Scholar ]
- Baptista JP, Gradim A. A working definition of fake news. Encyclopedia. 2022; 2 (1):632–645. doi: 10.3390/encyclopedia2010043. [ CrossRef ] [ Google Scholar ]
- Bastick Z. Would you notice if fake news changed your behavior? An experiment on the unconscious effects of disinformation. Comput Hum Behav. 2021; 116 :106633. doi: 10.1016/j.chb.2020.106633. [ CrossRef ] [ Google Scholar ]
- Batailler C, Brannon SM, Teas PE, Gawronski B. A signal detection approach to understanding the identification of fake news. Perspect Psychol Sci. 2022; 17 (1):78–98. doi: 10.1177/1745691620986135. [ PubMed ] [ CrossRef ] [ Google Scholar ]
- Bessi A, Ferrara E (2016) Social bots distort the 2016 US presidential election online discussion. First Monday 21(11-7). 10.5210/fm.v21i11.7090
- Bhattacharjee A, Shu K, Gao M, Liu H (2020) Disinformation in the online information ecosystem: detection, mitigation and challenges. arXiv preprint arXiv:2010.09113
- Bhuiyan MM, Zhang AX, Sehat CM, Mitra T. Investigating differences in crowdsourced news credibility assessment: raters, tasks, and expert criteria. Proc ACM Hum Comput Interact. 2020; 4 (CSCW2):1–26. doi: 10.1145/3415164. [ CrossRef ] [ Google Scholar ]
- Bode L, Vraga EK. In related news, that was wrong: the correction of misinformation through related stories functionality in social media. J Commun. 2015; 65 (4):619–638. doi: 10.1111/jcom.12166. [ CrossRef ] [ Google Scholar ]
- Bondielli A, Marcelloni F. A survey on fake news and rumour detection techniques. Inf Sci. 2019; 497 :38–55. doi: 10.1016/j.ins.2019.05.035. [ CrossRef ] [ Google Scholar ]
- Bovet A, Makse HA. Influence of fake news in Twitter during the 2016 US presidential election. Nat Commun. 2019; 10 (1):1–14. doi: 10.1038/s41467-018-07761-2. [ PMC free article ] [ PubMed ] [ CrossRef ] [ Google Scholar ]
- Brashier NM, Pennycook G, Berinsky AJ, Rand DG. Timing matters when correcting fake news. Proc Natl Acad Sci. 2021 doi: 10.1073/pnas.2020043118. [ PMC free article ] [ PubMed ] [ CrossRef ] [ Google Scholar ]
- Brewer PR, Young DG, Morreale M. The impact of real news about “fake news”: intertextual processes and political satire. Int J Public Opin Res. 2013; 25 (3):323–343. doi: 10.1093/ijpor/edt015. [ CrossRef ] [ Google Scholar ]
- Bringula RP, Catacutan-Bangit AE, Garcia MB, Gonzales JPS, Valderama AMC. “Who is gullible to political disinformation?” Predicting susceptibility of university students to fake news. J Inf Technol Polit. 2022; 19 (2):165–179. doi: 10.1080/19331681.2021.1945988. [ CrossRef ] [ Google Scholar ]
- Buccafurri F, Lax G, Nicolazzo S, Nocera A (2017) Tweetchain: an alternative to blockchain for crowd-based applications. In: International conference on web engineering, Springer, Berlin, pp 386–393. 10.1007/978-3-319-60131-1_24
- Burshtein S. The true story on fake news. Intell Prop J. 2017; 29 (3):397–446. [ Google Scholar ]
- Cardaioli M, Cecconello S, Conti M, Pajola L, Turrin F (2020) Fake news spreaders profiling through behavioural analysis. In: CLEF (working notes)
- Cardoso Durier da Silva F, Vieira R, Garcia AC (2019) Can machines learn to detect fake news? A survey focused on social media. In: Proceedings of the 52nd Hawaii international conference on system sciences. 10.24251/HICSS.2019.332
- Carmi E, Yates SJ, Lockley E, Pawluczuk A (2020) Data citizenship: rethinking data literacy in the age of disinformation, misinformation, and malinformation. Intern Policy Rev 9(2):1–22 10.14763/2020.2.1481
- Celliers M, Hattingh M (2020) A systematic review on fake news themes reported in literature. In: Conference on e-Business, e-Services and e-Society. Springer, Berlin, pp 223–234. 10.1007/978-3-030-45002-1_19
- Chen Y, Li Q, Wang H (2018) Towards trusted social networks with blockchain technology. arXiv preprint arXiv:1801.02796
- Cheng L, Guo R, Shu K, Liu H (2020) Towards causal understanding of fake news dissemination. arXiv preprint arXiv:2010.10580
- Chiu MM, Oh YW. How fake news differs from personal lies. Am Behav Sci. 2021; 65 (2):243–258. doi: 10.1177/0002764220910243. [ CrossRef ] [ Google Scholar ]
- Chung M, Kim N. When I learn the news is false: how fact-checking information stems the spread of fake news via third-person perception. Hum Commun Res. 2021; 47 (1):1–24. doi: 10.1093/hcr/hqaa010. [ CrossRef ] [ Google Scholar ]
- Clarke J, Chen H, Du D, Hu YJ. Fake news, investor attention, and market reaction. Inf Syst Res. 2020 doi: 10.1287/isre.2019.0910. [ CrossRef ] [ Google Scholar ]
- Clayton K, Blair S, Busam JA, Forstner S, Glance J, Green G, Kawata A, Kovvuri A, Martin J, Morgan E, et al. Real solutions for fake news? Measuring the effectiveness of general warnings and fact-check tags in reducing belief in false stories on social media. Polit Behav. 2020; 42 (4):1073–1095. doi: 10.1007/s11109-019-09533-0. [ CrossRef ] [ Google Scholar ]
- Collins B, Hoang DT, Nguyen NT, Hwang D (2020) Fake news types and detection models on social media a state-of-the-art survey. In: Asian conference on intelligent information and database systems. Springer, Berlin, pp 562–573 10.1007/978-981-15-3380-8_49
- Conroy NK, Rubin VL, Chen Y. Automatic deception detection: methods for finding fake news. Proc Assoc Inf Sci Technol. 2015; 52 (1):1–4. doi: 10.1002/pra2.2015.145052010082. [ CrossRef ] [ Google Scholar ]
- Cooke NA. Posttruth, truthiness, and alternative facts: Information behavior and critical information consumption for a new age. Libr Q. 2017; 87 (3):211–221. doi: 10.1086/692298. [ CrossRef ] [ Google Scholar ]
- Coscia M, Rossi L. Distortions of political bias in crowdsourced misinformation flagging. J R Soc Interface. 2020; 17 (167):20200020. doi: 10.1098/rsif.2020.0020. [ PMC free article ] [ PubMed ] [ CrossRef ] [ Google Scholar ]
- Dame Adjin-Tettey T. Combating fake news, disinformation, and misinformation: experimental evidence for media literacy education. Cogent Arts Human. 2022; 9 (1):2037229. doi: 10.1080/23311983.2022.2037229. [ CrossRef ] [ Google Scholar ]
- Deepak S, Chitturi B. Deep neural approach to fake-news identification. Procedia Comput Sci. 2020; 167 :2236–2243. doi: 10.1016/j.procs.2020.03.276. [ CrossRef ] [ Google Scholar ]
- de Cock Buning M (2018) A multi-dimensional approach to disinformation: report of the independent high level group on fake news and online disinformation. Publications Office of the European Union
- Del Vicario M, Quattrociocchi W, Scala A, Zollo F. Polarization and fake news: early warning of potential misinformation targets. ACM Trans Web (TWEB) 2019; 13 (2):1–22. doi: 10.1145/3316809. [ CrossRef ] [ Google Scholar ]
- Demuyakor J, Opata EM. Fake news on social media: predicting which media format influences fake news most on facebook. J Intell Commun. 2022 doi: 10.54963/jic.v2i1.56. [ CrossRef ] [ Google Scholar ]
- Derakhshan H, Wardle C (2017) Information disorder: definitions. In: Understanding and addressing the disinformation ecosystem, pp 5–12
- Desai AN, Ruidera D, Steinbrink JM, Granwehr B, Lee DH. Misinformation and disinformation: the potential disadvantages of social media in infectious disease and how to combat them. Clin Infect Dis. 2022; 74 (Supplement–3):e34–e39. doi: 10.1093/cid/ciac109. [ PMC free article ] [ PubMed ] [ CrossRef ] [ Google Scholar ]
- Di Domenico G, Sit J, Ishizaka A, Nunan D. Fake news, social media and marketing: a systematic review. J Bus Res. 2021; 124 :329–341. doi: 10.1016/j.jbusres.2020.11.037. [ CrossRef ] [ Google Scholar ]
- Dias N, Pennycook G, Rand DG. Emphasizing publishers does not effectively reduce susceptibility to misinformation on social media. Harv Kennedy School Misinform Rev. 2020 doi: 10.37016/mr-2020-001. [ CrossRef ] [ Google Scholar ]
- DiCicco KW, Agarwal N (2020) Blockchain technology-based solutions to fight misinformation: a survey. In: Disinformation, misinformation, and fake news in social media. Springer, Berlin, pp 267–281, 10.1007/978-3-030-42699-6_14
- Douglas KM, Uscinski JE, Sutton RM, Cichocka A, Nefes T, Ang CS, Deravi F. Understanding conspiracy theories. Polit Psychol. 2019; 40 :3–35. doi: 10.1111/pops.12568. [ CrossRef ] [ Google Scholar ]
- Edgerly S, Mourão RR, Thorson E, Tham SM. When do audiences verify? How perceptions about message and source influence audience verification of news headlines. J Mass Commun Q. 2020; 97 (1):52–71. doi: 10.1177/1077699019864680. [ CrossRef ] [ Google Scholar ]
- Egelhofer JL, Lecheler S. Fake news as a two-dimensional phenomenon: a framework and research agenda. Ann Int Commun Assoc. 2019; 43 (2):97–116. doi: 10.1080/23808985.2019.1602782. [ CrossRef ] [ Google Scholar ]
- Elhadad MK, Li KF, Gebali F (2019) A novel approach for selecting hybrid features from online news textual metadata for fake news detection. In: International conference on p2p, parallel, grid, cloud and internet computing. Springer, Berlin, pp 914–925, 10.1007/978-3-030-33509-0_86
- ERGA (2018) Fake news, and the information disorder. European Broadcasting Union (EBU)
- ERGA (2021) Notions of disinformation and related concepts. European Regulators Group for Audiovisual Media Services (ERGA)
- Escolà-Gascón Á. New techniques to measure lie detection using Covid-19 fake news and the Multivariable Multiaxial Suggestibility Inventory-2 (MMSI-2) Comput Hum Behav Rep. 2021; 3 :100049. doi: 10.1016/j.chbr.2020.100049. [ CrossRef ] [ Google Scholar ]
- Fazio L. Pausing to consider why a headline is true or false can help reduce the sharing of false news. Harv Kennedy School Misinformation Rev. 2020 doi: 10.37016/mr-2020-009. [ CrossRef ] [ Google Scholar ]
- Ferrara E, Varol O, Davis C, Menczer F, Flammini A. The rise of social bots. Commun ACM. 2016; 59 (7):96–104. doi: 10.1145/2818717. [ CrossRef ] [ Google Scholar ]
- Flynn D, Nyhan B, Reifler J. The nature and origins of misperceptions: understanding false and unsupported beliefs about politics. Polit Psychol. 2017; 38 :127–150. doi: 10.1111/pops.12394. [ CrossRef ] [ Google Scholar ]
- Fraga-Lamas P, Fernández-Caramés TM. Fake news, disinformation, and deepfakes: leveraging distributed ledger technologies and blockchain to combat digital deception and counterfeit reality. IT Prof. 2020; 22 (2):53–59. doi: 10.1109/MITP.2020.2977589. [ CrossRef ] [ Google Scholar ]
- Freeman D, Waite F, Rosebrock L, Petit A, Causier C, East A, Jenner L, Teale AL, Carr L, Mulhall S, et al. Coronavirus conspiracy beliefs, mistrust, and compliance with government guidelines in England. Psychol Med. 2020 doi: 10.1017/S0033291720001890. [ PMC free article ] [ PubMed ] [ CrossRef ] [ Google Scholar ]
- Friggeri A, Adamic L, Eckles D, Cheng J (2014) Rumor cascades. In: Proceedings of the international AAAI conference on web and social media
- García SA, García GG, Prieto MS, Moreno Guerrero AJ, Rodríguez Jiménez C. The impact of term fake news on the scientific community. Scientific performance and mapping in web of science. Soc Sci. 2020 doi: 10.3390/socsci9050073. [ CrossRef ] [ Google Scholar ]
- Garrett RK, Bond RM. Conservatives’ susceptibility to political misperceptions. Sci Adv. 2021 doi: 10.1126/sciadv.abf1234. [ PMC free article ] [ PubMed ] [ CrossRef ] [ Google Scholar ]
- Giachanou A, Ríssola EA, Ghanem B, Crestani F, Rosso P (2020) The role of personality and linguistic patterns in discriminating between fake news spreaders and fact checkers. In: International conference on applications of natural language to information systems. Springer, Berlin, pp 181–192 10.1007/978-3-030-51310-8_17
- Golbeck J, Mauriello M, Auxier B, Bhanushali KH, Bonk C, Bouzaghrane MA, Buntain C, Chanduka R, Cheakalos P, Everett JB et al (2018) Fake news vs satire: a dataset and analysis. In: Proceedings of the 10th ACM conference on web science, pp 17–21, 10.1145/3201064.3201100
- Goldani MH, Momtazi S, Safabakhsh R. Detecting fake news with capsule neural networks. Appl Soft Comput. 2021; 101 :106991. doi: 10.1016/j.asoc.2020.106991. [ CrossRef ] [ Google Scholar ]
- Goldstein I, Yang L. Good disclosure, bad disclosure. J Financ Econ. 2019; 131 (1):118–138. doi: 10.1016/j.jfineco.2018.08.004. [ CrossRef ] [ Google Scholar ]
- Grinberg N, Joseph K, Friedland L, Swire-Thompson B, Lazer D. Fake news on Twitter during the 2016 US presidential election. Science. 2019; 363 (6425):374–378. doi: 10.1126/science.aau2706. [ PubMed ] [ CrossRef ] [ Google Scholar ]
- Guadagno RE, Guttieri K (2021) Fake news and information warfare: an examination of the political and psychological processes from the digital sphere to the real world. In: Research anthology on fake news, political warfare, and combatting the spread of misinformation. IGI Global, pp 218–242 10.4018/978-1-7998-7291-7.ch013
- Guess A, Nagler J, Tucker J. Less than you think: prevalence and predictors of fake news dissemination on Facebook. Sci Adv. 2019 doi: 10.1126/sciadv.aau4586. [ PMC free article ] [ PubMed ] [ CrossRef ] [ Google Scholar ]
- Guo C, Cao J, Zhang X, Shu K, Yu M (2019) Exploiting emotions for fake news detection on social media. arXiv preprint arXiv:1903.01728
- Guo B, Ding Y, Yao L, Liang Y, Yu Z. The future of false information detection on social media: new perspectives and trends. ACM Comput Surv (CSUR) 2020; 53 (4):1–36. doi: 10.1145/3393880. [ CrossRef ] [ Google Scholar ]
- Gupta A, Li H, Farnoush A, Jiang W. Understanding patterns of covid infodemic: a systematic and pragmatic approach to curb fake news. J Bus Res. 2022; 140 :670–683. doi: 10.1016/j.jbusres.2021.11.032. [ PMC free article ] [ PubMed ] [ CrossRef ] [ Google Scholar ]
- Ha L, Andreu Perez L, Ray R. Mapping recent development in scholarship on fake news and misinformation, 2008 to 2017: disciplinary contribution, topics, and impact. Am Behav Sci. 2021; 65 (2):290–315. doi: 10.1177/0002764219869402. [ CrossRef ] [ Google Scholar ]
- Habib A, Asghar MZ, Khan A, Habib A, Khan A. False information detection in online content and its role in decision making: a systematic literature review. Soc Netw Anal Min. 2019; 9 (1):1–20. doi: 10.1007/s13278-019-0595-5. [ CrossRef ] [ Google Scholar ]
- Hage H, Aïmeur E, Guedidi A (2021) Understanding the landscape of online deception. In: Research anthology on fake news, political warfare, and combatting the spread of misinformation. IGI Global, pp 39–66. 10.4018/978-1-7998-2543-2.ch014
- Hakak S, Alazab M, Khan S, Gadekallu TR, Maddikunta PKR, Khan WZ. An ensemble machine learning approach through effective feature extraction to classify fake news. Futur Gener Comput Syst. 2021; 117 :47–58. doi: 10.1016/j.future.2020.11.022. [ CrossRef ] [ Google Scholar ]
- Hamdi T, Slimi H, Bounhas I, Slimani Y (2020) A hybrid approach for fake news detection in Twitter based on user features and graph embedding. In: International conference on distributed computing and internet technology. Springer, Berlin, pp 266–280. 10.1007/978-3-030-36987-3_17
- Hameleers M. Separating truth from lies: comparing the effects of news media literacy interventions and fact-checkers in response to political misinformation in the us and netherlands. Inf Commun Soc. 2022; 25 (1):110–126. doi: 10.1080/1369118X.2020.1764603. [ CrossRef ] [ Google Scholar ]
- Hameleers M, Powell TE, Van Der Meer TG, Bos L. A picture paints a thousand lies? The effects and mechanisms of multimodal disinformation and rebuttals disseminated via social media. Polit Commun. 2020; 37 (2):281–301. doi: 10.1080/10584609.2019.1674979. [ CrossRef ] [ Google Scholar ]
- Hameleers M, Brosius A, de Vreese CH. Whom to trust? media exposure patterns of citizens with perceptions of misinformation and disinformation related to the news media. Eur J Commun. 2022 doi: 10.1177/02673231211072667. [ CrossRef ] [ Google Scholar ]
- Hartley K, Vu MK. Fighting fake news in the Covid-19 era: policy insights from an equilibrium model. Policy Sci. 2020; 53 (4):735–758. doi: 10.1007/s11077-020-09405-z. [ PMC free article ] [ PubMed ] [ CrossRef ] [ Google Scholar ]
- Hasan HR, Salah K. Combating deepfake videos using blockchain and smart contracts. IEEE Access. 2019; 7 :41596–41606. doi: 10.1109/ACCESS.2019.2905689. [ CrossRef ] [ Google Scholar ]
- Hiriyannaiah S, Srinivas A, Shetty GK, Siddesh G, Srinivasa K (2020) A computationally intelligent agent for detecting fake news using generative adversarial networks. Hybrid computational intelligence: challenges and applications. pp 69–96 10.1016/B978-0-12-818699-2.00004-4
- Hosseinimotlagh S, Papalexakis EE (2018) Unsupervised content-based identification of fake news articles with tensor decomposition ensembles. In: Proceedings of the workshop on misinformation and misbehavior mining on the web (MIS2)
- Huckle S, White M. Fake news: a technological approach to proving the origins of content, using blockchains. Big Data. 2017; 5 (4):356–371. doi: 10.1089/big.2017.0071. [ PubMed ] [ CrossRef ] [ Google Scholar ]
- Huffaker JS, Kummerfeld JK, Lasecki WS, Ackerman MS (2020) Crowdsourced detection of emotionally manipulative language. In: Proceedings of the 2020 CHI conference on human factors in computing systems. pp 1–14 10.1145/3313831.3376375
- Ireton C, Posetti J. Journalism, fake news & disinformation: handbook for journalism education and training. Paris: UNESCO Publishing; 2018. [ Google Scholar ]
- Islam MR, Liu S, Wang X, Xu G. Deep learning for misinformation detection on online social networks: a survey and new perspectives. Soc Netw Anal Min. 2020; 10 (1):1–20. doi: 10.1007/s13278-020-00696-x. [ PMC free article ] [ PubMed ] [ CrossRef ] [ Google Scholar ]
- Ismailov M, Tsikerdekis M, Zeadally S. Vulnerabilities to online social network identity deception detection research and recommendations for mitigation. Fut Internet. 2020; 12 (9):148. doi: 10.3390/fi12090148. [ CrossRef ] [ Google Scholar ]
- Jakesch M, Koren M, Evtushenko A, Naaman M (2019) The role of source and expressive responding in political news evaluation. In: Computation and journalism symposium
- Jamieson KH. Cyberwar: how Russian hackers and trolls helped elect a president: what we don’t, can’t, and do know. Oxford: Oxford University Press; 2020. [ Google Scholar ]
- Jiang S, Chen X, Zhang L, Chen S, Liu H (2019) User-characteristic enhanced model for fake news detection in social media. In: CCF International conference on natural language processing and Chinese computing, Springer, Berlin, pp 634–646. 10.1007/978-3-030-32233-5_49
- Jin Z, Cao J, Zhang Y, Luo J (2016) News verification by exploiting conflicting social viewpoints in microblogs. In: Proceedings of the AAAI conference on artificial intelligence
- Jing TW, Murugesan RK (2018) A theoretical framework to build trust and prevent fake news in social media using blockchain. In: International conference of reliable information and communication technology. Springer, Berlin, pp 955–962, 10.1007/978-3-319-99007-1_88
- Jones-Jang SM, Mortensen T, Liu J. Does media literacy help identification of fake news? Information literacy helps, but other literacies don’t. Am Behav Sci. 2021; 65 (2):371–388. doi: 10.1177/0002764219869406. [ CrossRef ] [ Google Scholar ]
- Jungherr A, Schroeder R. Disinformation and the structural transformations of the public arena: addressing the actual challenges to democracy. Soc Media Soc. 2021 doi: 10.1177/2056305121988928. [ CrossRef ] [ Google Scholar ]
- Kaliyar RK (2018) Fake news detection using a deep neural network. In: 2018 4th international conference on computing communication and automation (ICCCA), IEEE, pp 1–7 10.1109/CCAA.2018.8777343
- Kaliyar RK, Goswami A, Narang P, Sinha S. Fndnet—a deep convolutional neural network for fake news detection. Cogn Syst Res. 2020; 61 :32–44. doi: 10.1016/j.cogsys.2019.12.005. [ CrossRef ] [ Google Scholar ]
- Kapantai E, Christopoulou A, Berberidis C, Peristeras V. A systematic literature review on disinformation: toward a unified taxonomical framework. New Media Soc. 2021; 23 (5):1301–1326. doi: 10.1177/1461444820959296. [ CrossRef ] [ Google Scholar ]
- Kapusta J, Benko L, Munk M (2019) Fake news identification based on sentiment and frequency analysis. In: International conference Europe middle east and North Africa information systems and technologies to support learning. Springer, Berlin, pp 400–409, 10.1007/978-3-030-36778-7_44
- Kaur S, Kumar P, Kumaraguru P. Automating fake news detection system using multi-level voting model. Soft Comput. 2020; 24 (12):9049–9069. doi: 10.1007/s00500-019-04436-y. [ CrossRef ] [ Google Scholar ]
- Khan SA, Alkawaz MH, Zangana HM (2019) The use and abuse of social media for spreading fake news. In: 2019 IEEE international conference on automatic control and intelligent systems (I2CACIS), IEEE, pp 145–148. 10.1109/I2CACIS.2019.8825029
- Kim J, Tabibian B, Oh A, Schölkopf B, Gomez-Rodriguez M (2018) Leveraging the crowd to detect and reduce the spread of fake news and misinformation. In: Proceedings of the eleventh ACM international conference on web search and data mining, pp 324–332. 10.1145/3159652.3159734
- Klein D, Wueller J. Fake news: a legal perspective. J Internet Law. 2017; 20 (10):5–13. [ Google Scholar ]
- Kogan S, Moskowitz TJ, Niessner M (2019) Fake news: evidence from financial markets. Available at SSRN 3237763
- Kuklinski JH, Quirk PJ, Jerit J, Schwieder D, Rich RF. Misinformation and the currency of democratic citizenship. J Polit. 2000; 62 (3):790–816. doi: 10.1111/0022-3816.00033. [ CrossRef ] [ Google Scholar ]
- Kumar S, Shah N (2018) False information on web and social media: a survey. arXiv preprint arXiv:1804.08559
- Kumar S, West R, Leskovec J (2016) Disinformation on the web: impact, characteristics, and detection of Wikipedia hoaxes. In: Proceedings of the 25th international conference on world wide web, pp 591–602. 10.1145/2872427.2883085
- La Barbera D, Roitero K, Demartini G, Mizzaro S, Spina D (2020) Crowdsourcing truthfulness: the impact of judgment scale and assessor bias. In: European conference on information retrieval. Springer, Berlin, pp 207–214. 10.1007/978-3-030-45442-5_26
- Lanius C, Weber R, MacKenzie WI. Use of bot and content flags to limit the spread of misinformation among social networks: a behavior and attitude survey. Soc Netw Anal Min. 2021; 11 (1):1–15. doi: 10.1007/s13278-021-00739-x. [ PMC free article ] [ PubMed ] [ CrossRef ] [ Google Scholar ]
- Lazer DM, Baum MA, Benkler Y, Berinsky AJ, Greenhill KM, Menczer F, Metzger MJ, Nyhan B, Pennycook G, Rothschild D, et al. The science of fake news. Science. 2018; 359 (6380):1094–1096. doi: 10.1126/science.aao2998. [ PubMed ] [ CrossRef ] [ Google Scholar ]
- Le T, Shu K, Molina MD, Lee D, Sundar SS, Liu H (2019) 5 sources of clickbaits you should know! Using synthetic clickbaits to improve prediction and distinguish between bot-generated and human-written headlines. In: 2019 IEEE/ACM international conference on advances in social networks analysis and mining (ASONAM). IEEE, pp 33–40. 10.1145/3341161.3342875
- Lewandowsky S (2020) Climate change, disinformation, and how to combat it. In: Annual Review of Public Health 42. 10.1146/annurev-publhealth-090419-102409 [ PubMed ]
- Liu Y, Wu YF (2018) Early detection of fake news on social media through propagation path classification with recurrent and convolutional networks. In: Proceedings of the AAAI conference on artificial intelligence, pp 354–361
- Luo M, Hancock JT, Markowitz DM. Credibility perceptions and detection accuracy of fake news headlines on social media: effects of truth-bias and endorsement cues. Commun Res. 2022; 49 (2):171–195. doi: 10.1177/0093650220921321. [ CrossRef ] [ Google Scholar ]
- Lutzke L, Drummond C, Slovic P, Árvai J. Priming critical thinking: simple interventions limit the influence of fake news about climate change on Facebook. Glob Environ Chang. 2019; 58 :101964. doi: 10.1016/j.gloenvcha.2019.101964. [ CrossRef ] [ Google Scholar ]
- Maertens R, Anseel F, van der Linden S. Combatting climate change misinformation: evidence for longevity of inoculation and consensus messaging effects. J Environ Psychol. 2020; 70 :101455. doi: 10.1016/j.jenvp.2020.101455. [ CrossRef ] [ Google Scholar ]
- Mahabub A. A robust technique of fake news detection using ensemble voting classifier and comparison with other classifiers. SN Applied Sciences. 2020; 2 (4):1–9. doi: 10.1007/s42452-020-2326-y. [ CrossRef ] [ Google Scholar ]
- Mahbub S, Pardede E, Kayes A, Rahayu W. Controlling astroturfing on the internet: a survey on detection techniques and research challenges. Int J Web Grid Serv. 2019; 15 (2):139–158. doi: 10.1504/IJWGS.2019.099561. [ CrossRef ] [ Google Scholar ]
- Marsden C, Meyer T, Brown I. Platform values and democratic elections: how can the law regulate digital disinformation? Comput Law Secur Rev. 2020; 36 :105373. doi: 10.1016/j.clsr.2019.105373. [ CrossRef ] [ Google Scholar ]
- Masciari E, Moscato V, Picariello A, Sperlí G (2020) Detecting fake news by image analysis. In: Proceedings of the 24th symposium on international database engineering and applications, pp 1–5. 10.1145/3410566.3410599
- Mazzeo V, Rapisarda A. Investigating fake and reliable news sources using complex networks analysis. Front Phys. 2022; 10 :886544. doi: 10.3389/fphy.2022.886544. [ CrossRef ] [ Google Scholar ]
- McGrew S. Learning to evaluate: an intervention in civic online reasoning. Comput Educ. 2020; 145 :103711. doi: 10.1016/j.compedu.2019.103711. [ CrossRef ] [ Google Scholar ]
- McGrew S, Breakstone J, Ortega T, Smith M, Wineburg S. Can students evaluate online sources? Learning from assessments of civic online reasoning. Theory Res Soc Educ. 2018; 46 (2):165–193. doi: 10.1080/00933104.2017.1416320. [ CrossRef ] [ Google Scholar ]
- Meel P, Vishwakarma DK. Fake news, rumor, information pollution in social media and web: a contemporary survey of state-of-the-arts, challenges and opportunities. Expert Syst Appl. 2020; 153 :112986. doi: 10.1016/j.eswa.2019.112986. [ CrossRef ] [ Google Scholar ]
- Meese J, Frith J, Wilken R. Covid-19, 5G conspiracies and infrastructural futures. Media Int Aust. 2020; 177 (1):30–46. doi: 10.1177/1329878X20952165. [ CrossRef ] [ Google Scholar ]
- Metzger MJ, Hartsell EH, Flanagin AJ. Cognitive dissonance or credibility? A comparison of two theoretical explanations for selective exposure to partisan news. Commun Res. 2020; 47 (1):3–28. doi: 10.1177/0093650215613136. [ CrossRef ] [ Google Scholar ]
- Micallef N, He B, Kumar S, Ahamad M, Memon N (2020) The role of the crowd in countering misinformation: a case study of the Covid-19 infodemic. arXiv preprint arXiv:2011.05773
- Mihailidis P, Viotty S. Spreadable spectacle in digital culture: civic expression, fake news, and the role of media literacies in “post-fact society. Am Behav Sci. 2017; 61 (4):441–454. doi: 10.1177/0002764217701217. [ CrossRef ] [ Google Scholar ]
- Mishra R (2020) Fake news detection using higher-order user to user mutual-attention progression in propagation paths. In: Proceedings of the IEEE/CVF conference on computer vision and pattern recognition workshops, pp 652–653
- Mishra S, Shukla P, Agarwal R. Analyzing machine learning enabled fake news detection techniques for diversified datasets. Wirel Commun Mobile Comput. 2022 doi: 10.1155/2022/1575365. [ CrossRef ] [ Google Scholar ]
- Molina MD, Sundar SS, Le T, Lee D. “Fake news” is not simply false information: a concept explication and taxonomy of online content. Am Behav Sci. 2021; 65 (2):180–212. doi: 10.1177/0002764219878224. [ CrossRef ] [ Google Scholar ]
- Moro C, Birt JR (2022) Review bombing is a dirty practice, but research shows games do benefit from online feedback. Conversation. https://research.bond.edu.au/en/publications/review-bombing-is-a-dirty-practice-but-research-shows-games-do-be
- Mustafaraj E, Metaxas PT (2017) The fake news spreading plague: was it preventable? In: Proceedings of the 2017 ACM on web science conference, pp 235–239. 10.1145/3091478.3091523
- Nagel TW. Measuring fake news acumen using a news media literacy instrument. J Media Liter Educ. 2022; 14 (1):29–42. doi: 10.23860/JMLE-2022-14-1-3. [ CrossRef ] [ Google Scholar ]
- Nakov P (2020) Can we spot the “fake news” before it was even written? arXiv preprint arXiv:2008.04374
- Nekmat E. Nudge effect of fact-check alerts: source influence and media skepticism on sharing of news misinformation in social media. Soc Media Soc. 2020 doi: 10.1177/2056305119897322. [ CrossRef ] [ Google Scholar ]
- Nygren T, Brounéus F, Svensson G. Diversity and credibility in young people’s news feeds: a foundation for teaching and learning citizenship in a digital era. J Soc Sci Educ. 2019; 18 (2):87–109. doi: 10.4119/jsse-917. [ CrossRef ] [ Google Scholar ]
- Nyhan B, Reifler J. Displacing misinformation about events: an experimental test of causal corrections. J Exp Polit Sci. 2015; 2 (1):81–93. doi: 10.1017/XPS.2014.22. [ CrossRef ] [ Google Scholar ]
- Nyhan B, Porter E, Reifler J, Wood TJ. Taking fact-checks literally but not seriously? The effects of journalistic fact-checking on factual beliefs and candidate favorability. Polit Behav. 2020; 42 (3):939–960. doi: 10.1007/s11109-019-09528-x. [ CrossRef ] [ Google Scholar ]
- Nyow NX, Chua HN (2019) Detecting fake news with tweets’ properties. In: 2019 IEEE conference on application, information and network security (AINS), IEEE, pp 24–29. 10.1109/AINS47559.2019.8968706
- Ochoa IS, de Mello G, Silva LA, Gomes AJ, Fernandes AM, Leithardt VRQ (2019) Fakechain: a blockchain architecture to ensure trust in social media networks. In: International conference on the quality of information and communications technology. Springer, Berlin, pp 105–118. 10.1007/978-3-030-29238-6_8
- Ozbay FA, Alatas B. Fake news detection within online social media using supervised artificial intelligence algorithms. Physica A. 2020; 540 :123174. doi: 10.1016/j.physa.2019.123174. [ CrossRef ] [ Google Scholar ]
- Ozturk P, Li H, Sakamoto Y (2015) Combating rumor spread on social media: the effectiveness of refutation and warning. In: 2015 48th Hawaii international conference on system sciences, IEEE, pp 2406–2414. 10.1109/HICSS.2015.288
- Parikh SB, Atrey PK (2018) Media-rich fake news detection: a survey. In: 2018 IEEE conference on multimedia information processing and retrieval (MIPR), IEEE, pp 436–441.10.1109/MIPR.2018.00093
- Parrish K (2018) Deep learning & machine learning: what’s the difference? Online: https://parsers.me/deep-learning-machine-learning-whats-the-difference/ . Accessed 20 May 2020
- Paschen J. Investigating the emotional appeal of fake news using artificial intelligence and human contributions. J Prod Brand Manag. 2019; 29 (2):223–233. doi: 10.1108/JPBM-12-2018-2179. [ CrossRef ] [ Google Scholar ]
- Pathak A, Srihari RK (2019) Breaking! Presenting fake news corpus for automated fact checking. In: Proceedings of the 57th annual meeting of the association for computational linguistics: student research workshop, pp 357–362
- Peng J, Detchon S, Choo KKR, Ashman H. Astroturfing detection in social media: a binary n-gram-based approach. Concurr Comput: Pract Exp. 2017; 29 (17):e4013. doi: 10.1002/cpe.4013. [ CrossRef ] [ Google Scholar ]
- Pennycook G, Rand DG. Fighting misinformation on social media using crowdsourced judgments of news source quality. Proc Natl Acad Sci. 2019; 116 (7):2521–2526. doi: 10.1073/pnas.1806781116. [ PMC free article ] [ PubMed ] [ CrossRef ] [ Google Scholar ]
- Pennycook G, Rand DG. Who falls for fake news? The roles of bullshit receptivity, overclaiming, familiarity, and analytic thinking. J Pers. 2020; 88 (2):185–200. doi: 10.1111/jopy.12476. [ PubMed ] [ CrossRef ] [ Google Scholar ]
- Pennycook G, Bear A, Collins ET, Rand DG. The implied truth effect: attaching warnings to a subset of fake news headlines increases perceived accuracy of headlines without warnings. Manag Sci. 2020; 66 (11):4944–4957. doi: 10.1287/mnsc.2019.3478. [ CrossRef ] [ Google Scholar ]
- Pennycook G, McPhetres J, Zhang Y, Lu JG, Rand DG. Fighting Covid-19 misinformation on social media: experimental evidence for a scalable accuracy-nudge intervention. Psychol Sci. 2020; 31 (7):770–780. doi: 10.1177/0956797620939054. [ PMC free article ] [ PubMed ] [ CrossRef ] [ Google Scholar ]
- Potthast M, Kiesel J, Reinartz K, Bevendorff J, Stein B (2017) A stylometric inquiry into hyperpartisan and fake news. arXiv preprint arXiv:1702.05638
- Previti M, Rodriguez-Fernandez V, Camacho D, Carchiolo V, Malgeri M (2020) Fake news detection using time series and user features classification. In: International conference on the applications of evolutionary computation (Part of EvoStar), Springer, Berlin, pp 339–353. 10.1007/978-3-030-43722-0_22
- Przybyla P (2020) Capturing the style of fake news. In: Proceedings of the AAAI conference on artificial intelligence, pp 490–497. 10.1609/aaai.v34i01.5386
- Qayyum A, Qadir J, Janjua MU, Sher F. Using blockchain to rein in the new post-truth world and check the spread of fake news. IT Prof. 2019; 21 (4):16–24. doi: 10.1109/MITP.2019.2910503. [ CrossRef ] [ Google Scholar ]
- Qian F, Gong C, Sharma K, Liu Y (2018) Neural user response generator: fake news detection with collective user intelligence. In: IJCAI, vol 18, pp 3834–3840. 10.24963/ijcai.2018/533
- Raza S, Ding C. Fake news detection based on news content and social contexts: a transformer-based approach. Int J Data Sci Anal. 2022; 13 (4):335–362. doi: 10.1007/s41060-021-00302-z. [ PMC free article ] [ PubMed ] [ CrossRef ] [ Google Scholar ]
- Ricard J, Medeiros J (2020) Using misinformation as a political weapon: Covid-19 and Bolsonaro in Brazil. Harv Kennedy School misinformation Rev 1(3). https://misinforeview.hks.harvard.edu/article/using-misinformation-as-a-political-weapon-covid-19-and-bolsonaro-in-brazil/
- Roozenbeek J, van der Linden S. Fake news game confers psychological resistance against online misinformation. Palgrave Commun. 2019; 5 (1):1–10. doi: 10.1057/s41599-019-0279-9. [ CrossRef ] [ Google Scholar ]
- Roozenbeek J, van der Linden S, Nygren T. Prebunking interventions based on the psychological theory of “inoculation” can reduce susceptibility to misinformation across cultures. Harv Kennedy School Misinformation Rev. 2020 doi: 10.37016//mr-2020-008. [ CrossRef ] [ Google Scholar ]
- Roozenbeek J, Schneider CR, Dryhurst S, Kerr J, Freeman AL, Recchia G, Van Der Bles AM, Van Der Linden S. Susceptibility to misinformation about Covid-19 around the world. R Soc Open Sci. 2020; 7 (10):201199. doi: 10.1098/rsos.201199. [ PMC free article ] [ PubMed ] [ CrossRef ] [ Google Scholar ]
- Rubin VL, Conroy N, Chen Y, Cornwell S (2016) Fake news or truth? Using satirical cues to detect potentially misleading news. In: Proceedings of the second workshop on computational approaches to deception detection, pp 7–17
- Ruchansky N, Seo S, Liu Y (2017) Csi: a hybrid deep model for fake news detection. In: Proceedings of the 2017 ACM on conference on information and knowledge management, pp 797–806. 10.1145/3132847.3132877
- Schuyler AJ (2019) Regulating facts: a procedural framework for identifying, excluding, and deterring the intentional or knowing proliferation of fake news online. Univ Ill JL Technol Pol’y, vol 2019, pp 211–240
- Shae Z, Tsai J (2019) AI blockchain platform for trusting news. In: 2019 IEEE 39th international conference on distributed computing systems (ICDCS), IEEE, pp 1610–1619. 10.1109/ICDCS.2019.00160
- Shang W, Liu M, Lin W, Jia M (2018) Tracing the source of news based on blockchain. In: 2018 IEEE/ACIS 17th international conference on computer and information science (ICIS), IEEE, pp 377–381. 10.1109/ICIS.2018.8466516
- Shao C, Ciampaglia GL, Flammini A, Menczer F (2016) Hoaxy: A platform for tracking online misinformation. In: Proceedings of the 25th international conference companion on world wide web, pp 745–750. 10.1145/2872518.2890098
- Shao C, Ciampaglia GL, Varol O, Yang KC, Flammini A, Menczer F. The spread of low-credibility content by social bots. Nat Commun. 2018; 9 (1):1–9. doi: 10.1038/s41467-018-06930-7. [ PMC free article ] [ PubMed ] [ CrossRef ] [ Google Scholar ]
- Shao C, Hui PM, Wang L, Jiang X, Flammini A, Menczer F, Ciampaglia GL. Anatomy of an online misinformation network. PLoS ONE. 2018; 13 (4):e0196087. doi: 10.1371/journal.pone.0196087. [ PMC free article ] [ PubMed ] [ CrossRef ] [ Google Scholar ]
- Sharma K, Qian F, Jiang H, Ruchansky N, Zhang M, Liu Y. Combating fake news: a survey on identification and mitigation techniques. ACM Trans Intell Syst Technol (TIST) 2019; 10 (3):1–42. doi: 10.1145/3305260. [ CrossRef ] [ Google Scholar ]
- Sharma K, Seo S, Meng C, Rambhatla S, Liu Y (2020) Covid-19 on social media: analyzing misinformation in Twitter conversations. arXiv preprint arXiv:2003.12309
- Shen C, Kasra M, Pan W, Bassett GA, Malloch Y, O’Brien JF. Fake images: the effects of source, intermediary, and digital media literacy on contextual assessment of image credibility online. New Media Soc. 2019; 21 (2):438–463. doi: 10.1177/1461444818799526. [ CrossRef ] [ Google Scholar ]
- Sherman IN, Redmiles EM, Stokes JW (2020) Designing indicators to combat fake media. arXiv preprint arXiv:2010.00544
- Shi P, Zhang Z, Choo KKR. Detecting malicious social bots based on clickstream sequences. IEEE Access. 2019; 7 :28855–28862. doi: 10.1109/ACCESS.2019.2901864. [ CrossRef ] [ Google Scholar ]
- Shu K, Sliva A, Wang S, Tang J, Liu H. Fake news detection on social media: a data mining perspective. ACM SIGKDD Explor Newsl. 2017; 19 (1):22–36. doi: 10.1145/3137597.3137600. [ CrossRef ] [ Google Scholar ]
- Shu K, Mahudeswaran D, Wang S, Lee D, Liu H (2018a) Fakenewsnet: a data repository with news content, social context and spatialtemporal information for studying fake news on social media. arXiv preprint arXiv:1809.01286 , 10.1089/big.2020.0062 [ PubMed ]
- Shu K, Wang S, Liu H (2018b) Understanding user profiles on social media for fake news detection. In: 2018 IEEE conference on multimedia information processing and retrieval (MIPR), IEEE, pp 430–435. 10.1109/MIPR.2018.00092
- Shu K, Wang S, Liu H (2019a) Beyond news contents: the role of social context for fake news detection. In: Proceedings of the twelfth ACM international conference on web search and data mining, pp 312–320. 10.1145/3289600.3290994
- Shu K, Zhou X, Wang S, Zafarani R, Liu H (2019b) The role of user profiles for fake news detection. In: Proceedings of the 2019 IEEE/ACM international conference on advances in social networks analysis and mining, pp 436–439. 10.1145/3341161.3342927
- Shu K, Bhattacharjee A, Alatawi F, Nazer TH, Ding K, Karami M, Liu H. Combating disinformation in a social media age. Wiley Interdiscip Rev: Data Min Knowl Discov. 2020; 10 (6):e1385. doi: 10.1002/widm.1385. [ CrossRef ] [ Google Scholar ]
- Shu K, Mahudeswaran D, Wang S, Liu H. Hierarchical propagation networks for fake news detection: investigation and exploitation. Proc Int AAAI Conf Web Soc Media AAAI Press. 2020; 14 :626–637. [ Google Scholar ]
- Shu K, Wang S, Lee D, Liu H (2020c) Mining disinformation and fake news: concepts, methods, and recent advancements. In: Disinformation, misinformation, and fake news in social media. Springer, Berlin, pp 1–19 10.1007/978-3-030-42699-6_1
- Shu K, Zheng G, Li Y, Mukherjee S, Awadallah AH, Ruston S, Liu H (2020d) Early detection of fake news with multi-source weak social supervision. In: ECML/PKDD (3), pp 650–666
- Singh VK, Ghosh I, Sonagara D. Detecting fake news stories via multimodal analysis. J Am Soc Inf Sci. 2021; 72 (1):3–17. doi: 10.1002/asi.24359. [ CrossRef ] [ Google Scholar ]
- Sintos S, Agarwal PK, Yang J (2019) Selecting data to clean for fact checking: minimizing uncertainty vs. maximizing surprise. Proc VLDB Endowm 12(13), 2408–2421. 10.14778/3358701.3358708 [ CrossRef ]
- Snow J (2017) Can AI win the war against fake news? MIT Technology Review Online: https://www.technologyreview.com/s/609717/can-ai-win-the-war-against-fake-news/ . Accessed 3 Oct. 2020
- Song G, Kim S, Hwang H, Lee K (2019) Blockchain-based notarization for social media. In: 2019 IEEE international conference on consumer clectronics (ICCE), IEEE, pp 1–2 10.1109/ICCE.2019.8661978
- Starbird K, Arif A, Wilson T (2019) Disinformation as collaborative work: Surfacing the participatory nature of strategic information operations. In: Proceedings of the ACM on human–computer interaction, vol 3(CSCW), pp 1–26 10.1145/3359229
- Sterret D, Malato D, Benz J, Kantor L, Tompson T, Rosenstiel T, Sonderman J, Loker K, Swanson E (2018) Who shared it? How Americans decide what news to trust on social media. Technical report, Norc Working Paper Series, WP-2018-001, pp 1–24
- Sutton RM, Douglas KM. Conspiracy theories and the conspiracy mindset: implications for political ideology. Curr Opin Behav Sci. 2020; 34 :118–122. doi: 10.1016/j.cobeha.2020.02.015. [ CrossRef ] [ Google Scholar ]
- Tandoc EC, Jr, Thomas RJ, Bishop L. What is (fake) news? Analyzing news values (and more) in fake stories. Media Commun. 2021; 9 (1):110–119. doi: 10.17645/mac.v9i1.3331. [ CrossRef ] [ Google Scholar ]
- Tchakounté F, Faissal A, Atemkeng M, Ntyam A. A reliable weighting scheme for the aggregation of crowd intelligence to detect fake news. Information. 2020; 11 (6):319. doi: 10.3390/info11060319. [ CrossRef ] [ Google Scholar ]
- Tchechmedjiev A, Fafalios P, Boland K, Gasquet M, Zloch M, Zapilko B, Dietze S, Todorov K (2019) Claimskg: a knowledge graph of fact-checked claims. In: International semantic web conference. Springer, Berlin, pp 309–324 10.1007/978-3-030-30796-7_20
- Treen KMd, Williams HT, O’Neill SJ. Online misinformation about climate change. Wiley Interdiscip Rev Clim Change. 2020; 11 (5):e665. doi: 10.1002/wcc.665. [ CrossRef ] [ Google Scholar ]
- Tsang SJ. Motivated fake news perception: the impact of news sources and policy support on audiences’ assessment of news fakeness. J Mass Commun Q. 2020 doi: 10.1177/1077699020952129. [ CrossRef ] [ Google Scholar ]
- Tschiatschek S, Singla A, Gomez Rodriguez M, Merchant A, Krause A (2018) Fake news detection in social networks via crowd signals. In: Companion proceedings of the the web conference 2018, pp 517–524. 10.1145/3184558.3188722
- Uppada SK, Manasa K, Vidhathri B, Harini R, Sivaselvan B. Novel approaches to fake news and fake account detection in OSNS: user social engagement and visual content centric model. Soc Netw Anal Min. 2022; 12 (1):1–19. doi: 10.1007/s13278-022-00878-9. [ PMC free article ] [ PubMed ] [ CrossRef ] [ Google Scholar ]
- Van der Linden S, Roozenbeek J (2020) Psychological inoculation against fake news. In: Accepting, sharing, and correcting misinformation, the psychology of fake news. 10.4324/9780429295379-11
- Van der Linden S, Panagopoulos C, Roozenbeek J. You are fake news: political bias in perceptions of fake news. Media Cult Soc. 2020; 42 (3):460–470. doi: 10.1177/0163443720906992. [ CrossRef ] [ Google Scholar ]
- Valenzuela S, Muñiz C, Santos M. Social media and belief in misinformation in mexico: a case of maximal panic, minimal effects? Int J Press Polit. 2022 doi: 10.1177/19401612221088988. [ CrossRef ] [ Google Scholar ]
- Vasu N, Ang B, Teo TA, Jayakumar S, Raizal M, Ahuja J (2018) Fake news: national security in the post-truth era. RSIS
- Vereshchaka A, Cosimini S, Dong W (2020) Analyzing and distinguishing fake and real news to mitigate the problem of disinformation. In: Computational and mathematical organization theory, pp 1–15. 10.1007/s10588-020-09307-8
- Verstraete M, Bambauer DE, Bambauer JR (2017) Identifying and countering fake news. Arizona legal studies discussion paper 73(17-15). 10.2139/ssrn.3007971
- Vilmer J, Escorcia A, Guillaume M, Herrera J (2018) Information manipulation: a challenge for our democracies. In: Report by the Policy Planning Staff (CAPS) of the ministry for europe and foreign affairs, and the institute for strategic research (RSEM) of the Ministry for the Armed Forces
- Vishwakarma DK, Varshney D, Yadav A. Detection and veracity analysis of fake news via scrapping and authenticating the web search. Cogn Syst Res. 2019; 58 :217–229. doi: 10.1016/j.cogsys.2019.07.004. [ CrossRef ] [ Google Scholar ]
- Vlachos A, Riedel S (2014) Fact checking: task definition and dataset construction. In: Proceedings of the ACL 2014 workshop on language technologies and computational social science, pp 18–22. 10.3115/v1/W14-2508
- von der Weth C, Abdul A, Fan S, Kankanhalli M (2020) Helping users tackle algorithmic threats on social media: a multimedia research agenda. In: Proceedings of the 28th ACM international conference on multimedia, pp 4425–4434. 10.1145/3394171.3414692
- Vosoughi S, Roy D, Aral S. The spread of true and false news online. Science. 2018; 359 (6380):1146–1151. doi: 10.1126/science.aap9559. [ PubMed ] [ CrossRef ] [ Google Scholar ]
- Vraga EK, Bode L. Using expert sources to correct health misinformation in social media. Sci Commun. 2017; 39 (5):621–645. doi: 10.1177/1075547017731776. [ CrossRef ] [ Google Scholar ]
- Waldman AE. The marketplace of fake news. Univ Pa J Const Law. 2017; 20 :845. [ Google Scholar ]
- Wang WY (2017) “Liar, liar pants on fire”: a new benchmark dataset for fake news detection. arXiv preprint arXiv:1705.00648
- Wang L, Wang Y, de Melo G, Weikum G. Understanding archetypes of fake news via fine-grained classification. Soc Netw Anal Min. 2019; 9 (1):1–17. doi: 10.1007/s13278-019-0580-z. [ CrossRef ] [ Google Scholar ]
- Wang Y, Han H, Ding Y, Wang X, Liao Q (2019b) Learning contextual features with multi-head self-attention for fake news detection. In: International conference on cognitive computing. Springer, Berlin, pp 132–142. 10.1007/978-3-030-23407-2_11
- Wang Y, McKee M, Torbica A, Stuckler D. Systematic literature review on the spread of health-related misinformation on social media. Soc Sci Med. 2019; 240 :112552. doi: 10.1016/j.socscimed.2019.112552. [ PMC free article ] [ PubMed ] [ CrossRef ] [ Google Scholar ]
- Wang Y, Yang W, Ma F, Xu J, Zhong B, Deng Q, Gao J (2020) Weak supervision for fake news detection via reinforcement learning. In: Proceedings of the AAAI conference on artificial intelligence, pp 516–523. 10.1609/aaai.v34i01.5389
- Wardle C (2017) Fake news. It’s complicated. Online: https://medium.com/1st-draft/fake-news-its-complicated-d0f773766c79 . Accessed 3 Oct 2020
- Wardle C. The need for smarter definitions and practical, timely empirical research on information disorder. Digit J. 2018; 6 (8):951–963. doi: 10.1080/21670811.2018.1502047. [ CrossRef ] [ Google Scholar ]
- Wardle C, Derakhshan H. Information disorder: toward an interdisciplinary framework for research and policy making. Council Eur Rep. 2017; 27 :1–107. [ Google Scholar ]
- Weiss AP, Alwan A, Garcia EP, Garcia J. Surveying fake news: assessing university faculty’s fragmented definition of fake news and its impact on teaching critical thinking. Int J Educ Integr. 2020; 16 (1):1–30. doi: 10.1007/s40979-019-0049-x. [ CrossRef ] [ Google Scholar ]
- Wu L, Liu H (2018) Tracing fake-news footprints: characterizing social media messages by how they propagate. In: Proceedings of the eleventh ACM international conference on web search and data mining, pp 637–645. 10.1145/3159652.3159677
- Wu L, Rao Y (2020) Adaptive interaction fusion networks for fake news detection. arXiv preprint arXiv:2004.10009
- Wu L, Morstatter F, Carley KM, Liu H. Misinformation in social media: definition, manipulation, and detection. ACM SIGKDD Explor Newsl. 2019; 21 (2):80–90. doi: 10.1145/3373464.3373475. [ CrossRef ] [ Google Scholar ]
- Wu Y, Ngai EW, Wu P, Wu C. Fake news on the internet: a literature review, synthesis and directions for future research. Intern Res. 2022 doi: 10.1108/INTR-05-2021-0294. [ CrossRef ] [ Google Scholar ]
- Xu K, Wang F, Wang H, Yang B. Detecting fake news over online social media via domain reputations and content understanding. Tsinghua Sci Technol. 2019; 25 (1):20–27. doi: 10.26599/TST.2018.9010139. [ CrossRef ] [ Google Scholar ]
- Yang F, Pentyala SK, Mohseni S, Du M, Yuan H, Linder R, Ragan ED, Ji S, Hu X (2019a) Xfake: explainable fake news detector with visualizations. In: The world wide web conference, pp 3600–3604. 10.1145/3308558.3314119
- Yang X, Li Y, Lyu S (2019b) Exposing deep fakes using inconsistent head poses. In: ICASSP 2019-2019 IEEE international conference on acoustics, speech and signal processing (ICASSP), IEEE, pp 8261–8265. 10.1109/ICASSP.2019.8683164
- Yaqub W, Kakhidze O, Brockman ML, Memon N, Patil S (2020) Effects of credibility indicators on social media news sharing intent. In: Proceedings of the 2020 CHI conference on human factors in computing systems, pp 1–14. 10.1145/3313831.3376213
- Yavary A, Sajedi H, Abadeh MS. Information verification in social networks based on user feedback and news agencies. Soc Netw Anal Min. 2020; 10 (1):1–8. doi: 10.1007/s13278-019-0616-4. [ CrossRef ] [ Google Scholar ]
- Yazdi KM, Yazdi AM, Khodayi S, Hou J, Zhou W, Saedy S. Improving fake news detection using k-means and support vector machine approaches. Int J Electron Commun Eng. 2020; 14 (2):38–42. doi: 10.5281/zenodo.3669287. [ CrossRef ] [ Google Scholar ]
- Zannettou S, Sirivianos M, Blackburn J, Kourtellis N. The web of false information: rumors, fake news, hoaxes, clickbait, and various other shenanigans. J Data Inf Qual (JDIQ) 2019; 11 (3):1–37. doi: 10.1145/3309699. [ CrossRef ] [ Google Scholar ]
- Zellers R, Holtzman A, Rashkin H, Bisk Y, Farhadi A, Roesner F, Choi Y (2019) Defending against neural fake news. arXiv preprint arXiv:1905.12616
- Zhang X, Ghorbani AA. An overview of online fake news: characterization, detection, and discussion. Inf Process Manag. 2020; 57 (2):102025. doi: 10.1016/j.ipm.2019.03.004. [ CrossRef ] [ Google Scholar ]
- Zhang J, Dong B, Philip SY (2020) Fakedetector: effective fake news detection with deep diffusive neural network. In: 2020 IEEE 36th international conference on data engineering (ICDE), IEEE, pp 1826–1829. 10.1109/ICDE48307.2020.00180
- Zhang Q, Lipani A, Liang S, Yilmaz E (2019a) Reply-aided detection of misinformation via Bayesian deep learning. In: The world wide web conference, pp 2333–2343. 10.1145/3308558.3313718
- Zhang X, Karaman S, Chang SF (2019b) Detecting and simulating artifacts in GAN fake images. In: 2019 IEEE international workshop on information forensics and security (WIFS), IEEE, pp 1–6 10.1109/WIFS47025.2019.9035107
- Zhou X, Zafarani R. A survey of fake news: fundamental theories, detection methods, and opportunities. ACM Comput Surv (CSUR) 2020; 53 (5):1–40. doi: 10.1145/3395046. [ CrossRef ] [ Google Scholar ]
- Zubiaga A, Aker A, Bontcheva K, Liakata M, Procter R. Detection and resolution of rumours in social media: a survey. ACM Comput Surv (CSUR) 2018; 51 (2):1–36. doi: 10.1145/3161603. [ CrossRef ] [ Google Scholar ]
Home — Essay Samples — Sociology — Social Media — Social Media Is Harmful To Society

Social Media is Harmful to Society
- Categories: Consumerism Social Media
About this sample

Words: 528 |
Published: Mar 20, 2024
Words: 528 | Page: 1 | 3 min read
Table of contents
Mental health, social relationships.
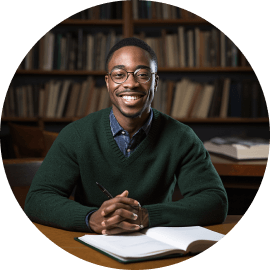
Cite this Essay
Let us write you an essay from scratch
- 450+ experts on 30 subjects ready to help
- Custom essay delivered in as few as 3 hours
Get high-quality help

Dr Jacklynne
Verified writer
- Expert in: Economics Sociology
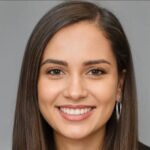
+ 120 experts online
By clicking “Check Writers’ Offers”, you agree to our terms of service and privacy policy . We’ll occasionally send you promo and account related email
No need to pay just yet!
Related Essays
3 pages / 1239 words
3 pages / 1269 words
4 pages / 1683 words
2 pages / 917 words
Remember! This is just a sample.
You can get your custom paper by one of our expert writers.
121 writers online
Still can’t find what you need?
Browse our vast selection of original essay samples, each expertly formatted and styled
Related Essays on Social Media
Social media has transformed the way we communicate, share information, and engage with the world. While it offers numerous benefits, it also poses significant challenges, including the spread of misinformation, threats to [...]
Rabin, Ruhani. 'Social Media - Impact on Society.' International Journal of Scientific and Research Publications, vol. 2, no. 5, May 2012.Subrahmanyam, K., and Smahel, D. 'Digital Youth: The Role of Media in Development.' [...]
Shannon, C. E., & Weaver, W. (1949). The Mathematical Theory of Communication. University of Illinois Press.Lasswell, H. D. (1948). The Structure and Function of Communication in Society. In L. Bryson (Ed.), The Communication of [...]
The era of social media has revolutionized how we connect, communicate, and share information. However, beneath the glossy filters and curated posts lies a pervasive issue - the prevalence of fake content. This essay delves into [...]
Many of us in this day and age can’t live without our cellphones and especially our social media accounts. We use our social media to get our news, to find out what events are going on in the world, in our communities, in our [...]
Social Media platforms such as Facebook, Twitter, Instagram and YouTube are the pinnacle of many of the current trends that we see in today’s society. This is something that the creative industries and in particular, the dance [...]
Related Topics
By clicking “Send”, you agree to our Terms of service and Privacy statement . We will occasionally send you account related emails.
Where do you want us to send this sample?
By clicking “Continue”, you agree to our terms of service and privacy policy.
Be careful. This essay is not unique
This essay was donated by a student and is likely to have been used and submitted before
Download this Sample
Free samples may contain mistakes and not unique parts
Sorry, we could not paraphrase this essay. Our professional writers can rewrite it and get you a unique paper.
Please check your inbox.
We can write you a custom essay that will follow your exact instructions and meet the deadlines. Let's fix your grades together!
Get Your Personalized Essay in 3 Hours or Less!
We use cookies to personalyze your web-site experience. By continuing we’ll assume you board with our cookie policy .
- Instructions Followed To The Letter
- Deadlines Met At Every Stage
- Unique And Plagiarism Free
IEEE Account
- Change Username/Password
- Update Address
Purchase Details
- Payment Options
- Order History
- View Purchased Documents
Profile Information
- Communications Preferences
- Profession and Education
- Technical Interests
- US & Canada: +1 800 678 4333
- Worldwide: +1 732 981 0060
- Contact & Support
- About IEEE Xplore
- Accessibility
- Terms of Use
- Nondiscrimination Policy
- Privacy & Opting Out of Cookies
A not-for-profit organization, IEEE is the world's largest technical professional organization dedicated to advancing technology for the benefit of humanity. © Copyright 2024 IEEE - All rights reserved. Use of this web site signifies your agreement to the terms and conditions.
More From Forbes
Social media-charged fraud waves: a new era of financial vulnerability.
- Share to Facebook
- Share to Twitter
- Share to Linkedin
In an era of nearly ubiquitous digital connectivity across all social strata, the intersection of universal banking technology and social media has given rise to a new take of financial vulnerabilities. Over the Labor Day weekend in the United States, a stark illustration of this phenomenon unfolded as Reddit forums, particularly those with an urban focus like Detroit's "CrimeInTheD," became epicenters of frenzied discussion surrounding a Chase Bank glitch.
The incident in question stemmed from an apparent system checking error that inadvertently allowed users to exploit a loophole in Chase Bank's deposit system. Customers discovered they could "kite" checks by making after-hours deposits, which would then become fully accessible the following day. This unintended flaw in the bank's infrastructure quickly transformed into a viral sensation, with individuals eagerly sharing photographic evidence of their illicit gains across various social media platforms.
As news of the glitch spread like wildfire, an impromptu industry of self-proclaimed financial "tutors" emerged on platforms such as TikTok. These opportunistic individuals offered step-by-step guidance through stories and instructional videos, promising to assist others in exploiting the glitch in exchange for a share of the ill-gotten proceeds. This rapid dissemination of information and techniques highlights the unprecedented speed at which financial vulnerabilities can be exposed and exploited in the digital age.
Best High-Yield Savings Accounts Of 2024
Best 5% interest savings accounts of 2024.
The timing of this incident, coinciding with a long bank holiday weekend, further exacerbated the situation. Financial institutions and retailers now face a dramatically compressed timeline for response and mitigation. What once may have afforded days for strategic planning and implementation has been reduced to mere hours, necessitating a paradigm shift in how these entities approach crisis management and security protocols.
The aftermath of such large-scale exploitation presents a complex web of legal, ethical, and practical challenges. While banks have the option to send affected accounts into overdraft and pursue collections, the feasibility of recovering potentially millions of dollars from individuals who likely lack the means to repay is questionable at best. The scale of the incident also raises significant logistical hurdles for law enforcement. The prospect of mass arrests seems both impractical and potentially counterproductive, given the strain it would place on the criminal justice system and the societal implications of such widespread prosecution.
As we move forward, it is clear that the frequency and sophistication of such hacks and system failures will only increase, driven by the ever-accelerating pace of information sharing and technological advancement. Financial institutions must invest heavily in robust security measures and agile response systems to stay ahead of potential vulnerabilities. Moreover, there is a pressing need for enhanced digital literacy education to help the public understand the legal and ethical implications of exploiting such glitches, even when they appear to offer easy financial gain.
Others have cited this as a class problem but I believe it's more a symptom of simple opportunist coupled with peer encouragement. It's a stark reminder of the evolving landscape of crime and punishment in the digital age. In a way it's a digital mob attacking a business where they were discovered to be weak the same way of a store left the till unlocked and people figured out they could come in and take money.
It underscores the need for a multifaceted approach involving financial institutions, technology companies, law enforcement agencies, and policymakers to develop comprehensive strategies for preventing, detecting, and responding to such incidents. A balance between technological innovation and security will be crucial in maintaining the integrity of our financial systems and the trust of the public they serve.
The glitch and its aftermath represent a fascinating case study in the intersection of technology and human behavior. But in the meantime, hey, "make sum bred, uncle"
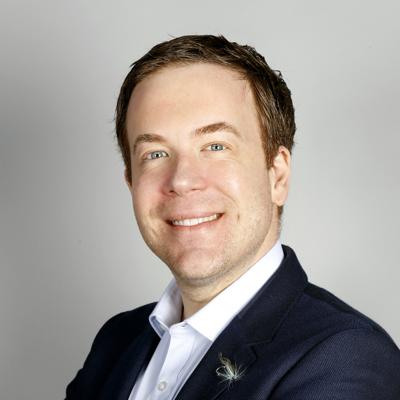
- Editorial Standards
- Reprints & Permissions
Join The Conversation
One Community. Many Voices. Create a free account to share your thoughts.
Forbes Community Guidelines
Our community is about connecting people through open and thoughtful conversations. We want our readers to share their views and exchange ideas and facts in a safe space.
In order to do so, please follow the posting rules in our site's Terms of Service. We've summarized some of those key rules below. Simply put, keep it civil.
Your post will be rejected if we notice that it seems to contain:
- False or intentionally out-of-context or misleading information
- Insults, profanity, incoherent, obscene or inflammatory language or threats of any kind
- Attacks on the identity of other commenters or the article's author
- Content that otherwise violates our site's terms.
User accounts will be blocked if we notice or believe that users are engaged in:
- Continuous attempts to re-post comments that have been previously moderated/rejected
- Racist, sexist, homophobic or other discriminatory comments
- Attempts or tactics that put the site security at risk
- Actions that otherwise violate our site's terms.
So, how can you be a power user?
- Stay on topic and share your insights
- Feel free to be clear and thoughtful to get your point across
- ‘Like’ or ‘Dislike’ to show your point of view.
- Protect your community.
- Use the report tool to alert us when someone breaks the rules.
Thanks for reading our community guidelines. Please read the full list of posting rules found in our site's Terms of Service.
Social Security
Protect yourself from scams ( en español ).
Be on the lookout for fake calls, texts, emails, websites, messages on social media, or letters in the mail
Report a Social Security-related scam
This is brought to you by the Social Security Administration and its Office of the Inspector General.
March 28, 2024 Don’t hand off cash to “agents.” This new scam trend introduces an element of physical danger to scams that never existed before. Read more .
FTC Video: Hang Up on Social Security Scam Calls
See All Social Security-related Scam Alerts
What Are Social Security-Related Scams?
Criminals continue to impersonate SSA and other government agencies in an attempt to obtain personal information or money.
Scammers might call, email, text, write, or message you on social media claiming to be from the Social Security Administration or the Office of the Inspector General. They might use the name of a person who really works there and might send a picture or attachment as “proof.”
Social Security employees do contact the public by telephone for business purposes. Ordinarily, the agency calls people who have recently applied for a Social Security benefit, are already receiving payments and require an update to their record, or have requested a phone call from the agency. If there is a problem with a person’s Social Security number or record, Social Security will typically mail a letter.
Four Basic Signs of a Scam
Recognizing the signs of a scam gives you the power to ignore criminals and report the scam.
Scams come in many varieties, but they all work the same way:
- Scammers pretend to be from an agency or organization you know to gain your trust.
- Scammers say there is a problem or a prize.
- Scammers pressure you to act immediately.
- Scammers tell you to pay in a specific way.
Known Tactics Scammers Use
Scammers frequently change their approach with new tactics and messages to trick people. We encourage you to stay up to date on the latest news and advisories by following SSA OIG on LinkedIn, Twitter, and Facebook or subscribing to receive email alerts.
These are red flags; you can trust that Social Security will never
- Suspend your Social Security number.
- Claim to need personal information or payment to activate a cost-of-living adjustment (COLA) or other benefit increase.
- Pressure you to take immediate action, including sharing personal information.
- Ask you to pay with gift cards, prepaid debit cards, wire transfers, cryptocurrency, or by mailing cash.
- Threaten to seize your bank account.
- Offer to move your money to a “protected” bank account.
- Demand secrecy.
- Direct message you on social media.
Be skeptical and look for red flags. If you receive a suspicious call, text message, email, letter, or message on social media, the caller or sender may not be who they say they are. Scammers have also been known to:
- Use legitimate names of Office of Inspector General or Social Security Administration employees.
- “Spoof” official government phone numbers, or even numbers for local police departments.
- Send official-looking documents by U.S. mail or attachments through email, text, or social media message.
Fraudsters create imposter social media pages and accounts using Social Security-related images and jargon. This helps them appear as if they’re associated with or endorsed by Social Security. The imposter pages could be for the agency or Social Security and OIG officials. The user is asked to send their financial information, Social Security number, or other sensitive information. Social Security will never ask for sensitive information through social media as these channels are not secure.
Here are some ways to spot an imposter page:
- Number of followers.
- Incorrect punctuation or spelling.
- Links to pages not on ssa.gov.
- Advertisements for forms or other SSA documents.
- Incorrect social media handle. To view the list of Social Security’s official social media channels, we encourage you to visit www.ssa.gov/socialmedia
It is illegal to reproduce federal employee credentials and federal law enforcement badges. Federal law enforcement will never send photographs of credentials or badges to demand any kind of payment, and neither will federal government employees.
Report the scam.
How to Avoid a Scam
Protect yourself, friends, and family — If you receive a suspicious call, text, email, social media message, or letter from someone claiming to be from Social Security:
- Remain calm . If you receive a communication that causes a strong emotional response, take a deep breath. Talk to someone you trust.
- Hang up or ignore the message . Do not click on links or attachments.
- Protect your money . Scammers will insist that you pay with a gift card, prepaid debit card, cryptocurrency, wire transfer, money transfer, or by mailing cash. Scammers use these forms of payment because they are hard to trace.
- Protect your personal information . Be cautious of any contact claiming to be from a government agency or law enforcement telling you about a problem you don’t recognize, even if the caller has some of your personal information.
- Spread the word to protect your community from scammers.
- Report the scam to the Office of the Inspector General at oig.ssa.gov/report .
How to Report
When you report a scam, you are providing us with powerful data that we use to inform others, identify trends, refine strategies, and take legal action against the criminals behind these scam activities.
Report a scam
If you are unsure about the type of scam, but want to report it, visit USA.gov’s Where To Report a Scam . The tool will help you to find the right place to report a scam.
What to Do if You Were Scammed
Recovering from a scam can be a long and difficult process. Here are some reminders:
- Do not blame yourself. Criminal behavior is not your fault.
- Stop contact with the scammer. Do not talk to them or respond to their messages.
- Notify the three major credit bureaus: Equifax , Experian , and TransUnion to add a fraud alert to your credit report.
- Protect your Social Security Number .
- Request a replacement SSN card or new SSN , if necessary.
The Federal Trade Commission’s “What To Do if You Were Scammed” article has information about what to do if you paid someone you think is a scammer or gave a scammer your personal information or access to your computer or phone.
Additionally, the Federal Trade Commission provides assistance in multiple languages. The Federal Trade Commission’s “New Help for Spotting, Avoiding, and Reporting Scams in Multiple Language” and “Consumer Education in Multiple Languages” has information about reporting and avoiding scams in your preferred language.
Help Us “Slam the Scam”!
Please visit our Resources page for more information on how you can help us “Slam the Scam”.

About the Social Security Administration Office of the Inspector General
The Social Security Administration Office of the Inspector General has independent oversight of SSA’s programs and operations. SSA OIG is responsible for conducting audits, evaluations, and investigations and reporting on and providing recommendations for programs, operations, and management improvements.
How-To Geek
8 compelling reasons to quit social media for your own good.

Your changes have been saved
Email is sent
Email has already been sent
Please verify your email address.
You’ve reached your account maximum for followed topics.
How to Get Google to Pay for Your Android Apps
Learn python basics: let's build a simple expense tracker, the new outlook for windows is getting more improvements, quick links.
- I Have Improved My Mental Health
- My Self-Esteem Has Increased
- I Have Cut Down on Screen Time
- I Am More Productive Now
- I Have Reduced Impulsive Spending
- I Focus on New Hobbies and Skills Building
- My Privacy Remains Protected
- I Am Less Concerned About Identity Theft
While social media platforms make it easy to stay connected with friends, they also bring a range of downsides. It can affect your mental health, reduce productivity, encourage impulsive spending, and more. I'll share some of the reasons why I took a step back from social media and how doing so has improved my life.
1 I Have Improved My Mental Health
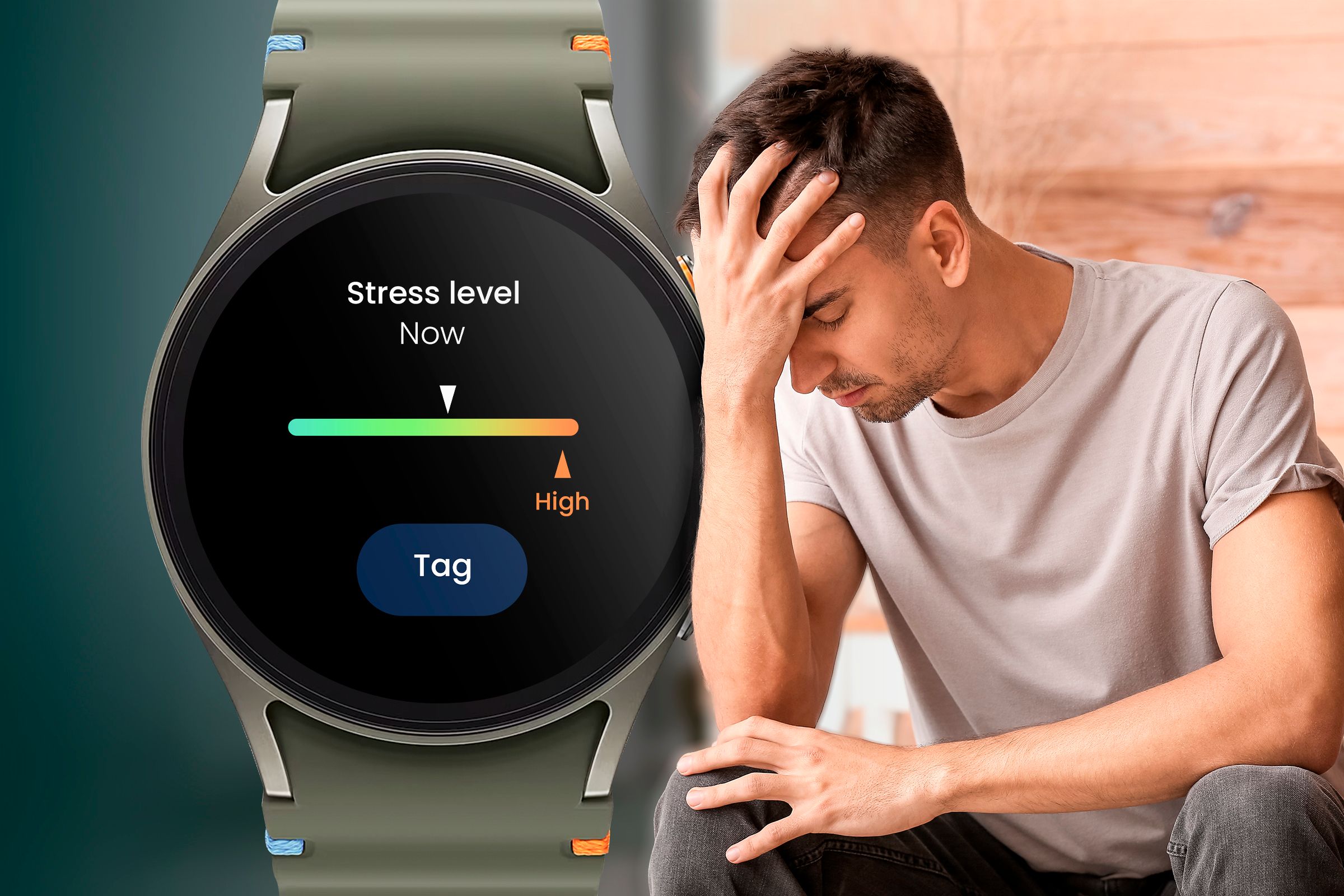
I've noticed a significant improvement in my mental health since quitting social media. I used to constantly dwell on bad arguments and mindlessly scroll through my feed for hours, all of which took a toll on my mental well-being. I also used to compare my life to others and stress over things beyond my control.
Since leaving social media, I've freed myself from the pressure of keeping up with others. The constant cycle of comparison has ended, and my mind now has the space to relax. I feel much less stressed, which clearly shows my mental health has improved. If you want to reclaim your mental well-being, I highly recommend taking a break from social media.
2 My Self-Esteem Has Increased
On social media, everyone showcases their best side, which used to give me the illusion that all my friends and the celebrities I followed had perfect lives. Seeing filtered images, glamorous lifestyles, and constant success made me compare myself to them. This led to self-doubt and a sense of inadequacy, even though I was working hard to improve my life.
It took me some time to realize that people only present a polished version of themselves online, and behind the scenes, no one’s life is truly perfect. Since quitting social media, I’ve redirected my focus toward my personal goals. I feel more confident, have improved my self-esteem, and appreciate my journey. I strive to be better than I was the day before.
3 I Have Cut Down on Screen Time
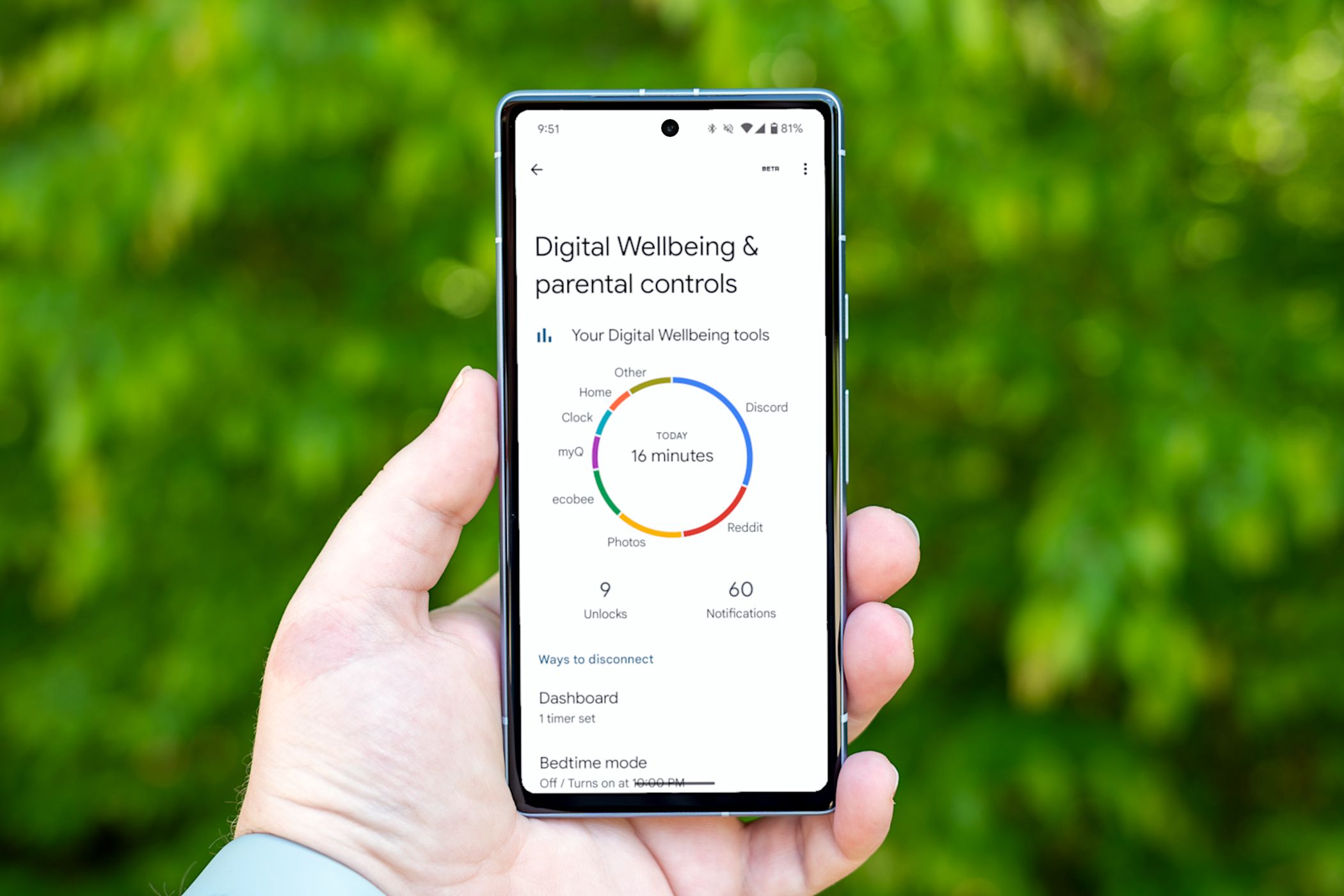
To manage IBS , one of my doctor’s recommendations was to reduce my screen time. However, the addictive nature of social media made this difficult. Every day, social media apps used to dominate my screen time. I also started to experience eye strain and neck pain from poor posture. My habit of scrolling before bed also disrupted my sleep cycle.
Quitting social media has drastically reduced my screen time. Now, I have more time to spend with loved ones and am more present in the moment. My friends no longer complain about me being glued to my phone when we’re together. Instead of scrolling before bed, I now read a book that helps my melatonin levels regulate, helping me enjoy a restful night’s sleep.
4 I Am More Productive Now
Social media used to be a big distraction in my life. What often began as a quick notification check used to spiral into hours of mindless scrolling, causing me to lose track of time and waste large portions of my day. Even after leaving social media, my mind used to fixate on waiting for replies on the posts I had commented on.
This habit negatively affected my freelance work. Tasks that should have taken just a few hours used to consume my entire day.
Quitting social media eliminated this distraction . Now, I’ve redirected my energy toward my work, with my full mental focus dedicated to it. Without constant notification pings, I can concentrate much better and complete tasks on time. If you’re also struggling with productivity, cutting off social media could make a noticeable difference.
5 I Have Reduced Impulsive Spending
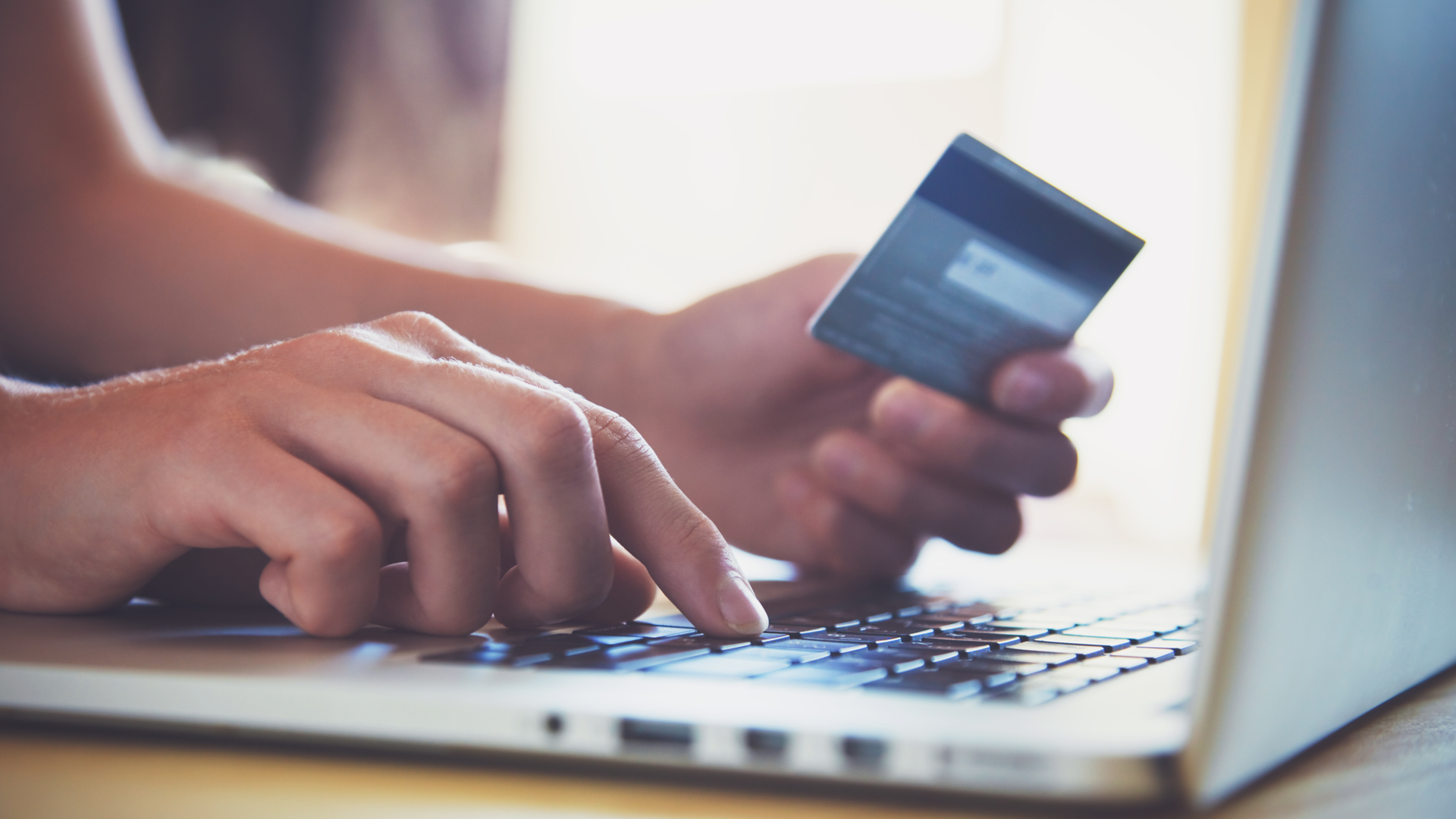
Have you ever mentioned a product to a friend or family only to see an advertisement for it on social media? The constant temptation from these personalized ads used to lead me to make impulsive purchases that I later regretted. Influencers promoting trendy products also used to push me to buy things I didn’t really need.
The ease of online shopping made it even worse—a few clicks, and the item was on its way. This habit was straining my budget. Since quitting social media, I’ve significantly cut back on my monthly spending. Now, I only make intentional purchases and buy things I genuinely need rather than what catches my eye.
6 I Focus on New Hobbies and Skills Building
Social media used to consume so much of my time that I struggled to complete essential tasks. Since quitting, I now have plenty of time , even after finishing the daily to-do lists I have in mind. The hours I once spent mindlessly scrolling are now devoted to my hobbies. It has given me the fulfillment I had been missing from passively consuming content.
With this social media detox, I now have the mental space to learn new skills to help me grow in my career. I now feel like I'm living a more balanced and fulfilling life.
7 My Privacy Remains Protected
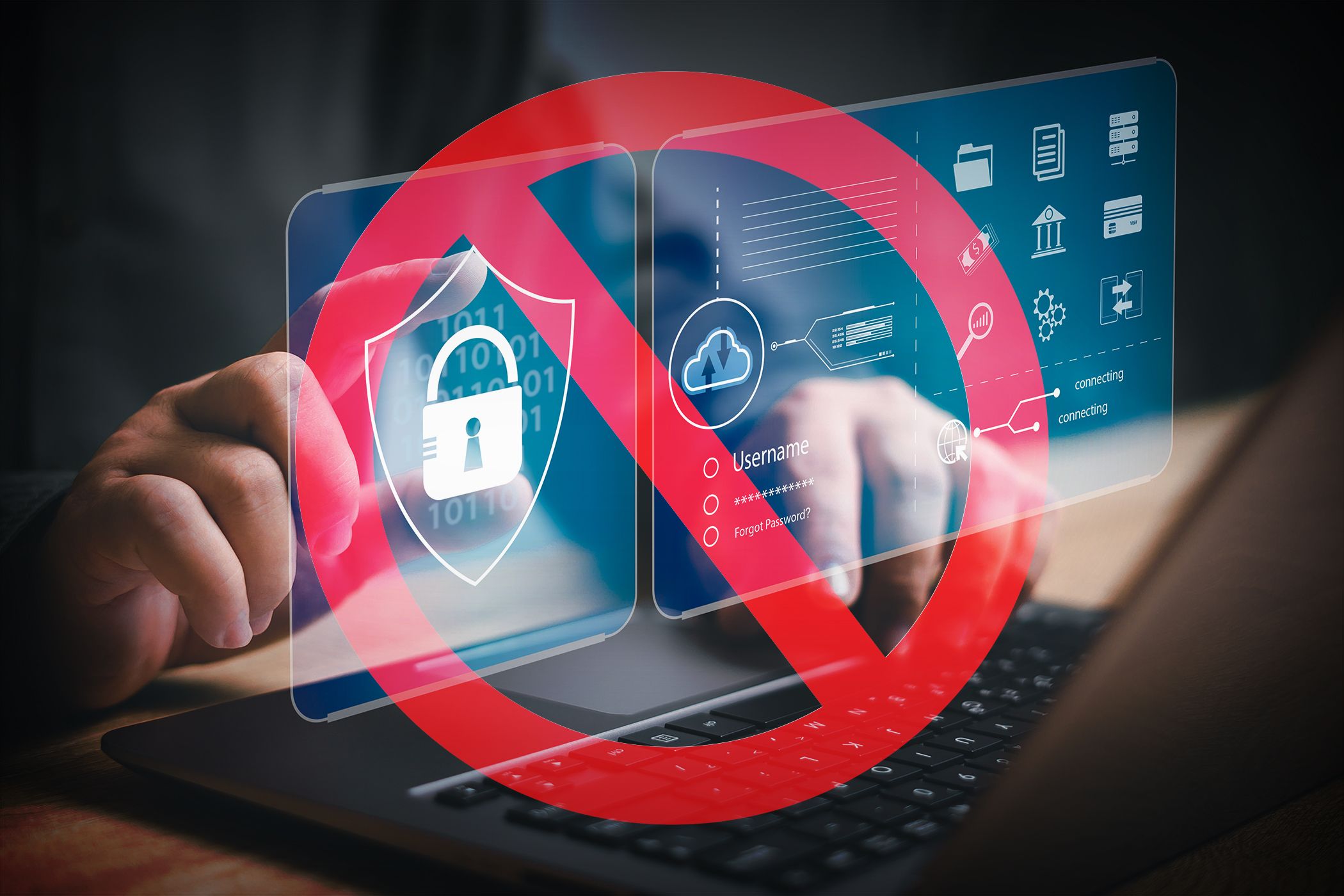
Social media algorithms track our activities and gather vast amounts of personal data. Most of us accept the terms and conditions without fully understanding what type of data these platforms collect. They use this information to display targeted ads or even sell it to third parties—something we can't be sure about. This used to be a constant concern for me.
Since limiting my online presence , I no longer have to worry about my data being collected. Because of this, I'm now less vulnerable to data breaches and other cybersecurity threats. Also, by not sharing my trips, relationships, and other personal activities, I keep my private life away from public view, even from friends.
8 I Am Less Concerned About Identity Theft
I’ve experienced identity theft a few times where scammers copied my profile data and impersonated me to deceive my contacts. They asked for financial help under my name, and some friends even fell for their schemes. This was an ongoing struggle, as scammers used to create new profiles whenever I reported and had their previous ones blocked.
Before quitting social media, I informed all my friends about my departure so they would recognize any new profile, messaging them as a potential impersonator.
I left social media for the reasons outlined above, and it has positively transformed my life. If social media platforms also consume your time and affect your life, you should seriously consider whether it's time to disconnect. If you find my reasons convincing, taking a break from social media apps or limiting their use could positively change your daily life.
- Social Media
- Apps & Web Apps
- Kreyòl Ayisyen
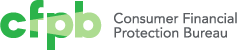
Can a debt collector contact me through social media?
A debt collector can contact you on social media, but they must follow certain rules and tell you how you can opt out of social media communications.
The message must be private.
A debt collector can only communicate with you on social media platforms about a debt if the message is private. A debt collector cannot contact you on social media about a debt if the message is viewable by the general public or viewable by your friends, contacts, or followers on the platform. This would include your publicly visible profile page or any part of the platform where other people can see the message.
The debt collector must identify themself.
If a debt collector attempts to send you a private message requesting to add you as a friend or contact, the debt collector must identify themself as a debt collector.
They must include a way for you to stop receiving messages from them.
Even when a debt collector properly identifies themself in a private social media message, they must give you a simple way to opt out of receiving further communications from them on that social media platform.
Learn more about what to do if a debt collector contacts you , and the rules they must follow.
If you're having an issue with debt collection, you can submit a complaint with the CFPB online or by calling (855) 411-CFPB (2372) .
Don't see what you're looking for?
Browse related questions.
- What should I do when a debt collector contacts me?
- What is harassment by a debt collector?
- What laws limit what debt collectors can say or do?
- Learn more about debt collection
Search for your question
Searches are limited to 75 characters.

IMAGES
VIDEO
COMMENTS
More than 95,000 people reported about $770 million in losses to fraud initiated on social media platforms in 2021. [3] Those losses account for about 25% of all reported losses to fraud in 2021 and represent a stunning eighteenfold increase over 2017 reported losses. Reports are up for every age group, but people 18 to 39 were more than twice ...
Social media was the top contact method ranked by fraud loss reports for all age groups under age 70, while phone call was the top contact method for the 70-79 and 80 and over age groups. 5 The top undelivered items were identified by hand-coding a random sample of 400 reports that contained a narrative description identifying the items ordered.
Social media has become an undeniable force in our lives, particularly for students. While it offers a platform for connection, information, and self-expression, it also presents a landscape ripe for fraudulent activity. Social media platforms have transformed the way we connect, communicate, and consume content.
Stories about scams are a weekly occurrence in the popular media, and scams have become one of the most common crimes globally. One report estimated the financial cost of fraud to the global economy at over $5 trillion per year (Gee & Button, 2019), almost 50% higher than the 2019 U.S. budget (about $3.5 trillion).
According to the Federal Trade Commission, consumers in this country lost nearly $8.8 billion to a wide variety of online, phone call initiated, and in-person scams in 2022 alone - via phishing attacks, Ponzi schemes, identity and credit card theft, investment scams, online shopping scams, romance fraud, sextortion, and other deceptive ...
Social media was the top contact method ranked by fraud loss reports for all age groups under age 70, while phone call was the top contact method for the 70-79 and 80 and over age groups. [5] The top undelivered items were identified by hand-coding a random sample of 400 reports that contained a narrative description identifying the items ordered.
s or known brands.Scams can spread with alarming speed across social media, as likes, shares and retweets propagate content to a wide. ange of audiences. In effect, the social media model allows scammers to sit back and let consumers, albeit involuntarily, do muc. 2 We Are Social, Global Digital Snapshot.
The paper explores why victims fall for online scams. It identifies a range of reasons including: the diversity of frauds, small amounts of money sought, authority and legitimacy displayed by scammers, visceral appeals, embarrassing frauds, pressure and coercion, grooming, fraud at a distance and multiple techniques.
Social media fraud is exploding: Per the FTC, 1 in 4 scams start on social media. Younger adults aged 18-39 were more than twice as likely as older adults to report losing money to social media ...
Abstract. Deception is a pervasive feature of the online marketplace: from phone calls by fake tech support workers at Microsoft, to fraudulent emails asking for advance fee payment, and fake postings for jobs on employment platforms. Building off interdisciplinary discussions within science and technology studies (STS), this special issue ...
Don't get scammed. Do this instead: If a quiz starts asking strange questions, stop there. Don't answer further questions, and immediately report the account to the social media platform. 9. Lottery, sweepstakes, and giveaway scams. In this type of scam, fraudsters DM you to say you've won a prize.
A new wave of online threats and attacks has been brought on by the growth of social media. Individuals and companies are becoming increasingly vulnerable to internet risks, such as. bullying ...
sample includes articles that were shared a total of 1.6 million times on Facebook before any. of the advertising bans, a decrease of 75% equates to a decline in total shares of 1.12 million. for the fake news sites in our sample. Second, we calculate a benchmark of how referrals from Facebook to fake news sites.
The majority of previous research conducted in this area predominantly focus on the persuasive influence of the scam message employed by the fraudster (see Chang and Chong 2010) or the knowledge of scams held by the potential victim (see Harrison et al. 2016a).The purpose of this systematic review is to extend that focus to incorporate variables related to individual psychological differences ...
Case Study #3: Facebook Security Scam. While the first two examples were intended as (relatively) harmless pranks, this next instance of social media fraud was specifically designed to separate social media users from their money. In 2012, a scam involving Facebook developed as an attempt to use social media to steal financial information from ...
Document analysis is conducted on data from dating sites, news and media sites, anti-scam commissions, law enforcement agencies, and government agencies, from 2000 to 2009.
Division of Consumer & Business Education. October 21, 2020. Scammers are hiding out on social media, using ads and offers to market their scams, according to people's reports to the FTC and a new Data Spotlight. In the first six months of 2020, people reported losing a record high of almost $117 million to scams that started on social media.
Many of the frauds that show up on social media are not unique to the Internet. These frauds range from "pump and dump" schemes to promises of "guaranteed returns," from "High Yield Investment Programs" to affinity fraud. To learn more about these frauds, see Types of Fraud. Many investors use the Internet and social media to help ...
At Get Safe Online, we applaud social media for its many positives. Report fraud to Action Fraud at www.actionfraud.police.uk or on 0300 123 2040. With social media sites being more popular than ever, it's important to make sure you are taking the necessary precautions and being social media smart. Get Safe Online offers their tops tips and ...
Social media outperformed television as the major news source for young people of the UK and the USA. 10 Moreover, as it is easier to generate and disseminate news online than with traditional media or face to face, large volumes of fake news are produced online for many reasons (Shu et al. 2017).Furthermore, it has been reported in a previous study about the spread of online news on Twitter ...
Mental Health. One of the most significant ways in which social media is harmful to society is through its negative impact on mental health. Research has shown that excessive use of social media is linked to increased rates of anxiety, depression, and other mental health disorders. The constant exposure to curated and often unrealistic ...
Facebook, Twitter, Instagram, and LinkedIn are all popular online social media sites these days. Everyone uses different social media platforms, from children to adults. The use of these social media applications is increasing, which leads to a rise in social media crime. Here is where the word "fake profiles" comes into play; these fake profiles are responsible for distributing misleading ...
However, using social media platforms may come with some risks if you aren't careful enough. And the reason for this is social media scams. 1 in 4 Americans who reported losing money to scams since 2021 said it began on social media, according to the Federal Trade Commission. Reported losses during this period reached an astounding $2.7 billion.
In an era of nearly ubiquitous digital connectivity across all social strata, the intersection of universal banking technology and social media has given rise to a new take of financial ...
Social Media Frauds; Social Media Frauds. Improved Essays. 1274 Words; 5 Pages; Open Document. ... Argumentative Essay On Social Media Pros And Cons. 1117 Words; ... and we are using it in our daily activities as part of our life. Social media such as Facebook, MySpace, Twitter, YouTube, and Instagram are some of the popular social media sites ...
Direct message you on social media. Be skeptical and look for red flags. If you receive a suspicious call, text message, email, letter, or message on social media, the caller or sender may not be who they say they are. Scammers have also been known to: Use legitimate names of Office of Inspector General or Social Security Administration employees.
The sheriff's office said they've seen these scams on local social media pages. What's worse, these fake posts can take away from the search for real missing children. "We have thousands of missing children on our website that could use that same type of attention," said John Bischoff, with the National Center for Missing and Exploited ...
To manage IBS, one of my doctor's recommendations was to reduce my screen time.However, the addictive nature of social media made this difficult. Every day, social media apps used to dominate my screen time. I also started to experience eye strain and neck pain from poor posture. My habit of scrolling before bed also disrupted my sleep cycle.
A number of viral TikTok videos had some people believing they could get "free" cash from Chase ATMs. But it was just a glitch - and those customers were actually committing fraud, according ...
The message must be private. A debt collector can only communicate with you on social media platforms about a debt if the message is private. A debt collector cannot contact you on social media about a debt if the message is viewable by the general public or viewable by your friends, contacts, or followers on the platform.